
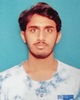
- Open Access
- Authors : Prajwal S , Shahid Afridi , Patel Sana Riyaj , Srihari Hegde G. K , Aditya C. R.
- Paper ID : IJERTV9IS070601
- Volume & Issue : Volume 09, Issue 07 (July 2020)
- Published (First Online): 30-07-2020
- ISSN (Online) : 2278-0181
- Publisher Name : IJERT
- License:
This work is licensed under a Creative Commons Attribution 4.0 International License
Personality and Traits score Prediction from Social Media for Students
Prajwal S., Shahid Afridi, Patel Sana Riyaj, Srihari Hegde G.K., Aditya C.R.
Vidyavardhaka College of Engineering
Abstract: Individual Personality can be predicted by using Online Social Networks. The Predicted personality finds its application in various fields. This paper proposes a system to predict the personality scores of the student without having to go through any personality analysis or taking any personality test. The results obtained clearly indicate that machine learning models can be effectively used for students personality prediction from Big-5 Traits.
-
INTRODUCTION
Social media has become one of the important platforms for social interactions. Social networking sites (SNS) make it easy to interact with people through social media. Another boon of using social media is to create, share as well as exchange information. There is abundant information available as we scroll through the timeline. Facebook, Twitter, Instagram are some of the examples of social media sites. Facebook is to be treated as one of the biggest used sites for human interaction, as we can build new relationships and safeguard the existing ones. Building new relationships is one of the biggest challenges as one personality interact with other new personality [3].
Personality is one of the important characteristic features. Personality can be predicted by using Online Social Networks (OSNs). The Predicted personality finds its application in various fields. One such field is academics. In this paper, we try to use student generated information on social network (Facebook), which is easy to get and predict students personality. We gather public data based on their Facebook profiles. The personality of a person predicts about the behaviour, weakness, activeness, the response made in certain situation [3][1]. This information can be used to have a better education planning for a particular student within the institution, which helps to improve the academic performance by fully utilizing the talent of the student.
-
PERSONALITY MODELS
The PEN model
PEN Model [4] is based on figure investigation. The factors for PEN model is Extraversion and Neuroticism. These super components are composed of calculate investigations of lower-order components. It incorporates friendliness and positive influence (components of Extraversion). These properties comprises of factor analysis of lower-order behaviour such as working together as a group in a total particular assignment.
PEN Model [4] is based on figure investigation. The factors for PEN model is Extraversion and Neuroticism. These super components are composed of calculate investigations of lower-order components. It incorporates friendliness and positive influence (components of Extraversion). These properties comprises of factor analysis of lower-order behaviour such as working together as a group in a total particular assignment.
With high score in neuroticism is constrained more towards tension, discouragement, self-question and other negative emotions. The Person will have an enthusiastic reaction to the occasions that would not influence the vast majority. Here the individual is increasingly inclined to state of mind issue, depression, hesitance, and anxiety. Psychoticism is described as the character type that is slanted to put it all on the line, participate in against social practices and tactlessness. This characteristic is generally in close relationship with the traits of un-empathetic, contemplative and ill will practices. [23] Previous works on character conjecture with PEN model, using the dataset from the site of Workshop from the Computational Personality Recognition, has demonstrated that male individuals inclined more towards extraversion sentences than female and as separation, the female respondents assessed to neuroticism sentences than that of male individuals. Regardless, the female tends to some degree higher to the psychoticism words than that of male respondents [23]. Be that as it may, this methodology was to recognize character of clients dependent on general recognitions from Malaysians point of view.
Myers Briggs Type Indicator (MBTI)
Myers Briggs Type Indicator (MBTI) [5] is a technique by which testing is done to indicate the personality of an individual based on ability to make decisions. This test is mainly used during the recruitment of people into job or choosing career path based on ones personality.
The BIG-FIVE Model
Big-5 model popularly known as OCEAN model [6] [2] consists of Openness, Conscientiousness, Extroversion, Agreeableness, and Neuroticism. Openness consists of six concepts, or a scale, counting consistent creative ability, tasteful affectability, thoughtfulness to inward sentiments, inclinations for assortment, and mental interest. Conscientiousness deduces on ache for to do a task well, and to expect responsibilities to others genuinely. Extroversion demonstrates how active and social a person is. Agreeableness is personality trademark showcased in persons social behaviour that are seen as mindful, pleasing, warm and valiant. People having high score in neuroticism encounter sentiments such as uneasiness, stress, fear, outrage, dissatisfaction, envy, blame, discourage temperament and depression.
The Big-5 model is one of the most studied models and many researches have proved that irrespective of any language, test or the method of analysis the validity of the model does not change [13] [14] [15] [16]. So Big5 is
considered as the current definitive model of personality [14].
Conscientious people tend to pay more attention to detail and are very efficient and well organized and show self- discipline, and motivate for achieving aim. The people with more conscientiousness tend to finish assignments and projects in advance, enjoy setting plans and be attentive and more specific. The people with less conscientiousness tend to be less organized, unlike to schedule plan. Agreeableness is considered to be subordinate trait that combines group of personality that cluster together statistically. This trait shows itself in individual behaviour, for example, helpful, warm and social congruity. More agreeableness people tend to be more naturally altruistic, have more concern for their community, and make their comfort easily. They are more likely to be patient with others.
-
BACKGROUND AND RELATED WORK Despite using traditional method of questionnaire to find the psychometrics and personality trait values, semantic and textual data of the user on social media has been proven to be reliable. It is more advanced and also effective and efficient in terms of the dataset. With the evolution of social media in recent times, the strong bond of writing styles and personalities acts as a revealing factor of characteristics of the user.
Oberlander and Nowson [21] has done research to differentiate the personality of weblog authors using text, by considering the data of report from the volunteers. They studied the machine learning arrangements on Big 5 attributes and said that few models work superior to the gauge. Since the work of Argamon et al., includes the study of personality of individuals from different viewpoints of the linguistics features [22,7], differentiating based on structure [8] and based on different machine learning algorithms [8,9]. There have been several studies based on different social media platforms.
Chirs Summer et al. [10] concentrate on Twitter clients predominantly centred around Dark Trait, for example, narcssism, Machiavellianism and psychopathy and furthermore the connection with Twitter action, Dark Trait and Big 5 Personality attributes. This examination has demonstrated that the publicly supported calculations were very flawed in foreseeing a person's Dark Trait from Twitter movement yet the model was effective when applied to huge gathering of individuals. This study helped to see whether the Dark Traits are increasing or decreasing over a population.
Sorayahakimi et al. [20] considered the associations between character characteristics and understudy's scholarly accomplishment and it was discovered that these qualities were firmly identified with scholastic accomplishment. The academic behaviour corresponding to the individual trait was studied. Regression analysis showed that personality traits were about in 48 percent of variance in academic achievement. Also, it showed academic achievement doesnt come into picture in case
of gender. Finally, the conclusion that conscientiousness was an important aspect of academic achievement was drawn.
The main focus here is the Facebook dataset and particularly the Facebook statuses of the students. In most of the research studies, dataset is built using forms collected by the users on filling the surveys offline. Lampe et al. [11], Nosko et al. and De Brabander and Boone [12] work showed us that, while college students react most noteworthy in the account things (59%), an example including college and non-college clients just complete 25% of the data required in the profile. Lampe et al. [11] proposed a model dependent on the quantity of gatherings and the absolute number of client's profile. This connection is greater with reference information than others subtleties of low significance, at that point comes contact and finally ideal information interests and side interests. Lo Coco et al. [13] have introduced a homogeneous order for character attributes of a client's Facebook profile. This grouping assesses analysed standards dependent on Facebook utilization, social and character qualities of social associations.
-
METHODOLOGY
The reason for this research is to create a method to predict the trait scores of the students using their Facebook statuses. For training the models for personality prediction, we used Random Forest algorithm. Since there are five traits, totally 5 models were trained. To train the models, vectorization for each of the statuses across the features was done. The Random Forest algorithm has been considered as the most precise prediction method for classification and regression [17]. Also, the Random Forest Algorithm can handle large databases efficiently and it is non-sensitive towards noise and overfitting [18]. For testing the accuracy of trained Random Forest models, the textual statuses from the students Facebook accounts of the selected students was used. Also, students were also made to fill a personality questionnaire and the actual values of those students personality information were collected using IPIP 50-item Big Five factor makers. This was proposed by Gold Berg [19]. The inventory contains 50 question and the answer of each question can be Strongly Disagree-1, Disagree-2, Neither Agree nor Disagree-3, Agree-4, Strongly Agree-5. The number indicates score of each study shown that the Goldbergs IPIP 50-factor Big Five factor makers is fairly accurate with only minor deviations [19]. In order to have a better accuracy, more than 10 statuses of each student were scraped. These statuses were then stored in a database. Now by using the trained models, personality prediction of each status is done and later the predictions across all the statuses are averaged to get personality prediction of each student. Now the student is allowed take the personality test which consists of a questionnaire based on Goldbergs model. The corresponding score is stored in the database.
After finding the scores for both the data (Facebook statuses and from questionnaire), a compare function is used to compare them and see how accurate the predicted
values from trained model are to that of Goldbergs IPIP Big five factor makers.
-
RESULT AND DISCUSSION
This Random Forest models trained on Big-5 Traits were tested on the textual statuses extracted from the students Facebook accounts of the selected students. The predicted scores were compared with the scores generated through IPIP 50-item Big Five factor makers from the answers to questionnaire. Then the percentage difference was taken between the scores generated by Facebook statuses and that of questionnaire.
The Tables 1 and 2 show the results of personality prediction for sample two students. The differences of individual traits for first student are 10,17.2,17.14,8.16 and 9.09(in %). And that of the second student are 14.54,18.18,16.66,7.5 and 8.33. Similar results were found for the remaining students selected for testing the models.
Traits
Facebook results
Question naire results
Difference (%)
Openness
20
18
10.00
Conscientiousness
24
29
17.2
Extraversion
29
35
17.14
Agreeableness
49
45
8.16
Neuroticism
55
50
9.09
Traits
Facebook results
Question naire results
Difference (%)
Openness
20
18
10.00
Conscientiousness
24
29
17.2
Extraversion
29
35
17.14
Agreeableness
49
45
8.16
Neuroticism
55
50
9.09
Table 1
Traits
Facebook results
Questionnaire results
Difference (%)
Openness
58
50
14.54
Conscientiousness
18
22
18.18
Extraversion
24
20
16.66
Agreeableness
40
37
7.5
Neuroticism
60
55
8.33
Traits
Facebook results
Questionnaire results
Difference (%)
Openness
58
50
14.54
Conscientiousness
18
22
18.18
Extraversion
24
20
16.66
Agreeableness
40
37
7.5
Neuroticism
60
55
8.33
Table 2
Finally, the average of percentage differences among all the 100 students was taken as shown in Table 3. The differences are in the range of 5-20% which clearly indicates that using machine learning models can be effectively used for personality prediction from Big-5 Traits.
Table 3
Personality Traits
Average Difference among 100 students
Openness
12.27
Conscientiousness
17.7
Extraversion
16.9/p>
Agreeableness
7.83
Neuroticism
8.71
As the number of statuses used to generate each students data was increased the difference was considerably reduced. It can also be said that if a student is more vocal on social media it becomes fairly simple to predict the personality trait scores without examining the student into any sort of
personality test. The predicted results can be used by the educational institutions to concentrate on the performance enhancement of students and w to utilize each students strength to the full extent.
-
CONCLUSIONS
Big-5 is considered as the most suitable and accurate model of personality. The statuses of students in social media can be scrapped to generate the Personality Traits for training machine learning models. Questionnaire consisting of IPIP 50-item Big Five factor makers can be considered to validate the traits prediction accuracy of machine learning techniques. The results obtained clearly indicate that machine learning models can be effectively used for students personality prediction from Big-5 Traits.
REFFERENCES
-
Tadesse, Michael M., Hongfei Lin, Bo Xu and Liang Yang. Personality Predictions Based on User Behavior on the Facebook Social Media Platform. In publication IEEE Access 6 (2018): 61959-61969.
-
Wan D., Zhang C., Wu M., An Z. (2014) Personality Prediction Based on All Characters of User Social Media Information. In: Huang H., Liu T., Zhang HP., Tang J. (eds) Social Media Processing. SMP 2014. Communications in Computer and Information Science,in vol 489. of Springer,
Berlin, Heidelberg
-
Souri, A., Hosseinpour, S. & Rahmani, A.M. Personality classification based on profiles of social networks users and the five-factor model of personality. In publication Hum. Cent. Comput. Inf. Sci. 8, 24 (2018). at https://doi.org/10.1186/s13673- 018-0147-4
-
K Jang – Contribution of Eysenck's PEN Model, 1998 Eysenck's PEN model: Its contribution to personality psychology
-
P. B. Kollipara, L. Regalla, G. Ghosh and N. Kasturi, "Selecting Project Team Members through MBTI Method: An Investigation with Homophily and Behavioural Analysis," 2019 Second International Conference on Advanced Computational and Communication Paradigms (ICACCP), Gangtok, India, 2019, pp. 1-9, doi: 10.1109/ICACCP.2019.8883022.
-
Goldberg, L. R. (1993). The structure of phenotypic personality traits. American Psychologist, 48(1), 26
34. https://doi.org/10.1037/0003-066X.48.1.26
-
Golbeck, Jennifer & Turner, Karen. (2011). Predicting personality with social media. Conference on Human Factors in Computing Systems – Proceedings. 253-262. 10.1145/1979742.1979614.
-
Iacobelli, F., & Culotta, A. (2013). Too Neurotic, Not Too Friendly: Structured Personality Classification on Textual Data. ICWSM 2013.
-
Schwartz HA, Eichstaedt JC, Kern ML, Dziurzynski L, Ramones SM, Agrawal M, et al. (2013) Personality, Gender, and Age in the Language of Social Media: The Open-Vocabulary Approach. PLoS ONE 8(9): e73791. https://doi.org/10.1371/journal.pone.0073791
-
C. Sumner, A. Byers, R. Boochever and G. J. Park, "Predicting Dark Triad Personality Traits from Twitter Usage and a Linguistic Analysis of Tweets," 2012 11th International Conference on Machine Learning and Applications, Boca Raton, FL, 2012, pp. 386-393, doi: 10.1109/ICMLA.2012.218.
-
Cliff A.C. Lampe, Nicole Ellison, and Charles Steinfield. 2007. A familiar face(book): profile elements as signals in an online social network. In Proceedings of the SIGCHI Conference on Human Factors in Computing Systems (CHI 07). Association for Computing Machinery, New York, NY, USA, 435444. DOI: https://doi.org/10.1145/1240624.1240695
-
De Brabander B, Boone C. Sex differences in perceived locus of control. J Soc Psychol. 1990;130(2):271. doi:10.1080/00224545.1990.9924580
-
Lo Coco, G., Maiorana, A., Mirisola, A., Salerno, L., Boca, S., & Profita, G. (2018). Empirically-derived subgroups of Facebook users and their association with personality characteristics: A Latent Class Analysis. COMPUTERS IN HUMAN BEHAVIOR, 86, 190-198.
-
Digman, John. (2003). Personality Structure: Emergence of the Five-Factor Model. Annual Review of Psychology. 41. 417-440. 10.1146/annurev.ps.41.020190.002221.
-
Golbeck, Jennifer, Cristina Robles and Karen Turner. Predicting personality with social media. CHI EA '11 (2011).
-
John, O.P. (1990) The Big Five factor taxonomy: Dimensions of personality in the natural language and in questionnaires. In: Pervin, L.A., Ed., Handbook of Personality: Theory and Research, Guilford Press, New York, 1990, 66-100.
-
Wang, L., Zhou, X., Zhu, X., Dong, Z., & Guo, W. (2016). Estimation of biomass in wheat using random forest regression algorithm and remote sensing data. Crop Journal, 4, 212-219.
-
Jin X-l, Diao W-y, Xiao C-h, Wang F-y, Chen B, Wang K-r, et al. (2013) Estimation of Wheat Agronomic Parameters using New Spectral Indices. PLoS ONE 8(8): e72736. https://doi.org/10.1371/journal.pone.0072736
-
Gow, A. J., Whiteman, M. C., Pattie, A., & Deary, I. J. (2005). Goldberg's 'IPIP' Big-Five factor markers: internal consistency and concurrent validation in Scotland. Personality and Individual Differences, 39(2), 317-329.
https://doi.org/10.1016/j.paid.2005.01.011
-
Hakimi, S., Hejazi, E., & Lavasani, M. (2011). The Relationships Between Personality Traits and Students Academic Achievement. Procedia – Social and Behavioral Sciences, 29. doi: 10.1016/j.sbspro.2011.11.312.
-
Nowson, Scott & Oberlander, Jon. (2006). The Identity of Bloggers: Openness and Gender in Personal Weblogs. 163-167.
-
Argamon, Shlomo & S, Dhawle & Koppel, Moshe & Pennebaker, James. (2005). Lexical Predictors of Personality Type.
-
Saravanan Sagadevana, Nurul Hashimah Ahamed Hassain Malima and Mohd Heikal Husina, Sentiment Valences for Automatic Personality Detection of Online Social Networks Users using Three Factor Modelat procedia computer science .