
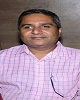
- Open Access
- Authors : Sushil Kumar , Sunil Kumar Atrey , D. P Singh , Rahul Garg
- Paper ID : IJERTV9IS030591
- Volume & Issue : Volume 09, Issue 03 (March 2020)
- Published (First Online): 04-04-2020
- ISSN (Online) : 2278-0181
- Publisher Name : IJERT
- License:
This work is licensed under a Creative Commons Attribution 4.0 International License
Process Parameters Optimization of Turning Operation for Surface Roughness Improvement at Shriram Pistons and Rings Limited, Ghaziabad
Sushil Kumara
a Department of Mechanical Engineering, Lecturer Mechanical D.N Polytechnic Meerut 250103,
Uttar Pradesh, India.
Sunil Kumar Atreyb
bDepartment of Mechanical Engineering, Lecturer Mechanical D.N Polytechnic Meerut 250103, Uttar Pradesh, India.
-
P Singhc
cDepartment of Mechanical Engineering, Lecturer Mechanical SCRIET C.C.S University Meerut 250004, Uttar Pradesh, India.
Rahul Gargd
dDepartment of Space Delhi Earth Station/Space application centre, Scientist/Engineer SD ISRO New Delhi
– 110021, Delhi India.
Abstract:- This paper presents the experimental study, development of mathematical model and parametric optimization for surface roughness in turning of Aluminium 1275 alloy pistons using PCD (Poly Crystalline Diamond) cutting tool insert using Taguchi parameter design. The experimental plan and analysis was based on the Taguchi L27 orthogonal array taking spindle speed (A3), feed rate (B1), depth of cut (C3) and tool nose radius (D3) as important cutting parameters. The influence of the machining parameters on the surface finish has also been investigated and the optimum cutting condition for improving the surface roughness is evaluated. The optimal parametric combination for PCD cutting insert is found to be A3-B1-C3-D3. The ANOVA and S/N ratio results show that feed rate is the most significant process parameter followed by tool nose radius. Depth of cut is the least significant process parameter. A confirmation run was used to verify the results, which indicated that this method was both efficient and effective in determining the best turning parameters for the optimal surface roughness. Optimum parameter setting for surface roughness is obtained at a spindle speed of 1500 rpm, feed rate of 0.25 (mm/rev), depth of cut of 0.40 mm and tool nose radius of 0.80 mm. Average surface roughness Ra value was improved in the required range of 4.5 µm to 5 µm.
Keywords: Taguchi method, average surface roughness Ra, optimization, Aluminium 1275 alloy, ANOVA, S/N ratio.
-
INTRODUCTION
Surface roughness has become the most significant technical requirement and it is an index of product quality. In order to improve the tribological properties, fatigue strength and corrosion resistance, a reasonably good surface finish is desired. Nowadays, the manufacturing industries specially are focusing their attention on dimensional accuracy and surface finish. In order to obtain optimal cutting parameters to achieve the best possible surface finish, manufacturing industries have resorted to the use of handbook based information and operators experience. This traditional practice leads to improper surface finish and decrease in productivity due to sub optimal use of machining capability. This causes high manufacturing cost and low product quality. Hence there is a need to optimize the process parameters in a systematic way to achieve the responses by using experimental methods. Taguchi employed design of experiments, which is one of the most important and efficient tools of TQM (total quality management) for designing high quality systems at reduced cost. Taguchi approach helps to reduce the large number of experimental trials when the number of process parameters increases.
-
LITERATURE REVIEW
Optimization of process parameters i.e. spindle speed, feed rate, depth of cut and tool nose radius is a critical problem to obtain desired surface roughness in less number of experiments. From the present literature review it can be concluded that Taguchi method is an important optimization method among various optimization techniques. Ahmet Hascalik and Ulas Caydas
[1] used parameter design of the Taguchi method in the optimization of turning operations. Effect and optimization of machining parameters on surface roughness and tool life in a turning operation on Ti-6 Al-4V alloy was studied. It was concluded that feed rate was the most influential factor for surface roughness and cutting speed was the most influential factor for tool life. M. Kaladhar et al. [2] applied Taguchi method to determine the optimum process parameters for turning of AISI 304 Austenitic Stainless Steel on CNC Lathe. It was concluded that machined surface roughness was affected mostly by cutting speed and material removal rate (MRR) was significantly affected by depth of cut. Ali Riza Motorcu [3] investigated surface roughness in turning of AISI 8660 hardened alloy steels on CNC Lathe by ceramic based cutting tools. Cutting speed, feed rate, depth of cut and tool nose radius was used as cutting parameters. It was concluded that feed rate was found to be the dominant factor among controllable factors on surface roughness followed by depth of cut and tool nose radius. Cutting speed showed an insignificant effect. Sijo M. T. and Biju N. [4] applied Taguchi parameter optimization method to determine optimal process parameters. Depth of cut and hardness of material had less contribution on surface roughness. Feed rate was the prime factor forsurface finish. Ilhan Asilturk and Harun Akkus [13] studied optimal turning parameters based on the Taguchi method to minimize surface roughness. S/N ratio and ANOVA methods were used to investigate effects of cutting speed, feed rate and depth of cut on surface roughness. Results indicated that feed rate had the most significant effect on surface roughness. M. Kaladhar et al. [14] used a multi characteristics response optimization model based on Taguchi and utility concept to optimize process parameters such as speed, feed, depth of cut and nose radius on multiple performance characteristics surface roughness and material removal rate during turning of AISI 202 Austenitic Stainless It was found that feed was the most significant parameter for surface roughness. Jitendra Verma et al. [17] focused on the analysis of optimum cutting conditions to get lowest surface roughness in turning of ASTM A 242 type 1 alloy steel on CNC lathe by Taguchi method. Experiments were conducted by using Taguchi method and 9 experiments were conducted. Results were analyzed by using analysis of variance method. It was concluded that cutting speed had significant role to play in producing lower surface roughness. The depth of cut had lesser role on surface roughness. S. Thamizhmanil et al. [21] focusssed on the analysis of optimum cutting conditions to get lowest surface roughness in turning soft SCM 440 alloy steel by Taguchi method. 18 experiments were conducted on soft SCM 440 alloy steel work piece by using TiN coated ceramic tool on Harrison 400 Lathe. The cutting speed had less role on surface roughness. It was concluded that Taguchi method gave a systematic simple approach and efficient method for the optimum operating conditions. D. Philip Selvaroj and P. Chandramohan [22] conducted experiments based on Taguchi method to collect data of effects on cutting parameters like cutting speed, feed rate and depth of cut on surface roughness of AISI 304 Austenitic Stainless Steel during dry turning on Kirloskar Turn Master 35 Lathe by using TiC and TiCN coated tungsten carbide tool. Feed rate had a great effect on surface roughness improvement.
-
EXPERIMENTAL METHODOLOGY
-
-
Experimental set up
In order to obtain desired average surface roughness Ra value on Aluminium 1275 alloy pistons, optimum value of process parameters was determined. Aluminium 1275 alloy pistons are machined on Finish Oval Turning Centre by using PCD (PolyCrystalline Diamond) cutting tool insert. Surfcom 480 A surface roughness measurement instrument measures average surface roughness Ra value in µm. Maximum spindle speed on Finish Oval Turning Centre is 2000 rpm. It has Fanuc control system. Its make is TAKISAWA of TPS-3100S series. Rake angle of cutting tool is 00. Front clearance angle (primary) is
100. Front clearance angle (secondary) is 120. Point angle is 900. Shape of cutting tool insert is triangular. Three cutting tool inserts of different tool nose radius of 0.4 mm, 0.6 mm and 0.8 mm were used during experimentation.
-
Work piece Material
Aluminium 1275 alloy is used to manufacture pistons of diesel engines used in trucks of Tata Cummins.
Table 1: Work piece Specifications
S. No.
Work piece Specifications
Value of Work piece Specifications
1
Name of Work piece
Pistons of Diesel Engines used in Trucks of TATA CUMMINS
2
Work piece Material
Aluminium 1275 alloy
3
Length of Aluminium 1275 alloy Pistons
104.6 mm
4
Initial Diameter of Pistons on Finish Oval Turning Centre
102.18 mm
5
Final Diameter of Pistons on Finish Oval Turning Centre
101.78 mm ± 10 µm
6
Ovality on Pistons
150 µm
7
Coolant Temperature
180 C to 200 C
8
Coolant Material Company
FUCHS USA
Table 2: Physical Properties of Aluminum 1275 alloy
S. No.
Physical Properties of Aluminium 1275 alloy
Value of Physical Properties of Aluminium 1275 alloy
1
Density
( 2.698 × 103 ) (kg / m3)
2
Melting Point
810 oC to 820oC
3
Thermal Conductivity
145 (W/m · K) at 27 oC
4
Thermal Expansion
21 (µm/m · K) at (-100 oC to 20 oC)
5
Modulus of Longitudinal Elasticity
79 GPa
6
Poissons Ratio
0.33
7
Percentage of Si
11% to 13.5%
8
Percentage of Cu
0.8% to 1.5%
9
Percentage of Mg
0.7% to 1.2%
10
Percentage of Ni
0.7% to 1.3%
-
Taguchi method
Taguchi method utilizes orthogonal arrays of experimental design with a small number of experiments to reduce time and cost. Taguchi uses the loss function to measure the performance characteristic deviating from the desired value. The value of loss function is then further transformed to S/N ratio. Usually, there are three categories of the performance characteristic in the analysis of the S/N ratio, that is, lower the better, the higher the better and the nominal the better. The S/N ratio for each level of process parameters is computed based on the S/N analysis and the larger S/N ratio corresponds to the better performance characteristic. Therefore, the optimal level of the process parameter is the level with the highest S/N ratio. Next, a statistical analysis of variance (ANOVA) is performed to judge the statistical significant process parameters affecting the responses. With the S/N ratio analysis and ANOVA analysis, the optimal combination of the process parameters can be predicted. As surface roughness is to be improved, hence lower the better S/N ratio was used.
4. RESULTS AND DISCUSSION
-
Orthogonal array experiment
Table 3: Selection of Process Parameters with Code, Units and Range
S. No.
Symbol
Process Parameter
Unit
Range
1
A
Spindle speed
rpm
1000 3000
2
B
Feed rate
mm/rev
0.10 – 0.40
3
C
Depth of Cut
mm
0.10 1.20
4
D
Tool Nose Radius
mm
0.20 – 0.80
Table 4: Parameters, Codes and Level Values used for the Orthogonal Array
Parameter
Code
Level 1
Level 2
Level 3
Control factors
Spindle speed (rpm)
A
1000
1250
1500
Feed rate (mm/rev)
B
0.25
0.30
0.35
Depth of cut (mm)
C
0.30
0.35
0.40
Tool nose radius (mm)
D
0.40
0.60
0.80
The number of process parameters is 4 and the number of levels is 3 and hence, a L27 orthogonal array (three level orthogonal array) was selected for the analysis.
Table 5: The Basic Taguchi L27 (34) Orthogonal Array (with value)
Run
Control factors and levels
Theoritical Average Surface Roughness ( Ra) (µm) by formula
f 2
Ra 32r
A
(rpm) Spindle Speed
B
(mm/rev) Feed Rate
C
(mm) Depth of Cut
D
(mm) Tool Nose Radius
Experimental Average Surface Roughness
( Ra) (µm)
1
1000
0.25
0.30
0.40
7.89
4.88
2
1000
0.25
0.35
0.60
6.52
3.26
3
1000
0.25
0.40
0.80
5.67
2.44
4
1000
0.30
0.30
0.60
7.79
4.69
5
1000
0.30
0.35
0.80
6.84
3.52
6
1000
0.30
0.40
0.40
10.73
7.03
7
1000
0.35
0.30
0.80
10.83
4.79
8
1000
0.35
0.35
0.40
13.07
9.57
9
1000
0.35
0.40
0.60
10.19
6.38
10
1250
0.25
0.30
0.60
6.71
3.26
11
1250
0.25
0.35
0.80
6.53
2.44
12
1250
0.25
0.40
0.40
8.39
4.88
13
1250
0.30
0.30
0.80
6.79
3.52
14
1250
0.30
0.35
0.40
11.03
7.03
15
1250
0.30
0.40
0.60
8.19
4.69
16
1250
0.35
0.30
0.40
12.59
9.57
17
1250
0.35
0.35
0.60
9.97
6.38
18
1250
0.35
0.40
0.80
8.29
4.79
19
1500
0.25
0.30
0.80
5.53
2.44
20
1500
0.25
0.35
0.40
8.03
4.88
21
1500
0.25
0.40
0.60
5.73
3.26
22
1500
0.30
0.30
0.40
8.13
7.03
23
1500
0.30
0.35
0.60
7.89
4.69
24
1500
0.30
0.40
0.80
6.13
3.52
25
1500
0.35
0.30
0.60
9.97
6.38
26
1500
0.35
0.35
0.80
8.09
4.79
27
1500
0.35
0.40
0.40
12.75
9.57
-
Analysis of raw Data and S/N ratios
As response variable i.e. average surface roughness Ra is to be improved, hence smaller the better S / N ratio is used.
Where is the S/N ratio, yi is the individual surface roughness measurements and n is the number of repetitions.
Table 6: Results of the L27 (34) Experiment
Ex. No.
Average Surface Roughness Ra (µm)
S/N Ratio (Lower the Better)
1
7.89
-17.94
2
6.52
-16.29
3
5.67
-15.07
4
7.79
-17.83
5
6.84
-16.70
6
10.73
-20.61
7
10.83
-20.69
8
13.07
-22.33
9
10.19
-20.16
10
6.71
-16.54
11
6.53
-16.30
12
8.39
-18.48
13
6.79
-16.64
14
11.03
-20.85
15
8.19
-18.27
16
12.59
-22.00
17
9.97
-19.97
18
8.29
-18.37
19
5.53
-14.85
20
8.03
-18.09
21
5.73
-15.16
22
8.13
-18.20
23
7.89
-17.94
24
6.13
-15.75
25
9.97
-19.97
26
8.09
-18.16
27
12.75
-22.11
TOTAL SUM (T)
230.27
AVERAGE
8.53
Table 7: Average Effect Response Table for the Raw Data Table 8: Average Effect Response Table for S/N Ratio
LEVEL
Spindle speed
Feed Rate
Depth of cut
Tool nose radius
L1
8.84
6.78
8.47
10.29
L2
8.72
8.17
8.66
8.10
L3
8.03
10.64
8.45
7.19
Max- Min
0.81
3.86
0.21
3.10
Rank
3
1
4
2
LEVEL
Spindle speed
Feed Rate
Depth of cut
Tool nose radius
L1
-18.62
– 16.52
– 18.30
– 20.07
L2
-18.60
– 18.09
– 18.51
– 18.01
L3
-17.81
– 20.42
– 18.22
– 16.95
Max- Min
0.82
3.89
0.29
3.12
Rank
3
1
4
2
LEVEL
Spindle speed
Feed Rate
Depth of cut
Tool nose radius
L1
8.84
6.78
8.47
10.29
L2
8.72
8.17
8.66
8.10
L3
8.03
10.64
8.45
7.19
Max- Min
0.81
3.86
0.21
3.10
Rank
3
1
4
2
LEVEL
Spindle speed
Feed Rate
Depth of cut
Tool nose radius
L1
-18.62
– 16.52
– 18.30
– 20.07
L2
-18.60
– 18.09
– 18.51
– 18.01
L3
-17.81
– 20.42
– 18.22
– 16.95
Max- Min
0.82
3.89
0.29
3.12
Rank
3
1
4
2
Tables 7 and 8 specify the average effect response tables for raw data and S/N ratio, these tables help to find out which parameter will effect more significantly. From these tables it is concluded that feed rate variation has major effect on surface quality of turned parts than tool nose radius, spindle speeds and finally the depth of cut.
-
ANOVA (Analysis of variance) analysis
Te main purpose of the analysis of variance is to investigate the significant parameters.
Table 9: ANOVA Table for Responded Raw Data Table 10: ANOVA Table for Responded Raw Data (pooled)
Source
SS
DOF
V
F
Ratio
SS
P
Spindle Speed
3.45
2
1.73
3.09
2.33
1.82
Feed rate
68.83
2
34.42
61.46
67.71
52.75
Depth of Cut
0.25
2
0.13
0.23
-0.87
-0.68
Tool Nose Radius
45.68
2
22.84
40.79
44.56
34.71
ERROR
10.16
18
0.56
——-
——
11.40
T
128.37
26
——
——-
——
——
Source
SS
DOF
V
F
Ratio
SS
P
Spindle Speed
3.45
*
*
*
*
*
Feed rate
68.83
2
34.42
54.64
67.57
52.64
Depth of Cut
0.25
*
*
*
*
*
Tool Nose Radius
45.68
2
22.84
36.25
44.42
34.60
ERROR
10.16
18
0.63
——-
——
12.76
T
128.37
26
——
——-
——
——
Source
SS
DOF
V
F
Ratio
SS
P
Spindle Speed
3.45
2
1.73
3.09
2.33
1.82
Feed rate
68.83
2
34.42
61.46
67.71
52.75
Depth of Cut
0.25
2
0.13
0.23
-0.87
-0.68
Tool Nose Radius
45.68
2
22.84
40.79
44.56
34.71
ERROR
10.16
18
0.56
——-
——
11.40
T
128.37
26
——
——-
——
——
Source
SS
DOF
V
F
Ratio
SS
P
Spindle Speed
3.45
*
*
*
*
*
Feed rate
68.83
2
34.42
54.64
67.57
52.64
Depth of Cut
0.25
*
*
*
*
*
Tool Nose Radius
45.68
2
22.84
36.25
44.42
34.60
ERROR
10.16
18
0.63
——-
——
12.76
T
128.37
26
——
——-
——
——
Pie Chart for Percent Effect of Parameters (P) of ANOVA Table
for Responded Raw Data
Pie Chart for Percent Effect of Parameters (P) of ANOVA Table
for Responded Raw Data
11.4
11.4
1.82
1.82
Spindle Speed Feed Rate
Depth of Cut
Spindle Speed Feed Rate
Depth of Cut
34.71
34.71
52.75 Tool Nose
Radius Error
52.75 Tool Nose
Radius Error
-0.68
-0.68
Depth of Cut
Tool Nose Radius Error
Depth of Cut
Tool Nose Radius Error
Pie Chart for Percent Effect of Parameters (P) of ANOVA Table for Responded Raw Data (Pooled)
12.76
52.64
34.6
Pie Chart for Percent Effect of Parameters (P) of ANOVA Table for Responded Raw Data (Pooled)
12.76
52.64
34.6
Figure 1: Pie Chart for Percent Effect of Parameters (P) of ANOVA Table for Responded Raw Data and Responded Raw Data (Pooled)
Table 11: ANOVA Table for S/N Data Table 12: ANOVA Table for S/N Data (pooled)
Source
SS
DOF
V
F
Ratio
SS
P
Spindle Speed
3.92
2
1.96
4.36
3.02
2.38
Feed Rate
69.14
2
34.57
76.82
68.24
53.84
Depth of Cut
0.42
2
0.21
0.47
-0.48
-0.38
Tool Nose Radius
45.26
2
22.63
50.29
44.36
34.99
ERROR
8.02
18
0.45
——-
—–
9.17
T
126.75
26
——
——-
——
——
Source
SS
DOF
V
F
Ratio
SS
P
Spindle Speed
3.92
*
*
*
*
*
Feed rate
69.14
2
34.57
93.43
68.40
53.97
Depth of Cut
0.42
*
*
*
*
*
Tool Nose Radius
45.26
2
22.63
61.16
44.52
35.12
ERROR
8.02
22
0.37
——-
——
10.91
T
126.75
26
——
——-
——
——
Source
SS
DOF
V
F
Ratio
SS
P
Spindle Speed
3.92
2/p>
1.96
4.36
3.02
2.38
Feed Rate
69.14
2
34.57
76.82
68.24
53.84
Depth of Cut
0.42
2
0.21
0.47
-0.48
-0.38
Tool Nose Radius
45.26
2
22.63
50.29
44.36
34.99
ERROR
8.02
18
0.45
——-
—–
9.17
T
126.75
26
——
——-
——
——
Source
SS
DOF
V
F
Ratio
SS
P
Spindle Speed
3.92
*
*
*
*
*
Feed rate
69.14
2
34.57
93.43
68.40
53.97
Depth of Cut
0.42
*
*
*
*
*
Tool Nose Radius
45.26
2
22.63
61.16
44.52
35.12
ERROR
8.02
22
0.37
——-
——
10.91
T
126.75
26
——
——-
——
——
Spindle Speed Feed Rat e Dept h of Cut
Tool Nose Radius
Error
Spindle Speed Feed Rat e Dept h of Cut
Tool Nose Radius
Error
Pie Chart for Percent Effect of Parameters (P) ANOVA
Table for S/N Data
Pie Chart for Percent Effect of Parameters (P) ANOVA
Table for S/N Data
9.17
9.17
2.38
2.38
34.99
34.99
53.84
53.84
– 0.38
– 0.38
Depth of Cut
T ool Nose r adi us E r r or
Depth of Cut
T ool Nose r adi us E r r or
Pie Chart for Percent Effect of Parameters (P) ANOVA Table for S/N Data (Pooled)
10.91
53.97
35.12
Pie Chart for Percent Effect of Parameters (P) ANOVA Table for S/N Data (Pooled)
10.91
53.97
35.12
Figure 2: Pie Chart for Percent Effect of Parameters (P) of ANOVA Table for S/N Data and S/N Data (Pooled)
Tables 9 and 10 show the result of the analysis of variance (ANOVA) for average surface roughness Ra for raw data and tables 11 and 12 show the analysis of variance (ANOVA) for S/N data. The analysis of variance was carried out for a 95% confidence level. The ANOVA table 9 shows that, the F value corresponding to all parameters are not greater than the tabulate value of F0.05 = 4.41. Feed rate and tool nose radius have F values greater than F0.05 = 4.41. Hence feed rate and tool nose radius are significant parameters and their optimal values are to be considered for the analysis. Spindle speed and depth of cut are insignificant parameters and their economical values are to be considered for the analysis.
All of these are responsible and have more or less effect on average surface roughness Ra value. The influences of feed rate (P = 52.75%) and tool nose radius (P = 34.71%) are the most significant. The influences of spindle speed (P = 1.82%) and depth of cut (P = – 0.68%) are less significant influencing factors.
-
Main effect plots
Feed rate and tool nose radius are shown to have a strong effect on surface roughness and their S/N ratios. The spindle speed and depth of cut had a smaller effect, as evidenced by the shallow slope of the lines.
The S/N ratio and Ra effects data plotted in these graphs can be used to determine the optimal set of parameters from this experimental design. As seen in figure 3, the optimal spindle speed was at level 3 (1500 rpm), the optimal feed rate was at level 1 (0.25 mm/rev), the optimal depth of cut was at level 3 (0.40 mm) and the optimal tool nose radius was at level 3 (0.80 mm). Therefore, the optimized combination of levels for the four control factors from the analysis so far was (A3-B1-C3-D3). The final confirmation run would be used to verify that this combination was ideal.
Main Effects Plot for Means
Data Means
Spindle Speed (rpm) Feed Rate (mm/rev)
11
10
9
8
7
-16
-17
-18
-19
-20
Main Effects Plot for SN ratios
Data Means
Spindle Speed (rpm) Feed Rate (mm/rev)
Main Effects Plot for Means
Data Means
Spindle Speed (rpm) Feed Rate (mm/rev)
11
10
9
8
7
-16
-17
-18
-19
-20
Main Effects Plot for SN ratios
Data Means
Spindle Speed (rpm) Feed Rate (mm/rev)
0.30
0.35
0.40
0.4
0.6
0.8
Signal-to-noise: Smaller is better
0.30
0.35
0.40
0.4
0.6
0.8
Signal-to-noise: Smaller is better
1000 1250 1500 0.25
1000 1250 1500 0.25
0.30
Tool Nose Radius (mm)
0.30
Tool Nose Radius (mm)
0.35
0.35
Depth of Cut (mm)
Depth of Cut (mm)
11
10
9
8
7
11
10
9
8
7
1000 1250
1000 1250
1500
1500
0.25
0.25
-16
-17
-18
-19
-20
-16
-17
-18
-19
-20
Depth of Cut (mm)
Depth of Cut (mm)
0.30 0.35
Tool Nose Radius (mm)
0.30 0.35
Tool Nose Radius (mm)
0.30
0.30
0.35
0.35
0.40
0.40
0.4
0.4
0.6
0.6
0.8
0.8
Mean of Means
Mean of Means
Mean of SN ratios
Mean of SN ratios
Figure 3: Response Effects and S/N Ratio Effects for Spindle Speed, Feed Rate, Depth of Cut and Tool Nose Radius
-
Prediction of optimal value of parameters
The estimated mean of the response characteristic (SSR) can be determined
B1 D3 T
Where µ = SSR = Scatter of surface roughness
µ = SSR = 6.78 + 7.19 8.53 = 5.44 µm
The 95% confidence interval of conformation experiments (CICE) and of population (CIPOP) was calculated.
1
1
DOF associated in theestimate of mean responce
DOF associated in theestimate of mean responce
N
N
neff
N= 27, neff = 5.4, Ve= 0.63, R= 3, F0.05 (1, fe) = 4.41, fe = 18
So, CI
F (1,f ) V 1
1 = 1.2
CE
e e n
eff
CI
F (1,fe ) Ve
R
R
= 0.96
POP n
eff
CICE = 1.20, CIPOP = 0.96
The predicted optimal ranges (for a confirmation run of ten experiments) is
(Mean SSR CI) < SSR < (Mean SSR + CI)
4.48 < SSR < 6.40 (on the basis of CIPOP )
4.24 < SSR < 6.64 ( on the basis of CICE )
-
Mathematical model
A mathematical model can be developed for the average surface roughness Ra value during finish oval turning of Alumimium 1275 alloy pistons by using MATLAB software.
Ra 3.6813 0.0016V 38.6111 f
0.1778d 7.7528r
-
Confirmation experiments
The confirmation experiment is the final step in verifying the conclusions from the previous round of experimentation. A sample of 10 work pieces of the same matrial and dimensions described earlier was turned by using the selected control parameter values. The average surface roughness Ra value was then measured by using the setup. Predicted values were compared with the experimental values to confirm its effectiveness in the analysis of average surface roughness Ra value.
Average Surface Roughness Ra (µm)
Average Surface Roughness Ra (µm)
5.1
5
Results of the Confirmation Experiments
4.97
4.89 4.91
4.94
Table 13: Results of the Confirmation Experiments
4.9
-
4.7
4.6
4.5
4.4
4.3
4.59
4.71
4.83
4.64
4.53
4.77
Sample No.
Average surface roughness Ra (µm)
1
4.59
2
4.71
3
4.83
4
4.97
5
4.64
6
4.53
7
4.89
8
4.91
9
4.77
10
4.94
Average surface roughness Ra (µm) mean
4.78
Sample No.
Average surface roughness Ra (µm)
1
4.59
2
4.71
3
4.83
4
4.97
5
4.64
6
4.53
7
4.89
8
4.91
9
4.77
10
4.94
Average surface roughness Ra (µm) mean
4.78
Experimental Value
1 2 3 4 5 6 7 8 9 10
Experiment Number
Figure 4: Results of the Confirmation Experiments Based on these results, it can be concluded with 95% confidence
that, by turning samples using the setup described in this study gave the average surface roughness Ra value within the interval as mentioned in above.
Predicted value of average surface roughness Ra by using plot of average response curves SSR = 5.44 µm. Predicted value of average surface roughness Ra by using MATLAB software = 4.66 µm.
Mean of experimental value of average surface roughness Ra for confirmation results by using optimum values of process parameters = 4.78 µm.
Range of experimental value of average surface roughness Ra for confirmation results by using optimum values of process parameters = 4.5 µm to 5 µm.
-
CONCLUSIONS
The value of average surface roughness Ra was improved in the range of the required value by using optimal values of process parameters. The use of Taguchis parameter design method certainly decreased the unnecessary wastage of time for performing a large number of total experiments to improve average surface roughness Ra value. Quality was improved. Experimental cost was reduced. Taguchi parameter design process was applied using a specific set of control parameters and a response variable. The control parameters were spindle speed, feed rate, depth of cut and tool nose radius. The response variable was average surface roughness Ra value. L27 (34) orthogonal array was used with four control parameters and experiments were conducted with a sample of 27 work pieces. It was found that the control parameters had varying effects on the response variable, with feed rate and tool nose radius having the highest effects. The measurement of the work pieces in the confirmation run of 10 samples led to the conclusion that the selected parameter values from this process produced average surface roughness Ra value that was in the range of required average surface roughness Ra value. Optimum parameter setting for surface roughness is obtained at a spindle speed of 1500 rpm, feed rate of 0.25 (mm/rev), depth of cut of 0.40 mm and tool nose radius of 0.80 mm. Formulation of equation is done by using MATLAB with the help of which average surface roughness Ra value can be predicted.
Ra 3.6813 0.0016V 38.6111 f
0.1778d 7.7528r
REFERENCES
-
Ahmet Hascalik and Ulas Caydas (2008), Optimization of Turning Parameters for Surface Roughness and Tool Life based on the Taguchi Method, International Journal of Advanced Manufacturing Technology, 38: 896 903.
-
M. Kaladhar, K. Venkata Subbaiah and Ch. Srinivasa Rao (2012), Parametric Optimization during Machining of AISI 304 Austenitic Stainless Steel Using CVD coated DuratomicTM Cutting Insert, International Journal of Industrial Engineering Computation 3, 577 586.
-
Ali Riza Motorcu (2010), The Optimization of Machining Parameters Using the Taguchi Method for Surface Roughness of AISI 8660 Hardened Alloy Steel, Journal of Mechanical Engineering 56, 6, 391 401.
-
Sijo M. T. and Biju N. (2010), Taguchi Method for Optimization of Cutting Parameters in Turning Operations, Proceedings of International Conference on Advances in Mechanical Engineering, DOI : 02. AMAE 2010.01.536.
-
Ashok Kumar Sahoo and Bidyadhar Sahoo (2011), Surface roughness model and parametric optimization in finish turning using coated carbide insert: Response surface methodology and Taguchi approach, Proceedings of International Journal of Industrial Engineering Computation 2, 819 830.
-
E. Daniel Kirby, Zhe Zhang, Joseph C. Chen and Jacob Chen (2006), Optimizing surface finish in a turning operation using the Taguchi parameter design method, International Journal of Advanced Manufacturing Technology, 30: 1021 1029.
-
M. Nalbant, H. Gokkaya and G. Sur (2007), Application of Taguchi method in the optimization of cutting parameters for surface roughness in turning, Materials and Design 28, 1379 1385.www.elsevier.com/locate/matdes.
-
N. Muthukrishnan and J. Paulo Davim (2009), Optimization of machining parameters of Al/SiC-MMC with ANOVA and ANN analysis, Journal of Materials Processing Technology 209, 225 232.
-
Mohan Singh, Dharmpal Deepak and Manoj Kumar Singla (2010), A robust parameter design study in turning bright mild steel based on taguchi method, International Journal on Emerging technologies1 (2):100 105, ISSN: 0975 8364.
-
Tian-Syung Lan and Ming-Yung Wang (2009), Competitive parameter optimization of multi quality CNC turning, International Journal of Advanced Manufacturing Technology, 41: 820 826.
-
Indrajit Mukherjee, Pradip Kumar Ray (2006), A Review of Optimization Techniques in Metal Cutting Processes, Elsevier, Computers and Industrial Engineering 50, 15-34, www.elsevier.com.
-
H. Yanda, J. A. Ghani, M.N.A.M. Rodzi, K. Othman and C.H.C. Haron (2010), Optimization of Material Removal Rate, Surface Roughness and Tool Life on Conventional Dry Turning of FCD 700, International Journal of Mechanical and Materials Engineering, Vol. 5., No. 2, 182 190.
-
Ilhan Asilturk and Harun Akkus (2011), Determining the Effect of Cutting Parameters on Surface Roughness in Hard Turning using the Taguchi Method, Measurement 44, 1697 1704, www.elsevier.com.
-
M. Kaladhar, K. V. Subbaiah, Ch. Srinivasa Rao and K. Narayana Rao (2011), Application of Taguchi Appproach and Utility Concept in Solving the Multi Objective Problem when Turning AISI 202 Austenitic Stainless Steel, Journal of Engineering Science and Technology 4 (1), 56 61.
-
M. Kaladhar, K. Venkata Subbaiah, Ch. Srinivas Rao and K. Narayana Rao (2010), Optimization of Process Parameters in Turning of AISI 202 Austenitic Stainless Steel, ARPN Journal of Engineering and Applied Sciences, Vol. 5, No. 9, ISSN 1819 6608.
-
nirban Bhattacharya, Santanu Das, P. Majumdar and Ajay Batish (2009), Estimating the Effect of Cutting Parameters on Surface Finish and Power Consumption during High Speed Machining of AISI 1045 Steel Using Taguchi Design and ANOVA, Springer Production Engineering Res. Devel., 3, 31 40.
-
Jitendra Verma, Pankaj Agrawal and Lokesh Balpai (2012), Turning Parameter Optimization for Surface Roughness of ASTM A 242 Type-1 Alloys Steel by Taguchi Method, International Journal of Advances in Engineering and Technology, ISSN: 2231 1963, Vol. 3, Issue 1, 255
261.
-
Marimuthu Uthayakumar, Gopalakrishnan, Prabhakaran, Sivanandham Aravindan and Jonna Venkata Sivaprasad, (2009), Precision Machining of an Aluminium Alloy Piston Reinforced with a Cast Iron Insert International Journal of Precision Enginering and Manufacturing, Vol. 10, No. 1, pp. 7 13.
-
Hardeep Singh, Rajesh Khanna and M. P. Garg (2011), Effects of Cutting parameters on MRR and Surface Roughness in Turning EN 8, Recent Trends in Engineering Research, Vol. 1, No. 1.
-
Anil Gupta, Hari Singh and Aman Aggarwal (2011), Taguchi Fuzzy Multi Output Optimization (MOO) in High Speed CNC Turning of AISI P 20 Tool Steel, Elsevier Expert Systems with Applications 38, 6822 6828, www.elsevier.com .
-
S. Thamizhmanil, S. Saparudin, S. Hasan (2007), Analysis of Surface Roughness by Turning Process using Taguchi Method, Journal of Achievements in Materials and Manufacturing Engineering, Volume 20, Issues 1 2.
-
D. Philip Selvaroj, P. Chandramohan (2010), Optimization of Surface Roughness of AISI 304 Austenitic Stainless Steel in Dry Turning Operation Using Taguchi Design Method, Journal of Engineering, Science and Technology, Vol. 5, No. 3, 293 301.
-
V. R. Kagade, R. R. Deshmukh (2011),Experimental Investigation of Turning Operation using Carbide Inserts, International Journal of Applied Research in Mechanical Engineering, Volume 1, Issue 1.
-
Ay Mustafa, Karagol Tanju (2011),Investigation of the Machinability of the Al 7075 alloy using DLC coated cutting tools, Scientific Research and Essays, Volume 6 (1), pp. 44 51, http;// www.academicjournals.org/SRE, ISSN 1992 2248 (c), Academic Journals.
-
Dr. E. Daniel Kirby (2010), Optimizing the Turning Process toward an Ideal Surface Roughness Target, Journal of Industrial Technology, Volume 26.
-
Ross P. J. (2012), Taguchi Techniques for Quality Engineering, Tata McGraw Hill Education Private Limited, New Delhi.
-
Roy RK (2001), Design of Experiments Using the Taguchi Approach, John Wiley & Sons, Inc., New York.
-
Mitra A. (2010), Fundamental of Quality control and improvement, PHI Learning Private Limited, Delhi.
-
Montgomery D. (2009), Design and Analysis of Experiments, John Wiley India (P.) Ltd.