
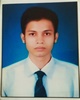
- Open Access
- Authors : Somil Vasal , Rajkumar Soni , Sourabh Kumar Jain
- Paper ID : IJERTV9IS060524
- Volume & Issue : Volume 09, Issue 06 (June 2020)
- Published (First Online): 22-06-2020
- ISSN (Online) : 2278-0181
- Publisher Name : IJERT
- License:
This work is licensed under a Creative Commons Attribution 4.0 International License
Comparative Analysis of Novel Coronavirus Organization and Recognition Methodology
Somil Vasal
Department of Computer Science & Engineering, Gyan Ganga Institute of Technology and Science, Jabalpur, India
Rajkumar Soni
Department of Computer Science & Engineering, Gyan Ganga Institute of Technology and Science, Jabalpur, India
Sourabh Kumar Jain
Department of Computer Science & Engineering, Gyan Ganga Institute of Technology and Science, Jabalpur, India
Abstract – The Novel coronavirus (COVID-19) to have a dangerous effect on the health and welfare of the global population. Novel coronavirus (COVID-19) is a transferable disease, and can also cause harm to the lungs. The main causes of quickly spread COVID-19 between the peoples are direct close contact or by coughing and sneezing of COVID-19 patients. Approximate 7,221,717 peoples are infected and 4,11,818 peoples are death worldwide due to COVID-19. In this significance, it is very much essential to recognize the infected people so that stop of spread can be taken. Therefore it is very important to identify, isolate, diagnosis, and treatment in this tenure. This paper is based on the different technique and literature based on the number of the article in the same field.
Keywordsnovel coronavirus; COVID; deep learning; analysis;
-
INTRODUCTION
The coronavirus (COVID-19) belong to SARS COV-2 family. The structure of coronavirus looks like a crown on their surfaces. SARS COV-2 can cause COVID-19, an infection that the reason primarily attacks your throat and lungs. coronavirus contains genetic information on making more copies of itself. The role of the protein shell offers a tough protective attachment to genetic material as the virus move between those who infect it. The part of the COVID virus imaged, called the spike protein, helps the virus attach to and infect human cells, and its construction comes just weeks after the virus's genome sequence was published.
Fig. 1. Structure of Novel Corona Virus [10]
Both a particular influenza virus and the new coronavirus use their spikes to enter inside a human body cell,
where it handles its internal system, remodel it to form components of the new virus. Whenever an infected person talks, coughs or sneezes, the virus-carrying droplets can enter in your mouth or nose, and then in their lungs. Once the virus comes inside the body, contact with cells in the throat, nose, or lungs and when the virus contacts the healthy cell membrane then the help of spikes it marge receptor – molecules. This action allows the virus to enter the cell and then specific flu virus will travel from the cell membrane to the nucleus. where the cell carries all its genetic material. On the other hand, coronaviruses are directly reaching parts of the host cell, called Ribosomes it not required to enter the host cell nucleus. Ribosomes use genetic information from viruses to make viral proteins. A packaging structure in the cell moves the spikes into the vesicles, which add with the cell membrane, the outer layer of the cell. All the fragments needed to make a new virus gathered under the membrane of the cell. Then a new virus formation starts from the cell membrane. Each lung has separate segments, called lobes.
Fig. 2. Structure of Lungs [11]
Normally, as breath, air moves freely through the trachea. the trachea has three main parts:- first large tube called bronchi, and second small tubes, called bronchioles and finally into tiny sacs, it is called alveoli. Airways and alveoli of the trachea are flexible and polymorphic. When the time of breathing, each air sac inflates like a small balloon and when releasing air, the sacs shrink. The alveoli are bounded by small blood vessels on all sides; the small blood vessels are called capillaries.
Every cell in your body requires oxygen to live. When we take breathe in the lungs, oxygen is carried into the bloodstream and carried through your body. At each cell of the body, oxygen is exchanged for a waste gas called carbon dioxide and then bloodstream carries this waste gas back to the lungs where it is removed from the bloodstream and then breath out from the body. Lungs and the respiratory system automatically perform this vital process, called gas exchange. The trachea contains mucus hold the most germs in mucus that pull trachea, bronchi and bronchioles. In a healthy body, the cilia tubes continuously emit mucus and germs from the trachea, that's by their reason cough. The cells of immune system attack viruses and germs that build it mucus and cilia and enter alveoli. In case if the immune system weak such as in the case of coronavirus infection, then the virus can affect immune cells and bronchiole and alveoli form, which causes your immune system to attack enlarging viruses. Due to the Infection, in alveoli gets fill fluids, making it difficult for the body to get the oxygen it requires. It may generate lobar pneumonia, where one lobe of the lung is affected, or may have bronchophenia that affects maximum areas of both lungs. Due to Pneumonia, uneasy to take breathing, cough, fever, Chest pain and coldness, headache, pain and fatigue. It may cause a lot of serious problems like Respiratory failure occurs when breathing becomes so tuff ventilator is required to help breathe. These machines are that save lives and all the medical equipment companies produce a large amount of upgrading equipment. In the current scenario Whether or not these symptoms will develop depends on many reasons, such as age, travel history and whether there is a pre-existing condition. There are several different approaches to a potential vaccine against coronavirus. All country are working hard for developing a vaccine. Till the absence of vaccine social distancing, cleanness and awareness are the very important factors to protect our life.
-
PROBLEM FORMULATION Coronaviruses are a large family of single-stranded RNA
viruses (+ssRNA) that can be isolated in different animal species. This virus has been identified as the coronavirus in the last few years. At the molecular level, coronaviruses employ a variety of unusual strategies to carry out a complex program of gene expression. The COVID-19 sign and symptoms usually take approximately 3-7 days after exposure sometimes it takes up to 14 days to appear. Manually classifying the COVID-19 from CT is very laborious and extremely hard. So a mechanized over strategy is worn to decrease the load on the operator and produces satisfactory outcomes for classified the COVID-19. The most common symptoms of COVID-19 disease are fever, dry cough, and tiredness. Other symptoms of the disease that are less common and may affect some patients include regular pains, nasal congestion, headache, conjunctivitis, sore throat, diarrhoea, loss of taste or smell or a rash on skin or discolouration of fingers or toes. Generally, these symptoms are light and begin gradually. for this reason, Some people become infected. Most people recover from the disease by primary treatment. But Around 1 out of every 5 people who suffer COVID-19 becomes seriously ill and that patient gets the difficulty of breathing. Old people, and those with underlying medical problems like high blood pressure, heart and lung problems, diabetes, or cancer, are at higher risk of developing a serious illness. However, everyone may be affected by COVID-19
and become seriously ill. People of all ages who experience fever and/or cough associated with difficulty breathing/shortness of breath, chest pain/pressure, or speaking problem should seek medical attention immediately. If possible, it i recommended to call the health care provider. Therefore the patient can be directed to the right clinic.
-
OBJECTIVE
Our purpose of this effort is to provide an automatic technique which locates the infection on CT image. Manual detection includes many deep learning algorithms. In the process, the human invention is regularly expected to initialize the technique to check the precision of outcome while in fully automatic decision method.
-
LITERATURE SURVEY
In current years, predictive classification is one of the very necessary, required and important tasks in deep learning. deep learning technology to the medical diagnosis has received a strong boost due to earnest research activities in the magical big data. several researchers have highlighted the potential of predictive classification to provide decision support for doctors and medical professional. Over the last few times, a great deal of research has been conducted on different data sets to predictive COVID-19.
Following is some of the researches which have been reviewed for the proposed system:–
TABLE I. COMPARISON VARIOUS METHODS
Literature
Metho d Used
Dataset
Result
Acc.
Sens.
Spec.
Jianpeng Zhang et al. [1]
ResNe t
1531 CT
Scans
–
96.0
%
70.7%
Xiaowei Xu et al. [2]
CNN
618 CT
Scans
86.7%
–
93.6%
Chen et al. [3]
UNet+
+
106 CT
Scans
95.2%
100%
93.6%
Cheng Jin et al. [4]
CNN
10250 CT
Scans
–
94.1
%
95.6%
Feng Shi et al. [5]
RF
2685 CT
Scans
87.9%
90.7
%
83.3%
Narin et al. [6]
ResNe t50
100 CT
Scans
98.0%
–
–
Lin Li et al. [7]
ResNe t 50
4356 CT
Scans
–
90.0
%
96.0%
Shuo Jin et al. [8]
UNet+
+
1136 CT
Scans
–
97.4
%
92.2%
Song Ying et al [9]
ResNe t 50
275 CT
Scans
86.0%
–
–
-
Zhang et al. [1] present a ResNet based model to detect COVID-19 from X-ray images. This model has two tasks, one task for the classification between COVID-19 and non-COVID-19, and another task for anomaly detection. The anomaly detection task gives an anomaly score to optimize the COVID-19 score used for the classification. X-ray images from 70 COVID-19 and 1431 non-COVID- 19 pneumonia patients X-ray images are included from these two datasets. The sensitivity and specificity are 96.0% and 70.7%, respectively, along with an AUC of 0.952.
-
Xiaowei Xu et al. [2] use 618 chest CT images from 219 patients with COVID-19, 224 patients with Influenza-A, and 175 healthy persons. A deep learning model based on V-Net is first used to segment out the candidate infection regions. The patches of infection regions are sent to the Resnet-18 network together with features of relative infection distance from the edge, and the output is one of these three groups. The model achieves an overall accuracy of 86.7%.
-
Chen et al. [3] employ chest CT images from 51 COVID- 19 patients and 55 patients with other diseases to train a UNet++ based segmentation model. This model is responsible for segmenting COVID-19 related lesions. The final label (COVID-19 or non-COVID-19) is determined based on the segmented lesions. The evaluation results of COVID-19 classification using the proposed model are 95.2% (Accuracy), 100% (Sensitivity), and 93.6% (Specificity). In an additional dataset including 16 viral pneumonia and 11 non- pneumonia patients, the proposed model could identify all the viral pneumonia patients and 9 of non-pneumonia patients. The reading time of radiologists is shortened by 65% with the help of AI results.
-
Cheng Jin et al. [4] use 10,250 chest CT images from 7,917 subjects. A 2D CNN based model is proposed to segment the lung and then identify slices of positive COVID-19 cases. Experimental results show that the proposed model achieves a sensitivity of 94.1%, a specificity of 95.5%, and AUC of 0.979.
-
Feng Shi et al. [5] employed chest CT images of 2685 patients, of which 1658 patients are of COVID-19 and 1027 patients are of common pneumonia. In the preprocessing stage, a VB-Net has adopted to segment the image into the left/right lung, 5 lung lobes, and 18 pulmonary segments. Many hand-crafted features are calculated and used to train a random forest model. Experimental results show a sensitivity of 90.7%, a specificity of 83.3%, and accuracy of 87.9%. Also, testing results are grouped based on infection sizes, showing that patients with small infections have low sensitivity to be identified.
-
Ali Narin et al. [6] propose three different deep learning models, i.e., ResNet50, InceptionV3, and Inception- ResNetV2, to detect COVID-19 infection from X-ray images. It is worth noting that the COVID-19 dataset and Kaggles Chest X-Ray Images (Pneumonia) are also used to form the dataset in this study. Chest X-ray images of 50 COVID-19 patients and 50 normal chest X-ray images are included. The evaluation results show that the ResNet50 model achieves the highest classification performance with 98.0% accuracy, compared to 97.0% accuracy by InceptionV3 and 87% accuracy by Inception- ResNetV2.
-
Lin Li et al. [7] used a large chest CT dataset, which contains 4356 chest CT images (i.e., 1296 COVID-19, 1735 community-acquired pneumonia, and 1325 non- pneumonia) from 3322 patients. A ResNet50 model (COVNet) is used on 2D slices with shared weights and combined with max-pooling to discriminate COVID-19 from community-acquired pneumonia (CAP) and non-
pneumonia. Results show a sensitivity of 90%, a specificity of 96%, and AUC of 0.96 in identifying COVID-19.
-
Shuo Jin et al. [8] include chest CT images of 1136 cases (i.e., 723 COVID-19 positives, and 413 COVID-19 negatives) in the study. Their proposed model contains a UNet++ based segmentation model and a ResNet50 based classification model. The segmentation model is used to highlight lesion regions for the classification model. In the experiment, the sensitivity and specificity using the proposed UNet++ and ResNet50 combined model are 97.4% and 92.2%, respectively.
-
Song Ying et al. [9] proposed a deep learning-based CT diagnosis system (called DeepPneumonia, using ResNet50) to detect patients with COVID-19 from bacteria pneumonia patients and healthy people. Chest CT images from 88 patients with COVID-19, 101 patients with bacterial pneumonia, and 86 healthy persons are used as training and testing data. Slices of complete lungs are derived from chest CT images, which are the inputs of DeepPneumonia. The model achieves promising results with an accuracy of 86.0% for pneumonia classification (COVID-19 or bacterial pneumonia), and an accuracy of 94.0% 8 for pneumonia diagnosis (COVID-19 or healthy).
-
-
FUTURE SCOPE
The proposed system can be developed in many different ways which have huge scope for improvement in the system. Some important roles are includes:-
-
For making efficient system it is necessary to improvising the algorithm. So continuously Increse the accuracy of the algorithm.
-
Some more attributes will use so to tackle COVID-19 even more.
-
It is compulsory to make an important and preventive health care diagnosis system to be used in research.
REFERENCES
-
Jianpeng Zhang et al. COVID-19 Screening on Chest X-ray Images Using Deep Learning based Anomaly Detection, arXiv:2003.12338, 2020
-
Xiaowei Xu et al. Deep Learning System to Screen Coronavirus Disease 2019 Pneumonia, arXiv:2002.09334, 2020
-
Chen et al. Deep learning-based model for detecting 2019 novel coronavirus pneumonia on 2 high-resolution computed tomography: a prospective study, https://doi.org/10.1101/2020.02.25.20021568, 2020
-
Cheng Jin et al. Development and evaluation of an AI system for COVID-19 diagnosis. https://doi.org/10.1101/2020.03.20.20039834, 2020
-
Feng Shi et al. Large-Scale Screening of COVID-19 from Community Acquired Pneumonia using Infection Size-Aware Classification, arXiv:2003.09860, 2020
-
Ali Narin et al. Automatic detection of coronavirus disease (COVID-
19) using X-ray images and deep convolutional neural networks, arXiv:2003.10849, 2020
-
Lin Li et al. Artificial Intelligence Distinguishes COVID-19 from Community Acquired Pneumonia on Chest CT, Radiology, p.200905, 2020
-
Shuo Jin et al. AI-assisted CT imaging analysis for COVID-19 screening: Building and deploying a medical AI system in four weeks, https://doi.org/10.1101/2020.03.19.20039354, 2020
-
Song Ying et al. Deep learning Enables Accurate Diagnosis of Novel Coronavirus (COVID-19) with CT images., https://doi.org/10.1101/2020.02.23.20026930, 2020
-
https://rebelem.com/wp-content/uploads/2020/03/COVID-19-
Structure.png
-
https://www.macmillan.org.uk/dfsmedia/1a6f23537f7f4519bb0cf14c45 b2a629/68-50036/macd086a-lung-close-up-labelled-20170118