
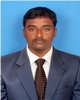
- Open Access
- Authors : Veeramani. S
- Paper ID : IJERTV9IS020335
- Volume & Issue : Volume 09, Issue 02 (February 2020)
- Published (First Online): 03-03-2020
- ISSN (Online) : 2278-0181
- Publisher Name : IJERT
- License:
This work is licensed under a Creative Commons Attribution 4.0 International License
Gaja Cyclone Induced Changes to Plantation Crops using NDVI Differencing and Classification Methods
Veeramani. S1
GIS Specialist,
Kerala forests and Wildlife Department, Periyar Tiger Conservation Foundation , Periyar Tiger Reserve, Thekkady-685509
Abstract:- A cyclone is one of the major hazards around the coastal area which leads to loss of life, damage to property and drastic changes in land use and land cover (LULC). After the disaster, the country must develop various mitigation plans to rehabilitate the affected area. The present research brings out the LULC changes and the major transitions observed during the Gaja cyclone occurred on 10th November 2018 in East coastal region of Tamilnadu and Puducherry, India. The normalized difference vegetation index (NDVI) differencing method and classification method are commonly used as a change detection method and provide detailed information for detecting and monitoring changes in land use- land cover (LULC). So in the presented study to raise responsiveness for the LULC change in Gaja cyclone-affected areas, two sentinel-2 images of the years 2018 and 2019 have been prepared and used to obtain NDVI images and execute image classification. At the initial stage, differences between two correspondent NDVI images of the area were calculated. From the results, very high vegetation regions NDVI values have decreased by about more than 5 % from pre-cyclone to post-cyclone. At the subsequent stage, a supervised classification was performed and outputs of the two time periods were compared to derive information on changes that occurred over a period of time. During the study period, plantation crops were decreased from 17.4 % of the total land in pre-cyclone to 6.8% in post-cyclone.
Keywords: LULC classification, Gaja Cyclone, Change Detection, NDVI Differencing Sentinel-2, plantation crops
INTRODUCTION
Remote sensing has a huge impact on disaster management; previously this technology has been used in explanation of impacts caused by disasters like earthquakes, tsunamis, hurricanes, floods, wildfires. Now the development in this field reduces the complicated strategies, which is previously followed in disaster management, through high resolution image we estimate the post disaster damage, monitoring recovery and mitigation activities.
Gaja cyclone was occurred in Tamilnadu and Puducherry coastal districts (i.e. Tiruvarur, Nagapatinam and karaikal) on 11th November 2018.the low pressure originated from Gulf of Thailand intensified into depression over Bay of Bengal on November 10 and further intensified to gaja cyclone. The highest wind gust was recorded in Athiramapattinam at 181km/h; 45 peoples were died,
18000 hectares of coconut trees were damaged, mostly uprooted.
In this study we find the area of plantation crops affected due to gaja cyclone in coastal districts of Tamilnadu and Puducherry using remote sensing methods, plantation crops play an important role in domestic requirements and employment generation in rural sector.
There are several methods for detection of changes in land cover and land use using geospatial data:
-
Supervised and unsupervised classification.
-
NDVI differencing.
-
Principle component analysis.
-
Image differencing.
-
Multi date unsupervised classification.
From this method we have chosen the supervised classification methods and NDVI differencing for our proposed research. The NDVI differencing method is common and successful in change detection of vegetation changes (D. Lu et al, 2004). The main theme of this method is vegetation index produced separately, and then the second-date vegetation index is subtracted from the first-date vegetation index. It has merits of emphasize differences in the spectral response of different features and reduces impact of topographic effects and illumination while the demerits are enhanced random noise or coherent noise (Y.Li et al,2001, D.Lu,et al 2005).
The post-classification method is commonly used to calculate changes. This method involves comparative analysis of independent spectral classifications of images acquired on two different dates (A. Singh,1989). It is characterized by easy calculation and provides from-to change information (G.M. Foody , 2002). It also has equal capability of mapping the kind of landscape transformation that has occurred between the two dates under NDVI Differencing and Post-classification to Detect Vegetation Changes in Halabja City, Iraq It is worth mentioning, however, that the overall accuracy of the product depends on the accuracy of the individual classification (J. Jensen,2005).
The present study aims to assess the pre and post disaster changes to plantation crops in cyclone affected area in East coastal region of Tamilnadu and puducherry. Research was
carried out based on LULC changes between two temporal satellite images. The present study includes mapping of natural changes that have occurred in the study area for the September 2018 (Pre- cyclone), and March 2019 (Post- cyclone)
OBJECTIVE
The main objective of the present paper is to find the plantation crops changes due to gaja cyclone (10 November 2018) using NDVI differencing and supervised Classification in coastal Districts of Tamilnadu and Pondicherry, India
MATERIALS AND METHODS
Study Area
Study area includes three districts from Tamilnadu and puducherry. District wise Geography profile was given below:
Sl.no
District
latitude
longitude
Temperature (max)
Area (sq.km)
State
1.
Nagapatinam
10.790 N
79.840 E
320 C
2715.83
Tamilnadu
2.
Tiruvarur
11.070 N
79.450 E
35.190C
2377
Tamilnadu
3.
Karaikal
11.010 N
79.710 E
280 C
161
Puducherry
MATERIALS AND METHODS
Sentinel-2 Data
Fig .1. Study Area Location
Top-of-Atmosphere reflectance products from the Scientific Hub (https://scihub.copernicus. eu): one relative
Sentinel-2 data have 13 bands with various resolutions like 10m, 20 m, and 60m. (Table.1) at 12-bit radiometric resolution. For the remainder of the analysis, we focused only on 10 m and 20 m bands (Table 1). The three 60 m spatial resolution bands were not used in this study because they are primarily relevant for atmospheric corrections. In this work, three S2 products were downloaded as Level-1C
to pre gaja cyclone and another one is post gaja cyclone image., product codes are in the (Table 2). The products were resample at a resolution of 10 m by the Sentinel Application Platform (SNAP), available at the ESA website (http://step.esa.int/ main/toolboxes/snap). Finally, the 10 bands were imported in ENVI software, stacked and cropped over the area of interest.
SENTINEL -2
BANDS
WAVELENGTH
SPATIAL RESOLUTION
Band-1
Coastal aerosol
0.443
60
Band-2
Blue
0.490
10
Band-3
Green
0.560
10
Band-4
Red
0.665
10
Band-5
Red Edge
0.705
20
Band-6
Red Edge
0.740
20
Band-7
Red Edge
0.783
20
Band-8
NIR
0.842
10
Band-8A
Red Edge
0.865
20
Band-9
Water vapor
0.945
60
Band-10
SWIR-Cirrus
1.375
60
Band-11
SWIR
1.610
20
Table 1 -spectral bands available from sentinel-2. Only bands
With finer spatial resolution (i.e. 10 m and 20 m) have been used in this work
S2 product code No
Time period
Date
S2B_MSIL1C_20180908T045649_N0206_R119_T44P_20180908T084331
Pre-cyclone
8-9-2018
S2B_MSIL1C_20190307T045659_N0207_R119_T44P_20190307T085605
Post cyclone
7-3-2019
Table .2. Details of Sentinel 2 Image
Fig .2. Sentinel-2 FCC Image (8-9-2018-Pre Cyclone)
Fig .2.1 Sentinel-2 FCC Image (7-3-2019-Post Cyclone)
METHODOLOGY:
Fig .3. Methodology
Multi temporal satellite data set observed by sentinel-2 dated on 8 September 2018 (Fig.2) and 07 March 2019 (Fig 2.1), Multi Spectral Imager (MSI), were used for the analysis (Table 2). The resolution is 10 meters/pixel. At first stage, the NDVI data layer was generated from sentinel-2 images in ENVI 5.3 environment. Band math was then performed on the resulting NDVI images by subtracting the 2019 image values from the 2018 image values to find the areas where the land cover has changed. The resultant image was threshold based on 10% changes and the areas with 10% increase or decrease in NDVI values were demonstrated. Finally, in order to the investigation of changes in NDVI values, the NDVI- change image was density sliced to the 4 categories included: areas with low, medium, high, and very high NDVI values. At the second stage, in order to investigate the changes in each land use / land cover classification through supervised classification method, based on the field knowledge is employed to perform the classification. Arc GIS 10.2 and ENVI 5.3 are powerful tools for extracting the land use, land cover layer, from satellite imageries.
The land use land cover classes include agriculture land, harvested land, and wasteland, forest, built up (settlement, road), water bodies and cloud cover areas. This classification is performed based on the classification scheme of National Remote Sensing Center (NRSC),
Department of Space, and Govt of India. Maximum likelihood classification was implemented, the results are validated with ground truth points, after assessing the accuracy we extract statistical information based on pixel count and then find the difference between two statistical outputs and finally we get results of changes happened due to gaja cyclone for each land use and land cover features.
RESULTS AND DISCUSSION
Once the option of change detection classification is determined, decision on the data processing necessities can be made. Requirements include geometric/radiometric corrections, data normalization, image enhancement, image classification and classification accuracy assessment (Lunetta and Elvidge, 1998).
Accurate per-pixel registration of multi-temporal remote sensing data is essential for change detection since the potential exists for registration errors to be interpreted as land-cover-land use change, leading to an overestimation of actual change (Stow, 1999). In the present study, DN value of NDVI images are categorized as low density from 0.1 to 0.2, medium density from 0.2 to 0.3, high density from 0.3 to 0.4 and very high density from 0.4 and more. Table.3 .shows the NDVI density classes in the date of 8-9-2018 and 7-3-2019. As it can be seen in this Table and Fig.4.
Fig .4. NDVI images of pre and post cyclone.
The most important changes have occurred in low and very high density classes. The category of very high NDVI density has reduced from 18.51% in pre cyclone to about 15.9% in post cyclone. Similarly the category of low NDVI density has reduced from 28.6 to 18.7 %. The Fig.4.represent the NDVI density map of the study area in
pre and post cyclone respectively. Band math was then performed on the resulting NDVI images by subtracting the post cyclone image values from the pre cyclone image values and the resulting image gave the changes in the vegetation amount and status occurred between the two time periods for every image pixels.
As shown in the Fig.4.1, the NDVI value decreased from pre cyclone to post cyclone for low and very high density classes but increased for the medium and high density classes. Differences between two correspondent NDVI images of the area acquired in two
different periods were calculated and the resulting image gave the changes in the vegetation amount and status occurred between two different times. In order to enhance the changes displayed, the 10% change thresholds were fixed on the resulting values.
NDVI density classes
Pre cyclone
Post cyclone
Change between pre and post cyclone
Sq.km
%
Sq.km
%
Sq.km
%
No vegetation
599.49
11.6
461.73
8.9
-137.76
-29.8
0.1-0.2 (low)
1478.33
28.6
966.39
18.7
-511.94
-53.0
0.2-0.3(medium)
1190.44
23.1
1656.73
32.1
466.29
28.1
0.3 -0.4 (high)
957.39
18.5
1257.557
24.4
300.17
23.9
> 0.4 (very high)
936.63
18.1
819.87
15.9
-116.76
-14.2
Total
5162.28
100.0
5162.277
100.0
–
–
Table .3. Change of the NDVI density classes between pre cyclone and post cyclone
35
30
25
20
15
10 PRE CYCLONE (%)
5 POST CYCLONE (%)
0
Fig4.1 Changes of NDVI density categories during the period of pre and post cyclone (%).
In classification process, Supervised Classification method was performed using the maximum likelihood algorithm based on a set of user-defined classes and training areas, by creating the appropriate spectral signatures from Sentinel-2 MSI imageries. Over 20 training areas were repeatedly selected from the whole study area by drawing a polygon around training sites of interests. LULC classes of these training areas were extracted with respect to general knowledge obtained from topographic maps and field visits. Then, supervised classification was performed using the maximum likelihood classifier. Seven land use classes as build up areas, plantation crops, salt pan and agricultural lands ,water bodies, others, land with scrub were identified. The Fig.5. Represent the LULC maps of the study area for pre and post cyclone, respectively.
To assess the accuracy of the classified images, error matrix was used based on random sampling method in which 156 points were automatically selected from
classified reference image. In error matrix utility, the reference class values were compared with the classified class values in a cxc matrix, where c is the number of classes. Then, overall accuracy and kappa values were computed by using users accuracy and producers accuracy of each class. According to the results, the overall accuracy of classification results were 88.12 % (K= 0.77) and 82.82% (K = 0.64) for the year pre cyclone and post cyclone, respectively.
The pattern of the changes between pre and post cyclone are presented in Table 5 and Figure 5.1. The spatial extent of agricultural lands was significantly increased in post cyclone. But the plantation crops and buildup area are decreased almost in same extent reversely. The plantation crops were accounted for 17.4 % of the total land in pre cyclone. This was decreased by 6.8 %in post cyclone.
Fig .5 LULC Classification Results of Pre and Post Cyclone
Year
Pre-Cyclone (8-9-2018)
Post Cyclone(7-3-2019)
LULC Feature
Area (sq.km)
%
Area(sq.km)
%
Plantation crops
898.8
17.4
350.5
6.8
Land with scrub
2932.7
56.8
2324.3
45.0
Salt pan
132.5
2.6
254.2
4.9
others
233.6
4.5
222.19
4.3
Buildup area
329.2
6.4
63.4
1.2
Water body
17.1
0.3
66.6
1.3
Agriculture land
618.3
12.0
1881
36.4
Total
5162.2
100.0
5162.19
100.0
Table.4. Total Area covered by individual LULC Class
Year
Area (sq.km)
Change in area (sq.km)
LULC Feature
Pre cyclone
Post cyclone
Plantation crops
898.8
350.5
-548.3
Land with scrub
2932.7
2324.3
-608.4
Salt pan
132.5
254.2
121.7
others
233.6
222.19
-11.41
Buildup area
329.2
63.4
-265.8
Water body
17.1
66.6
49.5
Agriculture land
618.3
1881
1262.7
Total
5162.2
5162.19
-548.3
Table.5.LULC Changes between the pre and post cyclone
60
50
40
30
PRE CYCLONE(%)
20
POST CYCLONE(%)
10
0
Fig.5.1 LULC coverage Area (sq.km) over the period of study
The change detection technique, which was employed in this study, was the post- classification comparison. The overlay consisting of LULC maps of pre cyclone andpost cyclone were made through post classification comparison. Then, a transition matrix was prepared for the over laid LULC maps of pre and post cyclone. Fig .6. Shows the thematic map of LULC change during the study period.
Fig.6. Land use/land cover change map (pre cyclone-post cyclone)
CONCLUSION
The NDVI differencing method and classification method are the most common procedures for detecting and monitoring LULC changes. The NDVI differencing method is relatively easy to implement and simple to interpret, but it cannot provide complete matrices of change directions and the index differencing is also subject to registration error. Comparing, two NDVI statistics shows the notably change in low vegetation area by 53 percentage from pre-cyclone to post-cyclone. Moreover, the classification of satellite imagery data is a proper tool to derive land cover-land use maps and statistics. Comparing between classified images of pre and post-Gaja cyclone provide the basis to discern those areas that depict changes of land cover-land use during the study period. Results of this study indicate that supervised classification provided satisfactory results in terms of distinguishing the plantation crops, land with scrub, salt pan, buildup area, others, water body, agriculture land. The main change observed for the time period of pre and post-cyclone was the decrease of plantation crops due to Gaja cyclone from 17.4 to 6.8 %. . Hence, the results of this study confirm that, change detection procedures including NDVI and supervised classification using sentinel-2 MSI data offer a good potential tool for characterizing and understanding LULC changes occurring in the disaster-affected area.
ACKNOWLEDGEMENT
The authors thank the European space agency for providing sentinel-2 satellite images. And thank to Dr. Muthu, Principal, AIT, Coimbatore, for his support to carry this work.
REFERENCES
-
D. Lu, P. Mausel, E. BrondÃzio and E. Moran, Change Detection Techniques, Int. J. Remote Sens. 25 (2004), pp. 2365-2407.
-
Y. Li, J. Chen, R. Lu, P. Gong and T. Yue, Study on Land Cover Change Detection Method Based on NDVI Time Series Batasets: Change Detection Indexes Design, Geoscience and Remote Sensing Symposium, 2005. IGARSS'05 Proceedings 2005 IEEE International, 2005
- [10] D. Lu, P. Mausel, M. Batistella and E. Moran, Landcover Binary Change Detection Methods for use in the Moist Tropical Region of the Amazon: A Comparative Study, Int. J. Remote Sens. 26 (2005), pp. 101-114.
-
Singh, Digital Change Detection Techniques using Remotely-
Sensed Data, Int.J.Remote Sens. 10 (1989), pp. 989-1003
-
Singh, Change Detection in the Tropical Forest Environment of Northeastern India using Landsat, Remote Sensing and Tropical Land Management (1986), pp. 237-254. [38]
-
G.M. Foody, Status of Land Cover Classification Accuracy Assessment, Remote Sens.Environ. 80 (2002), pp. 185-201.
-
J. Jensen, Introductory Digital Image Processing, 3rd (2005)
-