
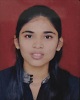
- Open Access
- Authors : Hetal Vora , Mamta Bhamare , Dr. K. Ashok Kumar
- Paper ID : IJERTV9IS050203
- Volume & Issue : Volume 09, Issue 05 (May 2020)
- Published (First Online): 16-05-2020
- ISSN (Online) : 2278-0181
- Publisher Name : IJERT
- License:
This work is licensed under a Creative Commons Attribution 4.0 International License
Personality Prediction from Social Media Text: An Overview
Hetal Vora
School of Computer Engineering & Technology MIT World Peace University Pune, India
Mamta Bhamare
School of Computer Engineering & Technology MIT World Peace University Pune, India
Dr. K. Ashok Kumar
School of Computing Sathyabama University Chennai, India
AbstractTodays world is witnessing great increase in the use of Social Media. People use them as a platform to share their feelings, emotions and experiences along with lot of personal information. All such information could be used in advantageous ways to help increase the Business and understand the user need. Personality prediction has gained lot of focus nowadays. It studies behavior of users and reflects the thinking, feelings etc. Traditional ways to take survey that was time consuming, for large number of users there is need of automatic prediction. Users are dynamic and can have their account on multiple platforms as they can have multi-context information. This survey gives an overview of different strategies used to predict the personality and behavior using the content available on social sites. Ability to predict users personality traits can help to build many customized services or products. Finally, the last section gives the Future trends and directions.
KeywordsPersonality prediction; Social Media
-
INTRODUCTION
Personality is defined as the set of different Characteristics such as behavior or emotions as a result of environmental or biological factors. It reflects the persons differences in persons thinking, behavior and feelings. Personality traits are continuous in nature as they reflect high and low of specific traits in a person on continuous trait rather than showcasing distinct personality. The term personality originally came from the Latin word persona that means mask. There are three criteria that are used to characterize personality traits: consistency along the situations, stability on basis of time and Individual differences that means Different Individuals have different behaviors. The field of study that studies the human personality and its variations among individual and group of people is called Personality Psychology.
With advent of technology the use of Social Networking sites has increased. People use as a platform to express and share their feelings, expectations, experiences etc. Along with this user often share their personal information such as profession, likes and dislikes etc. This information can be extracted [4]. This extracted data can give businesses opportunity to connect with their customers, understand their needs and thus improve the quality of service or product accordingly. It is used to find the patterns in connectivity, how they are
connected and similarity among them. Sentiment analysis is also done on social media data to understand the emotions positive and negative both on some topic by users. Researchers have also found and predicted the health problems like mental or Stress level based on their posts.
-
PERSONALITY COMPUTING BASICS
The two important basics in Personality Computing that is used to study personality from the social media data like Text, Multimedia are Theories that are used to explain, predict and understand the personality and the other is the Technique used for Obtaining the results.
-
Personality Theories
Personality Theories fall into main 4 categories [1] as Psychoanalytic Theory (also referred as psychodynamic), Trait theory, Humanistic theory and Social cognition theory. In the research purpose the trait theory is widely used [2].
-
Psychodynamic Theory: – According to Freud, the per- sodality is made up of three components as id, ego and superego. id refers to the impulse energy that is respon- sible for the human needs like nourishment, appreciation and urges like hate, urges etc. Superego or conscience, symbolize morality and Social norms, represent what a person wants to be. Ego the third component works on the principle of reality that mediates between the demands of the first component id and the second component superego and then chooses the most realistic solution for the long term.
-
Trait Theory: – Trait Theory suggests that human person- ality is composed of characteristics, or traits, that cause a person to act in a particular way. All these traits represent the blueprint for how person behaves examples introver- sion, sociability, aggressiveness, loyalty and ambition etc. There are various theories like Big Five, MBTI, Cattells 16PF Trait Theory etc.
-
Humanistic Theory: – Maslow believed that personality is based on personal choice not on nature or nurture. He suggested that people possess and are motivated to help them pursue their needs or desire that was represented in and the final level: self-actualization that is developing and growing to reach true potential
-
Social Cognition Theory: – The social cognition theory view personality in form of social interactions. Persons behavior is affected by the environment in which he stays.
The Trait theory is most widely used in studying the personality in field of Psychology. Unlike other theories this is based on finding the differences between the personalities of individuals. The combination of various traits forms a personality that is always unique for every individual.
-
Big Five: Todays Researchers believe that there are 5 personality traits. Big Five suggests that the traits can be categorized on 5 different classes. Exact labels for these 5 traits are still difficult to agree for some of them. Popular acronym is OCEAN for traits.
-
Openness: It reflects the intellectual level of a person. How curious, creative novel a person is. It also reflects how imaginative or independent a person is. Openness is related to peoples eagerness to try to new things, ability to be vulnerable, and capability to think outside the box. Common traits related to openness are: Imagination, dif- ferent interests, Originality, Daring, Cleverness, Intellect, Creativity, Curiosity etc.
-
Conscientiousness: It refers to the aptness of being steady, self-disciplined, responsible, focusing on achieving goals, and prioritizes plans instead of spontaneous behavior. It contrasts careless behavior. It denotes how careful, cautious, honest a person is. It is way to control impulses and act in a way that is acceptable socially by everyone around. This people are great at planning and organizing effectively. This include factors as planning, responsible, hard-work, determination, ambitious, control etc. They are good in leadership qualities.
-
Extroversion: People with high Extroversion quality have high confidence, Positive energy, and positive emotions, sociable and urge to interact more with other people. They are talkative in nature. It contradicts reserved behavior. Factors related to this trait are energy, talkativeness, fun loving, friendly, helping etc. These people feel good about themselves as well as about the world around them. People with low extroversion are reserved, quiet.
-
Agreeableness: This is tendency to be cooperative with others instead of being suspicious. They are friendly and liked by their colleagues as well as people surrounding them. They dont like to fight or argue rather than they are peace makers. Humble, politeness, helpful, patient, kind, sensitive etc are the traits that come under umbrella of agreeableness.
-
Neuroticism: It is contradict confident or secure nature. people withhigh neuroticism sensitive or nervous. This trait characterized by sadness, moodiness, and emotional instability. They experience negative emotions and feel- ings easily, like anger, anxiety, depression, negativity etc. It refers to the tendency to experience negative emotional states and see oneself and the world around negatively. Factors like temperamental,
anxious etc are some related traits.
With advent in the technology there had been wide increase in use of Social media like Facebook, Twitter, and Instagram etc. The information shared by users can be used to understand their personality and helps to understand needs and thus suggest services and facilities or predict their behavior for some situation.
-
-
-
-
Techniques Used
There have been many approaches used for personality prediction as shown below.
Fig. 1. Approaches Used
-
Questionnaire: The earliest form of approach used for personality prediction was in form of questions. Users were asked some questions that had multiple choices, from which user had to select one. These Questions were different for different personality traits. Based on the user selection of option, it was rated on some scale. Thus, help to predict the final score for each trait by adding the total scores related to that question.
-
Semantic Similarity: In this for the traits there are pre- defined vocabulary or dictionary words. The users words present in the posts are checked for the semantic similar- ity, i.e. similar meanings have same score. The distance is found out and thus the trait was predicted.
-
Machine Learning: Classical approaches cannot handle vast amount of data. This is one of the advantages of Machine learning algorithms. ML can also find the patterns from the data that might not be the visible by the humans.
-
Deep Learning: Deep learning [3] can be used to predict the personality traits with more accuracy. It processes the same way as human brains do. The feature extraction process is and there is no overload [5].
-
-
LITERATURE REVIEW
Maite et. al. [7] focused on Personality prediction from the Author Profiling task. They used PAN-AP-2015 corpus that was collected for social media users from twitter. Four languages were included but this paper focused on English language only. Self- online test was taken, and score was given between -0.5 to 0.5. Big Five model was used for traits. Then Glove representation in vector form was used for word embedding. For short
input data, the padding of many zero was done to as CNN require fixed amount of input. Different filters were used for Convolution layers. All the outputs were merged together, and the pooling layer was applied. ReLU is used as activation function. Fully connected neural network gives output as 5 neurons one for each stage. Deeper CNN can be implemented.
The authors in this paper [8] aim to predict the personality of twitter users for Arabic users in Egypt. They collected the data using AraPersonality. This data set was collected from Arabic dialect twitter user. Questionnaire consisting of several MCQs having 5 choices were translated to Arabic language and then filled by the users. And scores were assigned to each choice chosen by the user on the basis whether the question is Proportional or inversely proportional to the Big Five Personality Traits abbreviated as OCEAN. Apart from questionnaire their feeds were also collected. These Collected users feed then were pre-processed and cleaned by removing noisy data like user names, emails etc. and some non-Arabic words were converted to Arabic. Normalization was done to keep all the words in one form. The data is then divided into Train and test data. TF-IDF was calculated for every user. Three Supervised Machine learning as algorithms namely De- cision trees, Support vector Machine and Multinomial Naïve Bayes was used.
M. Hassanein et. al. [9] presented an approach to predict the personality on basis of semantics. They used big five model on MyPersonality Data-set. Vector Space model is used to represent the user text in the vector from that hold counts of every word in the text. Similarity measure is used to measure semantics using WordNet Database.
The Authors of the paper [10] proposed the model for text analysis and predict the personality of brands on Social Media Platform. Big Five model was used to predict the brand personality. This information could help brand to plan its Marketing Strategies as well as Improve relations with the Customers. MyPersonality data-set was used as well as the one was created for Brands pages and features were extracted from both these data-sets. Feature selection was done by done approaches namely Pearson Correlation and other was Gradient Boosting on 3 different Machine learning approaches as Support Vector Regression (SVR), Gradient Boosting and Feed- Forward Neural Network. XGB models perform best and predict personality.
Xiangguo et. al. [11] proposed a new model named as
2 CLSTM that is a bidirectional Long Short-Term Memory network interconnected with CNN to find personality of users.
It focused on structure of text as it can be important feature. Big Five model with 5 traits was used. Two data- sets were used for the experiment. One is long text data- set of essay data-set of 2467 essays tagged with their authors traits and another is Short text of YouTube vloggers. GloVe algorithm was used for word embedding. LSTM is used which has a self-loop and RNN loop as well, it is bidirectional so as to extract more features.
Paper also proposes the concept of Latent sentence Groups (LSG) that means several sentences that are closely related to each other. CNN was used for studying such latent features. Max pooling layer is used after LSTM to get sentence vectors. Softmax classifier was used as the classifier. Various contrast models were used like TF-IDF bayes, 2 and 3 dimensions CNN, one LSTM to compare the results with proposed model, which proved to perform better.
The authors of this paper [12] presented a system that could analyze the personality traits for Facebook users by using their status posts. Big Five personality model was used. They used MyPersonality data-set that had 250 users and about 10,000 posts updates from these users. These posts after extraction were pre-processed by removing links, symbols etc. All the words were converted in their lowercase. A spelling correction algorithm was used for real time data to correct all the incorrect spellings in the post. Posts also consisted of symbols like Hashtags (#) and emotions, these were removed by keeping the words as it is. TF-IDF was calculated to extract keywords from documents, thus feature vector was formed. This vector was too large so to reduce the size and to get only relevant features, Principal Component Analysis was used.
Machine learning algorithms KNN and SVM were used. KNN was best for Classification of traits.
-
Jia [13] focused on mental health problems like Stress and depression. For Stress detection, features were extracted at different granularity to describe each user as Tweet-level and user level. Author also created a benchmark data-set for the multi modal detection. For tweet level linguistic, visual, social features and for User level users posting behavior and Social features like Influence were extracted. 1-dimension CNN was applied with Cross Auto-Encoder units. Also analyzed the user content and posting style. Also, according to the paper there exists a correlation between the mental health of user and some social concepts like structure, influence, engagement etc.
According to Di Xue et. al. [14], language is common and better way to express their thoughts and feelings for others to understand, thus text can reflect the personality traits. They proposed a 2-level hierarchical deep learning architecture called AttRCNN, inspired by RCNN for sentence vectorization to extract thesemantic vectors. Big Five model was used on MyPersonality project that had 11 million Facebook users. SVR, Gradient Boosting and Random forest classifier were used.
J.Yu et. al. [15] Automatic prediction of personality from user social activities helps to predict his environment as well as has some important applications. Author used deep learning approach to predict the personality based on Big 5 model. The data-set was subset of 250 user released by Shared task. Pre- processing techniques were applied on it Skip-ngram method was used for word embedding. CNN with average pooling, RNN and FC neural network were used, and results were compared to Machine learning algorithms.
Skowron et. al. [16] used the traces left by users on Digital platforms like social media. Users with good reputation in US were selected and asked to fill the Questionnaire and then answers were used to score and for same users Instagram and Twitter posts were collected and pre-processed. In this paper, multimodal personality traits regression that were users information from two SNSs and evaluate them on the basis of trained data acquired from a one Social Network Sites.
Tadesse et. al. [17] uses Big 5 model to predict the personality of users based on Mypersonality data-set. Text features were extracted from posts that reflect language us- age and has expression and topic count using LIWC and SPLICE dictionaries. Second Social interaction features like connectivity, network size etc. Pearsons correlation is used to measure strength of relationship between variable and to get important features. XGBoost is used as classifier along with 3 baseline algorithms as Logistic regression, Gradient Boosting and SVM. XGBoost gave best results in predicting the personality traits.
The paper used a new Machine learning algorithm called Label Distribution learning [18]. The data collected was from Sina Weibo a microblogging site from Updates, status etc of the user and a test of 44 questions called as BFI was conducted to obtain their personality scores. The feature extraction was done in 3 categories that included static features that had little changes over time like gender, name etc, Dynamic features that changed over time like followers etc and last is Content features like blogs, linguistic, psychological features etc. Every Instance is given a label called as real valued. 8 LDL algorithms were used such as Knn, Bayes, and SVM etc. these were compared with some Baseline algorithms like M5 Rules, Random Forest and Tree, ZeroR,Gaussian, Linear Regression, Support Vector Regression, and MLP. Label distribution with Support vector Machine gave highest accuracy.
T. Yo et. al. [19] predicted personal attributes like Age, Gender, Occupation based on the Users Twitter data collected using API for 120 users. Mecab tool was used to collect the words from the post. Skip n gram method of Word2Vec tool was used to create the word embedding. Various ML algorithms along with deep learning algorithms were used like Linear SVC, Random Forests, KNN and AdaBoost. Full connection Neural network was used with varying parameters to get optimized results. Various attributes showed varies result for the Algorithms. Prediction of gender and occupation were more accurate using Linear SVC and deep learning. Whereas for age groups prediction using Random Forest And AdaBoost were more accurate.
The authors Wald et. al. [22] used Data mining techniques to predict the personality based on Social Networking site Facebook. Goal of the paper was to find out the topmost and bottom among the users exhibiting the traits. They used data of previously performed experiment called as Big 5 Experiment done conducted by Online Privacy Foundation. The aim of the experiment was to conduct a survey of 537 users of Facebook who
gave some answers to the Questions that categorize according to Big Five model. In addition to this, their information was also collected that would uniquely define every user with 32 attributes like sex, age, comments etc and 80 text attributes. LIWC was used to find positive and negative emotions from the text. Numerical algorithms such as Linear regression, REPTree, Decision Tables were used to predict top and bottom in the list. REPtree models along with all traits were accurate to predict users. Further, the automatic data mining techniques can be used for prediction thereby reducing the number of attributes used.
The authors [24] used Semi-Supervised method to use the large amount of unlabeled data in order to improve the prediction accuracy. Pseudo Multi-view Co-training algorithm was used. To extract the linguistic features the techniques such as LIWC and n-grams on Mypersonality data-set after pre- processing it. Words cloud were built to show the how the word is linked with particular personality trait using Wordle that displays the word with highest correlation.
The author of the paper [25] proposed a method to predict the personality of the Facebook users using their digital footprints. Big 5 model was used as the Trait model. There were 2 data-sets used, one was collected from 90,000 and plus Facebook users and other was the personality traits of all these users. The extraction of data had more than 600 features to get only the necessary features, the LASSO algorithm was used to extract only the main features. Model gave best accuracy for Openness and Extraversion, the lowest was for Agreeableness while Conscientiousness and Neuroticism had moderate accuracy.
The system [26] was developed that was a web application to predict the use personality based on the Twitter posts by the user. MyPersonality data-set was used with slight modifi- cations. Indonesian data-set was created by translating above data-set. And User text was taken from Twitter tweets and made a single document. Text data was to be represented in Vector form after pre- processing it like tokenization, removal of stop words, stemming etc. This Classification is Multi Class as person has combination of traits. A Binary classifier was built for every trait. Multinomial Nave Bayes model was used using multimodal distribution with occurrences of word or word weight as feature to classify. KNN with Cosine similarity for document Classification was used. SVM was also used. MNB gave best accuracy.
-
FUTURE TRENDS
One of the research areas that requires lot of attention is predicting the personality based on Social media data. A lot of work has been already done but still requires some work to be done to increase the accuracy of prediction. Thus, requires improvement in various aspects of system like Algorithms, Extraction of Features, and Data-set etc.
According to authors in [7] shallower and deeper CNN can be implemented that have not been implemented previously in Natural Language processing. More over
the predictability of model should be Checked on various data-sets.
Increasing the size of data-set and to improve the feature extraction that can improve the accuracy [8]. Using Multivari- ate regression instead of Single for all the traits at once. Also, it is necessary to check if persons and brand personality are similar, this could be used in advantageous ways to provide and recommend services as well as help plan marketing strategies [10]. Apart from the text other multi- media data can be used to such as photo, videos etc. [11]. Creating dictionary for the patois words used in the social media to predict personality [12].
To get proper mental health status, offline personalized measurement must be done for users so that proper care can be provided to them [13]. Regression algorithm designed to predict the personality can be used to increase the accuracy by giving input as semantic features [14]. As the amount of data increases it becomes impossible or difficult to label the data, thus Unsupervised learning [15] can be used to predict personality by using the external knowledge and thus cluster the text. Larger data set can help in improving accuracy and help recommend the users wit services, movies, music etc. [17]. Personality varies over time, so the data collected should include all the previous posts from past.
-
CONCLUSION
-
Behaviour on Social media sites of users can help in predicting the traits of User based on various personality models. Earlier questionnaire method was used that could be a Costly and time-consuming process. The goal of this paper is to give summary of the work done for Predicting the personality on text from Social media sites and to summarize the future trends. Table I Shows the Overview of the Current research techniques Performed analysis shows the Various techniques and models used. Working on the future directions, accuracy can be increased of prediction as well as can be used to provide some Customized services and other recommendations.
REFERENCES
-
Diener, E. and Lucas, R. (2019). Personality Traits. [online] Noba. Avail- able at: https://nobaproject.com/modules/personality-traits [Accessed 30 Sep. 2019].
-
Thompson, J. (2019). [online] Bizfluent.com. Available at: https://bizfluent.com/info-7745856-four-theories-personality.html [Accessed 30 Sep. 2019].
-
En.m.wikipedia.org. (2019). Deep learning. [online] Available at: https://en.m.wikipedia.org/wiki/Deep learning [Accessed 30 Sep. 2019].
-
En.wikipedia.org. (2019). Social media mining. [online] Available at: https://en.wikipedia.org/wiki/Social-media-mining [Accessed 30 Sep. 2019].
-
Bonner, A. (2019). The Complete Beginners Guide to Deep Learning. [online] Medium. Available at: https://towardsdatascience.com/intro-to- deep-learning- c025efd92535 [Accessed 30 Sep. 2019].
Using Convolutional Neural Networks, Springer Nature Switzerland AG pp- 313-323, 2018
[8]M. S. Salem, S. S. Ismail, and M. Aref, Personality Traits for Egyptian Twitter Users data-set, Proceedings of the 2019 8th International Conference on Software and Information Engineering– ICSIE 19, 2019.
[9]M. Hassanein, W. Hussein, S. Rady, and T. F. Gharib, Predicting Personality Traits from Social Media using Text Semantics, 2018 13th International Conference on Computer Engineering and Systems (ICCES), 2018.-
R. B. Tareaf, P. Berger, P. Hennig, and C. Meinel, Personality Ex- ploration System for Online Social Networks: Facebook Brands As a Use Case, 2018 IEEE/WIC/ACM International Conference on Web Intelligence (WI), 2018
-
X. Sun, B. Liu, J. Cao, J. Luo, and X. Shen, Who Am I? Personality Detection Based on Deep Learning for Texts, 2018 IEEE International Conference on Communications (ICC), 2018.
-
M. Vaidhya, B. Shrestha, B. Sainju, K. Khaniya, and A. Shakya, Personality Traits Analysis from Facebook Data, 21st International Computer Science and Engineering Conference (ICSEC), 2017.
-
J. Jia, Mental Health Computing via Harvesting Social Media Data, Proceedings of the Twenty-Seventh International Joint Conference on Artificial Intelligence, 2018.
-
D. Xue, L. Wu, Z. Hong, S. Guo, L. Gao, Z. Wu, X. Zhong, and J. Sun, Deep learning-based personality recognition from text posts of online social networks, Applied Intelligence, vol. 48, no. 11, pp. 42324246, May 2018.
TABLE I: OVERVIEW OF LITERATURE REVIEW
Sr. No |
Paper |
Dataset & Model |
Methodology |
1 |
Maite[6] |
Big five model , PAN AP 2015 |
Used CNN for text after pre-processing it and converting to the vector representation using Glove representation. For every trait gave one Neuron as output value using ReLu activation |
2 |
Salem[7] |
Big Five Ara Personality, Data collected of all users posts. |
Questionnaire was filled and Scores were assigned. Tf-idf for every user was calculated. Machine learning algorithms Decision trees, Support vector Machine and Multinomial Naïve Bayes was used. |
3 |
Hassanein[8] |
Big Five, My Personality |
Introduces a model in which the semantic similarity between the user posted text and the words that describes the personality trait is calculated. Vector Space model is used. |
4 |
Tareaf [9] |
Big Five MyPersonality and Created one for brand Pages. |
Extracted features using Pearsons correlation and Gradient Boosting. Used 3 approaches to predict the personality Gradient Boosting, Support Vector Regression and Neural Networks |
5 |
Sun [10] |
Big Five Essay data and YouTube vloggers |
Glove for Word embedding. Proposed LSTM method interconnected with CNN. CNN was used to study new concept Latent Sentence Groups i.e. Sentences that are Closely related to each other. Contrast model like Td-if, 2 CNN, 3 CNN were used to compare results with LSTM. |
6 |
Vaidhya [11] |
Big Five, My Personality |
Tf-idf was used to extract keywords. PCA was used to extract important features. KNN and SVM machine learning algorithms were used |
7 |
Jia [12] |
Collected data from Twitter, weibo |
Mental Health was found with problems of stress and depression. LIWC was used. DNN was used to calculate the personality traits scores. |
8 |
Xue [13] |
Big Five MyPersonality |
Proposed a2-level hierarchical deep learning architecture called AttRCNN, inspired by RCNN for sentence vectorization. SVR, Gradient Boosting and Random forest classifier were used. |
9 |
Yu [14] |
Big Five MyPersonality |
Applied deep learning to learn suitable data representation. Used Neural Networks like CNN, RNN and Fully Connected neural network. CNN with average pooling gave best prediction results. |
10 |
Skowron [15] |
Big Five, Crawled users data |
Extracted images, linguistic and mta features related to reputation and popularity. Images used features like in emotion detection. Linguistic features extracted using LIWC etc. |
11 |
Tadesse [16] |
Big Five model My Personality |
Text features extracted using LIWC and SPLICE along with Social Interaction Behavior features like Connectivity, network Size etc. XGBoost, Logistic Regression, Support Vector Machine and Gradient Boosting algorithm were used. |
12 |
Xue [17] |
Big Five Model User data collected from Sina Weibo, a Micro blogging site |
Uses ML technique Label distribution to give labels or real value vector to every instance. 8 LDL algorithms used along with baseline algorithms like SVM, Random forest etc. Result showed LD with SVM gave best results. |
13 |
Yo [18] |
Collected Twitter data of 120 users using Twitter API |
Predicted Age, Gender and Occupation from Collected data. Used AdaBoost, Linear SVC, Random Forest along with Fully Connected Neural Network. AdaBoost and random Forest best predicted Age whereas NN and Linear SVC best predicted Gender and Occupation |
14 |
Wald [22] |
Big Five Experiment done by Online privacy Foundation |
LIWC is used to predict positive and negative emotions from text. Numerical algorithms such as Linear regression, REPTree, Decision Tables were used to predict top and bottom in the list. REPtree models along with all traits were accurate to predict users. |
15 |
Zheng [24] |
Big Five Mypersonality |
LIWC and n-grams to extract linguistic features from the text. Used Semi-Supervised Co-learning algorithm, called as PMC. Also showed words with highest correlation to particular personality trait using Wordle to form Word Cloud. |