
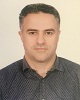
- Open Access
- Authors : Ahmad Qasim Mohammad Alhamad
- Paper ID : IJERTV9IS030305
- Volume & Issue : Volume 09, Issue 03 (March 2020)
- Published (First Online): 25-03-2020
- ISSN (Online) : 2278-0181
- Publisher Name : IJERT
- License:
This work is licensed under a Creative Commons Attribution 4.0 International License
Predicting the Intention to use Mobile Learning: A Hybrid SEM- Machine Learning Approach
Predicting the Intention to use Mobile Learning: A Hybrid SEM- Machine Learning Approach
Ahmad Qasim Mohammad AlHamad1
1(Management Information System, Military College/ Abu Dhabi University, UAE
Abstract:- This research deals with the present perceptions of university students regarding mobile learning when it comes to their higher education. The learning type that depends on the different mobile device features can be referred to as mobile learning. Its easy to spot a plethora of mobile devices in most of the colleges; however, how ready students are when it comes to adopting mobile learning in the UAE needs more research. This study develops an integrated model through the integration of two different theoretical models, namely the technology acceptance model (TAM), theory of planned behavior (TPB). Four hundred eighty-nine university students provided with self-report data, which was analyzed using structural equation modeling. According to the results, the TAM gave a fine explanation of the acceptance of m-learning by university students. In terms of specifics, they had a better intention of adopting mobile learning under the influence of behavioral control, subjective norm, and attitude. For raising the mobile learning acceptance by university students, several important implications were acquired from the results. The present research aims to have a better look at how UAEs university students can use mobile phones for learning. This was made possible by using a suggested TAM & TPB for analyzing how the university students of UAE can utilize mobile devices to conduct homework, share knowledge, look on the Internet for discipline-specific info, and access course material.
Keywords: Mobile learning; United Arab Emirates (UAE); Technology Acceptance Model (TAM); Theory of planned behavior (TPB).
-
INTRODUCTION
As it is becoming easier to use rich digital resources all thanks to mobile technology advancements, the learning discipline is reaching new heights beyond formal education (that is, informal learning) [1][3]. When it comes to formal education, there can also be the tremendous supplementary significance of mobile learning (m-learning) [3]. M-learning has a wide array of uses, which include location-based services, study aids, ubiquitous communications, and affordability. An example can be that the UAE government wants to encourage educational institutions to adopt digital textbooks in place of paper ones under a time limit of five years for saving money [4][7]. With the help of text messages, it would become possible for students to talk to their instructors or other students. Moreover, it is also possible for students to use mobile device applications (or Apps) from virtually any place, which can thus serve as study aids (for instance, algorithm flow charts for computer science students) [8][12]. Furthermore, the geolocation feature can also help students
to get useful location-specific info regarding different landmarks or buildings within the vicinity [13].
With that being said, it is imperative for students to adopt m-learning before realizing its benefits. Even if mobile gadgets are available doesnt mean that theyll be used for educational purposes; the initial requirement is assessing how ready students are when it comes to mobile learning [14], [15]. Although m-learning adoption has high significance, there is still a lack of research on the factors that affect the students m-learning acceptance in higher education [16][19]. College-goers have higher access to mobile devices than K-12 students and, thus, have higher readiness for adopting m-learning [20]. Nonetheless, there is much progress needed by m-learning in higher education [21]. An example of this can be that a number of the university has free Apps, which unfortunately come with non-teaching material (such as maps, event calendars, and news). There should be more research on the factors that affect university students m-learning adoption for achieving success in higher education m-learning [22] [25].
The current literature shows that limited empirical research exists on the use of m-learning in institutions across the UAE, which provides limited comprehension of the factors that have an impact on the students actual use. Concerning methodology, there is a tendency of the majority of the technology acceptance studies to use the structural equation modeling (SEM) approach to examine the theoretical models. Therefore, there is a two-fold objective of this study. The first is how a combination of technology acceptance model (TAM) [26], and theory of planned behavior (TPB) [27] can be used to analyze the actual use of m-learning by students, and the second is to use the PLS-SEM and ML algorithm to verify the theoretical model that has been developed.
-
RESEARCH MODEL AND HYPOTHESES DEVELOPMENT
Subjective norm (SUB) constructs and two theoretical models (i.e., TPB and TAM) combine to form the research model for this study. As per proposition, m-learning systems have their perceived ease of use (PEOU) and perceived usefulness (PU) highly dependent on and subjective norm and attitude towards mobile learning system. Other than that, the suggestion also entails that SUB, PU, and PEOU can influence the intention of using m-learning systems. The suggested theoretical model, hypotheses, and literature review can be seen in Figure 1.
-
Perceived Ease of Use (PEOU)
Perceived ease of use (PEOU) is defined as the degree to which an individual believes that using a particular system would be free of physical and mental effort [28]. Prior research found that PEOU has a significant influence on attitude towards the use of m-learning platforms [29], [30] and subjective norm [13]. Based on previous research, we proposed two hypotheses for the model.
H1: Perceived ease of use (PEOU) would predict the attitude towards the use of m-learning platforms (IU).
H2: Perceived ease of use (PEOU) would predict the subjective norm (SUB).
-
Perceived Usefulness (PU)
Perceived usefulness (PU) is defined as the degree to which a person believes that using a particular system would enhance his or her job performance. [28]. Previous research indicated that PU has a significant impact on attitude towards the use of m-learning platforms [29], [30] and subjective norm [13]. Based on previous research, we proposed two hypotheses for the model.
H3: Perceived usefulness (PU) would predict the attitude towards use of m-learning platforms (IU).
H4: Perceived usefulness (PU) would predict the subjective norm (SUB).
-
Attitude (ATD)
Attitude (ATD) is defined as ones desirability to use the system [31]. Previous m-learning studies pointed out that ATD has a significant association with IU. Previous
research indicated that ATD has a significant impact on the intention to use m-learning systems [3], [13], [32], [33]. Therefore, this leads to the following:
H5: Attitude (ATD) would predict the intention of using m-learning platforms (IU).
-
Subjective Norm (SUB)
Subjective norm (SUB) is defined as the degree to which a person believes that using a particular system would enhance his or her job performance [28]. Previous research indicated that subjective norm has a significant impact on intention of using m-learning platforms (IU). [3], [13], [34][36]. Therefore, this leads to the following:
H6: Subjective norm (SUB) would predict the intention of using m-learning pltforms (IU).
-
Perceived Behavioral Control (PBC)
Perceived Behavioral Control (PBC) is defined as peoples perception of the ease or difficulty of performing the behavior of interest [37]. Previous research indicated that PBC has a significant impact on the intention of using m-learning platforms (IU) [3], [13], [38], [39]. Therefore, this leads to the following:
H7: Perceived Behavioral Control (PBC) would predict the intention of using m-learning platforms (IU).
The proposed research model relies on these hypotheses, as illustrates in Fig. 1. The theoretical model is first given the form of a structural equation model, and then it is assessed by using machine learning methods.
Fig 1.The study model
-
-
RESEARCH METHODOLOGY
-
Context and Subject
As in Table 1, students from the British University in Dubai and the University of Fujairah, both of which are situated in the UAE, were chosen as the study participants. Self-administrated surveys were used to collect data between January and February 2020. The surveys were voluntarily filled by the participants, who did not receive
any payment for participating in the study. Data collection was carried out in this study using the convenience sampling method. A total of 500 surveys were distributed, out of which 489 students filled the complete survey, indicating a response rate of 97.8%. These comprised of
259 males and 230 females. The ages of 59% of the participants were between 18 and 29 years. Besides, the majority of the participants had a bachelors degree (48%), followed by a masters degree (30%), and Ph.D. (22%).
Table 1: Participants Details
University
No. of students
University of Fujairah (UOF)
270
The British University in Dubai (BUiD)
219
-
Study Instrument
There were two parts of the research instrument used in the study. The objective of the first part was to obtain the participants demographic data, while the second one sought responses about the factors in the conceptual model. A 5-point Likert scale was used to measure items in the
second part. PEOU and PU were measured using items adapted from [26]. Attitude, subjective norm, perceived behavioral control, and intention to use m-learning platform were measured using items adapted from [27]. The Appendix presents a list of constructs and their underlying items.
Table 2: Constructs and their Sources
Constructs
Number of items
Source
Attitude
3
[3], [13] Subjective Norm
3
[3], [13] Perceived Behavioral Control
3
[3], [13] Perceived Ease of Use
3
[3], [40], [41] Perceived Usefulness
3
[3], [40], [41] Intention to use m-learning
2
[3], [40], [41] -
Pre-Test of the Questionnaire
The reliability of the questionnaire items was measured using a pilot study before the final survey was carried out. Random selection of 50 students was carried out from the target population for this study. The internal reliability of the constructs items was determined using Cronbachs alpha. It was asserted by [42] that a reliability coefficient of
0.70 or more was acceptable. It can be seen in Table 3 that
the Cronbachs alpha values for each of the constructs of the study were more than 0.7. This confirms the reliability of all constructs, and so, they can be employed in the final study.
It can be seen in the table presented above that the five measurement scales used in the questionnaire are reliable. Therefore, they may be employed in the study.
Table 3: Cronbachs Alpha values for the pilot study (Cronbachs Alpha 0.70)
Construct
Cronbachs Alpha
Attitude
0.903
Subjective norm
0.856
Perceived Behavioral Control
0.828
Perceived Ease of Use
0.842
Perceived Usefulness
0.876
Intention to Use the M-Learning System
0.849
-
-
FINDING AND DISCUSSIONS
-
Data Analysis
Two distinct techniques are used in the study to evaluate the theoretical model developed. The first technique used in this study is the partial least squares-structural equation modeling (PLS-SEM) through the Smart PLS tool [43]. PLS-SEM is used in this study mainly because it offers a concurrent examination of the measurement as well as the
structural model, thus offering more precise results [1], [3], [11], [19], [44][46]. The second technique used in the study is the machine learning algorithms through Weka for predicting the dependent variables of the conceptual model [47].
-
Measurement Model Assessment
Reliability and validity are tested to examine the measurement model [48]. The Cronbachs alpha and
composite reliability (CR) measures were employed to determine the reliability. Each of these measures should have values 0.70 [48]. The findings in Table 1 show that both measures have satisfactory values, which confirms the reliability of the model.
It has been suggested by [48] that the convergent and discriminant validities should be evaluated to perform validity testing. AVE should have values 0.50 [49], while those of factor loadings should be 0.70 [50]. It can be
seen in the results given in Table 1 that the two measures have acceptable values, which confirms the convergent validity. It was suggested by [51] that the Heterotrait- Monotrait ratio (HTMT) of correlations should be carried out to determine to discriminate validity. HTML should have values < 0.85. The findings of Table 2 show that all the values of HTMT are acceptable, which confirms the discriminant validity.
Table 4: Convergent validity Results which Assures Acceptable Values (Factor Loading, Cronbachs Alpha, Composite Reliability 0.70 & AVE > 0.5)
Constructs
Items
Factor Loading
Cronbach's Alpha
CR
AVE
Attitude
ATD1
0.789
0.800
0.802
0.559
ATD 2
0.788
ATD 3
0.812
Subjective norm
SUB1
0.888
0.877
0.777
0.600
SUB2
0.874
SUB3
0.795
Perceived Behavioral Control
PBC1
0.820
0.750
0.803
0.766
PBC2
0.828
PBC3
0.875
Perceived Ease of Use
PEOU1
0.799
0.890
0.711
0.747
PEOU2
0.801
PEOU3
0.765
Perceived Usefulness
PU1
0.901
0.789
0.749
0.637
PU2
0.863
PU3
0.755
Intention to Use the E-Learning System
IU1
0.840
0.881
0.805
0.705
IU2
0.744
Table 5: Heterotrait-Monotrait Ratio (HTMT)
ATD
SUB
PBC
PEOU
PU
IU
ATD
SUB
0.365
PBC
0.256
0.210
PEOU
0.241
0.400
0.524
PU
0.565
0.630
0.502
0.663
IU
0.242
0.554
0.635
0.409
0.250
Note: IU, intention to use mobile learning; PEOU, perceived ease of use; PU, perceived usefulness; ATD, attitude; SUB, subjective norm; PBC, perceived behavioral control.
-
Hypotheses Testing and Coefficient of Determination
The structural equation modeling (SEM) approach [52] was used to test the aforementioned nine hypotheses together. The variance described (R2 value) by each path and every hypothesized connections path significance in the research model were assessed. The standardized path coefficients and path significances are demonstrated in Fig. 2 and Table 6.
Table 6 shows that the R2 values for attitude, subjective norm, and the intention to use m-learning system ranged between 0.569 and 0.656. Therefore, these constructs appear to have Moderate predictive power (Liu, Liao, & Peng, 2005). Generally, the data supported eight out of seven hypotheses. According to previous studies, all constructs were verified in the model (ATD, PEOU, PU,
SUB, PBC, and IU). Based on the data analysis hypotheses H1, H2, H3, H4, H5, H6, and H7 were supported by the empirical data. The results showed that Perceived Ease of Use (PEOU) significantly influenced Attitude (ATD) (= 0.248, P<0.001), and Subjective norm (SUB) (= 0135, P<0.05) supporting hypothesis H1 and H2 respectively. Perceived Usefulness (PU) was determined to be significant in affecting Attitude (ATD) (= 0.260, P<0.05), and Subjective norm (SUB) (= 0.509, P<0.001) supporting hypothesis H3 and H4 respectively. Finally, Intention to Use the M-Learning System (IU) has significant effects on Attitude (ATD) (= 0.826, P<0.05), Subjective norm (SUB) (= 0.420, P<0.05), and Perceived Behavioral Control (PBC) (= 0.469, P<0.05) respectively; hence, H5, H6, and H7 are supported.
Table 6: R2 of the Endogenous Latent Variables
Constructs
R2
Results
ATD
0.603
Moderate
SUB
0.569
Moderate
IU
0.656
Moderate
Note: IU, intention to use mobile learning; ATD, attitude; SUB, subjective norm.
Table 7: Summary of hypotheses tests at p**=<0.01, p* <0.05Significant at p**=<0.01, p* <0.05
H
Relationship
Path
t-value
p-value
Direction
Decision
H1
PEOU -> ATD
0.248
19.698
0.000
Positive
Supported**
H2
PEOU -> SUB
0.135
3.855
0.042
Positive
Supported*
H3
PU -> ATD
0.260
3.499
0.031
Positive
Supported*
H4
PU -> SUB
0.509
12.636
0.000
Positive
Supported**
H5
ATD -> IU
0.826
4.801
0.037
Positive
Supported*
H6
SUB -> IU
0.420
2.114
0.044
Positive
Supported*
H7
PBC -> IU
0.469
3.870
0.039
Positive
Supported*
Note: IU, intention to use mobile learning; PEOU, perceived ease of use; PU, perceived usefulness; ATD, attitude; SUB, subjective norm; PBC, perceived behavioral control.
Fig 2: Hypotheses testing results (significant at p** < = 0.01, p* < 0.05).
-
Hypotheses Testing Using Machine Learning Algorithms
Machine-learning classification algorithms are used in this study by employing several methodologies, such as decision trees, Bayesian networks, neural networks, and if- then-else rules to predict the correlations within the proposed theoretical model [47]. The predictive model was tested using Weka (ver. 3.8.3) based on various classifiers, such as AdaBoostM1, BayesNet, Logistic, LWL, OneR, and J48 [54], [55].
The findings presented in Table 8 show that better performance is exhibited by J48 in comparison to other classifiers when determining the attitude (ATD) of m- learning systems. ATD was predicted by J48 with 88.11% accuracy for the 10-fold cross-validation. Therefore, there is support for H1 and H3. An improved performance was shown by this classifier in comparison to rest of the classifiers with respect to the TP rate (.881), recall (.702) and precision (.786)
Table 8: Predicting the ATD by PEOU and PU
Classifier
CCI1 (%)
TP2 Rate
FP3 Rate
Precision
Recall
F-Measure
BayesNet
82.25
.823
.231
.645
.640
.644
Logistic
82.25
.823
.290
.655
.653
.648
LWL
78.17
.782
.224
.669
.668
.665
AdaBoostM1
82.19
.822
.320
.708
.705
.700
OneR
83.01
.830
.365
.703
.701
.702
J48
88.11
.881
.666
.786
.702
.708
1CCI: Correctly Classified Instances, 2TP: True Positive, 3FP: False Positive.
It is also shown in the findings that superior classifier performance was exhibited by J48 as compared to other classifiers in predicting the subjective norm (SUB), as demonstrated in Table 9. The SUB was predicted by J48
using the attributes of perceived ease of use (PEOU) and perceived usefulness (PU) with 79.90% accuracy. Hence, there was support for both H2 and H4.
Table 9: Predicting the SUB by PEOU and PU
Classifier
CCI1 (%)
TP2 Rate
FP3 Rate
Precision
Recall
F-Measure
BayesNet
7.00
.750
.425
.744
.750
.748
Logistic
75.20
.752
.369
.749
.752
.741
LWL
74.08
.741
.440
.742
.741
.740
AdaBoostM1
73.24
.732
.259
.732
.732
.730
OneR
74.53
.745
.458
.745
.745
.742
J48
79.90
.800
.364
.789
.800
.785
It is shown in the findings given in Table 10 that compared to other classifiers; improved performance was shown by J48 in predicting the intention to use mobile learning system (IU) using the attributes of attitude (ATD),
subjective norm (SUB) and perceived behavioral control (PBC). The intention to use the mobile learning system was predicted by J48 with 89.26% accuracy. Hence there was support for H5, H6, and H7.
Table 10: Predicting the IU by ATD, SUB, and PBC
Classifier
CCI1 (%)
TP2 Rate
FP3 Rate
Precision
Recall
F-Measure
BayesNet
81.29
.813
.802
.811
.813
.812
Logistic
83.26
.833
.832
.825
.833
.832
LWL
83.58
.836
.826
.826
.836
.835
AdaBoostM1
82.06
.821
.822
.823
.821
.822
OneR
82.20
.822
.823
.822
.822
.821
J48
89.26
.893
.880
.889
.893
.889
-
-
CONCLUSION AND FUTURE WORKS
In this paper, the factors that affect the intention of university students for using m-learning through TAM and TPB have been evaluated. According to the findings, the TPB elements were responsible for 65.6 percent of intention for adopting m-learning within UAE higher education, alongside behavioral control, subjective norm, and attitudes being the primary factors. This research presents a conceptual model for predicting the students intention of using m-learning mechanisms. The proposed model was tested by this research using machine learning algorithms and PLS-SEM for analysis. When it comes to the subjective norm (SUB) prediction, a strong relationship between subjective norm (SUB) and the perceived ease of use (PEOU) and perceived usefulness (PU) was shown by the PLS-SEM results. Additionally, the subjective norm (SUB) was better predicted with the help of a decision tree known as J48 in comparison to other classifiers, as seen from the machine learning findings. In terms of the 10-fold cross-validation, the PEOU prediction was accurate by 79.90% by the J48 tree. Generally, there is a possibility that the realization of the subjective norm (SUB) is positively related to the perceived ease of use (PEOU) and perceived
usefulness (PU) that colleagues of students have regarding these systems.
In terms of the attitude (ATD) of m-learning systems prediction, it can be seen from the PLS-SEM findings that there is a significant, positive relationship between the attitude (ATD) of m-learning systems and perceived ease of use (PEOU) alongside perceived usefulness (PU) in combination. Apart from that, the machine learning findings include that the J48 is better in the prediction of the ATD by being 88.11% accurate. From this, there is a possibility that the ATD of m-learning systems is directly proportional to the perceived ease of use (PEOU) and perceived usefulness (PU) regarding how m-learning systems will perform. When it comes to predicting the attitude (ATD), there is a great significance of perceived ease of use (PEOU) and perceived usefulness (PU), as per the findings of PLS-SEM. In terms of using the attitude (ATD) to predict the perceived ease of use (PEOU) and perceived usefulness (PU), the J48 and OneR classifiers outmatch other classifiers in terms of performance, as suggested by the machine learning findings. In addition, it was seen by the machine learning outcomes that the J48 was accurate by 89.26% when it comes to the intention
prediction (IU), thus outmatching the other classifiers. According to our findings, the likelihood of students continually using m-learning systems later on is directly proportional to the perceived behavioral control (PBC), perceived ease of use (PEOU), and perceived usefulness (PU). When it comes to the prediction of its actual use, the intention use of m-learning systems has a significant, positive effect on its actual use, as per the findings of PLS- SEM. Moreover, it was found through machine learning that J48 is better at the prediction of the actual use as it was accurate by 89.26%.
-
Limitations and Future Directions
There are several critical limitations that need to be discussed. Firstly, caution should be exhibited when generalizing the findings to other institutes in the UAE or other countries. This is because of two reasons: a) the focus is merely on two institutes for data collection, and b) a convenience sampling approach is used for choosing the respondents. It is important to carry out additional research to take into account these issues to improve the opportunity of generalizing the findings. Secondly, the focus of the study was on assessing the actual use of m-learning systems by only the students. It is recommended that the educators actual use of m-learning systems should be measured in the subsequent studies to obtain additional information regarding the influencing determinants and a holistic view of the implementation of these systems.
-
Research Implications
A complementary approach with the PLS-SEM and machine learning classification algorithms was used in the study to test the proposed model. It is believed that using a complementary multi-analytical approach makes a distinct contribution to the information system (IS) literature, as this study serves as one of the few efforts in which machine learning algorithms are used to predict the actual use of m- learning systems. A significant point to note is that PLS- SEM can be employed for predicting a dependent variable and validating a conceptual model on the basis of the extension of existing theory [56]. In the same way, to predict a dependent variable on the basis of independent variables [47], supervised machine learning algorithms (those with a pre-defined dependent variable) can be used. Another interesting point is that various classification algorithms are used in the study with distinct methodologies, like decision trees, association rules, if- then-else rules, and Bayesian networks. The findings also suggested that J48 (a decision tree) in particular showed better performance compared to other classifiers in the majority of the cases. It should also be noted that continuous (numerical), as well as categorical variables, were classified using the decision tree (nonparametric), which divided the sample into homogenous subsamples on the basis of the most significant independent variable [47]. In contrast, the significant coefficients were tested using PLS-SEM (a nonparametric procedure) with replacements from the smple to obtain a large number of samples randomly.
ACKNOWLEDGEMENTS
An I would like to express my special thanks for my parent and my wife for encouraging me to complete this research; they walk with me and support me during my research.
REFERENCES
-
B. Al Kurdi, M. Alshurideh, S. A. Salloum, Z. M. Obeidat, and R.
M. Al-dweeri, An Empirical Investigation into Examination of Factors Influencing University Students Behavior towards Elearning Acceptance Using SEM Approach, Int. J. Interact. Mob. Technol., vol. 14, no. 02, pp. 1941, 2020.
-
M. Alghizzawi, M. Habes, S. A. Salloum, M. A. Ghani, C. Mhamdi, and K. Shaalan, The effect of social media usage on studentse-learning acceptance in higher education: A case study from the United Arab Emirates, Int. J. Inf. Technol. Lang. Stud., vol. 3, no. 3, 2019.
-
M. Al-Emran, I. Arpaci, and S. A. Salloum, An empirical examination of continuous intention to use m-learning: An integrated model, Educ. Inf. Technol., pp. 120, 2020.
-
T. Priest and K. Schoepp, Implementing mobile learning devices into tertiary classrooms, Int. Handb. E-Learning Vol. 2 Implement. Case Stud., vol. 189, 2015.
-
S. A. Salloum and K. Shaalan, Investigating students acceptance of e-learning system in higher educational environments in the UAE: Applying the extended technology acceptance model (TAM). The British University in Dubai, 2018.
-
S. A. Salloum, W. Maqableh, C. Mhamdi, B. Al Kurdi, and K. Shaalan, Studying the Social Media Adoption by university students in the United Arab Emirates, Int. J. Inf. Technol. Lang. Stud., vol. 2, no. 3, 2018.
-
S. A. Salloum, M. Al-Emran, M. Habes, M. Alghizzawi, M. A. Ghani, and K. Shaalan, Understanding the Impact of Social Media Practices on E-Learning Systems Acceptance, in International Conference on Advanced Intelligent Systems and Informatics, 2019, pp. 360369.
-
J. R. Young, Smartphones on campus: the search for killerapps, Chron. High. Educ., vol. 57, no. 36, pp. B6B7, 2011.
-
S. A. Salloum, A. Q. AlHamad, M. Al-Emran, and K. Shaalan, A survey of Arabic text mining, vol. 740. 2018.
-
A. Al-Hamad, N. Yaacob, and A. Y. Al-Zoubi, Integrating learning styleinformation into personalized e-learning system, IEEE Multidiscip. Eng. Educ. Mag., vol. 3, no. 1, pp. 26, 2008.
-
R. S. Al-Maroof, S. A. Salloum, A. Q. AlHamadand, and K. Shaalan, Understanding an Extension Technology Acceptance Model of Google Translation: A Multi-Cultural Study in United Arab Emirates, Int. J. Interact. Mob. Technol., vol. 14, no. 03, pp. 157178, 2020.
-
A. Q. M. AlHamad, Acceptance of E-learning among university students in UAE: A practical study., Int. J. Electr. Comput. Eng., vol. 10, 2020.
-
J. Cheon, S. Lee, S. M. Crooks, and J. Song, An investigation of mobile learning readiness in higher education based on the theory of planned behavior, Comput. Educ., 2012.
-
J. Keller, The slow-motion mobile campus, Chron. High. Educ., vol. 2, pp. B4B6, 2011.
-
A. Q. AlHamad, N. Yaacob, and F. Al-Omari, Applying JESS rules to personalize Learning Management System (LMS) using online quizzes, in 2012 15th International Conference on Interactive Collaborative Learning (ICL), 2012, pp. 14.
-
A. Q. AlHamad, K. I. Al Qawasmi, and A. Q. AlHamad, Key Factors in Determining Students Satisfaction in Online Learning Based onWeb Programmingcourse within Zarqa University, Int. J. Glob. Bus., vol. 7, no. 1, p. 7, 2014.
-
M. A. Otair and A. Q. A. Hamad, Expert personalized e-learning recommender system, Retrieved June, vol. 13, p. 2010, 2005.
-
I. F. Al-Mashaqbeh and A. Al Hamad, Students perception of an online exam within the Decision Support System Course at Al al Bayt University, in 2010 Second International Conference on Computer Research and Development, 2010, pp. 131135.
-
R. S. Al-Maroof, S. A. Salloum, A. Q. M. AlHamadand, and K. Shaalan, A Unified Model for the Use and Acceptance of Stickers in Social Media Messaging, in International Conference
on Advanced Intelligent Systems and Informatics, 2019, pp. 370 381.
-
J. Traxler, Current state of mobile learning, Mob. Learn. Transform. Deliv. Educ. Train., vol. 1, pp. 924, 2009.
-
Y. Park, A pedagogical framework for mobile learning: Categorizing educational applications of mobile technologies into four types, Int. Rev. Res. open Distrib. Learn., vol. 12, no. 2, pp. 78102, 2011.
-
M. T. Alshurideh, S. A. Salloum, B. Al Kurdi, A. A. Monem, and
K. Shaalan, Understanding the Quality Determinants that Influence the Intention to Use the Mobile Learning Platforms: A Practical Study, Int. J. Interact. Mob. Technol., vol. 13, no. 11, pp. 157183, 2019.
-
S. F. S. Alhashmi, S. A. Salloum, and S. Abdallah, Critical Success Factors for Implementing Artificial Intelligence (AI) Projects in Dubai Government United Arab Emirates (UAE) Health Sector: Applying the Extended Technology Acceptance Model (TAM), in International Conference on Advanced Intelligent Systems and Informatics, 2019, pp. 393405.
-
M. Alshurideh, B. Al Kurdi, and S. A. Salloum, Examining the Main Mobile Learning System Drivers Effects: A Mix Empirical Examination of Both the Expectation-Confirmation Model (ECM) and the Technology Acceptance Model (TAM), in International Conference on Advanced Intelligent Systems and Informatics, 2019, pp. 406417.
-
S. A. Salloum, C. Mhamdi, B. Al Kurdi, and K. Shaalan, Factors affecting the Adoption and Meaningful Use of Social Media: A Structural Equation Modeling Approach, Int. J. Inf. Technol. Lang. Stud., vol. 2, no. 3, 2018.
-
F. D. Davis, Perceived usefulness, perceived ease of use, and user acceptance of information technology, MIS Q., pp. 319 340, 1989.
-
I. Ajzen, From intentions to actions: A theory of planned behavior, in Action control, Springer, 1985, pp. 1139.
-
F. D. Davis, Perceived Usefulness, Perceived Ease of Use, and User Acceptance of Information Technology, MIS Q., vol. 13, no. 3, pp. 319340, 1989.
-
K. M. Üzdoan, N. Baolu, and G. Erçetin, Exploring major determinants of mobile learning adoption, in 2012 Proceedings of PICMET12: Technology Management for Emerging Technologies, 2012, pp. 14151423.
-
M. E. Seliaman and M. S. Al-Turki, Mobile learning adoption in Saudi Arabia, World Acad. Sci. Eng. Technol., vol. 69, no. 9, pp. 293391, 2012.
-
H. Karjaluoto, M. Mattila, and T. Pento, Factors underlying attitude formation towards online banking in Finland, Int. J. Bank Mark., vol. 20, no. 6, pp. 261272, 2002.
-
N. T. V. Khanh and G. Gim, Factors influencing mobile-learning adoption intention: An empirical investigation in high education, J. Soc. Sci., vol. 10, no. 2, pp. 5162, 2014.
-
J. C. S. Prieto, S. O. Migueláñez, and F. J. GarcÃa-Peñalvo, Mobile learning adoption from informal into formal: an extended TAM model to measure mobile acceptance among teachers, in Proceedings of the Second International Conference on Technological Ecosystems for Enhancing Multiculturality, 2014, pp. 595602.
-
Y. Liu and N. S. Chen, An adoption model for mobile learning, in Proceeding for the IADIS international Conference e- Commerce, 2008, pp. 251256.
-
J. Mtebe and R. Raisamo, Investigating students behavioural intention to adopt and use mobile learning in higher education in East Africa, Int. J. Educ. Dev. using ICT, vol. 10, no. 3, 2014.
-
S. Y. Park, M. Nam, and S. Cha, University students behavioral intention to use mobile learning: Evaluating the technology acceptance model, Br. J. Educ. Technol., vol. 43, no. 4, pp. 592 605, 2012.
-
I. Ajzen, The theory of planned behavior, Organ. Behav. Hum. Decis. Process., vol. 50, no. 2, pp. 179211, 1991.
-
J. Cheong, S. Lee, S. M. Crooks, and J. Song, An investigation of mobile learning readiness in higher education based on the theory of planned behaviour, Comput. Educ., vol. 59, pp. 1054 1064, 2012.
-
B. Kim, An empirical investigation of mobile data service continuance: Incorporating the theory of planned behavior into the expectationconfirmation model, Expert Syst. Appl., vol. 37,
no. 10, pp. 70337039, 2010.
-
G. W.-H. Tan, K.-B. Ooi, L.-Y. Leong, and B. Lin, Predicting the drivers of behavioral intention to use mobile learning: A hybrid SEM-Neural Networks approach, Comput. Human Behav., vol. 36, pp. 198213, 2014.
-
Y. Bao, T. Xiong, Z. Hu, and M. Kibelloh, Exploring gender differences on general and specific computer self-efficacy in mobile learning adoption, J. Educ. Comput. Res., vol. 49, no. 1, pp. 111132, 2013.
-
J. C. Nunnally and I. H. Bernstein, Psychometric theory, 1978.
-
C. M. Ringle, S. Wende, and J.-M. Becker, SmartPLS 3. Bönningstedt: SmartPLS. 2015.
-
D. Barclay, C. Higgins, and R. Thompson, The Partial Least Squares (pls) Approach to Casual Modeling: Personal Computer Adoption Ans Use as an Illustration. 1995.
-
S. F. S. Alhashmi, S. A. Salloum, and C. Mhamdi, Implementing Artificial Intelligence in the United Arab Emirates Healthcare Sector: An Extended Technology Acceptance Model, Int. J. Inf. Technol. Lang. Stud., vol. 3, no. 3, 2019.
-
S. A. Salloum, A. Q. M. Alhamad, M. Al-Emran, A. A. Monem, and K. Shaalan, Exploring Students Acceptance of E-Learning Through the Development of a Comprehensive Technology Acceptance Model, IEEE Access, vol. 7, pp. 128445128462, 2019.
-
I. Arpaci, A hybrid modeling approach for predicting the educational use of mobile cloud computing services in higher education, Comput. Human Behav., vol. 90, pp. 181187, 2019.
-
J. F. Hair Jr, G. T. M. Hult, C. Ringle, and M. Sarstedt, A primer on partial least squares structural equation modeling (PLS-SEM). Sage Publications, 2016.
-
C. Fornell and D. F. Larcker, Evaluating Structural Equation Models With Unobservable Variables and Measurement Error, J. Mark. Res., vol. 18, no. 1, pp. 3950, 1981.
-
J. F. Hair, W. C. Black Jr, B. J. Babin, and R. E. Anderson, Multivariate Data Analysis, Pearson Prentice Hall, USA, 2010.
-
J. Henseler, C. M. Ringle, and M. Sarstedt, A new criterion for assessing discriminant validity in variance-based structural equation modeling, J. Acad. Mark. Sci., vol. 43, no. 1, pp. 115 135, 2015.
-
F. D. Davis, R. P. Bagozzi, and P. R. Warshaw, Extrinsic and intrinsic motivation to use computers in the workplace, J. Appl. Soc. Psychol., vol. 22, no. 14, pp. 11111132, 1992.
-
S.-H. Liu, H.-L. Liao, and C.-J. Peng, Applying the technology acceptance model and flow theory to online e-learning users acceptance behavior, E-learning, vol. 4, no. H6, p. H8, 2005.
-
E. Frank et al., Weka-a machine learning workbench for data mining, in Data mining and knowledge discovery handbook, Springer, 2009, pp. 12691277.
-
K. M. Alomari, A. Q. AlHamad, and S. Salloum, Prediction of the Digital Game Rating Systems based on the ESRB.
-
M. Al-Emran, V. Mezhuyev, and A. Kamaludin, PLS-SEM in Information Systems Research: A Comprehensive Methodological Reference, in 4th International Conference on Advanced Intelligent Systems and Informatics (AISI 2018), 2018, pp. 644653.