
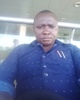
- Open Access
- Authors : Allois Kioko Luvai , John P. O. Obiero , Christian T. Omuto
- Paper ID : IJERTV9IS050395
- Volume & Issue : Volume 09, Issue 05 (May 2020)
- Published (First Online): 20-05-2020
- ISSN (Online) : 2278-0181
- Publisher Name : IJERT
- License:
This work is licensed under a Creative Commons Attribution 4.0 International License
Methods for Erosion Estimates in Assessment of Soil Degradation: A Review for Catchments in Kenya
Allois Kioko Luvai Department of Crop Sciences, Pwani University, Kilifi, Kenya
John. P. O. Obiero
Department of Environmental and Biosystems Engineering University of Nairobi, Nairobi, Kenya
Christian T. Omuto
Department of Environmental and Biosystems Engineering University of Nairobi, Nairobi, Kenya
Abstract Soil is a medium which supports a number of biological activities and for this reason its degradation must be minimized in order to enhance its productivity. In Kenya, much government attention has been directed towards salinization and water logging problems not forgetting that soil erosion risk is the most significant. Soil erosion poses a serious threat in tropical areas as the push for more agricultural land and grazing activities intensifies. Such transformations on the ecology have strongly influenced soil erosion rates. The problem of soil erosion in Kenya is attributed to the arid and semi-arid climate (about 83 percent of land mass), poor management of land and intensified agricultural activities. Several studies have demonstrated that the rate of soil erosion accelerates substantially in areas where natural vegetation has been removed. Catchments are heterogeneous in nature given the different biophysical factors at play. This paper summarizes the review findings of soil erosion rates and estimation methods in Kenya. The purpose of this review was to establish the potential threat to soil degradation due to erosion through comparison of erosion rates in selected areas to acceptable soil loss. It was established from the review that soil erosion rates in most rural catchments in Kenya are above the permissible annual rates of between 2.2 to 10 t ha-1 yr-1 obtained using various methods most of which integrate the use of GIS framework in erosion degradation assessment. The findings reveal that most rural catchments are susceptible to soil degradation by erosion. Findings from the review would be useful in establishing methodology for identifying hotspots in the planning of targeted intervention for conservation to minimize further degradation by soil erosion and sedimentation.
Keywords Soil erosion; Erosion modelling; catchment; prediction; RUSLE
-
INTRODUCTION
Soil is a medium which is extremely complicated in terms of structure in addition to being variable, (Gianinetto et al., 2019). Its components are mineral elements, organic matter content, water and air, (Chen & Shah, 2019). The medium is open to serious degradation threats such as soil erosion, reduction in organic matter content, contamination at local and diffuse scales, landslides among others, (Panagos et al., 2018). Formation of soil is considered as a non-renewable because the manner in which it takes place is extremely slow, (Rulli et al., 2013; Chen & Shah, 2019). Biggelaar et al. (2003)
estimated that the average rates of soil erosion on a global perspective ranged between 12 and 15 t ha-1 yr-1. FAO (2015) estimated that every year between 0.90 and 0.95 mm of soil is lost from the land surface.
Soil erosion is an important player in the degradation of soil, (Siddique et al., 2017). It affects the quality of soil and so as its productivity, (Panagos et al., 2018). Some other soil erosion effects include degradation of water quality and reduced capacity to prevent occurrence of some natural disasters for instance floods, (Mutua et al., 2006). Soil erosion is a naturally occurring process which affects different types of landforms, (Chen & Shah, 2019). It is a global problem which continues to threaten natural resources as well as agricultural production, (Pimentel, 2006). The main causal agents are water and wind. The consequences of soil erosion by water are so severe on soils, (Panagos & Katsoyiannis, 2019; Fenta et al., 2020). In vulnerable areas for instance, the production capacity of the soil is compromised and as such the land is subjected to desertification issues, (Kirkby et al., 2008). According to Oldeman et al. (1994) an estimated 11 million km2 of land has been degraded by water erosion.
The processes of soil erosion strongly relate with different zones of climate, (Fenta et al., 2020). It is more pronounced in tropical areas especially developing countries in Africa, (Onyando et al., 2005). Tropical areas are more vulnerable because intensive climatic regimes tend to circumstantially converge here, the soils are fragile, the use of fertilizers and conservation practices are quite limited, and most livelihoods strongly depend on the quality of soils, (Claessens et al., 2008). The rough environmental conditions and poor management of land in Africa have caused significant rates in soil erosion, (Haregeweyn et al., 2006). Climate change on the other hand is expected to worsen the current vulnerabilities facing the rural population who mainly derive their living from semi-subsistence, (Herrero et al., 2010). Erosion rates in tropical catchments have not been well known like in temperate ones because most of the erosion occurs during the rainy season, (Brown et al., 1996).
Agriculture is the backbone of Kenya's economy, (Onyando et al., 2005). However, increased agricultural production is only possible if the soils are good in terms of fertility, (Fleitmann et al., 2007). About 75 percent of the soils in Kenya are environmentally fragile, (Fwamba et al., 2017; Wekulo, 2017). This has been attributed to soil erosion which has severely degraded agricultural lands and has lowered the capacity of the country to produce in addition to increasing its vulnerability to some natural hazards, (Biamah et al., 1997; Mulinge et al. 2016). On the other hand, agriculture in Kenya and just like in many countries depends strongly on irrigation water most of which is stored in reservoirs. There is need for managers of land as well as policy makers to find effective and more efficient solutions targeted towards reducing sedimentation in reservoirs, (Hunink et al., 2013). Nearly 20 percent of the Kenyan land was reported to have suffered permanent reduction in terms of soil productivity due to water erosion, (Dregne, 1990). De Graff (1993) estimated that 72 t ha yr-1 had been lost due to soil erosion.
Human induced loss of soil has serious consequences in the economy and environment all over the world, (De Graffenried & Shepherd, 2009). The current trend in land cover/land use is enough evidence that Kenya faces immense anthropogenic pressure, (Kieti et al., 2016). This is common within catchments in which massive encroachment by rural communities has been accelerated by the urge to create more land for settlement and for agricultural activities, (Mutua & Klik, 2004). Population pressure within the Kenyan highlands has led to cultivation within the steep slopes while on the other hand intensive grazing has been practiced in semi-arid lands hence soil erosion has been enhanced, (Mutua & Klik, 2004). Future pressure towards expansion of agricultural as well as grazing activities will undoubtedly accentuate the current high rates of soil loss, (Mutua and Klik, 2004). These changes in catchments may affect soil erosion processes and as such there is need to assess soil loss given the present and possible future use and management of land, (Hunink et al., 2013).
Successful rate at which measures for control of soil erosion are implemented require information for optimal intervention for guiding dedication of resources and efforts too. In this regard, it is important to monitor the status of soil erosion and carry out field urveys as ways of targeting interventions, (Hunink et al., 2013). The risk of soil erosion expresses the integral susceptibility of uncovered soil to erosion, (Renard et al., 1997). This helps in giving information on the worst case scenario that would probably occur, (Fwamba et al., 2017). Hence, the process of assessing soil erosion must focus on identification of erosion factors. In addition, the process must use parameters whose data is available for the particular area of interest and it should be capable of being adjusted in an easy manner in the event that more or better information becomes available.
Effective solution to the soil erosion problem may be through strategic planning on the long-term on the basis of sustainable management of the catchment, (Fenta et al., 2020). To this end, the risk of soil erosion has been predicted at various scales which range from individual field plots to drainage basins using models, (Fwabwa et al., 2017).
However, assessment of soil erosion in large catchments and further testing of the impacts of the various soil erosion strategies, the use of distributed soil erosion modelling is inevitable because it gives a complete picture of the whole process, (Hunink et al., 2013). Additionally, there is need to develop an understanding of the spatial processes involved in soil erosion phenomenon as this will go a long way in identifying the critical areas which are highly susceptibility to water erosion and hence guide the planning stage for effective conservation, (Angima et al., 2003; Onyando et al., 2005; Fenta et al., 2020). The objective of this study was to review the extent of soil erosion in Kenya and the methodologies applied.
-
HISTORY OF SOIL EROSION IN KENYA
Just like other developing countries, problems of soil erosion have been on the rise in Kenya, (Onyando et al., 2005). It is caused by runoff when it traverses bare land surfaces. It is attributed to increased population, unregulated uses of land, severe droughts and deforestation, (Fleitmann et al., 2007). The erosion problem is more severe in marginal lands where intensive agricultural activities are practiced. In 1935, soil erosion was identified as a serious environmental problem in Kenya with the dangers increasing tremendously in the 1960s following which the approach on conservation of soil changed from enforcement to advisory, (Ongwenyi et al., 1993). In the following decade, attention focused on management of land and adoption of conservation practices whose goal was to reduce the erosion problem.
-
METHODOLOGY FOR ESTIMATING SOIL EROSION RATES IN KENYA
Njiru et al. (2018) modeled soil loss for better management of land in Galole ungauged catchment in Northern Kenya. The study used the RUSLE model which constituted the main causal agents of soil erosion adopted in a GIS environment. The study was able to produce a soil erosion map of the catchment following it was established that the loss of soil was heterogeneous and the risk was variable. The study proposed immediate measures to decelerate the high soil erosion rates to manageable levels by adopting proper mitigation measures.
Wekulo (2017) determined the actual risk of soil erosion in Isiukhu river catchment by taking into account the trends in the use of land as well as its cover. The study established that soil erosion was dominant in the river catchment because of the prevailing environmental and anthropogenic pressures. The study measured the actual soil erosion risk by adopting the RUSLE model implemented in a GIS framework. The potential risk of soil erosion was determined by considering the environmental factors particularly the rainfall erosivity, erodibility of soil and the length of slope and its steepness. The land use/cover factor was computed for the period 1990 to 2015 while the control factor for soil erosion was computed too.
Moses (2017) modelled soil erosion risk in addition to estimating sediment yield within Nzoia River basin in Kenya
using a GIS-RUSLE interphase. For this particular study, the data base comprised of rainfall data, soil data, a 90 m DEM and Landsat imagery. The simulated factors of the RUSLE model were multiplied interactively in ArcGIS after which a soil erosion risk map for the river basin was generated. The spatial variation of soil erosion within the river basin was identified through the use of modelling. The study pointed out that the mean annual rate of soil loss is strongly influenced by the interplay between the RUSLE factors (equation 1).
Fwabwa et al. (2017) estimated the risk of soil erosion spatially within Isiukhu river catchment in the western region of Kenya by employing RUSLE model in ArcGIS 10.3. Sugarcane and maize production are the key economic activities in the region. For this particular study, RUSLE model adopted in a GIS framework was able to estimate the distribution of the potential risk of soil erosion in a spatial manner following which the environmental factors of the model were keenly studied. The study further demonstrated that for that particular catchment, the spatial extent of the potential risk of soil erosion varied with rainfall erosivity, erodibility of soil, length and steepness of the slope. It was found that the texture of the soil, land use/cover and so as the slope are the greatest determinants of loss of soil through erosion. The study reported that intensive agricultural activities to lead intolerable limits of soil loss.
Mati et al. (2006) tested the applicability of the European Soil Erosion Model (EUROSEM) in two Kenyan catchments: Embori and Mukogodo. Embori represented an intensive cultivated land while Mukogodo was a dry rangeland which served as communal grazing. Interrill erosion resulting from single storm events was simulated by EUROSEM on plots measuring 10m by 2m. The results showed that the model was applicable to both situations. For dry rangeland scenario, the model proved inadequate in predicting the loss of soil particularly in vegetated plots which have grass or shrubs. However, runoff from bare plots and grassed ones was adequately predicted by the model. The study pointed out that the model required some improvements in order to make it more effective in predicting runoff and loss of soil in Kenyan semi-arid areas.
Mutua et al. (2006) developed a simple approach for predicting soil loss together with sediment yield from an extensive rural catchment, Masinga dam catchment whose area is 6262 km2. The study applied the RUSLE model and developed hill-slope sediment delivery distributed (HSDD) model which were both embedded in GIS. The HSDD model was used to estimate the sediment delivery ratio (SDR) by utilizing the travel time concept for sediments as a function of catchment characteristics. The performance of the model was verified by comparing the predicted results with field data. The results showed that the approach provided a better way of estimating the spatial extent of soil erosion and sediment yield at catchment level.
Onyando et al. (2005) estimated the potential of soil erosion for Perkerra river catchment, Kenya. The river has a catchment area of 1207 km2 and drains into Lake Baringo which has a drainage area of 6820 km2. It is reported that the
depth of the lake reduced from the initial 8m in the year 1972 to about 2.5m in 2003. High rates of erosion within the catchment area were behind the siltation of the lake. The catchment is characterized by slopes which quite steep especially on the hillsides but it becomes gentle in the middle as well lower reaches. The ecosystem is considered fragile and as such interventions for controlling soil erosion have been challenged by unavailable data on loss of soil and its spatial distribution. The study applied the USLE model implemented in GIS Arc/Info and the Integrated Land and Water Information Systems (ILWIS) to estimate the potential soil erosion within River Perkerra catchment. Some physical parameters of the model equation were derived from spatial data from Landsat TM satellite imageryfor the catchment.
Mutua and Klik (2004) carried out a study to interrogate the management of soil erosion within a diverse catchment, a case of Masinga catchment in Eastern Kenya. The study estimated soil erosion within the catchment by adopting RUSLE model implemented in a GIS framework. It is regarded as the present state of the art in soil erosion modeling. GIS environment quantifies parameters from the available sets of data. The methodology was reported as quite easy, fast and even cost effective in estimating the spatial distribution of soil erosion as well as sediment delivery. RUSLE and GIS therefore serve as useful and effective tools through which long-term potential of soil erosion may be predicted.
Angima et al. (2003) conducted a study to predict the annual loss of soil in Kianjuki catchment, central Kenya. The study used the RUSLE model after which the erosion hazard was determined and target areas for initiation of appropriate conservation measures identified. All the RUSLE factors were calculated for the entire catchment by use of locally available data. The study relied on field data for three years which when incorporated in the RUSLE model specific sites which showed erosion hazards were pinpointed and different mitigation measures proposed. The study pointed out that erosion was so severe in highlands but long-term data was required to validate the RUSLE model so that it suits local conditions.
Mati et al. (2000) assessed the erosion hazard within Upper Ewaso Ng'iro North basin, Kenya using USLE and GIS. The methodology showed that adoption of USLE in a GIS environment can be used to determine loss of soil at field level in a quantitative and spatial manner and even predict the erosion risk over extensive watersheds. Thematic data layers for the study area such as rainfall erosivity, soil erodibility, steepness of slope, land cover and support practices in addition to the erosion risk map for the basin were obtained. The study was able to pin point the main factors which contribute to loss of soil within the watershed.
-
SPATIAL DISTRIBUTION OF SOIL EROSION IN KENYA
Soil erosion is a serious problem in many Kenyan rural catchments (table 1). It is evident from the results that erosion rates in most catchments studied reveal potential threat of degradation by erosion. It was further noted that soil erosion
rates in Kenya vary from region to region, (Ongwenyi et al., 1993). From a national perspective, high erosion rates are experienced in the most productive parts of the country.
These include the Kenyan highlands which comprise the central region, some parts of the eastern region and western and these
TABLE 1: SOIL EROSION DATA FROM SELECTED CATCHMENT AREAS IN KENYA
Region
Catchment
Methodology
Estimated Soil Erosion
Reference
Central
Kianjuki
RUSLE& GIS
134 t ha-1yr-1 – 549 t ha-1yr-1
Angimaet al. 2003
Central
Upper EwasoNgiro North basin
USLE & GIS
1 – 25 t ha-1 yr-1
Matiet al. 2000
Central
Embori
EUROSEM
35 t/ha
Matiet al. (2006)
Mukogodo
EUROSEM
102 t/ha
Matiet al. (2006)
Western
River Nzoia
RUSLE & GIS
0.51 – 8.84 Mt ha-1 yr-1
Moses (2017)
Western
Isiukhu
RUSLE & GIS
63 t ha-1 yr-1
Wekulo (2017)
Western
Isiukhu
RUSLE & GIS
0 128 t ha-1 yr-1
Fwabwaet al. (2017)
Eastern
Golole
RUSLE & GIS
272 t/ha
Njiruet al., (2018)
Rift Valley
River Perkerra
USLE & GIS
1.73 m tonnes/year
Onyandoet al. (2005)
Central/Eastern
Masinga
RUSLE, HSDD & GIS
57.2 t ha-1 yr-1
Mutuaet al. (2006)
Central, western & eastern
Sagana, Sirimon, NzoiamTana, Kambure, EwasoNgiro
Suspended sediment samples and discharge records
8 3,117 t km-2 yr-1
Ongwenyiet al. (1993)
are areas which have intense agricultural activities. It was also established that soil erosion rates were also higher in marginal lands where grazing was practiced for instance some parts of central, eastern and rift valley regions. On the other hand, soil erosion rates were low in undisturbed forested areas. However, soil erosion rates in areas under agricultural activities were found to be much higher than overgrazed lands in ASALs.
Angima et al. (2003) reported that the estimated permissible annual rate of soil loss in most rural catchments in Kenya is between 2.2 to 10 t ha-1 year-1. Table 1 indicates that all the catchments are experiencing soil erosion rates which are above the tolerable rates. The high rate of soil loss from catchments is an indication of sediment yield and siltation of water bodies such as reservoirs and dams, (Ongwenyi et al., 1993). Sediment finds it way in watercourses where river flows are low and physical conditions are favourable. This implies that the risk of siltation on river catchments where dams have been constructed is on the rise. Hence, sedimentation of great concern to matters of development of water resources.
Kenya is basically an agricultural country in which three quarters of her people are engaged in agricultural activities. And just like many African countries, soil erosion in the country has substantially increased due to the harsh conditions of the environment and management practices which render the land poor, (Haregeweyn et al., 2006). Significant changes in agricultural activities have occurred in the last century. However, there is limited amount of arable land in Kenya with about 30 % of the country's area experiencing high to very high levels of soil degradation which is human induced. Depletion of vegetation covers due to grazing and increased agricultural activities have subjected catchments to severe soil degradation by soil erosion. It is therefore important to check the movement of sediments especially from croplands and grazing fields.
-
SOIL EROSION MODELING
Modeling as applied in estimation of soil erosion provides an option and an advanced tool through which appropriate practices for conserving soil especially at catchment or even regional levels,Mutua et al., 2006; Moses, 2017). Various types of soil erosion models which range from simple empirical models for instance USLE (Wischmeier & Smith, 1978) and RUSLE (Renard et al., 1997) to complex process- based models for instance European Soil Erosion Model, EUROSEM (Morgan et al., 1998) have been applied to assess the rates of soil loss by water erosion in Kenya catchments. Models for predicting the spatial distribution of soil erosion at catchment scale have been promoted by the recent advancements in the use of GIS, RS and DEM, (Efthimiou et al., 2014; Saha et al., 2018).
Catchment areas show some distribution over a broad range of environmental conditions in terms of topography, geological formations, land use and land cover, (Ongwenyi et al., 1993). Thus the determination of soil erosion at catchment level is challenging because of the heterogeneity of the catchment since it is characterized by different biophysical factors, (Onyando et al., 2005). Further, catchments experience a variation in hydrological regimes which occur at different spatial and temporal scales hene complicating the dynamics of soil erosion, (Onyando et al., 2005). The distributed approach considers the spatial heterogeneity of the catchment with regards to land use, topography, soil properties taking into account their spatial variation and interaction, (Mutua et al., 2006; Moses, 2017).
The complexity of the soil erosion variables in addition to their interaction in spatial domain calls for a simple ways of managing data, (Mati et al., 2000). Petersen et al. (1998) pointed out that the use of GIS framework and soil erosion models serves as one of the ways of overcoming the above challenge. Models for predicting soil erosion help to predict a long range of land management planning by taking into account both natural agricultural situations, (Angima et al.,
2003). However, it is important to note that not even a single model can consider the forms of soil erosion in totality. Nonetheless, some particular models were developed to aid in identification of areas which would have the greatest impact on reduction of soil upon introduction of some given conservation measures of soil, (Angima et al., 2003).
The behavior of a catchment is modeled by first simplifying or generalizing it to a level where real-world situations are reduced to model capabilities, (Mutua et al., 2006). In this regard, a raster GIS format is used to adequately represent the changes associated with the environmental landscape factors in addition to giving the scale at which the said changes occur, (Mutua et al., 2006). The RUSLE model is able to lump the spatio-temporal factors to give an average figure across a variable landscape (Renard et al., 1997). The RUSLE factors depend on the geography of the area and as such they are made as separate layers after which they are processed within a raster GIS. Hence, the spatial and technological challenges of the RUSLE model have been overcome by the use of the GIS framework, (Fenta et al., 2020).
The Universal Soil Loss Equation (USLE) and the Revised Universal Soil Loss Equation (RUSLE) are the two most widespread soil erosion models which have been applied at catchment level. The USLE is an empirical model which was developed by Wischmeier & Smith (1978) and it was calibrated for use in the USA and other areas, (Claessens et al. 2008). The Revised Universal Soil Loss Equation (RUSLE), Renard et al., (1997) model was developed for prediction of soil erosion due to water in temperate conditions but it was found to adapt easily in the tropics than any one of the existing models, (Angima et al., 2003).
RUSLE is an empirical model (equation 1) which was founded on the USLE and it is advantageous in the sense that it is quite diverse and it integrates more databases, (Renard et al., 1997). The model predicts the rate of soil erosion of mean annual basis by involving the cropping systems, management practices and practices of erosion control, (Angima et al., 2003). Additionally, soil erosion rates in ungauged catchments may be predicted using the model so long as the characteristics of the catchment and so as its hydro-climatic conditions area known, (Garde & Kathyari, 1990). The RUSLE factors are analyzed spatially after which the raster layers are superimposed to compute the mean annual loss of soil, (Renard et al., 1997; Kidane et al., 2019).
A = R * K * L * S * C * P (1) Where; A = soil loss (t/ha/yr), R = rainfall erosivity factor [MJ (mm/ha/year)], K = soil erodibility factor [t h/(MJ mm)], L = slope length factor (dimensionless), S = slope steepness factor (dimensionless), C = soil cover and management factor (dimensionless), and P = soil and water conservation practices factor (dimensionless).
-
SUMMARY OF MAIN FINDINGS
Prediction of Soil erosion in Kenya
The review showed that spatial loss of soil from different catchments in Kenya was predicted using RUSLE model and geographic information system, (Angima et al., 2003; Mutua et al., 2006; Fwamba et al., 2017; Moses et al., 2017; Wekulo et al., 2017; Njiru et al., 2018). For a given catchment, the RUSLE factors were generated from five primary data themes. These included precipitation, soil data, land use/land cover, digital elevation model (DEM) and conservation support practices, (Renard et al., 1997). The data themes were multiplied in GIS environment from which a spatial soil erosion map was generated, (Moses, 2017). From the soil erosion map, critical areas that were in urgent need of soil and water conservation management were easily identified.
Soil Erosion Risk
The susceptibility of soil erosion is evaluated by considering factors such as climate, topography, soil erodibility, land use/cover, surface cover and conservation measures, (Renard et al., 1997). Thus, assessing the risk of soil erosion potential is an essential step in conservation of soil and management of the environment, (Fwamba et al., 2017). Importantly, the risk of soil erosion serves as a benchmark for management of land and for developing support services for control of soil erosion. However, erosion hazard studies have not been widely carried out in Kenya. With the fact that nearly 83 percent of Kenyas land is arid and semi-arid, there is need to extensively map out the erosion hazard since erosion rates are high in such areas.
Erosion hazard is evaluated on the basis of some environmental factors which include erosivity of rainfall, erodibility of soil and the length and steepness of the slope. Because anthropogenic pressures play a significant role in loss of soil within catchments, their effects should be monitored first prior to the estimation of the risk of soil erosion. This may be achieved using the catchment approach, (Wekulo, 2017). With the use of a DEM with significant resolution comparison of precision may be carried out before the RUSLE model is executed for estimation of the risk of soil erosion, (Wekulo, 2017).
In conclusion, catchment erosion and sedimentation in surface water bodies is a serious problem in Kenyas semi-arid areas. Therefore, management of soil and water in catchments must be prioritized. Most importantly, proper monitoring of catchments should be done in view of establishing the rates at which soil is lost with time. This step would provide crucial site-specific data which could guide appropriate conservation measures within catchments. Therefore, an all-inclusive environmental management approach is recommended in order to develop sound mitigation plans for control of soil erosion.
REFERENCES
-
S. Angima, D. Stott, M. ONeill, C. Ong, and G. Weesies, Soil Erosion Prediction Using RUSLE for Central Kenyan Highland Conditions, Agricultural, Ecosystems and Environment, vol. 97, pp. 295-308. 2003.
-
C.D. Biggelaar, R. Lal, K. Wiebe, and V. Breneman, The global impact of soil erosion on productivity: I: absolute and relativity erosion-induced yield losses, Adv. Agro, vol, 81:1 48. 2013.
-
T. Brown, H. Schneider, and D. Harper, Multi-scale estimates of erosion and sediment yields in the Upper Tana basin, Kenya. Erosion and Sediment Yield: Global and Regional Perspectives (Proceedings of the Exeter Symposium, July 1996). IAHS, vol. 236: 49 54, 1996.
-
Z. Chen, and T.M. Shah, An Introduction to the Global Soil Status in R Schaldach & R Otterpohl (eds), RUVIVAL Publication Series, Hamburg, Vol. 5:7 17, 2019.
-
L. Claessens, P. Van Breugel, A. Notenbaert, M. Herrero, and V.J. De Steeg, Mapping potential soil erosion in East Africa using the Universal Soil Loss Equation and secondary data. Sediment Dynamics in Changing Environments (Proceedings of a symposium held in Christchurch, New Zealand), IAHS Press, 398
407. 2008
-
J. De Graff. Soil conservation and sustainable land use: An economic approach Amsterdam, The Netherlands: Royal Tropical Institute.
-
J.B. De Graffenried, and D.K. Shepherd, Rapid Erosion Modeling in a Western Kenya Watershed using Visible Near Infrared Reflectance, Classification Tree Analyis and 137Cesium,
Geoderma, vol. 154, issu. 1-2, pp. 93 – 100. 2009
-
E.H. Dregne, Erosion and soil productivity in Africa, J. Soil Water Conserv., vol. 45, pp. 431 – 436. 1990.
-
N. Efthimiou, E. Lykoudi, and C. Karavitis, Soil erosion assessment using the RUSLE model and GIS, European Water, vol. 47 pp. 15 – 30. 2014.
-
A.A. Fenta, A. Tsunekawa, N. Haregeweyn, J. Poesen, M. Tsubo,
P. Borelli, P. Panagos, M. Vanmaercke, J. Broeckx, H. Yasuda, T. Kawai, and Y. Kurosaki, Land susceptibility to water and wind erosion risks in the East Africa region, Science of the Total Environment, vol. 703, pp. 1 20. 2020.
-
D. Fleitmann, R.B. Dunbar, M. McCulloch, M. Mudelsee, M Vuille, T.R. McClanahan, J.E. Cole, and S. Eggins, East African soil erosion recorded in a 300 year old coral colony from Kenya, Geophysical Research Letters, vol. 34: 1 5. 2007.
-
W.S. Fwamba, S.S. China, and E.N. Masibayi, Estimation of Spatial Distribution of Potential Soil Erosion Risk in Isiukhu River Catchment, Kakamega County, Kenya, International Journal of Engineering Research & Technology, vol. 6, issu. 7, pp. 159 163. 2017.
-
M. Gianinetto, M. Aiello, F. Polinelli, F. Frassy, M.C. Rulli, G. Ravazzani, D. Bocchiola, D.D. Chiarelli, A. Soncini, and R. Vezzoli, R., D-RUSLE: a dynamic model to estimate potential soil erosion with satellite time series in the Italian Alps, European Journal of Remote Sensing, 1 – 20. 2019.
-
N. Haregeweyn, J. Poesen, J. Nyssen, J. De Wit, M. Haile, G. Govers, and S. Deckers, Reservoirs inTigray ( Nothern Ethiopia): Characteristics and Sediment Deposition Problems, Land Degradation & Development, vol. 17, pp. 211 230. 2006.
-
M. Herrero, C. Ringler, J. Van De Steeg, P. Thornton, T. Zhu, E. Bryan, A. Omolo, J. Koo, and A. Notenbaert, A, Climate variability and climate change and their impacts on Kenyas agricultural sector. Nairobi: ILRI, ILRI Research report 22. 2010.
-
J.E. Hunink, I.A. Niadas, P. Antonaropoulos, P. Droogers, and J. De Vente, J., Targeting of intervention areas to reduce reservoir sedimentation in the Tana catchment (Kenya) using SWAT, Hydrological Sciences Journal, AHS Press, pp. 1 – 15. 2013.
-
M. Kidane, A. Bezie, N. Kesete, and T. Tolessa, The impact of land use and land cover (LULC) dynamics on soil erosion and sediment yield in Ethiopia, Heliyon, vol. 5, pp. 1 13. 2019.
-
J.M. Kirkby, J.B. Irvine, J.R. Jones, G. Govers, and P. Team, The PESERA coarse scale erosion model for Europe. I.Model rationale and implementation, Eur. J. Soil Sci. vol. 59, pp. 1293 1306. 2008.
-
B.M. Mutua, and A. Klik, Soil erosion management at a large catchment scale using the RUSLE-GIS: The case of Masinga catchment, Kenya, Management Information Systems, C. A. Brebbia (Editor), WIT Press, pp. 287 296.
-
B.M. Mutua, A. Klik, and W. Loiskandl, Modelling Soil Erosion and Sediment Yield at a Catchment Scale: The Case of Masinga Catchment, Kenya. Land Degradation & Development, vol. 17, pp. 557 570. 2006.
-
B.M. Mati, C.P.R. Morgan, G.N. Francis, J.N. Quinton, T.R. Brewer, and H.P. Liniger, Assessment of erosion hazard with the USLE and GIS: A case study of the Upper Ewaso Ng'iro North basin of Kenya, JAG, vol. 2, issu. 2, pp. 78 86. 2000.
-
B.M. Mati, C.P.R. Morgan, and J.N. Quinton, Soil erosion modelling with EUROSEM at Embori and Mukogodo catchments, Kenya, Earth Surface Processes and Landforms, vol. 31, pp. 579
588. 2006.
-
C.P.R. Morgan, J.N. Quinton, E.R. Smith, G. Govers, A.W.J. Poesen, K. Auerswald, G. Chisci, D. Torri, and E.M. Styczen, The European soil erosion model (EUROSEM): A dynamic approach for predicting sediment transport from elds and small catchments, Earth Surface Processes and Landforms, vol. 23, pp. 527544. 1998.
-
A.N. Moses, GIS-RUSLE Interphase Modelling of Soil Erosion Hazard and Estimation of Sediment Yield for River Nzoia Basin in Kenya, Journal of Remote Sensing & GIS, vol. 6, issu. 3, pp. 1 13. 2017.
-
Njiru, N.G., Kariuki, P. and Mwetu, K. (2018). Modelling Soil Erosion for Land Management in Ungauged Golole Catchment in Marsabit County, Kenya. Open Journal of Soil Science, Vol. 8(11): 277 302
-
J.O. Onyando, P. Kisoyan, and C.M. Chemilil, Estimation of Potential Soil Erosion for River Perkerra Catchment in Kenya, Water Resources Management, vol. 19, pp. 133 – 143. 2005.
-
G.S. Ongwenyi, S.M. Kithiia, and F.O. Denga, An overview of the soil erosion and sedimentation problems in Kenya. Sediment Problems: Strategies for Monitoring, Prediction and Control (Proceedings of the Yokohama Symposium, July 1993), IAHS Publ. no. 217. 1993.
-
P. Panagos, and A. Katsoyiannis, Soil erosion modelling: The new challenges as the result of policy developments in Europe, Environmental Research, vol. 172, pp. 470 474. 2019.
-
P. Panagos, C. Ballabio, P. Borrelli, K. Meusburger, A. Klik, S. Rousseva, M.P. Tadic, S. Michaelides, M. Hrabalikova, P. Olsen,
L.M. Aalto, A. Rymszewicz, A. Dumitrescu, S. Begueria, and C. Alewell, Rainfall Erosivity in Europe, Science of the Total Environment, vol. 511, pp. 801 814. 2018.
-
K. Renard, G. Foster, G. Weesies, D. Mc Cool, and D. Yoder, Predicting soil erosion by water: A guide to conservation planning with the Revised Universal Soil Loss Equation (RUSLE), USDA Agriculture Handbook No.703. United States Government Printing Ofce, Washington, DC. 384. 1997.
-
C.M. Rulli, L. Oeddu, and M. Santini, Modeling post-re water erosion mitigation strategies, Hydrology and Earth System Science, vol. 17, issue 6, pp. 23232337. 2013.
-
A. Saha, P. Ghosh, and B. Mitra, GIS Based Soil Erosion Estimation Using Rusle Model: A Case Study of Upper Kangsabati Watershed, West Bengal, India, International Journal of Environmental Sciences & Natural Resources, vol. 13, issu. 5, pp. 119 126. 2018.
-
M.N.A. Siddique, J. Sultana, M.R. Abdullah, and K.N. Azad, Modelling of Soil Loss through RUSLE2 for Soil Management in an Agricultural Field of Uccle, Belgium, British Journal of Environment & Climate Change, vol. 7, issu. 4, pp. 252-260. 2017.
-
S.F. Wekulo, Determination of Actual soil erosion risk with change in land use/land cover in Isiukhu river catchment, Kakamega County, Kenya, International Journal of Scientific and Research Publications, vol.7, issu. 7, 248 254. 2017.