
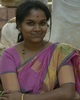
- Open Access
- Authors : Vindhya Shree M P , Shashikiran C R , Nandish Shanabog C S
- Paper ID : IJERTV9IS050465
- Volume & Issue : Volume 09, Issue 05 (May 2020)
- Published (First Online): 25-05-2020
- ISSN (Online) : 2278-0181
- Publisher Name : IJERT
- License:
This work is licensed under a Creative Commons Attribution 4.0 International License
Prioritization of Accident Black Spots using GIS
Vindhya Shree M P
Department of Civil Engineering, Rajarajeshwari College of Engineering, Bangalore, India.
Nandish Shanabog C S
Shashikiran C R
Department of Civil Engineering,
-
College of Engineering, Bangalore, India.
Capgemini Technology Services India Limited, Bangalore, India.
Abstract In a dubious distinction for the country, the World Health Organization has revealed in its first ever Global Status Report on Road Safety that more people die in road accidents in India than anywhere else in the world, including the more populous China. At least 13 people die every hour in road accidents in the country, the latest report of the National Crime Records Bureau reveals. In 2009, 1.25 lakh people in India lost their lives in road mishaps. Bangalore today is obviously one of the most sought after cities in the country with the rapid growth in the IT industry and the rise in the number of job opportunities in the city. With the rising population in the city there is also a corresponding increase in the number of vehicles as well as accidents. Accidents in the present era are contributing to major deaths worldwide due to increase in vehicular density. It has been estimated that over 3, 00,000 persons die and 1-1.5 lakh persons are injured every single year in road accidents throughout the world. Though the occurring of accidents cannot be completely excluded but still it is necessary to incorporate steps, which can reduce road accident rates and implement mitigating actions, to reduce the number and severity of road accidents. Present study includes the accident analysis in which prioritization of some major accident spots generally referred to as Black spots is done by the use of ARCGIS 10 software package. The study area includes major accident spots in south Bangalore, Karnataka. The study includes visiting these accident prone sites, collecting required data for GIS analysis and cross-checking the data with Bangalore traffic police records. Selected sites for present study include East end circle, Kudlu gate junction, Anepalya road, Khodays circle, Binny mills circle and Gubbalala gate. Two data points were taken for each black spot. Road maps of selected sited were collected and fed into software. The analysis was carries out and Anepalya road was found out to be the prime accident prone location. The results were cross checked with respective traffic police station records to verify the validity of the model.
KeywordsGIS, black spots, accidents, ARC GIS.
-
INTRODUCTION
It has been estimated that over 3,00,000 persons die and 1-
-
lakh persons are injured every single year in road accidents throughout the world. Detailed analyses of global accident statistics indicate that fatality rates per licensed vehicle in developing countries are very high in comparison with the industrialized countries. Moreover, road accidents have been shown to cost around 1% of annual gross national product (GNP) resources of the developing countries, which they can ill-afford to lose. Rapid population growth because of IT and other associated industries in Bangalore led to an increase in the vehicular population to about 1.5 million, with an annual
growth rate of 7-10%. Quite obviously personalized modes of transport have grown at a tremendous rate and two wheelers along with the cars almost 90 percent of total registered vehicular population in the city. A table below shows the trip sharing mode in Bangalore city.
As the vehicular density increases, quite obviously the chance of occurrence of accidents also increases, hence it is necessary to incorporate steps, which can reduce road accident rates and implement mitigating actions, which can be taken to reduce the number and severity of road accidents. A table below shows the reported number of accidents both fatal and non-fatal occurred in Bangalore till 2012, June.
Various strategies and countermeasures have been taken from time to time to reduce road accidents. One of the most important factors in preventing road accidents is systematic identification and treatment of hazardous locations. The various factors that because accidents can be broadly categorized into road related, vehicle related and driver related. In this paper, an attempt is made to implement the road related factors for predicting the accident prone points on roads and thus help in identifying the required remedial measures.
Methodologies for predicting accidents have been widely studied in the past. The prediction models are mostly causative types in which the number of accidents is taken as a function of number of independent variables. Recently there have been studies to identify accident-prone locations using fuzzy and neural network classifier approaches. The most common methodological approach used in the research efforts to model interaction between the highway geometries, traffic characterization and accident frequency is regression analysis. The ease of modeling readily favors the regression approaches. However, such methods are highly dependent on traffic flow data like Average Daily Traffic (ADT) and the data collected by the traffic police from the accident sites. But traffic flow data are rarely available in sufficient quantity or accuracy to justify these regression approaches. Moreover, the traffic police may not be able to collect all the necessary data required to carry out the analysis using that data.
Considering all the factors mentioned above, it is necessary to develop a model which can assist in identifying black spots on a given road network. This paper describes a model developed to identify black spots on roads using GIS. As a road network is distributed over a given area, it always possesses a spatial characteristic i.e. geographic locations associated with it. Thus, in order to model a road network, an in-formation system (GIS) capable of processing spatial data is required. A GIS can easily handle, store, analyze, manipulate and retrieve
spatial data. Therefore, by implementation of GIS a model for identifying accident-prone location on roads can be easily developed.
-
-
OBJECTIVES
The prime objectives for this study are mentioned below.
-
To identify various road related factors causing accidents
-
To develop a model to prioritize hazardous accident locations termed as black spots.
-
To carry out analysis of Black spots using GIS.
-
Comparison of real time data with records available in the police station.
-
-
SCOPE
A Geographic Information System (GIS) is a computer sys- tem for capturing, storing, querying, analyzing and display-ing geographic data. The essential aspect of GIS is the use of concept of location as the basis of structuring of information systems. A GIS can be effectively used to identify accident black spots on roads. The capability of GIS to link
attribute data with spatial data facilitates prioritization of accident occurrence on roads and the results can be displayed graphically which can be used for planning and decision making. The work done in this project will show the severity of black spot in comparison with others and help the agencies to work out prioritization as to undergo treatment. The study investigated sites based on the experienced accident history.
-
LITERATURE EVIEW
General
GIS permits users to display information geographically. It can also provide a common link between two or more previously unrelated databases The most useful aspect of GIS as a management tool is its ability to associate spatial objects (street names, milepost, route number, etc.) with attribute in- formation (accidents, cause, etc.). Most of the documents re- viewed consider the use of GIS in transportation under either for general data maintenance or for simple data analysis.
Several studies describe how GIS help the integration of many transportation elements. Meyer and Sarasua (1996) envisioned a common and coordinated database system that will serve all aspects of transportation management such as congestion, pavement, bridges, safety, inter-modal activities, and public transportation. Martin (1993) did a similar study, in which he proves that incorporating GIS in a pavement management program improves the reporting and analysis of data through the production of maps and graphic displays.
GIS has been proven to work well in addressing transportation problems related to safety. Affum and Taylor (1996) de-scribed the development of a safety Evaluation Method for a Local Area Traffic Management (SELATM), which is a GIS based program for analyzing accident patterns over time and the evaluation of the safety benefits. GIS can also be implemented in determining roadway and surface conditions. This was proven by Gharaibeh et. Al. (1994) when they proposed to use GIS to obtain statistical and spatial analyses of road-way characteristics such as safety, congestion level and pavement conditions.
In a separate study by Johnson and Demetsky (1994), the capabilities of GIS in providing a framework for a management system were proven once again. It highlighted the fact that many transportation analysts reap benefits from improved access to data. Establishment of the geographic referencing scheme is the major contributor in making data more readily available.
There also have been studied that aimed at showing how GIS can be applied in accident management systems. Faghri and Raman (1995) developed a GIS-based traffic accident information system for Kent County, Delaware. Their system included knowledge about the occurrence of crashes, such as conditions of incident site, and frequency of incidents at any given location (mile-point) on a roadway. Since the early stages of GIS, it was noticed that a vision of information technology outside the traditional transportation data analysis and even outside GIS was needed to implement this technology (Lewis, 1990)
GIS in Railway Transportation
Soheil Ghyasvand, Asadollah Noroozi and Abbasali Jandaghi Alaei presented a paper on Identification and prioritization of Black spots on Iranian Railway network. They Railway traffic as a system consisting of three basic components that interact with each other, namely, the railway user, the vehicle and the track and its environment. According to their studies, an accident occurs when one or a combination of these components fails. As they quoted, The risk of a crash is not uniform throughout the railway network. At certain locations, the level of risk will be higher than the general level of risk in surrounding areas. Accident will tend to be concentrated at these relatively high-risk locations. Locations that have an abnormally high number of accidents are described as accident concentrated, high hazard, hazardous or black spot sites. Track safety engineering plays a vital role in influencing driver behavior as engineering measures. In this sequence, the track and its environment should assist the driver in making a series of correct decisions and, if not correct, pro- vide a forgiving track environment to reduce the severity of the accident. Track safety engineering can create such an environment by:
-
Controlling the rate of decision-making to a level that a driver is able to accommodate.
-
Providing information to the driver in such a manner that it facilitates quick and correct decisions.
According to their studies existing statistics in Iran showed that 21% of accidents were related to track and substructure factors and therefore, was a high potential for decreasing the number and severity of accidents by assessing the track safety of the Iranian railway network.
In this paper, they have analyzed the rail accidents in Iran and presented a suitable method according to track safety engineering, by comparing existing methods of other countries to detect high risk accident locations. Finally, they tried to adopt these methods to Iranian railway network. For short term periods (e.g. 3 years), they suggested reactive methods such as "using cumulative frequency of accidents in sections", to identify and prioritize black spots. Then by concentrating over these spots and offering corrective strategies, its effect on the number of accidents can be evaluated. They suggested that the best method to identify black spots was "safety engineering
assessment. According to them the usage of this method gave rise to a more exact identification of dangerous sections. Also they mentioned that imposing this method required efficient manpower, long term planning and cooperation of related organizations such as the ministry of roads, municipalities, police etc.
Block spot Investigation
I.A Sayer presented a paper on Accident Black spot investigation (1994). In his studies he suggested two complimentary approaches for accident investigation work. Accident reduction (in which measures are taken to reduce the number and severity of accidents) and accident prevention. Accident prevention is the application of remedial measures preventing accidents from taking place in the future.
As he quoted, Approaches to accident cluster reduction includes Single Site, Mass, Area, and Route Action plans. Of the four basic strategies, the potential for accident reduction using simple low-cost remedial measures at single hazardous sites is particularly high. In terms of accident reduction and prevention, local authorities in the UK have had considerable success with low-cost engineering safety improvements directed towards treating accident clusters at localized sites. He classified treatment into three -main categories as road safety engineering measures, vehicle safety improvements and measures aimed at improving road user behavior. Ac-cording to his studies advantages of the low-cost black spot approach included that the measures involved are affordable and that they can be introduced on an experimental basis until they have proven their worth. Low-cost measures can also provide a demonstration that the existing roads network can be made safer by small scale improvements without the need for large financial investments. He outlined twelve steps to be carried out by investigators in Black spot investigation.
Determining the block spots
In the Design Mobility Plan Flanders (2001), the Flemish government states that a long term increase in traffic safety can only be achieved by means of an integrated approach towards vehicle safety, road safety (and its environment) and the required behavior of the road user. Especially the interaction of these three factors will cause an accident to occur or not. Therefore, ideally traffic safety measures should aim at the interaction of these three factors.
To determine the most dangerous accident sites, the Flemish government analyses the accident data that are obtained from the Belgian Analysis Form for Traffic Accidents. This form should be filled out by a police officer for each traffic accident that occurs with injured or deadly wounded casualties on a public road in Belgium. Based on these data, the following criterion is used. First, each site where in the last three years three or more accidents have occurred is selected. Then, a site is considered to be dangerous when its priority value (P), calculated using the following formula, equals 15 or more:
P = X + 3*Y + 5*Z (1)
Where, X = total number of light injuries Y = total number of serious injuries
Z = total number of deadly injuris
Based on this criterion, in Flanders currently approximately 800 sites should still be considered as dangerous. Starting
from 2003, the Flemish minister of Traffic, Steve Stevaert, will invest 100 million euro to redesign these dangerous locations for a period of 5 years. When evaluating the trends in accident figures, the Design Mobility Plan (2001) concludes that their policy aimed at the reduction of the number of accidents on these black spots certainly has been successful so far. However, these trends also point out that accidents tend to occur less concentrated in space, which in future will limit the effectiveness of similar infrastructure safety measures. Furthermore, being able to determine where crashes occurred is vital for the analysis of Black Spots. There are two issues associated with this: correctly identifying the location of the crash; and consistently recording the location in the road crash database so that details of all crashes at that location are readily accessible.
As mentioned in the Design Report of the Status Questioners Flanders (2002), the accident data for Flanders are located in a Geographical Information System (GIS). When necessary, this pinpointing is done with the help of official police reports. The GIS tools allow for the local authorities to analyze the located accident data in Access databases. However, this re-search is usually limited to the analysis of the evolution of accident data in relation to certain infrastructure parameters such as the number of accidents in the built-up area or the number of accidents on numbered roads. After the black spots are identified by means of the priority value criterion, the GIS software is also used to map these locations on the Belgian road network (see e.g. the Design Mobility Plan Flanders (2001)).
Hotspot safety work
On the Danish road network, the national Road Directorate is the primary developer of the theoretical foundation of the hot spot safety work. The Road Directorate has classified the state and regional road network into road sections, roundabouts and intersections. Road sections are further classified into a number of groups, defined by geographical, geometrical and environmental (site) characteristics. For each group, a model describing variation in accident counts within the groups is used. These accident models are fairly simple (few parameters) because environmental characteristics, other than traffic flow, are not directly included as traits in the model. Research (Vistisen 2002) has pointed out that Poisson-gamma hierarchical generalized linear models better describe the variation in accident counts on the Danish roads and accordingly provide better estimates of site safety than the models currently at use in Denmark. The proposed accident models are disaggregated on time periods of one year, which assures that yearly changes in traffic as well as in other traits may be accounted for. In these models not only general trends in accident counts may be included, but also the so-called dispersion effects. These effects represent the site-specific conditions not included as traits in the model. In addition, a dispersion effect models interdependence between yearly accident counts at the same location. Since this dispersion effect expresses how the expected accident frequency at a location deviates from the expected accident frequency at locations with similar traits, it may be used for targeting black spots in the road network.
In general, this results in a marginally higher sensitivity than the method used on the Danish road network today. In Vistisen (2002) also a new method for estimating the effect of
hot spot treatment work is proposed. The model is based on the site safety estimates provided by the accident models, and takes into account the regression to the mean effect as well as changes in traffic flow and other traits. The proposed method is found to give better estimates of the effect of treatment than the method currently used in Denmark. In addition, the re- searchers claim that it outperforms the methods as yet suggested in the international literature. The improved estimates of treatment effect will improve the foundation for prioritizing of black spots and safety measures.
For over a decade, the Federal Government of Australia has operated programs to improve the physical condition or management of hazardous locations with a history of crashes involving death or serious injury. This evaluation relates to the Capital Funding for Black Spots Roads Program, more generally known as the Federal Road Safety Black Spot Program, which commenced in 199697 and is scheduled to conclude in 200102. In total, 983 black spot projects had been implemented under the Program as at 30 June 1999. The Australian Transport Safety Bureau (2001) administered the Pro-gram. A sample of 608 black spot projects around Australia undertaken between 1 July 1996 and 30 June 1999 was analyzed. The total cost of these projects was approximately $59.5 million.
This study adopted a before and after treatment approach. This methodology was chosen because of its compatibility with the nature of the data available for analysis. The evaluation compared the number and severity of crashes after the black spots were treated with the number and severity of crashes that would have been expected with no treatment. The expected crash history was estimated using the actual crash history of the black spots before treatment and data on other variables expected to affect crashes at black spots after treatment. A Poisson regression model was used to determine whether black spot treatments had a statistically significant effect.
Overall, the evaluation provides very strong evidence that the Program achieved its aim of improving safety at locations with a history of crashes involving death or serious injury. Nevertheless, the Program was not uniformly effective in reducing the number of casualty crashes. Not all road engineering treatments had a statistically significant effect.
In the capital cities, sealing road shoulders, edge lines, pedestrian facilities, and signs had no statistically significant effect on road safety. The lack of a statistically significant result on road safety from sealing road shoulders in capital cities is particularly interesting, as this was the fifth most popular treatment in expenditure terms, and accounted for nearly seven per cent of expenditure on urban black spot treatment. Attempts to improve lighting in the capital cities appear to have had a counterproductive effect. In regional areas, traffic islands on approach, indented right and left turn lanes, non-skid surfaces, and pedestrian facilities had no statistically significant effect on road safety.
On the other hand, there were many areas in which the Program had a dramatic effect in reducing the number of casualty crashes, and some engineering treatments were consistently very successful. Roundabouts and new traffic lights with no turn arrows appeared to be very successful in improving safety in both capital cities and regional areas, with the probability of such large improvements being due to chance being less than one in ten thousand. There was very strong
evidence that installing roundabouts successfully improved safety regardless of how expensive, and presumably how large, the roundabouts were. New traffic lights with turn arrows, medians and non-skid surfaces were similarly successful when used in capital cities. In regional areas, there is very strong evidence that signs and new traffic lights with turn arrows improved safety, and moderate evidence that medians, shoulder sealing, edge lines, and improved lighting increased safety. In capital cities, there is very strong evidence that traffic islands on approaches and indented right and left hand turns improved safety.
Overall, the Black Spot Program appears to have been highly effective in reducing the number of casualty crashes. It is estimated that the Program prevented around 32 fatal crashes and 1 539 serious cashes between 199697 and 199899. The Program is therefore estimated to have saved at least 32 lives and prevented a large number of injuries over these three years. Further benefits will continue to accrue over the life of the black spot treatments that were applied.
-
-
CASE STUDIES
2.6.1[6] Lim Yu Liang, Dadang Mohamed MA some and Law TeikHua from University Putra Malaysia have presented a report on Traffic Accident application using Geographic Information System in 2005, in which they concluded with six major steps in accident diagnosis and treatment framework in Malaysia.
Step 1: Collection and Compilation of Accident Data. The first step in the production of the national database was the compilation of the accident form in the respective Police Districts.
The forms were stocked temporary in a special storeroom at Police Districts before they were dispatching to the Road Safety Research Center (RSRC) in UPM.
Step 2: Computerization and Processing of the Accident Records. The data input, processing and analysis framework is summarized schematically. The MAAP Version 5 has been designed and customized in line with the POL27. This package has been adopted as the standard analysis package for analysis accident black spot problems in Malaysia.
Step 3: Identification and Prioritization of the Accident Black spot Locations. There are a number of the location identification systems that can be used for referencing the location of accidents. The location identification system can be based either on the road maps that are digitized and converted in DCM format or in MAAP textual output.
Step 4: Black spots Prioritization and Diagnosis. Ranking of black spot could then be carried out such as: Ranking by Accident Maps, Nodal Analysis, Analysis on Link Accidents, Analysis on Cell Accidents, ranking by Accident Point, Ranking by Accident Cost, and Kilometer-Post Analysis.
Step 5: Detailed Diagnosis and Countermeasure. This step outlines the methods of in-depth diagnosis and developed of countermeasure of a selected black spot. Site study was carried out to capture the near misses, approach speeds, vehicles and pedestrian flows and their maneuvers.
Step 6: Evaluation Techniques. There is also a number of evaluation techniques currently used in evaluating safety
interventions. The selection of each technique depends on the nature of work, availability of data and precision required. Among the available techniques are the cumulative plot techniques, chi-squared before and after analysis and multivariate analysis.
The GIS based application combines the information collection capabilities with the visualization. The GIS and Road Accident View System are a set of applications developed for managing accident database entries. The developed system was designed based on the University Putra Malaysia (UPM) community area and may be adapted very easily to any other places. The system was developed using Microsoft Visual Basic 6.0 in Windows XP platform. The database was designed in term of textual format. The Accident Report from UPM security unit used as the source for information needed in the database development. The location of the accident was recorded on a map. And by indication on it, the user can perform queries on a particular condition to get the number of accidents.
2.6.2[8] Nilesh Deshpande, Ishan Chanda and Shriniwas S. Arkatkar from BITS Pilani, Pilani presented a report on Accident Mapping and Analysis using Geographical Information Systems. Their report concerns regarding road safety in a developing country like India, which further amplifies in absence of proper framework to analyze the accident related data using modern tools and techniques, especially in semi-urban or rural setting. Geographical Information System (GIS) is being used as a tool for statistical analysis, to achieve the objectives of the paper. The study was conducted for Pilani town and surrounding areas.
Following conclusions were made regarding this report:
Overview: Feasibility of the implementation of safety analysis methods was checked in the case study of Pilani town and surrounding area. Data collection: First Information Report (FIR), available in Police station, was considered as most practically viable alternative for a data source, while collecting accident related information. The accuracy of the locations related data was found to be limited.
Analysis: Due to some issues such as lack of digitized data, inaccuracy in records of locations, improper mapping of accident locations and lack of a proper platform for analysis, a need for proper analysis using GIS was established. Methods: Locations with high frequency of accidents were determined using methods such as query based, point density estimation, kernel density estimation and Spatial auto-correlation. Empirical Bayes, kernel Density Estimation and spatial autocorrelation are considered as the three most appropriate methods for analyzing accident related data. Empirical Bayes could not be implemented in this study, due to certain restrictions, such as small dataset and constraints regarding complete set of data required for proper implementation of the method. Kernel Density method was found to be easily implementable, easy to understand and at the same time, very effective. Statistically significant cluster locations were determined using Spatial Autocorrelation. Spatial Autocorrelation method can be properly implemented on addition of a few spatial parameters such as demographics, geometrical design etc. Due to the possibility of utilization of spatial variables it can prove to be very beneficial and effective in analysis. On comparison, pertaining to the data currently available, Kernel Density can be considered as a more
appropriate method of accident data analysis. On availability of spatial data, Spatial Auto-correlation (Local) may be better suited for the analysis of accident locations. Recommendation & Future Scope: Proper data collection methods need to be conveyed to the personnel doing the ground work. This will result in improved accuracy of the accident dataset. The locations indicated in the analysis as high risk zones, need to be surveyed. Proper recommendations, related to increasing its safety are needed to be given and be readily implemented. Applicability of the proposed system needs to be checked over a larger location, with more number of data points available for analysis.
2.6.3[14] Tom Brijs, Dimitris Karlis, Filip Van den Bossche and Geert Wets presented a paper A Model for identifying and ranking dangerous accident locations. In this paper, a Bayesian procedure using a Markov Chain Monte Carlo (MCMC) was developed for ranking accident locations in Flanders, Belgium. The procedure takes into account not only the number of fatalities, but also the number of injuries (severe and light) and combines this information by means of a cost function in order to rank the sites.
From the traffic safety point of view, the most interesting insight offered by their model was that it did not only rank the sites but that it also took into account the variability of this ranking. Hence, for decision making, one can see whether the chosen sites are really the most dangerous or whether there are other sites with almost similar characteristics. It is important to note, however, that this paper does not provide a cost-benefit analysis of road infrastructure investments. To this end, one would need the cost of alternative road infrastructure investments together with their respective accident modification factors (AMFs). Indeed, per location, a number of alternatives may be available (e.g. decrease speed, construct a median barrier, etc.) to increase safety with different respective costs and effectiveness. Since this information is lacking, one could say that this paper proposes the optimal ranking given an unlimited available budget.
To illustrate the proposed model, accident data from 23184 accident locations in Flanders, Belgium are used anda cost function proposed by the European Transport Safety Council is adopted to illustrate the model. It is shown in the paper that the model produces insightful results that can help policy makers in prioritizing road infrastructure investments.
2.6.4[8] Mikulik Josef presented a paper on Identification of accident location by use of GPS and possibilities of its application in September, 2009. He concluded that accurate location of accident spot is the key for the correct evaluation of a link between road and accident process and for the right choice of improvement measures. He mentioned that use of GPS can offers this desirable level of exactitude and some countries have already introduced this tool in a limited scope.
According to his studies the use of GPS was introduced in the Czech Republic as the standard procedure of the accident registration system since July 1, 2006. As the follow-up the on- line system of visualization of accidents, including all registered parameters for each accident, was opened for the internal use of traffic police in 2007. In the next phase the system was adapted for wider use of road safety professionals and for open access of wide public. The publicly accessible web site was opened since December 1, 2008 and evoked lively interest of hundred thousand accesses during the first
days. The system enables to access each accident described by parameters investigated by police (excluding personal data) and to make own individual selections and summaries according these parameters.
The implementation was based on the results of the pilot project undertaken jointly by Traffic Police and Transport Research Centre. This cooperation, including further applications of the GPS use, demonstrates the efficient way of cooperation between traffic police and road safety research and its contribution in road accidents prevention.
2.6.5[2] Deepthi Jayan.K and B.Ganeshkumar presented a paper on identification of accident hot spots: A GIS based implementation for Kannur district, Kerala. In this study, an effort has been made to identify the accident prone zones within Kannur district, Kerala using GIS. For this purpose, the road accident data for the years 2006, 2007 and 2008 pertaining to Kannur district were used. Accident particulars like date, location, type of vehicle involved, number of persons injured or died were included in GIS database.
Accident analysis studies aimed at the identification of high rate accident location and safety deficient areas. The density function available in spatial analyst extension of the ARCGIS software was applied to identify the accident prone areas in Kannur district during the years 2006, 2007 and 2008. Both kernel and simple densities were applied in identifying the accident patterns. The road geometry was measured in accident prone locations to find out the cause for the accident. Based on the result, suggestions were provided to reduce the accidents in the future.
2.6.8[12] Sanjay Kumar Singh and Ashish Mishra have presented a paper on Road accident analysis entitled as Road accident analysis: A case study of Patna city in which they came up with some shocking facts and data. This study is based on the data collected from 14 police stations in Patna city. They concluded that the economic loss due to road accidents is of the order of Rs. 60 billion each year in India. According to their report as Patna is suffering from the problem of deaths and injuries, the number of fatal accidents as well as related fatality is increasing over the years. In 2000, persons killed per 100 accidents are alarmingly high, as high as 45. They mentioned that despite fatality rate being relatively low in Patna, fatality risk is higher than Indian average. During recent years 90% of road fatalities caused pedestrian deaths. Patna city traffic police identified NH-38 as the most accident prone location in the city where about 15% of all the accidents occurred during the period of their study.
-
THEORITICAL ASPECTS
-
Block Spot
The main problem in identifying the accident black spot is to determine the highest rate accident locations and the cause of these accidents. So far, there is no assured definition of black spots. Given a range of approaches to data collation and variations in areas and locations under consideration, investigating bodies differ in defining what constitutes a Black Spot. True black spots are locations that because of deficient, incorrect or inappropriate detailed road layout contain some locale accident or injury factors. On the contrary, false black spots are locations that wrongly have been identified due to a randomly high number of accidents in the used identification period.
Black Spot may not necessarily be selected on the basis of number and severity of accidents reported alone. They can also be chosen on ranking or weighting of accident severity or traffic flow. Traffic flow based criteria requires a reliable and constant source of traffic data. Reliable, long term traffic counting information is difficult and expensive to acquire. Other factors reflected in accident weighting schemes can include type of road user injured, severity of injury and accident costs.
At certain sites, the level of risk will be higher than the general level of risk in surrounding areas. Crashes will tend to be concentrated at these relatively high-risk locations. Locations that have an abnormally high number of crashes are described as crash concentrated, high hazard, hazardous, hot spot or black spot sites. Generally we can define an accident Black Spot as a term used in road safety management to denote a place where road traffic accidents have historically been concentrated. It may have occurred for a variety of reasons, such as a sharp drop or corner in a straight road, so oncoming traffic is concealed, a hidden junction on a fast road, poor or concealed warning signs at a cross-road. The points which have potentially dangerous features are described as accident prone spots or black spots.
In a general classification, the identification of accident prone areas can be made either by reactive or proactive methods. Reactive method develops according to statistical data of the traffic amount, number and type of accidents. The accuracy of reactive method relies on a great extent to the accuracy of data collection and storage. This method can identify black spots. Proactive method is based on physical properties and operational conditions. In fact, safety of operation in a route can be evaluated by means of key factors and the way of operations and hence the safety indicator can be identified. These methods require a lot of time, precision and also making use of safety experts. The result of these methods is a prioritized list of accident prone areas or black spots. In this study black spots or accident prone areas were identified by reactive approach that included collecting accident record data from Bangalore traffic police stations.
-
Geographic Information System (GIS) Introduction
A geographic information system (GIS) is a computer system for capturing, storing, querying, analyzing and displaying geographic data. GIS represents a new paradigm for the organization of the information and the design of information system, the essential aspect of which is the use of concept of location as the basis of structuring of information systems. GIS technology can be viewed as an offshoot from two major software technologies i.e., database management system (DBMS) and computer aided design (CAD), with the addition of specialized functions for managing and analyzing spatial data i.e., data that can be referenced to a geographical location. The objective of any GIS system is to integrate hardware, software, and data for capturing, managing, analyzing, and displaying all forms of geographically referenced information. GIS allows us to view, understand, question, interpret, and visualize data in many ways that reveal relationships, patterns, and trends in the formof maps, globes, reports, and charts.
Today, GIS is used by thousands of different organizations or individuals to access varied sets of geographically related information. A GIS helps you answer questions and solve problems by looking at your data in a way that is quickly understood and easily shared.
GIS is a powerful tool for managing large amounts of heterogeneous data. A GIS can be effectively used to prioritize black spots on roads. The capability of GIS to link attributes data with spatial data facilitates prioritization of accident occurrence on roads and the results can be displayed graphically which can be used for planning and decision making. In the present study, GIS analysis is performed using ARCGIS 10 package.
Benefits of GIS
GIS benefits organizations of all sizes and in almost every industry. There is a growing awareness of the economic and strategic value of GIS. The benefits of GIS generally fall into five basic categories:
-
Cost saving and increased efficiency GIS is widely used to optimize maintenance schedules and daily fleet movements. Typical implementations can result in a savings of 10 to 30 percent in operational expenses through reduction in fuel use and staff time, improved customer service, and more efficient scheduling.
-
Better decision making GIS is the go-to technology for making better decisions about location. Common examples include real estate site selection, route/corridor selection, evacuation planning, conservation, natural resource extraction, etc. Making correct decisions about location is critical to the success of an organization.
-
Improved communication GIS-based maps and visualizations greatly assist in understanding situations and in storytelling. They are a type of language that improves communication between different teams, departments, disciplines, professional fields, organizations, and the public.
-
Better recordkeeping Many organizations have a primary responsibility of maintaining authoritative records about the status and change of geography. GIS provides a strong framework for managing these types of records with full transaction support and reporting tools.
-
Managing geographically GIS is becoming essential to understanding what is happening and what will happen in geographic space. Once we understand, we can prescribe action. This new approach to management (managing geographically) is transforming the way that organizations operate.
Functions
Some of the basic functions of GIS are mentioned below:
-
Map where things are
Mapping where things are lets you find places that have the features you're looking for and to see patterns.
-
Map quantities
People map quantities to find places that meet their criteria and take action. Public health officials might want to map the
numbers of physicians per 1,000 people in each census tract to identify which areas are adequately served, and which are not.
-
Map densities
A density map lets you measure the number of features using a uniform areal unit so you can clearly see the distribution. This is especially useful when mapping areas, such as census tracts or counties, which vary greatly in size.
-
Find whats inside
Use GIS to monitor what's happening and to take specific action by mapping what's inside a specific area. For example, a district attorney would monitor drug-related arrests to find out if an arrest is within 1,000 feet of a school–if so, stiffer penalties apply.
-
Map change
Map the change in an area to anticipate future conditions, decide on a course of action, or to evaluate the results of an action or policy. By mapping where and how things move over a period of time, you can gain insight into how they behave. For example, a meteorologist might study the paths of hurricanes to predict where and when they might occur in the future.
The Geographic Approach
The approach to any geographical problem and its solution is divided into following five phases:
-
Ask
Approaching a problem geographically involves framing the question from a location-based perspective. What is the problem you are trying to solve or analyze, and where is it located?
-
Acquire
After clearly defining the problem, it is necessary to determine the data needed to complete your analysis and ascertain where that data can be found or generated.
-
Examine
You will not know for certain whether the data you have acquired is appropriate for your study until you thoroughly examine it.
-
Analyze
The data is processed and analyzed based on the method of examination or analysis one chooses, which is dependent on the results one hopes to achieve.
-
Act
The results and presentation of the analysis are important parts of The Geographic Approach. The results can be shared through reports, maps, tables, and charts and delivered in printed form or digitally over a network or on the Web. You need to decide on the best means for presenting your analysis. You can compare the results from different analyses and see which method presents the information most accurately.
GIS Application in Transportation
In recent years, there has been much discussion about GIS technology and applications across a wide variety of settings. Moreover, there have been many GIS-related developments in
transportation planning and engineering (FHWA, 1993; Lewis, 1990; Kim and Levine, 1996). The power of them is rooted in the fact that GIS allows inferences to be drawn about the spatial nature of the data. Examples of GIS applications in transportation include pavement management systems that work with road segments, optimal vehicle routing, Automated Mapping Facilities Management (AM/FM) used for infrastructure management, drainage design, traffic modeling and accident analysis, demographic analysis for funding justification, and the option of displaying any form of tabular data that has a spatial component.
Spatial Relationships and Technology
When viewing a map, the map-reader must interpret a variety of points, lines and other symbols to identify spatial relationships among the geographic entities represented. For example, you can use a map to find a route from one city to another. The information required to perform this analysis is not explicit in the map; rather, the map-reader must interpret the required spatial relationships from mapped objects. In a GIS database, the method by which spatial relationships are explicitly represented is termed topology. Topology is used to describe how linear objects connect, to define areas, and to identify the areas lying to either side of a linear object. Information about the spatial relationships is stored in a topological data structure and is essential to carrying out most GIS functions.
ArcGIS 10
Esri's ArcGIS is a geographic information system (GIS) for working with maps and geographic information. It is used for: creating and using maps; compiling geographic data; analyzing mapped information; sharing and discovering geographic information; using maps and geographic information in a range of applications; and managing geographic information in a database. The system provides an infrastructure for making maps and geographic information available throughout an organization, across a community, and openly on the Web.
ArcGIS includes the following Windows desktop software:
-
ArcReader is a basic data viewer for maps and GIS data published in the proprietary Esri format using ArcGIS publisher. The software also provides some basic tools for map viewing, printing and querying of spatial data. ArcReader is included with any of the ArcGIS suite of products, and is also available for free to download. ArcReader only works with preauthored published map files, created with ArcGIS Publisher.
-
ArcView is the entry evel of ArcGIS licensing offered. With ArcView, one is able to view and edit GIS data held in flat files, or view data stored in a relational database management system by accessing it through ArcSDE.
-
ArcEditor is the midlevel software suite designed for advanced editing of spatial data published in the proprietary Esri format. It provides tools for the creation of map and spatial data used in GIS, including the ability of editing geodatabase files and data, multiuser geodatabase editing, versioning, raster data editing and vectorization, advanced vector data
editing, managing coverages, coordinate geometry, and editing geometric networks. ArcEditor is not intended for advanced spatial analysis.
-
ArcInfo allows users the most flexibility and control in all aspects of data building, modeling, analysis, and map display. ArcInfo includes increased capability in the areas of spatial analysis, geoprocessing, data management, and others.
-
ArcMap is the application used to view, edit and query geospatial data, and create maps. The ArcMap interface has two main sections, including a table of contents on the left and the data frame which display the map. Items in the table of contents correspond with layers on the map.
-
-
RESEARCH METHODOLOGY
-
-
Methedology
The model described in this paper requires a map of the desired road network digitized in a suitable form and certain specified road attributes to carry out prioritization The analysis then ranks black spots on the given road network. While carrying out the analysis the model incorporates only the road related factors such as road geometries, which lead to accidents. The factors considered are as follows:
-
Road width
-
Number of lanes in each direction
-
Approximate number of vehicles per day
-
Drainage facilities
-
Surface condition of the pavement
-
Frequent vehicle type
-
Presence of shoulders, edge obstructions and median barriers
-
-
Procedure
In order to model using mentioned road related factors and achieve the desired result, a step by step procedure as given below is adopted.
-
Scanning of the map containing the desired road network and feeding this image to Arc View for digitizing.
-
Digitizing the road network with due considerations for separation of every link and assigning ID number to every link.
-
Specifying the attributes for every road link using the questionnaire provided.
-
Exporting the road attribute table generated in database format so that it can be imported by Arc view.
-
Joining the road attribute table to the digitized road map and prioritizing the road network for accident occurrence using total weights assigned to every link.
-
Black spots on a given road network are ranked by result obtained from prioritization.
-
-
Prioritization
Prioritization involves assigning suitable weights to different factors so as to achieve a desired result. In this model, the various factors, which tend to influence the occurrence of accidents on roads, are assigned weights on a scale of 0-10 in
such a manner that the factors which tend to increase the probability of the accidents have lower weights. In order to prioritize roads for occurrence of accidents, the various factors
-
General
-
RESEARCH ANALYSYS
Factors affecting occurrence of accidents
Possible variations
Weights assigned
Number of lanes in each direction
1
2
3
4
4
6
8
10
Number of vehicles per day
0-40,000
40,001-60,000
60,001-80,000
80,001-1, 00,000
1, 00,000 above
2
4
6
8
10
Width of the road
Less than 6 m 6.1-7.5 m
7.6-10.5 m
10.6-15 m
15 m above
2
4
6
8
10
Drainage facilities provided
Good Satisfactory Poor
No drainage
10
7
4
1
Surface condition of the pavement
Concrete WBM
Other bituminous Surface painted Earth roads
10
8
6
4
2
Frequent vehicle type on the road
Bus/Truck Car/3 wheeler Two wheeler Bicycles Carts
2
4
6
8
10
Presence of shoulders
Yes No
10
4
Presence of edge obstructions
Yes No
4
10
Provision of median barriers to channelize the traffic
Yes No
10
4
Factors affecting occurrence of accidents
Possible variations
Weights assigned
Number of lanes in each direction
1
2
3
4
4
6
8
10
Number of vehicles per day
0-40,000
40,001-60,000
60,001-80,000
80,001-1, 00,000
1, 00,000 above
2
4
6
8
10
Width of the road
Less than 6 m 6.1-7.5 m
7.6-10.5 m
10.6-15 m
15 m above
2
4
6
8
10
Drainage facilities provided
Good Satisfactory Poor
No drainage
10
7
4
1
Surface condition of the pavement
Concrete WBM
Other bituminous Surface painted Earth roads
10
8
6
4
2
Frequent vehicle type on the road
Bus/Truck Car/3 wheeler Two wheeler Bicycles Carts
2
4
6
8
10
Presence of shoulders
Yes No
10
4
Presence of edge obstructions
Yes No
4
10
Provision of median barriers to channelize the traffic
Yes No
10
4
considered and the weights assigned to them are given in following table:
Factors used in prioritization with possible weights.
The final weight assigned to each road link is obtained by adding all the individual weights and normalizing the value using maximum weight (in this case 90) that can be assigned.
Hence,
Total weight = ( Individual Weights) X 100 / 90 (1)
Thus road links with high final weight are less prone to accidents than the road link with low final weight. The classification of roads for occurrence of accidents based on final weights is done using the following classification scheme:
Final Weight
Accident Prone Level
80 100
Very low
60 80
Low
40 60
Medium
0 40
High
Prioritization scheme
The following places were selected for investigation on the
basis of experienced accident history in south Bangalore city.
-
East end circle
-
Kudlu gate junction
-
Anepalya road
-
Khodays circle
-
Binny mills circle
-
Gubbalala gate
The values of the various factors contributing to accidents were collected from these sites mentioned above. Accident records were also collected from the police stations of south Banglore city for validating the present work.
General steps involved in ARCGIS 10 are mentioned as follows:
-
For selected places, Road maps are downloaded using Google earth and USGS.
-
Using GPS, Latitude and longitude values for selected points are found.
-
The map image is then imported in to ARCGIS 10.
-
Geo-referencing is done with minimum four points for one spot.
-
Geo-referencing is done for all the sites in a map.
-
Map image is then digitized with shape file (a point in this case).
-
In the attribute table, desired columns are entered with their values.
-
Analysis is done to rank black spots.
-
-
-
Data Collection
Survey work includes collecting road and vehicle related data for all the selected points. Road related data includes counting the number of lanes, checking the conditions of drainage facilities, presence of edge obstructions, presence of median barriers at site whereas vehicle related data includes taking classified traffic volume surveys for each data point. Following data were collected from below mentioned sites:
East End Circle
Two points were selected at East end circle and from each point following data were collected.
Point1
Road width=15m
Number of lanes/direction=2
Approximate number of vehicles/day=82,696 PCU Drainage conditions=Satisfactory
Frequent vehicle type=2 wheelers Presence of shoulders=Yes
Edge obstructions=No Median barriers=Yes
Surface type=other bituminous
Point 2
Road width=15m
Number of lanes/direction=2
Approximate number of vehicles/day=85,552 PCU Drainage conditions=Satisfactory
Frequent vehicle type=2 wheelers Presence of shoulders=Yes
Edge obstructions=Yes Median barriers=Yes
Surface type=other bituminous
Kudlu Gate
Two points were selected near Kudlu gate junction and following data was collected:
Point1
Road width=40m
Number of lanes/direction=4
Approximate number of vehicles/day=1,04,010 PCU Drainage conditions=poor
Frequent vehicle type=2 wheelers Presence of shoulders=Yes
Edge obstructions=yes Median barriers=Yes
Surface type=other bituminous
Point2
Road width=40m
Number of lanes/direction=4
Approximate number of vehicles/day=1,01,172 PCU Drainage conditions=Satisfactory
Frequent vehicle type=2 wheelers Presence of shoulders=no
Edge obstructions=yes Median barriers=Yes
Surface type=other bituminous
Anepalya Road
Two points were selected on Anepalya road with following data recorded:
Point1
Road width=6m
Number of lanes/direction=1
Approximate number of vehicles/day=30,051 PCU Drainage conditions=Poor
Frequent vehicle type=2 wheelers Presence of shoulders=no
Edge obstructions=yes Median barriers=No
Surface type=other bituminous
Point2
Road width=6m
Number of lanes/direction=1
Approximate number of vehicles/day=29,511 PCU Drainage conditions=Satisfactory
Frequent vehicle type=2 wheelers Presence of shoulders=no
Edge obstructions=no Median barriers=no
Surface type=other bituminous
Khodays Circle
Two points were selected at Khodays circle with following data recorded:
Point1
Road width=15m
Number of lanes/direction=2
Approximate number of vehicles/day=1,11,528 PCU Drainage conditions=satisfactory
Frequent vehicle type=2 wheelers Presence of shoulders=yes
Edge obstructions=yes Median barriers=yes
Surface type=other bituminous
Point2
Road width=15m
Number of lanes/direction=2
Approximate number of vehicles/day=1,07,742 PCU Drainage conditions=poor
Frequent vehicle type=2 wheelers Presence of shoulders=yes
Edge obstructions=yes Median barriers=yes
Surface type=other bituminous
Binny mill Circle
Two points were selected at Binny mills circle with following data recorded:
Point1
Road width=10.5m
Number of lanes/direction=1
Approximate number of vehicles/day=34,920 PCU Drainage conditions=satisfactory
Frequent vehicle type=2 wheelers Presence of shoulders=yes
Edge obstructions=yes Median barriers=no
Surface type=other bituminous
Point2
Road width=10.5m
Number of lanes/direction=1
Approximate number of vehicles/day=34,497 PCU Drainage conditions=poor
Frequent vehicle type=2 wheelers Presence of shoulders=yes
Edge obstructions=no Median barriers=no
Surface type=other bituminous
Gubbalala Gate
Two points were selected at Gubbalala gate with following data recorded:
Point1
Road width=15m
Number of lanes/direction=2
Approximate number of vehicles/day=78,555 PCU Drainage conditions=Poor
Frequent vehicle type=2 wheelers Presence of shoulders=no
Edge obstructions=yes Median barriers=no
Surface type=other bituminous
Point2
Road width=15m
Number of lanes/direction=2
Approximate number of vehicles/day=78,183 PCU Drainage conditions=satisfactory
Frequent vehicle type=2 wheelers Presence of shoulders=no
Edge obstructions=yes Median barriers=no
Surface type=other bituminous
Name of black spots with value of factors
Name of Data Point
Total Weight
Name of Data Point
Total Weight
East end circle (point 1)
71
Khodays circle (point 1)
67
East end circle (point 2)
65
Khodays circle (point 2)
64
Kudlu gate (point 1)
70
Binny mills circle (point 1)
49
Kudlu gate (point 2)
67
Binny mills circle (point 2)
52
Anepalya road (point 1)
38
Gubbalala gate (point 1)
48
Anepalya road (point 2)
47
Gubbalala gate (point 2)
51
Name of Data Point
Total Weight
Name of Data Point
Total Weight
East end circle (point 1)
71
Khodays circle (point 1)
67
East end circle (point 2)
65
Khodays circle (point 2)
64
Kudlu gate (point 1)
70
Binny mills circle (point 1)
49
Kudlu gate (point 2)
67
Binny mills circle (point 2)
52
Anepalya road (point 1)
38
Gubbalala gate (point 1)
48
Anepalya road (point 2)
47
Gubbalala gate (point 2)
51
According to above table respective weights are given for specific values of factors and total weights are calculated for all the points as shown in the table below.
Data points with latitude and Longitude values
3. Importing of image to ARCMAP 10
The map images downloaded from USGS are imported in to ARCGIS 10.
Data points will be marked as (Square) and reference points as (Circle) in all the figures.
Khodays Circle
-
Work on ArcGIS 10
Points with assigned weights
The work carried out in ARCGIS 10 software is as follows.
-
Capturing images from USGS and other sources.
Every problem is GIS requires map of the desired places. In this case, images for the desired black spots were downloaded from site of Unites states geological survey which provides free map images for personal and professional use.
-
Collecting latitude and longitude values
Longitude and latitude values for all the data points were collected by use of GPS device.
Kudlu Gate
East end Circle
Binny mill Circle
The steps for georeferencing are outlined as follows:
-
Click on the add control points as highlighted in red circle. Then click on data points once.
-
Then right click to enter coordinates as shown above for data point 2 in Khodays circle.
-
After this, go to georeferencing bar on top and update georeferencing.
-
Repeat this for each data point for each site.
-
Click saves to complete georeferencing.
-
Creation of Shape File
Shape file is needed for digitization process in GIS analysis. The steps for creating a shape file are outlined below:
-
Go to ARC catalog on right hand side of screen.
-
Right click on project name, highlight new and select new Shape file.
-
Give name and type of Shape file (in this case type is point).
-
Go to edit and assign coordinate system as WGS- 1984.
Anepalya Road
-
-
Digitization
Creation of shape file
-
-
-
Georeferencing
There is a great deal of geographic data available in formats that cannot be immediately integrated with other GIS data. In order to use these types of data in GIS it is necessary to align it with existing geographically referenced data, this process is also called georeferencing.
After choosing an appropriate map, it is georeferenced so that it can be used in the analysis. Georeferencing means assigning latitude and longitude values (collected earlier using GPS) to the data points.
Image being georeferenced in ARCMAP
Digitizing is the process of making features we can see on the image and making them features to which additional spatial and non-spatial attributes can be assigned. This means we are going to follow a process of making digital versions of objects that will have an attribute table associated with them.
-
Add shape file to table of contents by add data bar.
-
Drop down editor tool bar and start editing.
-
Select shape file and feature and click on your data points.
-
Save edits and stop editing.
-
Adding attribute data
After digitization of image data is added to attribute table as follows:
-
Right click on shape file and open attribute table.
-
In table window, click table options and click add field.
-
Assign name for field and type of data you want to enter for that field.
-
Go to editor tool bar and start editing.
-
Add data in attribute table as shown below.
-
Save edits and stop editing.
Adding field in attribute table
Adding data in attribute table
-
-
Symbolization
There are several methods with which you can represent quantity on a map e.g. using colors, graduated symbols, proportional symbols, dot densities, bar/columns or charts. Bar and column charts have been used in this report which can present a number of quantitative fields in an eye-catching fashion. Generally, you'll draw a layer with bar and column charts when your layer has a number of related numeric attributes that you want to compare. Bar/column charts are useful for showing relative amounts rather than proportions or percentages. Steps have been outlined below:
-
Right click on shape file and go for properties.
-
Click symbology and select your type of chart e.g. Pie, Bar/column etc.
-
Select fields you wish to view.
-
Apply Ok.
-
As shown in above figure, Anepalya road (data point 1) has the minimum of weight from all the data points which is 38. So the analysis shows that Anepalya road (data point 1) is the most accident prone site amongst all the other sites.
Data points with total weights shown as bars
-
RESULTS, RECOMMENDATIONS AND CONCLUSIONS.
-
Results
A graph is developed for GIS analysis using ARCMAP 10 showing the following results:
Comparison of weights via graphical analysis
From the graph it is clear that Anepalya road (data point 1) has minimum weightage i.e.38, which according to the concept of prioritization in this report is the most accident prone point in South Bangalore. To compare the results and verify the validity of GIS approach, visits were made to traffic police stations for real time records. Visits were made to respective traffic police stations.
With purely sincere and kind help of Bangalore traffic police, following findings were made from police records.
List of traffic police stations visited are given below.
-
Mico layout traffic police station (East end circle)
-
Ashok nagar traffic police station (Anepalya road)
-
Subramanyapura traffic police station (Gubbalala gate)
-
Upparpet traffic police station (Khodays circle and Binny mills circle)
-
Madiwala traffic police station (Kudlu gate)
Name of the Block spot
No of Accidents (Pedestria ns with Vehicles)
No of Accidents (Pedestrian s with Vehicles)
No. of injuries
No. of deaths
Total no. of accidents
East End Circle (Point 1)
3
2
4
0
5
East End Circle (Point 2)
4
2
3
0
6
Kudlu Gate (Point 1)
5
2
4
1
7
Kudlu Gate (Point 2)
5
1
3
0
6
Anepalya Road (Point 1)
9
8
13
1
17
Anepalya Road (Point 2)
7
5
9
0
12
Khodays Circle (Point 1)
3
4
5
0
7
Khodays Circle (Point 1)
5
5
7
1
10
Binni mill Circle
5
4
6
0
9
Accident records of black spots from Jan-12 to july-12 (Source: Bangalore Traffic Police Stations)
As per the records given by Ashok nagar police station regarding Anepalya road, Anepalya road (data point 1) has reported maximum number of accidents i.e. total of 17 accidents including 13 injuries and one death and has minimum weightage of 38. Looking at other sites, East end circle (data point 1) has reported 5 accidents in total as per records of Mico layout traffic police station, which is a minimum among all other data points and also has maximum weightage of 71. So it is clear from the records that Anepalya road (data point 1) is the most accident prone location in South Bangalore and East end circle (data point 1) the least amongst the selected black spotswhich verifies that the GIS approach is valid and model can be applied to accident sites successfully.
-
-
Conclussions and Recommendations
The main advantage of using this approach for prioritizing accident black spots on roads is that it requires very less additional data other than road network map. So the results obtained from this model can be easily used for planning road safety measures. Also these can be supplemented with the results obtained by using other approaches. Moreover, the results can act as aquick guideline for road network planners and the authorities concerned with accident mitigation measures.
However, the accuracy of this model highly depends on the way in which the road network is digitized. The road geometry can be inferred incorrectly if it is not properly digitized.
The study shows that the highly prone accident point Anepalya road (data point 1) is ranked top most in priority list. The reasons for it being extremely dangerous are no provision of median barriers, presence of edge obstruction in form of a wall which goes along with the road and the wavy alignment of road.
Overview: Feasibility of the implementation of GIS analysis was checked in the case of south Bangalore.
Data collection: First Information Report (FIR), available in Police station, was considered as most practically viable alternative for a data source, while collecting accident related information. The accuracy of the locations related data was found to be limited.
Analysis: Due to some issues such as lack of digitized data, inaccuracy in records of locations, improper mapping of accident locations and lack of proper platform for analysis, a need for proper analysis using GIS was established.
(Point 1) |
|||||
Binni mill Circle (Point 2) |
4 |
4 |
6 |
0 |
8 |
Gubbalala Gate (Point 1) |
6 |
3 |
7 |
0 |
9 |
Gubbalala Gate (Point 2) |
7 |
1 |
5 |
1 |
8 |
(Point 1) |
|||||
Binni mill Circle (Point 2) |
4 |
4 |
6 |
0 |
8 |
Gubbalala Gate (Point 1) |
6 |
3 |
7 |
0 |
9 |
Gubbalala Gate (Point 2) |
7 |
1 |
5 |
1 |
8 |
Recommendation and Future Scope: Proper data collection methods need to be conveyed to the personnel doing the ground work. This will result in improved accuracy of the accident dataset. The locations indicated in the analysis as high risk zones need to be surveyed. Proper recommendations, related to increasing its safety are needed to be given and be readily implemented. Applicability of the proposed model needs to be checked over a larger location, with more number of data points available for analysis.
REFERENCES
-
D. Maguire, M.F. Goodchild, and D.W. Rhind (1994). Geographical Information systems, Principles and Application. Longman Scientific & Technical.
-
Deepthi Jayan.K and B.Ganesh Kumar, Identification of accident hot spots: A GIS based implementation for Kannur district-Kerala, 2009
-
G. B. Korte (1999). The GIS book, 4th Edition Updated & Expanded, onward Press.
-
I A Sayer Accident Black spot investigation 1994, pp. 151-156
-
K Gearts & G Wets, Black Spot Analysis Methods: Literature Review, 2003
-
Lim Yu Liang, Law Teik and Dadang Mohammad Masoem, Traffic Accident Application using Geographic Information System, Journal of the Eastern Asia Society for Transportation Studies, Vol. 6, pp. 3574 – 3589, 2005
-
Melissa Alexandra Larsen, Philadelphia Traffic Accident cluster analysis using GIS and SANET, Master of urban spatial analytics capstone project, 2010
-
Mikulik Josef, Identification of accident location by use of GPS and possibilities of its application, September, 2009
-
Michael J De Smith, Michael F Goodchild and Paul A Longley, Geospatial Analysis-A comprehensive guide to principles, techniques and software tools by, Troubador Publishing Ltd, 2007
-
Nilesh Deshpande, Ishan Chanda and Shriniwas S Arkatkar, Accident Mapping and Analysis Using Geographical Information Systems, International Journal of Earth Sciences and Engineering, ISSN 0974- 5904, Volume 04, No 06 SPL, pp. 342-345, 2011
-
Peter Connor, GIS for A-level geography, 2008
-
Sanjay Kumar Singh and Ashish Mishra, Road Accident Analysis-A case study of Patna city, Urban transport journal, pp. 60-75, 2005
-
Soheil Ghyasvand, Asadollah Noroozi , Abbasali Jandaghi Alaei, Development of a proper method for identifying hazardous locations by assessing the track safety, in IRAN, in Press.
-
Tom Brijs, Dimitris Karlis, Filip Van Den Bossche and Geert Wets, A Model for Identifying and Ranking Dangerous Accident Locations: A Case-Study in Flanders, 2007