
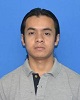
- Open Access
- Authors : Raymond Wahlang , S. S. Chaturvedi
- Paper ID : IJERTV9IS050128
- Volume & Issue : Volume 09, Issue 05 (May 2020)
- Published (First Online): 09-05-2020
- ISSN (Online) : 2278-0181
- Publisher Name : IJERT
- License:
This work is licensed under a Creative Commons Attribution 4.0 International License
Relationship Between Above-Ground Biomass and Different Vegetation Indices of Forests of Ri-Bhoi District, Meghalaya, India
Raymond Wahlang
Department of Environmental Studies North-Eastern Hill University Shillong- 793022, Meghalaya, India
S. S. Chaturvedi
Department of Environmental Studies North-Eastern Hill University Shillong- 793022, Meghalaya, India
Abstract- Estimates of biomass in forests are generally based on allometric models relating either carbon or biomass to diameter at breast height (DBH). The aim of this study is to investigate the relationship between the above-ground biomass (AGB) and four different vegetation indices, viz. NDVI (Normalized Difference Vegetation Index), RDVI (Renormalized Difference Vegetation Index), MSAVI (Modified Soil Adjusted Vegetation Index) and OSAVI (Optimized Soil Adjusted Vegetation Index) of the subtropical broadleaf/ subtropical evergreen and semi evergreen forests of Nongkhyllem Wildlife Sanctuary, and Umsaw Reserve forests in Ri- Bhoi district of Meghalaya in Northeast India. The main objective of this study is to correlate the biomass obtained by allometric models through field investigations and the values of the Vegetation Indices obtained from Landsat 8 satellite imagery.
Keywords NDVI; RDVI; MSAVI; OSAVI; Nongkhyllem Wildlife Sanctuary; Umsaw Reserve Forest
-
INTRODUCTION
Biomass assessment is of great significance in the fields of forestry, ecology, economics and environmental science. It plays a very important role in determining forest ecosystems productivity through carbon (C) budgeting, and has complemented studies on the role that forests have in the global carbon cycle (Hall et al., 2006; Somogyi et al., 2006; Zianis and Mencuccini, 2004). Biomass is a key parameter in the global carbon cycle as it can be used to quantify the carbon sequestration rate of ecosystems or carbon pools; this also helps in quantifying the fluxes of greenhouse gases absorbed and released from terrestrial pools to the atmosphere through changing patterns of land cover and land use (Cairns et al., 2003). Forest biomass is an indicator of carbon sequestration and most researchers estimate carbon by assuming the carbon content of dry biomass to be a constant 50% by weight (Brown, 1986; Montagnini and Porras, 1998) as opposed to other researchers who have determined the total concentration of carbon from biomass to be 45% of its total weight (Whittaker and Likens, 1973). The total biomass is usually divided into two fractions viz. above-ground biomass (AGB) and below-ground biomass (BGB) and most methods of biomass assessments are determined for the above-ground biomass (AGB) of tree species as this fraction generally represents a greater, more significant fraction of the total biomass in forest ecosystems and this fraction is comparably less cumbersome to deal with during field measurements (IPCC, 2007). In any carbon related study, determining the above-ground forest biomass is the juxtaposition for estimating the carbon sequestration potential as well as the
carbon fluxes and thus assists in ascertaining the contribution that terrestrial ecosystems such as tropical forests have on the global carbon cycle. Moreover, estimates of AGB can also be used to predict root biomass, which is generally estimated to be 20% of the above-ground forest biomass (Achard et al., 2002)
Traditional biomass assessment methods based on field measurements are the most accurate methods; however, they are difficult to conduct over large areas and are costly, time consuming, and labour intensive (Attarchi and Gloaguen, 2014) hence Remote Sensing data now plays a significant role in the estimation of biomass over large areas in tropical regions owing to the availability and accuracy of high resolution images. RS technology has been applied to biomass assessment in many studies (Maynard et al., 2007; Kankare et al., 2013; Wannasiri et al., 2013). Remote Sensing can obtain forest information over large areas at a reasonable cost and with acceptable accuracy based on repetitive data collection with minimal effort (Lu, 2006). Many studies have shown that the method of determining relationships between field measurements and RS data and then extrapolating these relationships over large areas is very useful (Foody, Boyd and Cutler, 2003). To estimate the relationship between the field measurement of above-ground biomass and the Remote Sensing data, researchers have used linear regression models (Kobayashi et al., 2012) and multiple regressions with or without stepwise selection (Lu, 2006; Zheng et al., 2004; Coulibaly et al., 2008).
There are innumerable methods employed for mapping and estimating the above-ground forest biomass for all the different land-cover types; to name one: the judicious use of Landsat imagery, which are medium-resolution satellite images in estimating the attributes of forests through direct correlations or stepwise regression analyses with spectral bands, band ratios, or vegetation indices is a very practical method (Main-Knorn et al., 2011; Terakunpisut et al., 2007). Generally, mapping of changes in land cover cannot be accurately determined through poor quality medium and low resolution satellite images, in this context however, mapping of large areas by using high-resolution images is expensive and users are required to possess a certain degree of technical skill for data interpretation; thus, these issues pose a problem for developing countries who rely on open sources for data acquisition or who lack skilled personnel. Landsat series data is the most commonly used data for many geoprocessing applications as the data can be obtained easily for free or at a fair price.
Data sources such as forest inventory (field estimations), land use patterns, elevation, and Remote Sensing data can be combined and used for the prediction or various vegetation variables over large areas (Labrecque, 2006). A hybrid supervised/unsupervised classification approach coupled with a geographical information systems (GIS) analysis has been employed to improve land use/cover mapping for Landsat data (Labrecque, 2006; Ohmann and Gregory, 2002; Kamusoko and Aniya, 2009). For the estimation of biomass, many studies show that satellite based vegetation indices are most commonly employed (Schlerf et al., 2005; Foody et al., 2003; Hurcom and Harrison, 1998). The present study seeks to correlate the relationship between above-ground biomass (AGB) and the different vegetation indices viz. NDVI (Normalized Difference Vegetation Index), RDVI (Renormalized Difference Vegetation Index), MSAVI (Modified Soil Adjusted Vegetation Index) and OSAVI (Optimized Soil Adjusted Vegetation Index). Vegetation has a high near infrared reflectance due to the scattering of radiation by mesophyll cells of leaves and low red reflectance due to absorption by chlorophyll pigments, this is the fundamental concept in understanding vegetation indices which are used in a variety of satellite based vegetation index models for the evaluation of vegetation parameters such as leaf area index, biomass and other physiological activities (Verrelst et al., 2008; Baret and Guyot, 1991). Mathematical transformations of the original spectral reflectance used in the interpretation of biomass and land cover are known as vegetation indices (Patel et al., 2007; He et al., 2006; Rahman et al., 2003). Vegetation indices (VIs) are used to remove the variations caused in spectral reflectance measurement while measuring biophysical properties caused due to soil background, sun view angles, and atmospheric conditions (Lu, 2006). There are studies that suggest the existence of a signficant positive correlation between biomass and vegetation indices (Das and Singh, 2012; Heiskanen, 2006; Steininger, 2000; Boyd et al., 1999; Hurcom and Harrison, 1998) nonetheless; other studies have shown poor results (Schlerf et al., 2005; Foody et al., 2003). This field based and remote sensing approach is of great significance in todays contemporary world as the same can be used in the regulation of global carbon cycles under the REDD+ initiative.
-
MATERIALS AND METHODS
-
The study area
The area of study is located in Ri-Bhoi district of the state of Meghalaya in Northeast India. The district lies between 90°5 to 91°16 N latitude and 25°40 to 25°21 E longitude. It is bounded on the north by Kamrup district and on the East by Jaintia Hills and Karbi Anglong district of Assam and on the West by West Khasi Hills district. The recorded forest area of the state is about 17,146 square kilometres which is 76.44
% of its geographical area (FSI 2017). According to legal classification, Reserve forests, Protected forests and
unclassified forests constitute 11.71%, 0.13% and 88.16% of the total forests, respectively. The control of unclassified forests mostly rests with the autonomous district councils of Khasi hills, Jaintia hills and Garo hills. Ri-Bhoi district is one of the components of the Meghalaya plateau and has an estimated area of 2448 sq. km, with a forest area of about 50% of the total area of the district; the physiographical features of the district are almost similar to that of East Khasi Hills district. Nongkhyllem Reserve forest has a total area of 125.91 sq. km of which 29 sq. km was designated as a wildlife sanctuary whereas Umsaw Reserve Forest has an area of 0.44 sq. km (Meghalaya Forest and Environment Department, 2019). Ri-Bhoi district experiences a varied range of climate; similar to the tropical climate of Assam at the adjoining areas near Assam and a temperate climate at the areas adjoining of the East Khasi Hills district. The areas bordering Assam experience hot – humid weather during summer seasons with an average temperature of 30 ° C, especially during the month of May to July of whereas the other areas adjoining Umiam and the East Khasi Hills district, the climate is relatively cold in winters and comparatively pleasant during the summer months. Thus, the temperature in the district ranges approximately between 2° C and 36° C. The district receives an average annual rainfall of 1636.46 mm. The maximum rainfall is between the months of June and August. The forest type of the district is dominated by subtropical broadleaf, subtropical evergreen and semi evergreen forests. (Barik et al., 2006). The main tree species are Tectona grandis, Shorea robusta, Castanopsis spp. and Schima wallichii.
-
Methodology
-
Field assessment of above-ground biomass
In the present study stratified random sampling was used for estimation of biomass in which 12 plots were laid down in different homogenous strata depending on the accessibility of the locations. The locations of the sampling sites were recorded by using a handheld GPS. The layout of all sampling plots were 31.6×31.6 m for vegetation, 5×5 m for shrubs and 1×1 m for herbs, the plots of shrubs and herbs were within the plot of tree quadrats. The field data collected was in the form of DBH of tree and tree height. Further the biomass of trees was calculated by using different biomass equations which are related to its biophysical variables such as DBH, height, etc. (Zianis et al., 2005). The biomass of each tree was calculated by computing the exponential of the different tree constants while adding and subtracting with the natural logarithm of the DBH of the individual trees as shown in the given table. The coefficient of determination (R2) of the different models (table
1) was then obtained by correlating the generated value of above-ground biomass and the diameter at breast height (DBH). The model which depicted the highest value of R2 was used as the main model and this model was then used for correlation of the biomass value with the different vegetation indices.
Figure 1: Study area
Table 1: Allometric Models
Model
Allometric Equation
Parameters
Chambers et al., (2001)
Y= exp [-0.37+0.33 ln (D) + 0.933 ln(D)2 0.122 ln(D)3]
Chave et al., (2001)
Y= exp (-2.00+2.42) ln (D)
FAO. 3.2.5. (1997)
Y= 21.297- 6.953 (D) + 0.740(D2)
Diameter (D) in cm
FAO. 3.2.4. (1997)
Y= exp {-2.134+2.530*ln (D)}
FAO. 3.2.3. (1997)
Y= 42.69-12.800(D) + 1.242(D2)
Brown et al., (1989)
Y= 38.4908-11.7883 (D) + 1.1926 D2
Brown and Iverson (1992)
Y= 1.276+0.034 (D2*H)
Diameter (D) and Height (H) in cm
Brown et al., (1989)
Y= exp [-3.114+0.972*ln (D2H)]
-
Image acquisition and pre-processing
Landsat 8 satellite imagery for the month of October 2016 was used in this study as the field assessment was done in this month. Layer stacking of the different bands was performed on ArcGIS 10.3 software for desktop. The different tiles (paths/ rows) obtained were mosaicked and area of study was clipped using a shapefile of the area of study. The downloaded images are in Universal Transverse Mercator projection and it was re-projected to Geographic WGS 84 zone 46 N, spheroid and datum Everest. A point shapefile was generated for the sampling sites and overlaid on the corrected image for
verification of the matching plots with respect to the ground. The Normalized Difference Vegetation Index of the areas of study was then obtained automatically from the composite image, as for the rest of the vegetation indices; raster calculation was performed using the raster calculator tool. The NDVI, RDVI, MSAVI and OSAVI pixel values thus obtained were then used to carry out the correlation analysis between the VIs and AGB.
-
Vegetation Indices
-
The normalized difference vegetation index (Rouse et al., 1974) is the most commonly and widely used VIs for many
applications in forestry related studies. The NDVI is the ratio between maximum absorption of red wavelength due to chlorophyll pigments and maximum reflectance of infrared wavelength owing to leaf cellular structure (Piao et al., 2007; Rahman et al., 2003; Paruelo et al., 1997. Image noise such as topographic variations, illumination differences, cloud shadows and atmospheric attenuation, which are usually present in the separate bands has been reduced in NDVI (Huete et al., 2002); however, in dense and multi-layered canopy, NDVI shows some saturation (Tenkabail et al., 2000; Baret and Guyot, 1991; Lillesaeter, 1982). The NDVI is given by the equation (Rouse et al., 1974) in table 2. Therefore substantial efforts were taken in improving and developing new vegetation indices like Renormalized Difference Vegetation Index (RDVI; Roujean and Breon, 1995). RDVI index is a modification of NDVI. The RDVI was developed to
be a more advanced index, which would have the combined advantage of the Difference Vegetation Index (DVI = NIR – Red; Jordan, 1969) and the NDVI. The soil reflectance is dependent on various factors viz. soil moisture, organic matter (OM), soil types and the presence of iron oxide (Hoffer 1978). Dry soils exhibit higher reflectance in comparison to wet in the visible, mid infra-red and near infra-red spectrum (Hoffer and Johannsen 1969). Soil adjusted vegetation indices are used for reducing the effect of soil background reflectance. A modified soil adjusted vegetation index (MSAVI) developed by Qi et al. (1994) replaces the manual adjustment factor L, with a self-adjustment L. nother soil adjusted vegetation index (OSAVI) developed by Rondeaux et al., 1996 gives satisfactory results in reduction of soil noise for both low and high vegetation cover conditions. The different vegetation indices used in this study in given in table 2.
Table 2: Vegetation Indices
Vegetation Index
Equation
Reference
NDVI
( )
=
( + )
Rouse et al. (1974)
RDVI
( )
=
( + )
Roujean and Breon (1995)
MSAVI
1
= [ 2 + 1 (2 + 1)2 8 ( )] 2
Qi et al. (1994)
OSAVI
= (1 + 0.16)( )/( + + 0.16)
Rondeaux et al. (1996)
-
-
RESULTS AND DISCUSSIONS
-
Above-ground Biomass
Model
R2
Chambers et al., (2001)
0.89
Chave et al., (2001)
0.71
FAO. 3.2.5. (1997)
0.80
FAO. 3.2.4. (1997)
0.68
FAO. 3.2.3. (1997)
0.79
Brown et al., (1989)
0.79
Brown and Iverson (1992)
0.77
Brown et al., (1989)
0.79
Model
R2
Chambers et al., (2001)
0.89
Chave et al., (2001)
0.71
FAO. 3.2.5. (1997)
0.80
FAO. 3.2.4. (1997)
0.68
FAO. 3.2.3. (1997)
0.79
Brown et al., (1989)
0.79
Brown and Iverson (1992)
0.77
Brown et al., (1989)
0.79
The biomass assessment was done using the different allometric models as shown in table 1. The most promising allometric model was Chambers et al. (2001) as it showed higher correlation between above-ground biomass (AGB) and diameter at breast height (DBH), thus this model was more suited for obtaining the biomass values from the different plots. The biomass values contributed by shrubs and herbs was determined through destructive method, the data was then extrapolated to all the separate plots and added with the tree biomass values. Table 3 shows the respective allometric models used as well as the R2 value of each models after
Table 3: R2 values of different allometric models
correlation between above-ground biomass and diameter at breast height.
-
Comparative correlation analysis between above-ground biomass and vegetation indices
Comparative analysis of biomass and the different vegetation indices revealed the following results as shown in table 4 which indicates that the vegetation indices have significant strong positive correlations with above-ground biomass. The linear model with the highest significance was the NDVI model with an R2 value of 0.785 which is then followed by MSAVI with an R2 value of 0.773, then RDVI with an R2 value of 0.753 and lastly OSAVI with and R2 value of 0.7185. The results show that the correlation between NDVI/ RDVI/ MSAVI/ OSAVI and above-ground biomass is highly significant.
Table 4: R2 values of AGB and Vegetation Indices
Vegetation Index
R2
NDVI
0.78
RDVI
0.75
MSAVI
0.77
OSAVI
0.71
Table 4 represents the regression analysis obtained from correlation between the field biomass and the corresponding pixel values generated from the rasters after employing the different methodologies (table 2). The following figures (figures 2, 3, 4 and 5) also show the computed maps along with their pixel values that have been generated for NDVI, RDVI, MSAVI and OSAVI.
0.35 NDVI VS BIOMASS
0.3
0.25
0.2 y = 0.0025x + 0.1967
0.15
0.1
0.05 R² = 0.78
0
0 10 20 30 40 50
BIOMASS
RDVI
RDVI
NDVI
NDVI
Figure 2: Computed NDVI and relationship between NDVI and Biomass
60 RDVI VS BIOMASS
50
40
30 y = 0.7x + 15.926
20
10 R² = 0.75
0
0 10 20 30 40 50
BIOMASS
MSAVI
MSAVI
Figure 3: Computed RDVI and relationship between RDVI and Biomass
1.2 MSAVI VS BIOMASS
1
0.8
y = 0.0051x + 0.7639
0.6
0.4
R² = 0.77
0.2
0
0 10 20 30 40 50
BIOMASS
Figure 4: Computed MSAVI and relationship between MSAVI and Biomass
OSAVI VS BIOMASS
0.45
0.4
0.35
0.3
0.25
0.2 y = 0.0041x + 0.1791
0.15
0.1
0.05 R² = 0.71
0
0 10 20 30 40 50
BIOMASS
OSAVI
OSAVI
Figure 5: Computed OSAVI and relationship between OSAVI and Biomass
-
-
CONCLUSION
The different vegetation indices showed positive correlation with the aboveground biomass of the subtropical broadleaf/ subtropical evergreen forests of Ri-Bhoi district; this study highlights the importance of vegetation indices in forestry related studies and this technique can be used for conservation related applications and management of forest resources. The application of remote sensing techniques have provided a more rapid, more efficient and timely estimates of biomass for the scientific management of forest resources as compared to the traditional approach of biomass assessment which are cumbersome, labour intensive and time consuming. The study sites most likely had large amount of photosynthetically active vegetation, hence more reflectance, thus more vegetation implies a higher correlation with biomass, and this property however is reduced during the dry months due to the annual senescence of the deciduous trees and the low productivity during these months.
ACKNOWLEDGMENT
The authors would like to acknowledge the Department of Forests and Environment, Government of Meghalaya for granting the permissions required for field visits to Umsaw Reserve Forests and Nongkhyllem Wildlife Sanctuary, as well as the Department of Science and Technology, Government of India for sponsorship of the study
REFERENCES
-
Attarchi, S. and Gloaguen, R. (2014). Improving the Estimation of Above-ground Biomass Using Dual Polarimetric PALSAR and ETM plus Data in the Hyrcanian Mountain Forest (Iran). Remote Sensing. 6. 3693-3715. 10.3390/rs6053693.
-
Bai, Jun-hoa., Li, Shao-kun., Wang, Ke-ru., Sui, Xue-yan., Chen, Bing., and Wang, Fang-yong. (2007). Estimating Aboveground Fresh Biomass of Different Cotton Canopy Types with Homogeneity Models Based on Hyper Spectrum Parameters. Agricultural Sciences in China. 6 (4), 437-445
-
Baret, F., and Guyot, G. (1991). Potentials and limits of vegetation indices for LAI and APAR assessment. Remote Sensing of Environment. 35. 161173.
-
Barik, S.K. 2006. Ecology of Diversity and Conservation of Plants and Ecosystems in India. Daya Publishing House, New Delhi, India. ISBN- 13: 978-8189233440
-
Boyd, D.S., Foody, G.M. and Curran, P.J. (1999). The relationship between the biomass of Cameroonian tropical forests and radiation reflected in middle infrard wavelengths. International Journal of Remote Sensing, 20(5), 1017-1023
-
Brown, S. 1997. Estimating Biomass and Biomass Change of Tropical Forests: A Primer. FAO Forestry Paper. 134.
-
Brown, S. and Iverson, L. (1992). Biomass estimates for tropical forest.
World Resource Review. 4. 366-383.
-
Brown, S., Gillespie, A. and Lugo, A. (1989). Biomass Estimation Methods for Tropical Forests with Applications to Forest Inventory Data. Forest Science. 35. 881-902.
-
Cairns, M.A., Olmsted, I., Granados, J. and Argaez, J. (2003). Composition and aboveground tree biomass of a dry semi-evergreen forest on Mexico's Yucatan Peninsula. Forest Ecology and Management, 186(1-3), 125-132
-
Chambers, J.Q., Santos, J.D., Ribeiro, R.J., Higuchi, N. (2001) Tree damage, allometric relationships, and above-ground net primary production in central Amazon forest. Forest Ecology and Management. 152, 7384.
-
Chave, J., Andalo, C., Brown, S., Cairns, M., Chambers, J.C., Eamus, D., Fölster, H., Fromard, F., Higuchi, N., Kira, T., Lescure, J., Nelson, B.W., Ogawa, H., Puig, H., Riéra, B. and Yamakura, T. (2005). Tree allometry and improved estimation of carbon stocks and balance in tropical forests. Oecologia. 145:87-99.
-
Das, S. and Singh, T. P. (2012). Correlation analysis between biomass and spectral vegetation indices of forest ecosystem. International Journal of Engineering Research & Technology. 1(5). 1-13. ISSN: 2278-0181
-
Foody, G.M., Boyd, D.S. and Cutler, M.E.J., (2003). Predictive relations of tropical forest biomass from Landsat TM data and their transferability between regions. Remote Sensing of Environment. 85(4), 463-474.
-
Forest Survey of India. 2017. India State of Forest Report 2017. Allied printers, Dehradun, India. pp 243-247
-
Frédéric, A., Eva, H., H.J., Stibig., Mayaux, P., Gallego P., Francisco, J., Richards, T., and J.P., Malingreau. (2002). Determination of deforestation rates of the worlds humid tropical forests. Science. 297.
-
Hall, R.J., Skakun, R.S., Arsenault, E.J. and Case, B.S. (2006). Modelling forest stand structure attributes using Landsat ETM+ data: Application to mapping of aboveground biomass and stand volume. Forest Ecology and Management. 225(1-3), 378-390.
-
He, C., Zhang, Q., Li, Y., Li, X., Shi, P., 2005. Zoning grassland protection area using remote sensing and cellular automata modelling a case study in Xilingol steppe grassland in northern China. Journal of Arid Environments. 63 (4), 814826.
-
Heiskanen, J. (2006). Estimating aboveground tree biomass and leaf area index in a mountain birch forest using ASTER satellite data. International Journal of Remote Sensing. 27. 10.1080/01431160500353858.
-
Hoffer, R. M. (1978). Biological and physical considerations in applying computer-aided analysis techniques to remote sensor data. Chapter 5 in Remote Sensing: The Quantitative Approach, McGraw-
Hill, New York
-
Hoffer, R. M., and Johannsen, C. J. (1969). Ecological potentials in spectral signature analysis. Chapter 1 in Remote Sensing in Ecology,
Johnson, University of Georgia Press, Athens
-
Huete, A. et al., (2002). Overview of the radiometric and biophysical performance of the MODIS vegetation indices. Remote Sensing of Environment. 83. 195-213.
-
Hurcom, S. J. and Harrison, A. R. (1998). The NDVI and spectral decomposition of semi arid vegetation abundance estimation.
International Journal of Remote Sensing. 19(16). 3109- 125
-
IPCC. (2007). Climate Change 2007: Impacts, Adaptation and Vulnerability. Contribution of Working Group II to the Fourth Assessment Report of the Intergovernmental Panel on Climate Change,
M.L. Parry, O.F. Canziani, J.P. Palutikof, P.J. van der Linden and C.E.
Hanson, Eds., Cambridge University Press, Cambridge, UK, 976pp
-
Jordan, C. F. (1969). Derivation of leaf area index from quality of light on the forest floor. Ecology. 50. 663 666
-
Kamusoko, C. and Aniya, M. (2009). Hybrid classification of Landsat data and GIS for land use/cover change analysis of the Bindura district, Zimbabwe. International Journal of Remote Sensing. 30. 97-115. 10.1080/01431160802244268.
-
Kankare, V., Vastaranta, M., Holopainen, M., Räty, M., Yu, X., Hyyppä, J., Hyyppä, H., Alho, P. and Viitala, R. (2013). Retrieval of Forest Aboveground Biomass and Stem Volume with Airborne Scanning LiDAR. Remote Sensing. 5. 2257-2274. 10.3390/rs5052257.
-
Kobayashi, S., Omura, Y., Sanga-Ngoie, K., Widyorini, R., Kawai, S., Supriadi, B. and Yamaguchi, Y. (2012) Characteristics of decomposition powers of L-band multi-polarimetric SAR in assessing tree growth of industrial plantation forest in the tropics. Remote Sensing. 4, 30583077
-
L. Coulibaly, P. Migolet, H. G. Adegbidi, R. Fournier and E. Hervet. (2008). Mapping Aboveground Forest Biomass from Ikonos Satellite Image and Multi-Source Geospatial Data using Neural Networks and a Kriging Interpolation. IGARSS 2008 – 2008 IEEE International Geoscience and Remote Sensing Symposium, Boston, MA, 2008, pp. III – 298-III – 301.
-
Labrecque. S., Fourier, R.A., Luther, J.E. and Piercey, D. 2006. A comparison of four methods to map biomass from Landsat-TM and inventory data in western Newfoundland. Forest Ecology and Management. 226: 129-144
-
Lillesaeter, O. (1982). Spectral reflectance of partly transmitting leaves: Laboratory measurements and mathematical modelling. Remote Sensing of Environment. 12. 247 254.
-
Lu, D. (2006). The Potential and Challenge of Remote SensingBased Biomass Estimation. International Journal of Remote Sensing. 27. 10.1080/01431160500486732.
-
Main-Knorn, M., Moisen, G. G., Healey, S. P., Keeton, W. S., Freeman, E. A. and Hostert, P. (2011). Evaluating the remote sensing and inventory-based estimation of biomass in the western Carpathians. Remote Sensing. 3: 1427-1446.
-
Maynard, C.L., Lawrence, R.L., Nielsen, G.A. and Decker, G. (2007). Modelling vegetation amount using bandwise regression and ecological site descriptions as an alternative to vegetation indices. GIScience & Remote Sensing. 44(1), 68-81.
-
Meghalaya Forests and Environment Department. (2019). Forests. http://www.megforest.gov.in/forest_reserved.html
-
Montagnini, F. and Porras, C. (1998). Evaluating the Role of Plantations as Carbon Sinks: An Example of an Integrative Approach from the Humid Tropics. Environmental management. 22. 459-70. 10.1007/s002679900119.
-
Ohmann, J. L., Gregory, M. J. (2002). Predictive mapping of forest composition and structure with direct gradient analysis and nearest neighbor imputation in coastal Oregon, U.S.A. Canadian Journal of Forest Research. 32: 725-741
-
Paruelo, J.M., Epstein, H.E., Lauenroth, W.K., and Burke, I.C., (1997). ANPP estimates from NDVI for the central grassland region of the United States. Ecology. 78. 953-958.
-
Patel, N., Saha, S., Dadhwal, V. (2007). Investigating the vegetation and agricultural responses to El Nino/Southern Oscillation using AVHRR data. Geocarto International. 22(4), 237-249. 10.1080/10106040701204289
-
Piao, S.L., Fang, J.Y., Zhou, L.M., Tan, K., and Tao, S. (2007). Changes in biomass carbon stocks in Chinas grasslands between 1982 and 1999. Global Biogeochemical Cycles, 21, 1-10
-
Qi, J., Chehbouni, A., Huete, A. R., Keer, Y. H., and Sorooshian, S. (1994). A modified soil vegetation adjusted index. Remote Sensing of Environment. 48, 119126.
-
Rahman, A. F., Gamon, J. A., Sims, D. A., and Schmidts, M. ( 2003). Optimum pixel size for hyperspectral studies of ecosystem function in southern California chaparral and grassland. Remote Sensing of Environment, 84, 192-207.
-
Rondeaux, G., Steven, M., & Baret, F., (1996). Optimization of soil- adjusted vegetation indces. Remote Sensing of Environment. 55, 95 107.
-
Roujean, J. L. and Breon, F. M., (1995). Estimating PAR absorbed by vegetation from bidirectional reflectance measurements. Remote Sensing of Environment. 51. 375 384.
-
Rouse, J.W., Haas, R.H., Schell, J.A., Deering, D.W., and Harlan, J.C., (1974). Monitoring the vernal advancement of retrogradation of natural vegetation. Greenbelt, MD, USA: NASA/GSFC, Type III Final Report, 1-371.
-
Schlerf, M., Atzberger, C. and Hill, J. (2005). Remote sensing of forest biophysical variables using HyMap imaging spectrometer data. Remote Sensing of Environment. 95. 177-194.
-
Somogyi, Z., Cienciala, E., Mäkipää, R., Muukkonen, P., Lehtonen, A., Weiss, P. (2007). Indirect methods of large-scale forest biomass estimation. European Journal of Forest Research. 126. 197-207. 10.1007/s10342-006-0125-7.
-
Steininger, M.K. 2000. Satellite estimation of tropical secondary forest aboveground biomass data from Brazil and Bolivia. International Journal of Remote Sensing, 21: 11391157.
-
Tenkabail, P.S., Smith, R.B. and Pauw, E.D. (2000). Hyperspectral vegetation indices and their relationships with agricultural crop characteristics. Remote Sensing of Environment, 75, 158-182.
-
Terakunpisut, J. (2007). Carbon sequestration potential in aboveground biomass of Thong Pha Phum forest ecosystem. Applied Ecology and Environmental Research. 5. 10.15666/aeer/0502_093102.
-
Verrelst, J., Schaepman, M., Koetz, B., Kneubühler, M., (2008). Angular sensitivity analysis of vegetation indices derived from CHRIS/PROBA data. Remote Sensing Environment. 112 (5), 2341 2353.
-
Wannasiri, W., Nagai, M., Kiyoshi, Honda., Santitamnont, P. and Miphokasap, P. (2013). Extraction of Mangrove Biophysical Parameters Using Airborne LiDAR. Remote Sensing. 5. 1787-1808. 10.3390/rs5041787.
-
Whittaker, R. H., Likens, G. E. (1973). Primary production: The biosphere and man. Human Ecology. 1. 357369. https://doi.org/10.1007/BF01536732
-
Zianis, D. and Mencuccini, M. (2004). On simplifying allometric analyses of forest biomass. Forest Ecology and Management. 187. 311- 332. 10.1016/j.foreco.2003.07.007.
-
Zianis, D., Muukkonen, P., Mäkipää, R., Mencuccini, M. (2005). Biomass and stem volume equations for tree species in Europe. Silva Fenn Monograph 4:263