
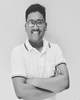
- Open Access
- Authors : Nitesh Kumar Sahoo
- Paper ID : IJERTV9IS060857
- Volume & Issue : Volume 09, Issue 06 (June 2020)
- Published (First Online): 01-07-2020
- ISSN (Online) : 2278-0181
- Publisher Name : IJERT
- License:
This work is licensed under a Creative Commons Attribution 4.0 International License
Efficiency Improvement by Reducing Rework and Rejection on the Shop Floor
Nitesh Kumar Sahoo1
1Masters of Fashion Technology,
National Institute of Fashion Technology, Gandhinagar, Jajpur, Odisha, India
Abstract: For any garment industry the production and quality management or wastages reductions have a major impingement on overall factory economy. In garment industry maximum quality constraints arise in the sewing department. The minimization of reworks in sewing department of any apparel industry for quality improvement plays a significant role on overall factory economy. This project discusses the quality improvement of garment industry by applying quality tools such as checklist, cause and effect diagram and Traffic Light System. The purpose of the implementation is to reduce the reworks rate with improved quality by eliminating loopholes of quality. This work provides the guidelines to control the rejection and reworks through reduction of defects in garment industry by identifying the root causes and traffic light system. The DHU percentage was compared with respect to the initial and final state and it was analysed that there was a fall of 47.6% in the DHU. The outcome of this observation depict that an industry may gain higher productivity and profitability, by reducing the costs and improving the quality constraints through minimization of rework activities.
Keywords: Efficiency, DHU, Rework, Traffic Light System, Quality and Quality Tools
-
INTRODUCTION
The study conducted in Bangalore base suit manufacturing company. There is always spontaneous change occurs in global economy, in an industry specific focus area is given for profit margin, consumer demand for quality produce and increased productivity. In garment manufacturing, after shipment there are only a few rejected pieces. According to many manufacturers garments are considered as soft goods and non-repairable damage occurred due to use of poor quality row materials or faulty manufacturing or casual behaviour of an employee. If a product defect is identified at the end line or in the final inspection the complete cost involved in the process wasted as the product cant export. Sometime manufacturer rework on the defective pieces to make it exportable but extra cost involved in that process also more resources are used which affect the profit of the company. During the process of manufacturing some defects arises in swing section which should be reduced to maintain quality of garment. The defects hamper the total quality of the factory, because of these defects the rework rate reaches greater than 7% (Approx.) based on the company daily rework rate which also reduces the productivity and efficiency of the sewing section. The shop floor must reduce the rework percentage to achieve maximum production efficiency and avoid non-productive activity. Rework and rejection affect the shipment time and quantity resulting buyer dissatisfaction, business loss.
Immediate action on the rework and rejection can save cost. Cost of poor quality (COPQ) will affect the manufacture with respect to profit, buyer dissatisfaction and lose business relationship.
-
OBJECTIVE
-
To ensure that the product has achieved the quality parameters of buyers.
-
To restrict the defects entering into the final product
-
To improve efficiency by reducing rework and rejection.
-
-
REVIEW OF LITERATURE According to Juran, 1999 Quality indicates features of products which meet customer needs and satisfaction. Quality is directly proportional to income. Providing more or better quality features usually requires an investment and hence increases in costs. Quality means freedom from deficienciesfreedom from errors that require doing work over again (rework) or that result in field failures, customer dissatisfaction, and customer claims, and so on. (Joseph M. Juran, 1999)[1] In this case, the meaning of quality is oriented to costs, and higher quality usually costs less. Philip B. Crosby revealed that Quality is free because the cost spends towards prevention will resulted with lower cost of detection, correction and failure. (Crosby, Quality Is Free, 1979)[2]. Production and material costs are the same for first quality products (those that meet the standards) as for sub-standard products (those that do not meet standards) manufacturer have same investment in a sub- standard product as in first quality product. Ishikawa Diagram-identifies many possible causes for an effect or problem, which can help us to structure a brainstorming session (Ishikawa, Guide to quality control, 1976)[3]. It immediately sorts ideas into useful categories. Used when identifying possible causes for a problem. The causes are usually divided into 6 main branches which are commonly referred to as the 6Ms of manufacturing efficiency Improvement.
According to Juran Trilogy defines quality Planning is the structured process for designing products and services that meet new breakthrough goals and ensure that customer needs are met. Quality Control can also be described as a process for meeting the established goals by evaluating and comparing actual performance and planned performance, and taking action on the difference. Quality Improvement: The process for creating breakthrough levels of
performance by eliminating wastes and defects to reduce the cost of poor quality (Joseph M. Juran, 1999)[1].
It is very essential to apply all seven QC tools for troubleshooting issues within production processes in the organizations (Neyestani, Seven Basic Tools of Quality Control: The Appropriate Techniques for Solving Quality Problems in the Organizations, March 2017). It is very essential for management to identify quality problems and solve that during production. Thus, the production processes can be affected and improved by multiple factors of this statistical QC tool [4]. Sandra Helena Published an article on Use of Quality Tools for Problem Analysis (FMEA and Ishikawa Diagram) in a Small Textile Business in which a research was carried out by analysing the quality tools being used in the textile factory [3]. FMEA and Ishwikawa diagram (quality tools) was used for the research in which the defects were categorized in accordance to its occurrence time (Sandra Helena da Silva de Santis J. P., January 2016)[5]. The cause of the problem was recognized and thus the corrective is action is taken for the same. In this research the effect of delays and defects were also studied using 6Ms (measurement, material, manpower, machine, method). Thus both the tools were used to formulate actions to solve the problem. Minimize the occurrences of rework, reduce rejection rate and eliminate time waste in a garment industry using six sigma tools and techniques. To achieve this target six sigma tools such as SIPOC diagram, Pareto analysis, brainstorming and fishbone diagram were used. As a result, reduction in standard minute value (SMV) is reduced; production rate and labour productivity is improved. The SMV has been reduced from 7.312 minutes to 6.571 minutes, labour productivity has been increased from 29.56 to 35.55 and finally line efficiency has been increased from 45.03 % to
48.66 % which increased the productivity of 31 pieces per day of 8 hours shift. The total defective percentage recorded was 5.66 % and it was reduced to 3 % by implementing the remedial actions. Thus reworks, rejection rate and waste of time was reduced ultimately thus improving productivity (Bharath S, Minimizing Reworks, Rejection Rate and Time Waste in a Textile Industry Using Sixsigma Tools, 2017) [6]. Using7 Quality Control tools and techniques are used to reduce rejections and defects of product. Most of the rejections and defects are occurred due to improper control of quality of product. So the best way to reduce rejection is 7 quality tools. The impact of implementation in this study Rejection of drill is 5.036% which is reduced by concentration on margin over size and grinding defects. Major seam defect was around 20% with main cause of improper surface finishing stitching which came down to approximately 8% , uncut thread came down to approximately 10% from 22% as earlier. Rejection level reduced from 37.17% to 16.3%. The major Cold shut defect was reduced by up to 50% i.e. from 12.3% to 6.6%. Defect had reduced from 48.8% in December to 36.4% in January & also reduces the inner track size variation from 12.2% in December to 1.5% in January (Shyam H. Bambharoliya H. R., 2015) [7]. Major Defects Position and Percentage in Sewing Lines of a Garments Factory with the Help of Pareto Chart, Cause Effect Diagram and Sigma
Level in this paper by using the quality tools for analysing and implementing defects on the sewing line. From Pareto Chart Analysis 7 major defects found which contains 78.94% of total defects, 9 major defects which contain 11.86% defect position area where 54.02% of major defects occur (Tarikul Islam, Analysis of Major Defects Position and Percentage in Sewing Lines of a Garments Factory with the Help of Pareto Chart, Cause Effect Diagram and Sigma Level, July 2017) [8].
5S (Sort, Set in order, Shine, Standardize, Sustain) and PDCA (Plan-Do-Check-Act) to identifying swing defect in a particular product and minimize the rework rate. Pareto analysis is performed to identify top defect positions from all the defects and seven are identified where 80% defects occur which should be the major concerning areas to minimize defects percentage. Use cause-effect diagram for finding the major causes for that defect and taking the corrective action for the causes identified in the cause- effect diagram also use 5S lean tool for minimizing defects and the PDCA cycle helps to plan for continuously improving the quality by removing defects (Md. Tahiduzzaman M. R., 2018) [9]. Implementation of traffic light system in a swing line resulted, quality improvement and calculated the cost of poor quality and saving. It will also help to improve the quality status, reduced the cost, improve the lead time and thus strengthen the supply chain performance without increasing the resource (Md. Mazharul Islama, 2013)[10]
Quality and productivity improvement by introducing a framework which will identify quantify and eliminate sources of variation in an operational process, will optimize the operational variables, and improve process performance with a well-executed control plan. The paper also includes the existing quality system being used in a garment industry and identified the defects occurring in different departments of the industry. The defects were categorized and accordingly a model is developed to give an optimal solution to all the defects (Md. Mazedul Islam A. M., 2013) [11]. Major issue in apparel manufacturing is defect occur due to improper maintenances, machine adjustment, improper needle and pressure foot adjustment by analysis the defects recommended several solution to prevent defects (Hashi, Different Types of Defects Identification and Controlling Method for Quality and Productivity Improvement, 2016) [12].DMAIC (define, measure, analyse, improve and control) methodology of six sigma in a selected garment factory to minimize the defect percentage. DMAIC methodology of six sigma is a problem solving method where six sigma tools are used to analyse the process data and finally the root causes behind the defects produce in the product are identified (C.M.L., 2014)[13]. Six sigma is a process of quality improvement of a final product. According to a study it indicates six sigma, as a more superior level of quality which will definitely implemented in those organizations that are devoted to business excellence after QMS certification. Cause and effect analysis of those defect to find root cause (Uddin S.M, 2014) [14].The continuous improvement process in a small area resulted improve the overall productivity, efficiency and quality of garment. Implement
Kaizen process, increased efficiency of production line from 45% to 60% with minimized sewing defects significantly (Taposh Kumar Kapuria M. R., 2017) [15].Lean Tools and techniques to increase the efficiency and productivity of an apparel sewing section. Analysis of operator psychology, motivate the workers as well as training should provide to work consciously and efficiently. Learned about the reasons for being low performers and what steps to take to avoid the condition. Operational processes standardize to maintain the quality and quantity. Resulting sewing line efficiency improved from 53.79% to 61.92% and productivity improved by 29% to 44% (Chowdhury, Increase the Efficiency and Productivity of Sewing Section through Low Performing Operators Improvement by using Eight Wastes of Lean Methodology
, 2018) [16]. Quality improvement of a garment factory by cause-effect diagram in which the main aim was to minimize the defects that will reduce rework and rejection rate. An application of pareto analysis and cause and effect diagram was done to detect and minimize the defect percentage in sewing section. The defect data has been collected for four months and Pareto Analysis was performed in which it was found that the top defect positions are identified where 78.56% defects occur. The top defect was identified as 71.40%. Thus, hierarchies of causes for individual defect types are organized and Cause- Effect Diagrams are constructed for those defect types (Tanvir Ahmed R. N., 2013)[17]. Minimization of rework swing section of garment manufacturing ensuring quality. However, 1% defective product for organization is considered 100% defect for a buyer (Ms.N.S. Patil M. , January 2017) [18]. Garment industry can implement TQM, modification of reward systems by making and implementing the pillars of TQM can improve the quality. By implementing TQM, defect percentage reduced to 47%, Repair/Rework percentage reduced to 36%, Reject/Scrap percentage reduced to 40%, overall quality improved by 5% and efficiency improved to 13%. Using the TQM (Total Quality Management) tools we can analyse the product quality and process and get significant improvement by reducing defect and rework (M. M.Rahman, 2011)[19].
-
METHODOLOGY
This study contains use of quality tools to minimize defects and rework on garment industry. This process includes various defects, various quality tools specially Pareto Analysis and Cause-Effect diagram. The first step of the project is to review the existing production and quality inspection process. The first hand data needed for this study was obtained from the production department and quality department of the company. The details about the types of defects are collected from by the last six month data available in the company. To find out about the various defects for on-going styles by collecting data with the help of end line checking formats and implement a in line audit formats to find out various defects of inline by checking the pieces thereby with the checker to evaluate the defective rates and DHU percentage.
Also analyse available last six month end line data to find various defects responsible for high DHU percentage. The data will be represented in Pareto charts where the lengths of the bars represent frequency of the defects which will be arranged with longest bars on the left and the shortest to the right. It is based on the 80-20 principle which means 80% problems are caused by 20% defects. Again further Pareto analysis is done to show the most dominant occurring defects out of the pool of top defects by cause and effect analysis.
Implement of traffic light system for effective inspection to reduce defect generation at source. Random inspection system is more ffective in controlling shop floor quality. Reduction of alteration rate as traffic light system will help identify the defect causing area and operator. Due to higher alteration rate it causes bottleneck and reduce the production efficiency. By implementing traffic light system minimize rework, tracking operator performance.
Through the Pareto analysis, Cause and effect analysis was used to find out the possible causes or the root causes for the major defects. The root causes were identified in the form of man, machine, material and method and corrective actions were proposed thereby. After that, solution was proposed including Traffic Light System, data was again collected for the last 30 days of the project period in order to compare the initial and final scenario.
-
DATA COLLECTION
-
Defects on End line of Sewing Floor
1200
1200
40.95%
40.95%
1000
1000
987
987
35.02%
35.02%
800
800
844
844
600
600
45.00%
40.00%
35.00%
30.00%
25.00%
45.00%
40.00%
35.00%
30.00%
25.00%
15.31%
15.31%
400
400
20.00%
15.00%
10.00%
5.00%
0.00%
20.00%
15.00%
10.00%
5.00%
0.00%
Final Inspection data of last six month was analysed and its found that the highest 40.95% of defects are due to sewing and 35.02% defects are due to seaming (Figure 1). Due to operator carelessness 15.31% of defects happen in the shop floor.
Sum of Number of Defects
Sum of Percentage of defects
Sum of Number of Defects
Sum of Percentage of defects
369
369
200
200
6.06%
6.06%
1.58% 1.08%
1.58% 1.08%
0
0
SEAMING SEWING
SEAMING SEWING
DEFECTS DEFECTS
DEFECTS DEFECTS
146 38 26
OPERATPR FABRIC STATIN REJECTED ERROR DEFECTS
146 38 26
OPERATPR FABRIC STATIN REJECTED ERROR DEFECTS
Figure 1 Defects by defects category Final inspection
-
Impact of DHU percentage on Production efficiency It was analysed that when the number of defective pieces increases the efficiency of the factory decrease. (Figure 2) In the month of January the efficiency was 43.42% where the DHU percentage is 6.13% and defective garment percentage is 5.76%, similarly, in the month of October the
50
50
44
44
60.00%
60.00%
efficiency was 26.83% where the DHU percentage is 7.66% and defective garment percentage is 6.87%
50.00%
40.00%
42.90% 8.57% 7.66%
37.72%
6.68%
43.42%
39.21%
6.32% 6.13%
10.00%
34
33
32
34
33
32
40
40
50.00%
50.00%
40.00%
40.00%
30
30
8.00%
26
26
30.00%
20.00%
10.00%
6.20%
30.16%
26.83%
6.00%
20.00%
20.00%
30.00%
30.00%
20
20
12.57%
12.57%
9.71% 9.43%
9.71% 9.43%
7.43 10.00%
7.43 10.00%
4.00%
10
9.14%
10
9.14%
%
%
Label
Reveres/size wrong/ Po Change
Label
Reveres/size wrong/ Po Change
CB seam Main Label/Size
ope/uneven label/washcare
label missing
CB seam Main Label/Size
ope/uneven label/washcare
label missing
2.00%
0
Collar Point up & D/I bartack
0.00%
0
Collar Point up & D/I bartack
0.00%
0.00%
Down
Down
miss/open
miss/open
August September October November December January
Efficiency DHU %
0.00%
Figure 2 Production efficiency and DHU Percentage
-
Primary Data
100
90
80
70
60
50
40
30
20
10
0
100
90
80
70
60
50
40
30
20
10
0
95
95
70.0%
60.0%
70.0%
60.0%
69
69
50.0%
50.0%
54
48 42
54
48 42
40.0%
40.0%
30.0%
30.0%
As there is no data collection done on the front, lining and assembly sewing section. A data collection sheet or checklist was developed and implemented on the sewing floor on the 1st February 2019.Primary Data collection for the Front, Lining and Assembly section.
No of Defects
% Defect
Cumulative % Defects
No of Defects
% Defect
Cumulative % Defects
19.
19.
14.3%
14.3%
20.0%
20.0%
11.2%
11.2%
10.0%
10.0%
8.7%10.0%
8.7%10.0%
0.0%
0.0%
Seam open/ miss Vent UP & Down OBW width Margin uneven Flap bartack
match uneven / Shape miss/finishing Out not good
Seam open/ miss Vent UP & Down OBW width Margin uneven Flap bartack
match uneven / Shape miss/finishing Out not good
Figure 3 Jacket front section Defects Feb 2019 Pareto Analysis
From the (Figure 3) Pareto chart it observed that 64% defects are due to the top five defects. Seam Open is most common defect with as much as 19.8% of total. Vent up & down is second most common defect with as much as 14.3% of total. OBW Pocket Shape is third most common defect with as much as 11.2% of total. Margin Uneven is fourth most common defect with as much as 10.0% of total. Flap Bar-tack miss/Finishing not good is fifth most common defect with as much as 6.67%.
No of Defects % Defect Cumulative % Defects
No of Defects % Defect Cumulative % Defects
Figure 4 Jacket Lining section Defects Feb 2019 Pareto Analysis
62
62
61
61
50.00%
50.00%
From the (Figure 4) Pareto chart it observed that 48.29% defects are due to the top five defects. Label Reveres/size wrong/ Po Change are most common defect with as much as 12.57% of total D/I bar-tack miss/open is second most common defect with as much as 9.71% of total. Label miss/reverse/size wrong/PO change is third most common defect with as much as 9.43% of total. Centre Back Seam Open/uneven is fourth most common defect with as much as 9.14% of total. Main Label/Size label/wash care label missing is fifth most common defect with as much as 7.43%.
100
70.00%
100
70.00%
84
80
75
71
60.00%
84
80
75
71
60.00%
60
40.00%
60
40.00%
40
30.00%
40
30.00%
14.33%
12.80%
20.00%
20
14.33%
12.80%
20.00%
20
0
Sleeve A/H pleat Lapel peak up & Sleeve gap
/ open down uneven
Front edge Vent pleat/ high piping & low
improper/bottom shape out
% Defect
0.00%
0
Sleeve A/H pleat Lapel peak up & Sleeve gap
/ open down uneven
Front edge Vent pleat/ high piping & low
improper/bottom shape out
% Defect
0.00%
12.12% 10.58% 10.41%
12.12% 10.58% 10.41%
10.00%
10.00%
No of Defects
Cumulative % Defects
No of Defects
Cumulative % Defects
Figure 5 Jacket Assembly section Defects (Feb 2019 Pareto Analysis
From the (Figure 5) Pareto chart it observed that 60.24% defects are due to the top five defects. Sleeve Aram Hole pleat open are most common defect with as much as 14.33% of total Lapel peak up & down is second most common defect with as much as 12.80% of total. Sleeve gap uneven is third most common defect with as much as 12.12% of total. Front edge piping improper/bottom shape out is fourth most comon defect with as much as 10.58% of total. Vent pleat/ high & low is fifth most common defect with as much as 10.41%.
50
35.00%
8.00%
50
35.00%
8.00%
0
0
No of Defects
% Defect
Cumulative % Defects
No of Defects
% Defect
Cumulative % Defects
Figure 6 Jacket End Line Defects (Feb 2019 Pareto Analysis
From the (Figure 6) Pareto chart it observed that 31.29% defects are due to the below five defects. Weaving are most common defect with as much as 7.01% of total. Sleeve kinari open is second most common defect with as much as 6.65% of total. Keyhole miss/open/uneven/broken is third most common defect with as much as 6.12% of total. Armhole Lining lock open/miss is fourth most common defect with as much as 5.76% of total. Vent up & down is fifth most common defect with as much as 5.76%.
-
-
RESULT AND DISCUSSION
This is the most difficult phase in any projects because all the ideas do not follow the theory strictly and hence multiple problems were faced while implementing Traffic Light system. Some of the problems are discussed below: Negligence of the workers and adapting to the new process took time. Explanations were not easily understood and there was a lot of confusion amongst them. The existing inspection system had to be transformed and individual demonstration had to be provided to the quality checkers which resulted in errors in the first week. The marking system was quite laborious and hectic. Hence, tried to maintain a new format for keeping a record of the regular data in bulk. Inspecting all the critical operations individually every hour was a tough task. A two weeks trial implementation was also done to find whether its effective or not.
Root-cause analysis for top defect in each sewing section also Traffic light system implementation resulting defects rate gradually decreased. The highest DHU% marked on the 2nd week of February which is 8.16% and after implementation the lowest DHU% recorded on 3rd week of March which is 2.01%. The average DHU % reduced from Moth of February to March is 60.1% at the front section of jacket line (Figure 7).
40
40
39
39
37
37
30.00%
30.00%
34
34
32
32
32
32
30
20
30
20
25.00%
20.00%
15.00%
25.00%
20.00%
15.00%
7.01%
7.01%
10
10
6.65%
6.65%
6.12%
6.12%
5.76%
5.76%
5.76%
5.76%
10.00%
5.00%
0.00%
10.00%
5.00%
0.00%
6.82%
6.82%
6.00%
4.00%
6.00%
4.00%
2.72%
2.72%
2.00%
0.00%
2.00%
0.00%
Feb
Feb
March
March
Figure 7 Front DHU% After & Before Implementation
Lining section (Figure 8) defect rate gradually decreased Figure 8. The highest DHU% marked on the 3rd week of February which is 5.25% and after implementation the lowest DHU% recorded on 4th week of March which is 2.52%. The average DHU % reduced from Month of February to March is 27.9%
5.00%
4.56%
5.00%
4.56%
4.00%
3.29%
4.00%
3.29%
3.00%
2.00%
1.00%
0.00%
3.00%
2.00%
1.00%
0.00%
February
March
February
March
Figure 8 Lining DHU% After & Before Implementation
10.00%
9.00%
8.00%
7.00%
6.00%
5.00%
4.00%
3.00%
2.00%
1.00%
0.00%
10.00%
9.00%
8.00%
7.00%
6.00%
5.00%
4.00%
3.00%
2.00%
1.00%
0.00%
8.81%
8.81%
3.80%
February
3.80%
February
The highest DHU% marked on the 2nd week of February which is 12.51% and after implementation the lowest DHU% recorded on 3rd week of March which is 2.79%. The average DHU % reduced from Month of February to March is 56.9% at the assembly section of the sewing line (Figure 9).
March
March
Figure 9 Assembly DHU% After & Before Implementation
(Figure 10) Final inspection rework rate gradually reduced. The highest DHU% marked on the 2nd week of February which is 8.68% and after implementation the lowest DHU% recorded on 4th week of March which is 2.8%. The
average DHU % reduced from Month of February to March is 47.6%
8.00%
7.28%
8.00%
7.28%
7.00%
6.00%
5.00%
4.00%
3.82%
7.00%
6.00%
5.00%
4.00%
3.82%
3.00%
2.00%
1.00%
0.00%
3.00%
2.00%
1.00%
0.00%
February
March
February
March
Figure 10 Final Inspection DHU% After & Before Implementation
After all the analysis, certain results were obtained. From all the above given figures its clear in the report:
49.00%
48.00%
47.00%
46.00%
45.00%
44.00%
43.00%
42.00%
41.00%
40.00%
39.00%
49.00%
48.00%
47.00%
46.00%
45.00%
44.00%
43.00%
42.00%
41.00%
40.00%
39.00%
8.00%
8.00%
7.28% 47.79%
7.28% 47.79%
7.00%
7.00%
6.13%
6.13%
6.00%
6.00%
5.00%
5.00%
43.42%
43.42%
3.71%
3.71%
4.00%
4.00%
42.77%
42.77%
3.00%
3.00%
After Implementation of traffic light system and Root- cause analysis of top defect of each section it has resulted in an increase in the production efficiency by 11.72%. Which was resulted manufactured around 92 more pieces due to reduction of rework (Figure 11).
2.00%
1.00%
0.00%
January
Efficiency
2.00%
1.00%
0.00%
January
Efficiency
February
February
March
March
DHU % Linear (Efficiency)
DHU % Linear (Efficiency)
Figure 11 Efficiency & DHU Before & After Implementation
-
Cost of Poor Quality
The Cost reduced as the quality improved. The cost of poor quality Percentage for the month January and February is 1.59% and 1.79% respectively after implementation of traffic light system the cost of poor quality percentage for the month March and April is 1.09% and 1.04%.Hence the cost of poor quality reduced by 31.5% comparing to month of January and March. (Figure 12)
2.00%
1.79%
2.00%
1.79%
1.50%
1.59%
1.04%
1.50%
1.59%
1.04%
1.00%
1.09%
1.00%
1.09%
0.50%
0.00%
0.50%
0.00%
0
1
2
3
4
5
0
1
2
3
4
5
Figure 12 Cost of poor quality in Percentage
-
-
CONCLUSION:
Quality is the most important for customer satisfaction in apparel manufacturing. Higher rework rate in garment manufacturing one of the major loss or profit minimization. Manufacturer considerable concern should be to manufacture and deliver quality product right at the first time. Although defect in garment manufacturing generally lead to rework rejection, time consuming, and customer or buyer disappointment. The Check sheet was used to capture the defects in the sewing section. Pareto Analysis was performed to identify the top defects types in the Front, lining, Assembly and Final inspection of Jacket. Those identified top defects are identified by using the Cause-Effect Diagram. Implementation of traffic Light system which is effective inspection tool to reduce. The total defect percentage was 7.28 % and it reduced to 3.82 % and efficiency improved 11.72% by implementing the quality tools. Thus efficent minimize reworks, rejection rate and waste of time that will ultimately improves productivity and efficiency.
REFERENCES
-
Joseph M. Juran, A. B. (1999). JURANS QUALITY HANDBOOK. McGraw-Hill.
-
Crosby, P. B. (1979). Quality Is Free. McGraw-Hill.
-
Ishikawa, K. (1976). Guide to quality control. Tokyo : Asian Productivity Organization.
-
Neyestani, B. ( March 2017). Seven Basic Tools of Quality Control: The Appropriate Techniques for Solving Quality Problems in the Organizations. ResearchGate.
-
Sandra Helena da Silva de Santis, J. P. (2016). Use of Quality Tools for Problem Analysis (FMEA and Ishikawa Diagram) in
-
Journal of Textile Science & Engineering.
-
-
Bharath S, A. V. (2017). Minimizing Reworks, Rejection Rate and Time Waste in a Textile Industry Using Sixsigma Tools. Scholars Journal of Engineering and Technology , 70-75.
-
Shyam H. Bambharoliya, H. R. (2015). Reducing Rejection Rate in Small Scale Machining Unit Using 7 Quality Control Tools – A Review. International Journal of Engineering Development and Research , 582-586.
-
Tarikul Islam, S. A. (2017). Analysis of Major Defects Position and Percentage in Sewing Lines of a Garments Factory with the Help of Pareto Chart, Cause Effect Diagram and Sigma Level. International Journal of Scientific & Engineering Research, 8(7), 1885-1890.
-
Md. Tahiduzzaman, M. R. (2018). Minimization of Sewing Defects of an Apparel Industry in Bangladesh with 5S & PDCA. American Journal of Industrial Engineering, 17-24.
-
Md. Mazharul Islam, M. S. (2013). Enhancing lean supply chain through traffic light quality management system . Management Science Letters , 3, 867-878.
-
Md. Mazedul Islam, A. M. (2013). MINIMIZATION OF REWORKS IN QUALITY AND PRODUCTIVITY IMPROVEMENT IN THE APPAREL INDUSTRY. International Journal of Engineering and Applied Sciences, 147-164.
-
Hashi, M. R. (2016). Different Types of Defects Identification and Controlling Method. IOSR Journal of Polymer and Textile Engineering, 01-18
-
C.M.L., U. S. (2014). Minimization of Defects in the Sewing Section of a Garment Factory through DMAIC Methodology of Six Sigma . Research Journal of Engineering Sciences, 21- 26.
-
Uddin S.M, R. C. (2014). Minimization of Defects in the Sewing Section of a Garment Factory through DMAIC Methodology of Six Sigma. Research Journal of Engineering Sciences, 21-26.
-
Taposh Kumar Kapuria, M. R. (2017). Root Cause Analysis and Productivity Improvement of An Apparel Industry in Bangladesh Through Kaizen Implementation. JARIE, 227-239.
-
Chowdhury, S. A. (2018). Increase the Efficiency and Productivity of Sewing Section through Low Performing Operators Improvement by using Eight Wastes of Lean Methodology . Global Journal of Researches in Engineering, 43-60.
-
Tanvir Ahmed, R. N. (2013). An Application of Pareto Analysis and Cause-Effect Diagram for Minimizing Defect Percentage in Sewing Section of a Garment Factory in Bangladesh. International Journal of Modern Engineering Research, 3700-3715
-
Ms.N.S. Patil, M. (January 2017). Minimization of Defects in Garment during Stitching. International Journal on Textile Engineering and Processes, 24-29.
-
Masud, M. M. (2011). QUALITY IMPROVEMENT IN GARMENTS INDUSTRY THROUGH TQM APPROACH . International Conference on Mechanical Engineering , 1-7..
It is very useful