
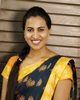
- Open Access
- Authors : Bharathi R , Yuvaraja M S , Pooja M V , Sahana B T, Shailaja T
- Paper ID : IJERTV9IS080030
- Volume & Issue : Volume 09, Issue 08 (August 2020)
- Published (First Online): 07-08-2020
- ISSN (Online) : 2278-0181
- Publisher Name : IJERT
- License:
This work is licensed under a Creative Commons Attribution 4.0 International License
Leveraging Product Characteristics for Online Collusive Detection in Big Data Transactions
Bharathi R1
, Assistant Professor , Department of Electronics & Communication
Engineering
BGS Institute of Technology, BG Nagara Mandya, Karnataka, India
Yuvaraja M S2, Pooja M V3, Sahana B T4, Shailaja T5
Department of Electronics and Communication Engineering
BGS Institute of Technology, BG Nagara Mandya, Karnataka, India
Abstract: It has been a big concern for e-business platform in online fraud transaction. The reputation scores supplied by the platform will be always evaluated by e-commerce users, as developed by big data technology. High reputations always bring high profit to sellers is the reason why the seller prefer chasing high reputation scores supplied by the platform. The fraud by acquiring high reputation scores forms a collusion which will attract more potential buyers. It is a crucial task to recognize the fake reputation information by the e-commerce website. This continues and growing problems is tried to be solved by e-commerce platforms by adopting data mining techniques. The big data plays a crucial role in economic society, with highly developed Internet of Things. The economic growth is done in different domains using Big data. By analyzing operational data, the management and decision-making ability in e-business supplies support . The online commerce, provide users with a fair and healthy reputation system, also improves the shopping experience using big data technology, The main aims of this paper is to include individual- and transaction-related indicators by putting forward a conceptual framework which extract the characteristics of fraud transaction. Product type and product nature is the two product features. The accuracy of fraud detection is obviously enhanced by this two features. To verify the effectiveness of the indicators in the detection model a real-world dataset is used, which puts forward to recognize the fraud transactions from the legitimate ones.
Keywords: E-Business, FraudiDetection, Reputation System, SNA, K core.
-
INTRODUCTION
By the usage of the internet of Things a huge amount of data is generated. The relevant data Such as data mining and machine learning is adopted by online commerce to get valuable business information by big data technology. By taking advantage of IOT and big data management and healthy shopping platform environment is created and also it boosts the sales.By the support of big data technology and high efficiency and low cost of internet of things the popularity of online shopping has been promoted. The Chinese Internet Network Information Center (CNNIC) shows that China has 772 million online users, which was established in 2017 by China Internet development
statistic and report was published on 2018. Recently, with 500 million transactions and 10 billion dollars Taobao platform has more than million fraudulent sellers per year.
Most of the online business websites to solve this problem offers the recommendation system or credit information system to assist potential buyers in distinguishing legitimate shopper from fraudsters. To prevent fake transactions most of online shopping platforms such as JD, Dingdong, Yelp and Taobao, uses reputation system. Reputation system has been essential to e-business environment. The goods need to be delivered, after the buyer pays online, Due to the geographically distance. It makes the risk higher. There have been some reputation systems on historical transaction, that show users reviews. These systems play crucial roles in online transactions. When their transaction is completed some reputation systems ask both sides of a trade to give rating score to counterparty. Current reputation systems may sum or average the gathered ratting. The system just simply keeps a final score by using the positive scores minus the negative scores In this way, Taobao platform uses the sum of ratting. Normally, Potential buyers are more likely to shop with the sellers who have high reputation seem more reliable.
In online market there are significant amounts of fraud transactions. From the big data generated by IOT Platform can collect the relevant mass data and acquire valuable information to improve service and profits. The high reputations always bring high profit to sellers so sellers prefer chasing high reputation scores. A significant amount of fraud activities led the temptation of economic gains and the difficulty of internet supervision. Reputation systems always be attacked by illegal organization and cannot perfectly reflect the traders reputation. The detection of the inflated reputation fraud has been an important task for online shopping platforms, Since online buyers rely on the reputation system to evaluate the sellers.
Based on the valuable information acquired by IOT and big data the online collusive detection is necessary to find out illegal users. However, collusion between users though undermines the reliability of reputation systems has very limited attention has been
put. Systematic anti-fraud solutions are scarce in some way, so Limited resource has been put in the anti-fraud field. By solving fraud transactions Taobao shows great interest. Some studies have been made on auction fraud, such as the fraud types, motivations behind fraud, the influence of bogus websites, Chae etc. Different methods have been put forward, including graph mining methods, decision trees, regression models, model checking, statistical methods, the classical classification methods of neural networks, clustering, neighbor diversity, a detailed overview of the methods for fraud detection.
There are three aspects in our studys contributions: (1) We are introducing two new features of fraud transaction and combine them with other user characters for fraud recognition. Including the product type and product nature etc, many aspects of online transaction behavior have been extracted. While developing detection models it should be useful to other types of online collusion. Including money laundering, tax evasion, smuggling and drug trafficking, the way of acquiring the indicators could be
will significantly enhance the reputation of the collusive sellers. Collusive transaction that misleads benign purchasers could be treated as deception.
-
HOMO ECONOMICUS PRECONDITION
AS the fundamental assumption of neo-classical economics,Homo economicus treats people as a self- serving and opporunistic individual. Sociologists do not affirm the pure economic man assumption and they intended to reconcile homo economicus with homo sociologicus assumption [53]. However, Neo-classical economist, Becker [7], suggested that offenders also make rational decisions. Most scholars insist that the homo economicus paradigm is crucial for survivaland success in terms of some aspects of life [36]. So, when theadvantage of their crime outweighs the disadvantage, criminals, as individuals who willingly commit crime, do fraudtransactions.
In light of the context, Fig. 1 shows the seven hypotheses based on two types of variables.
Control Variables
generalized to other collusion behavior detection. (2) The real-world dataset is used to verify the practical of our detection model. (3) For protecting online reputation environment we give some implications to platform
Activenes
Product
iValue
Likelihood iof i ollu io
Research
Product
c s n
c s n
policy in online e-commerce.
-
-
EXISTING SYSTEM
Big data plays a crucial role in economic society, ith the high development of the Internet of things. Big data increases the economic growth in different domains. By analyzing operational data, the management and decision-making ability in e-business supplies support. The online commerce, provide users with a fair and healthy reputation system, also improves the shopping experience using big data technology. It has been difficult to be identified the accurate identity of the e-commerce participant, as the online shopping environment features is virtual. Buyers always not feel easy to get desired product quality due to asymmetric information.
-
PROPOSED METHOD
-
HYPOTHESES
In our study, fraud transaction aiming to inflate reputation refers to the illegal transaction that many illegal communities are undertaking for benefit. The term puppet buyers refer to the ID registered by collusion gang with the aim of accomplishing fraud transactions with sellers who paid for inflated reputation. Collusive seller refers to the fraudster who tries to obtain high credit score by illegal way. The usual way to inflate reputation is illustrated in Fig. 1. Illegal organization will recruit so many puppet buyers to fulfill multiple collusion transactions with fake delivering of products, after the collusive sellers pay for the service. As a result, positive ratings and comments which were evaluated by these puppet buyers
Seller Reputation Product
Detail
Figure 1: Seven hypotheses in the research model
In our study, collusive transaction for improvingcredibility are considered as a crime in the online shopping environment [74]. This study attempts to make use of the characters of fake transactions according to the reason behind the economics of crime. It assumes that all crimes make sensible decision. We put forward seven hypotheses based on the homo economicus precondition by analyzing cost and gaining of fraud transactions.
-
COST OF FRAUD TRANSACTIONS
There are two types of costs should be taken into consideration as a fraudster. One is the economic costs and the otherone is behavioral costs. Behavioral costs refer to what thecollusion organization should pay to finish the order bookedby sellers. The acts contain instrumental acts and goal actsas proposed by Verhallen [69]. Goal acts will bring aboutexpected benefits, while instrumental acts are conducted withthe aim to achieve the goal. Registration helps to generatepuppet buyer in the online business environment. All thebehaviors conducted to implement those processes in a faketrading can be treated as goal acts, including the selection ofproducts, payments, giving rating level and writing commentsand advices.
Normally, it is simple for the buyer to register a new useraccount. As we known, only a valid telephone
number isasked to acquire a user identity. Users then should inputvalidation code that is sent to their registered phone numberswhen setting up an account. So, the cost is relatively low.Nevertheless, a fair amount of time and efforts are requiredto develop computer programs or hire people to register andMaintain many phone accounts. The best way to save cost is to make as many orders as possible with multiple sellers. There is a feature of fraudsters; Activeness was also mentioned by Blume et al. (2006). Therefore, first hypothesis is presented below:
Hypothesis 1:(The Fraud Users Liveness Hypothesis):Fraudsters are involved in more transactions than benign ones. To protect buyers interests, most platform use third-party Payment software to hold money before the shopper confirms the reception of goods. It generates an economic cost for the collusion organization caused by moneys time value. When a buyer is satisfied with the product, he can click theconfirm button on the e-commerce website to complete the order or just let it automatically closed after 15 days. The money will be transferred to sellers when trading is successful. Otherwise, the money will return to the buyers. The third-party payment service has been wildly adopted in online market and it promotes the online transactions by suppling institution- based credit [55]. Taobao uses Alipay as their escrow service to secure per transaction. When it comes to fake transaction, the seller needs to prepay the payment for goods to collusion organization. The money finally will return to seller by going through the third- party payment institution. This process usually cost two to five days because the time spent on delivery. It will be even longer if buyers forget to confirm reception. The moneys time cost will be high if per product is too expensive. The same amount of money can undertake more transaction about low-price products. So, the fraud shopkeeper prefers to low value goods when improving the reputation scores of collusive sellers. Therefore, low value items are the better choice to gain more positive ratings with the same amount of money. Chau et al. [17] indicated the low-value products are observed in activities that aim to inflate reputation. As mentioned above, we come to the next hypothesis:
Hypothesis 2:(Low-value Product Hypothesis): Fake transactions prefer cheaper products than expensive ones. The e-commerce platforms usually take actions to detect and prevent the fraud transaction. Then they will close the fraud accounts. The collusion organizations have to keep registering new account to guarantee enough accounts that are supplied to fulfill the collusion tasks. It is reasonable to conclude that many new registered accounts participate intake trading. Therefore, we build hypothesis below:
Hypothesis 3: The fake transaction gangs attempt to avoid the risks of being detected by website operator, they need to supply the fake express logistics
information though they do not delivery anything. If the transactions refer to tangible products, illegal organizations are required buying express number from express companies for the fake express information. Therefore, the cost for fake delivery goes up. Intuitively, collusive communities do not want to face the fee. They need to take the product nature into consideration. A feasible solution is to transact virtual product which is no need for physical delivery. Such as: online software, music, digit photos, Game card, telephone recharging, etc. So, next hypothesis is put forward:
Hypothesis 4: (The Collusion Product Nature Hypothesis):Collusion organization prefers virtual products than tangible ones.
Hypothesis 5:(The Collusive Transaction Product Type Hypothesis):
Fake transactions groups have a different preference for sixteen types of products. As online comments become more and more influential in guiding online participators to make purchase decisions, review fraud
[35] has emerged as a major threat to this process. This black hat practice intends to affect peoples buying decisions by creating misleading reviews about domains of businesses (e.g., restaurants, hotels). By posting good and long comments for their own or leaving low scores and terrible comments for rivals, malicious business owners can often achieve sales increase. It has been estimated that about 16% of Yelp reviews written for the restaurants in the metropolitan Boston area are fake [48]. To make the situation even worse, review fraud practitioners today have evolved in specialization. They are found to collaborate, and form coordinated campaigns [4], [72]. Such that richer manpower and trickier tactics can be put into use to achieve more covert and cost-effective fraud practices.There are prior attempts attacking such collusive fraud. Livingston [46] found out that higher reputation always results in higher profit for sellers. So, the fraud sellers always chase high reputation even hiring collusion service. Many researches have been conducted to verify that positive comments have positive relationship with the sales volume [44].When customers surfing on e-commerce market; the comments evaluated by previous consumers play a part in the decision of potential byers. The motivation behind the same level rating may be totally different. Some people give high rating after they receive the desired quality. While others think highly of the enthusiastic service or the delivery speed. Compared with reputation score, more valuable information be reflected by detail comments writing by previous buyers. Those comments enable the potential buyers to make sensible decision based on more information. By interviewing certain amount of e- commerce users, we reach a conclusion that, if a buyer is willing to spend time in writing praise words to the seller, the seller should be good enough. Therefore, to improve the service of collusive organizations, the
puppet fraudsters are always asked to make detail good comments. Therefore, we put forward our sixth hypothesis:
Hypothesis 6:(The informative reviews Hypothesis):
The puppet buyers are asked to provide positive rating and detailed evaluation. Many researchers have focused on improving the current reputation systems [22]. Sellers have intension to inflate their reputation because they eager to acquire more notice and trust of potential buyer. The shopkeepers who have already possessing high credit score also join fake transaction by using low-value product to inflate reputation, because they want to attract good buyers to consume luxury goods. It brings more huge profit for sellers with high reputation scores than the new player. It is not easy to make a buyer shop high value product in an online shop with low reputation. Thus, we infer that:
Hypothesis 7: (The High Reputation Sellers Hypothesis):
Seller with high credit score is motivated to take part in fake transactions.
-
-
DATA COLLECTION AND DATASET PREPARATION
-
DATA SOURCE
In our empirical study, we use a real-world dataset supplied by Taobao platform, which is one of the leading e-commerce websites over the world [44]. This platform was set up in 2003. Taobao only took two years to be top in Chinese e-business list [34]. Our data were acquired under a non-disclosure agreement. The data includes fraudulent accounts related to fraud transaction and corresponding transaction records. Several efforts have been made to detect collusive transaction. There are two kinds of detection mechanism. One is complaint-driven mode, while the other is all-seller investigation. Complaint-driven mode refers to that Taobao will undertake an investigation in specific seller who are complained by buyers. If the seller cannot supply strong evidence to explain the suspicious case, he will be labeled as a fraud account. All-seller investigation is conducted by Taobao to detect the shady organization professional in reputation inflation. The platform aims to identify whose reputation grows too fast. We have both kinds of data. We used a self-developed web crawler program to capture benign trading records. It contributes to build our transaction network.We collect data about well- behaved users who have relationswith fraudulent sellers who are labeled by platform. Finally,we obtained a mass of information of legitimate users whohave been recognized as benign users by platform. Thereare 170899 transactions, including 2917 fraud
transactions(1.71%), 803 collusive accounts and 23,401 non-collusiveaccounts that we used for analysis.
-
PRODUCT FEATURES
We then use the data collected from Taobao to generatetwo variables:If virtual good and Product type. ThelabelIf virtual good is dummy variable which is used toidentifying whether the product is physical goods. It equalsone, if a product is virtual, otherwise zero. We use variableproduct type to represent the type of product. There are16 categories in all. When we sort the products, we refer tothe 16- category listing from Taobao homepage.
TABLE 1: THE DETAIL OF SIXTEEN CATEGORIES
After the data processing, those two properties were converted into digital information from text information.To generate the attribute if virtual good, we classified 6,040 records manually. We took five thousand records fortraining in the naive Bayesian model, while the remaining as the test set.
TABLE 2: VARIABLE DEFINITIONS
-
CONTROL VARIABLES
In our empirical study, the dependent variable is a dummy variable. If a transaction is fraud, the label is 1, and 0isused to label benign transaction. In our study, we treat transaction as a collusion when both the seller and buyer are fraudsters. If one side is benign user and the other side Normally, the seller gets the payment when the buyer con-firms reception. This rule is published to protect the sellers benefits if the buyer forgets to confirm the order. System will rate the trade as good level in default. We
mark Default good comment as A3. Intuitively, there should be seven types of evaluation. However, there only have five types with B1 and C1 in our dataset. Because, buyers who comment seller with degree B or C are unsatisfied with the shopping experience strongly which related to the product quality or service. They need to complain, so they all write comment. That is why our data do not have B1 or C1 that one-click comment. So, we classify the evaluation into five groups finally (Table 3). We should notice that Type A3 comments should rarely appear in collusion transaction. Because in that way, their cost will be higher. Alipay hold a significantly long time before paying it to sellers. User-written comments are taken into consideration. Various types of comments supply different value to sellers:
-
RATING LEVEL AND DETAIL REVIEWS
After each transaction, buyer will be asked to rate the corresponding seller within three choices mentioned above. Of course, buyer can do nothing. Then the system will automatically add one score to the sellers reputation with a default praise remark A default good comment. After rating step, shopper can write detail review or advice to seller of his own accord. In our experiment, we take both rate levels and detail reviews into account. Here, we use A BC to mark three rating level: positive, neutral, and negative respectively. Label 1 denotes detailed text comments, and label 2 mean no text comment. Our study defines three levels of evaluation grade. The positive rate can be divided into three categories based on different appraisal behavior. The neutral and negative also be classified into two subclasses respectively according to platforms regulations. The positive rating has three types. The first one is called one-click positive reputation, namely, type A1. The buyer just needs to click the good button on the screen and leaves nothing in the detail review area. The second type named An evaluation in detail is denoted as A2. It occurs when buyer supplies good comment and provides writing reviews to the shopkeeper at the same time. Third, if the buyer does not rate the sellers after half month, the transaction should to be closed because the third-party payment holds the money. Normally, the seller gets the payment when the buyer confirms reception. This rule is published to protect the sellers benefits if the buyer forgets to confirm the order. System will rate the trade as good level in default. We mark A default good comment as A3. Intuitively, there should be seven types of evaluation. However, there only have five types with B1 and C1 in our dataset. Because, buyers who comment seller with degree B or C are unsatisfied with the shopping experience strongly which related to the product quality or service? They need to complain, so they all write comment. That is why our data do not have B1 or C1 that one-click comment. So, we classify the evaluation into five groups finally (Table 3). We should notice
that Type A3 comments should rarely appear in collusion transaction. Because in that way, their cost will be higher. Alipay hold a significantly long time before paying it to sellers.
TABLE 3: FIVE KINDS OF COMMENTS
-
THE DESCRIPTIVE STATISTICS
The descriptive statistics for our indicators.There are nine indexes and five types of reviews. As we cansee fromthe descriptive statistics for our indicators.There are nine indexes and five types of reviews. As we cansee from the table 4, the mean price of products is RMB52.8981, and S.D (the stand deviation) for it is 154.52 withthe value range from
0.01 to 10100. The rest variables can becomprehended in the same way.
TABLE 4: THE DESCRIPTIVE STATISTICS
-
-
EMPIRICAL MODEL
A fraud detection model use logical regression because logistic regression model has an advantage over characteristic counting and the model are improved by using characteristics that characteristic counting is limited or does not have [50]. With a usage of thirteen percent used in detecting financial fraud, the logistic regression model is placed on the cutting edge of data mining tool [1]. In collusion prediction this model has been widely utilized, although linear relation does not reflect the about the variables [49]. In basic form statistics model widely use logistic model and also logistic function to model a binary dependent variable. In a form of binomial regression, logistic regression is
estimating the parameters of a logistic model; using regression analysis. Mathematically, with two possible values a binary logistic model has a dependent variable; these are represented by an indicator variable, where0 and 1 two values are labeled. Collusive transaction as 1 and benign one as 0 is label using logistic model. We use a real-world dataset supplied by Taobao platform, in empirical study. Under a nondisclosure agreement our data were acquired. Related to fraud transaction and corresponding transaction records, the data includes fraudulent accounts. We used a self-developed web crawler program to capture benign trading records. It contributes to build our transaction network. We collect data about well-behaved users who have relations with fraudulent sellers who are labeled by platform. Finally, we obtained a mass of information of legitimate users who have been recognized as benign users by platform. There are 170899 transactions, including 2917 fraud transactions (1.71%), 803 collusive accounts and 23,401 non-collusive accounts that we used for analysis.After cleaning and preparing the data and built the detection model, we use sklearn package to realize the logical regression under the python environment. Released in 2007, scikit-learn has become an important machine learning library for Python. Scikit-learn, or sklearn for short, supports four machine learning algorithms including classification, regression, reduction and clustering. It also includes three modules: feature extraction, data processing and model evaluation. Sklearn is an extension of Scipy, based on the NumPy and matplotlib libraries. By taking advantage of these modules, the efficiency of machine learning can be greatly improved. Sklearn has a rich API and is popular in academia. Sklearn already encapsulates a number of machine learning algorithms, including Logical regression. At the same time, Sklearn has a large number of built-in data sets, which saves the time of acquiring and sorting data sets. We verify the proposed indexes by adopting the approach of logic regression. Some variables including buyers k-score, buyer age, the volume of transactions per month, review length, product price and sellers reputation are replaced by corresponding logarithmic equivalents, because the considerable skewness emphasizes the need for these transformations. The detection model is presented as below:
Likelihood of collusion= 0+1*ln Frequency
+2* ln Buyers k_core
+ 3*ln Sellers Reputation
+ 4*ln Buyers Age
+ 5* ln Comments + 6* Price
+ 7* ln If Product Virtual
+ 8* ln Product Type
+9 * ln Review Length (1)
-
EXPERIMENTAL RESULTS
The experiment results are shown in Table 5. All the indexes are remarkable at the level of 0.01. Through the coefficient (0.124) of indicator buyer k core, our first hypothesis (The Fraud Users Liveness Hypothesis) is supported. From conception of k core, we find out that high frequency does not mean high k- core. A node with high frequency may be with low k- core. The result verifies the fact that employed customers are interacting with many shop keep those buyers are active in making fake transactions. The negative coefficient (0.398) for the price indicator supports the low value product hypothesis (H2). It reflects the relationship between product price and the possibility of collusive users quantitatively. It indicates that cheap goods are more likely to be involved in fake transactions.
The second type of evaluation, A2 represents an evaluation in detail. It has significantly positive relationship with fake transactions as the coefficient shows. It demonstrate scour sixth hypothesis, the informative reviews hypothesis. The negative coefficient (1.311) of the variable if virtual indicate that products form is a feature of collusive transactions. The outcome means tangible products are less involved in collusive transaction. Namely, it validates the fact that collusive transactions prefer virtual than physical one. The result supports the collusive transaction product form hypothesis (H4). The positive coefficient of six kinds of product (type 3, 5, 7, 8, 11, 16) indicates that fake transaction groups have a different preference for the 16 types of product. It focuses on six types as table 6 shown. We check in every category in details. When user clicks on the type, Taobao homepage shows more detail about the category. Also, we look in the fraud transaction in dataset to find out In our study, behaviors between benign participator and fraudster are significantly different. The process of obtaining these indexes, short text classification, can be generalized to other kinds of fraud activities.
TABLE 5: LIKELIHOOD OF COLLUSION: LR-ESTIMATION RESULTS
The specific product name. Type 3 includes studying material and early education. Type 5 includes tissue. Type 7 supplies sports class. Type 8 embraces
dancing and music training. Type 11 involves online design. Type 11 covers learning ticket local service. We could find out that most of them do not need delivery. The result supports our collusive transaction product type hypothesis (H5). Besides, the individual- related indicators are also significant in this model. As we can see from table 6, the fake buyer is always new user of the platform. To maximum the profit of illegal organization, those accounts often begin their fake transactions as soon as possible. Therefore, the new registered fraud buyer hypothesis (H3) is verified. Similarly, the shopkeeper with higher reputation is more likely to participate in fake transactions, consistence with the High Reputation Hypothesis (H7). In brief, the seven hypotheses shown in Fig. 1 are supported in our experiment.
-
KEY FINDINGS
Presenting a framework to acquire the characters of fake transactions in e-business platform is one of our goals. Seven key findings are shown in table 6, and we also give our corresponding explanation. There is not a very effectively method in recognizing fraudsters. Many efforts have been made to this area. However, not all the available information has been extracted from the user data, such as the detection in fraudster (Weijia et al., 2011), collusive fraud detection in online reviews (Chang, 2016).
We identify the costs and the benefits of collusive organization, then select a set of features with two new derived factors that are hypothesized to be valid in differentiating benign and undesirable behavior. We make use of more available information by putting forward variables like review length, product type, product nature. In our study, behaviors between benign participator and fraudster are significantly different. The process of obtaining these indexes, short text classification, can be generalized to otherkinds of fraud activities.
TABLE 6: KEY FINDS
-
PERFORMANCE
Figure 2: Cross-validate ROC curve
In our model. we leverage two product features to enhance the accuracy of online collusive detection. To test the significantly effective of the product features. In figure 2, we compare our model with the previous one which is without product features in the same data context by ROC graph. The ROC graph (receiver operating characteristics) is proposed by Spackman in 1989.In our study, we use the cross- validate ROC curve to visualize the performance for our detection model. The result is shown in figure 2.Our detection model adopts the form of logit model to recognize the fake transactions. Lim (2000) pointed out that the logistic regression has a good performance in classification area, no matter about training time, mean rank of error rate, or the mean error. ROC is an effective tool to measure the performance of a classifier. (Fawcett, 2006).In Figure 2, the vertical axis refers to the true positive rate which is also named recall. The horizontal axis means the false alarm rate. The black dotted line is a diagonal line, with y equals
x. The line y=x stands for the strategy which guesses a class randomly. The blue line represents the ROC curve for the detection model proposed in our study, while the red one stands for the model without product features. Our model outputs a probability and we choose a threshold by experiment. Every threshold generates a various dot in the ROC space. There is a significant improvement in our result from the red line (0,765) to blue one (0.866) by adding our two new variables. To test a classifiers performance, AUC, the area under the ROC curve, is widely used. (Bradley, et al., 1997). As shown in figure 2, the AUC for our detection model is 0.866. We make comparison between our model and the previous one which is without product features in the same data context. The result is significantly improved (0.765). The results show that the two variables are significantly effective. It also shows that we supply a valid means for detecting collusive transactions.
-
-
CONCLUSION
The contribution in our study is three-fold: (1) we introduce two new features of fraud transaction and combine them with other user characters for fraud recognition. Our way of acquiring the indicators could
be generalized to other collusion behavior detection. (2) We use a real-world dataset to verify the practicability of our detection model. (3) We give some implications to platform policy in online e-commerce for protecting online reputation environment. Key findings are shown in table 6. We also give our corresponding explanations. The process of obtaining these indexes, short text classification, can be generalized to other kinds of fraud activities.
There are significantly different behaviors between benign participator and fraudster. In collusive transactions, many new registered forged buyers actively participate fake transactions by shopping virtual and cheap products. They will supply high praise by giving high rating level and detailed writing reviews. Further research could pay more attention to develop the model mentioned above to a more universal one. Diversified informative data from other e-business platforms can be used to uncover the gains and the costs of fraud transactions to check the effectiveness of the collusion detecting model. What is more, the collusive organizations are trying to improve their abilities to avoid being detected by Taobao. So, one direction of our further research is to develop a more adaptive detection model.
-
Extracting and reasoning about implicit behavioral evidences for detecting fraudulent online transactions in e-commerce, Decis. Support Syst., vol. 86, pp. 109121, Jun. 2016.
-
M. Luca and G. Zervas, Fake it till you make it: Reputation, competition, and yelp review fraud, Harvard Bus. School, Boston, MA, USA, Working Paper 14-006, 2013.
-
C. Xu, J. Zhang, C. Long, and C. Long, Uncovering collusive spammers in Chinese review websites, in Proc. ACM Int. Conf. Inf. Knowl. Manage., 2013, pp. 979988.
-
J. A. Livingston, How valuable is a good reputation? A sample selection model of Internet auctions, Rev. Econ. Statist., vol. 87, no. 3, pp. 453465, 2005.
REFERENCES
-
M. Albashrawi and M. Lowell, Detecting financial fraud using data mining techniques: A decade review from 2004 to 2015, J. Data Sci., vol. 14, no. 3, pp. 553569, 2016.
-
A. APAMukherjee, B. Liu, and N. Glance, Spotting fake reviewer groups in consumer reviews, in Proc. Int. Conf. World Wide Web, 2012, pp. 191200.
-
G. S. Becker, Crime and punishment: An economic approach,
J. Political Econ., vol. 76, no. 2, pp. 169217, 1968.
-
D. H. Chau, S. Pandit, and C. Faloutsos, Detecting fraudulent personalities in networks of online auctioneers, in Proc. Eur. Conf. Princ. Pract. Knowl. Discovery Databases, vol. 4213. New York, NY, USA: Springer-Verlag, 2006, pp. 103114.
-
W. You, L. Liu, M. Xia, and C. Lv, Reputation inflation detection in a Chinese C2C market, Electron. Commerce Res. Appl., vol. 10, no. 5, pp. 510519, 2011.
-
T. M. M. Verhallen and R. G. M. Pieters, Attitude theory and behavioral costs, J. Econ. Psychol., vol. 5, no. 3, pp. 223249, 1984.
-
I. C. L. Ng and L.-M. Tseng, Learning to be sociable: The evolution of homo economicus, Amer. J. Econ. Sociol., vol. 67, no. 2, pp. 265286, 2010.
-
P. A. Pavlou and D. Gefen, Building effective online marketplaces with institution-based trust, Inf. Syst. Res., vol. 15, no. 1, pp. 3759, 2004.
-
G. D. Randels, The contingency of business: Narrative, metaphor, and ethics, J. Bus. Ethics, vol. 17, no. 12, pp. 12991310, 1998.
-
W. Li, D. Wu, and H. Xu, Reputation in Chinas online auction market: Evidence from Taobao.com, Frontiers Bus. Res. China, vol. 2, no. 3, pp. 323338, 2008.
-
J. Zhao, R. Y. K. Lau, W. Zhang, K. Zhang, X. Chen, and D. Tang,