
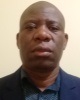
- Open Access
- Authors : Ngwarai Shambira , Golden Makaka , Patrick Mukumba , Mahali Lesala, Kittessa Roro, Jody Julies, Henerica Tazvinga
- Paper ID : IJERTV9IS080159
- Volume & Issue : Volume 09, Issue 08 (August 2020)
- Published (First Online): 22-08-2020
- ISSN (Online) : 2278-0181
- Publisher Name : IJERT
- License:
This work is licensed under a Creative Commons Attribution 4.0 International License
Wind Resource Assessment in the Upper Blinkwater area in the Province of Eastern Cape, South Africa
Ngwarai Shambira1*, Golden Makaka1, Patrick Mukumba1 Mahali Lesala1, Kittessa Roro2, Jody Julies2, Henerica Tazvinga3
1Department of Physics, Faculty of Science and Agriculture, University of Fort Hare, P. Bag X1314, Alice, 5700, South Africa.
2CSIR Energy Centre, Building 34, Room 17, Pretoria, South Africa
3South African Weather Service, 01 Eco park Drive, Eco glades Block B, Centurion, Pretoria, South Africa
Abstract In South Africa, the Eastern Cape Province partnering with lower Saxony State of Germany has led to an initiative of electrifying a small village of Upper Blinkwater in the Raymond Mhlaba Local Municipality through the construction of a hybrid mini-grid system in two phases. The first phase which includes solar photovoltaic, battery bank for storage and diesel generator as a backup system was installed. The second phase of the project still outstanding is the integration of the wind component to the mini grid. The project seeks to demonstrate that off- grid communities can be electrified using renewable technologies thereby improving living conditions, economic opportunities and slowing down rural to-urban migration. This study presents the wind potential assessment of the outstanding component using Weibull distribution function. The wind data was collected at 10-minute intervals using the ZephIR Lidar at heights between 11 and 100 m above the ground level (AGL) from April to November 2019. Wind data at 11, 20 and 30 m heights was used. The Weibull Scale (c) and Shape (k) parameters was obtained through Openwind, WAsP, Maximum Likelihood (ML) and Least Squares (LS) algorithms. Seven statistical indicators namely: Coefficient of determination (COD) Mean absolute bias error (MAE), Mean absolute percentage error (MPE), Root mean square error (RMS), Relative root mean square error (RRMS), Correlation coefficient (R), and Index of agreement (IOA) were calculated to establish the most effective algorithm. The results revealed that Openwind algorithm was the best. For heights of 11, 20 and 30 m, Upper Blinkwater recorded 4.36, 4.61 and 4.78 m/s overall average wind speeds respectively. The overall wind power density obtained was 144.99, 171.52 and 192, 19 W/m2 at heights 11, 20 and 30 m AGL respectively. The most dominant wind came from the north-west direction. These findings suggest that Upper Blinkwater is only suitable for standalone applications.
KeywordsWeibull distribution; Wind speed;Wind rose; Wind Potential; Renewable energy
-
INTRODUCTION
The world is experiencing high energy demand due to the exponential increase in population growth [1]. The situation is aggravated by governments in many countries failing to cope with the provision of energy to all its people especially in remote areas [2]. Traditionally fossil fuels have been the leading energy supplier especially for household energy, use in industries and the transport sector [3]. This has led to an increased emission of greenhouse gases into the atmosphere such as carbon dioxide (CO2) which has serious negative effects to both the environment and the earths climate [4]. Notable effects of these changes in climate were witnessed between 2017 and 2019 in South Africa and its neighboring countries in the form of droughts, floods, cyclones and heat waves [5]. In spite of all this South Africa is among the top nations in Africa, trying to provide alternative energy sources (wind, solar, hydropower, bioenergy etc.) which are clean, sustainable and environmental friendly with a total of installed onshore wind capacity of 2094.00MW in 2019 [6].Wind energy is undisputed the second largest, fastest growing, clean and inexhaustible renewable energy source. It is traditionally considered to be the oldest source of energy used for many centuries primarily for pumping water for drinking, in agriculture for irrigation purposes as well as grinding of grain [7][9]. In 2019 the world installed capacity of onshore wind was recorded as 594,396.20 MW and the renewable generation capacity increased by 59 GW (+10%) in 2019 [6]. Countries such as China, USA and Germany are leading in installed wind power capacity in the world with 204,548.38 MW,103,555.20 MW and 53,315.00 MW respectively [6], [9]. It is therefore necessary to collaborate with these leading countries so that skills, expertise and experiences can be learnt for successful implementation of generating electricity using wind energy. South Africa has made spirited efforts in this regard and partnered with the Lower Saxony state of Germany to provide electricity to the Upper Blinkwater area of Eastern Cape Province using wind-solar- biodiesel hybrid system. In addition to this it is important to stress that the Upper Blinkwater community does not have electricity and there are no prospects that they will be connected to the national grid. This is because currently South Africa is experiencing a national energy crisis and high infrastructure cost in expanding the national grid to remote areas. It is therefore tempting to conclude that the electrification of Upper Blinkwater community through the mini grid system is the only viable option. Wind energy is converted into electricity using wind turbines, however their performance is highly depended on the availability of wind with enough kinetic energy to turn them [10]. Therefore an assessment of the resource potential on the location before installation of wind turbine is mandatory [11]. This assessment will enable the wind project to reduce its investment risk and also to maximize its efficiency by choosing appropriate wind turbines that are suitable for the prevailing wind speeds [12] .
-
LITERATURE REVIEW
2.1 Current researches in wind assessment
A wide range of researches have been done on wind resource assessment worldwide. To emphasize its significance, some current researches are presented here. Ouedraogo et al [7] conducted wind potential evaluation at locations from Burkina Faso to establish if it was suitable to install wind pumping system to draw water for use by breeders and their herds. They obtained wind speed for the area between 2.4 and 4.2 m/s. Their conclusion was that the two sites would be suitable for installing wind pumping system and the third site needed hybrid systems because its wind speed was below 2.5 m/s at 10m hub height. Assowe Dabar et al [13] performed wind assessment for the first time in the Republic of Djibouti from eight sites. Three sites from the eight investigated were having above 6.0 m/s of average wind speed and therefore wind projects could be established on these three sites. Kassem et al [10] analysed wind resource capacity in three locations in Lebanon by utilizing Weibull distribution function. Wind power densities (WPd) varying from 14.634 up to 25.280 W/m2 were also recorded at the locations. Based on these findings, they recommended that small scale wind turbines must be utilized in these locations. Galarza et al [14] conducted an evaluation of five techniques used to calculate c and k. Five years of weather data collected from four weather stations located in Junin-Peru was used. Maximum likelihood and both empirical methods of Justus and Lysen were found to be most effective techniques. The six performance indicators employed to evaluate this were R, IOA, MAE, MPE, RMS and RRMS. Sadullayev et al [15] also did an examination of wind available in Bukhara Oblast located in southwestern Uzbekistan. The results depicted average values of wind speeds over 3.4 m/s at all heights considered in the study .The power densiies were
40.98 and 164.79 W/m2 measured at heights of 10 and 100 m respectively. Due to low wind potential in Bukhara it was suggested that low-powered wind turbine units must be used. Yazidi et al [16] analysed weather data at Lamhiriz village in the south of Morocco. The data was collected at three heights above ground level. The analysis depicted that Weibull distribution fitted well the observed wind data. They also found that the annual mean wind speeds were around 8.6, 9.2 and 9.7 m/s at 40, 60 and 80 m heights respectively. It was recommended that Lamhiriz village was suitable for electricity generation. Kutty et al
[17] did an analysis of wind resource availability for Suva, capital of Fiji.The weather data collected for 5 years was analysed employing ten algorithms for k and c parameter estimation. Performance analysis applying RMS, COD, MAE and MPE led to EMJ rated as most effective algorithm. Suva recorded power density of 159 W/m2.These findings demonstrated that Suva was suitable for utilizing wind power.-
Wind potential in South Africa
Even though South Africa is making greater strides in utilizing wind power, just few scientific researches have been presented on wind resource assessment. The site location is not spared from lack of information on wind power available hence the need to carry out the current study. Recent evaluation of wind potential in South Africa by Fant et al [18] confirmed that South Africa has good wind potential resources but less reliable due to fluctuations that occur over different time scales. It was noted that the wind resource was more secure and available in winter (June, July and august) than summer season (January, February and March) and also reliable during the day than at night. The authors also asserted that the wind resource availability did not always match with peak demands as observed over daily and yearly timeframes. They recommended that for wind harvesting to be possible and more secure they should invest also in wind power storage technologies. Tshimbiluni and Tabakov [19] evaluated the wind power available for Port Elizabeth in Eastern Cape, South Africa. The power density of 138 W/m2 with wind speed above 5 m/s was measured at 10m AGL with an overall wind power density (WPd) of 138 W/m2 was obtained. Weibull distribution was used to fit the actual data and maximum likelihood algorithm was used to calculate its scale and shape parameters. They recommended that the area was suitable for small scale wind projects.
Manyeredzi and Makaka [20] carried out wind potential assessment of Fort Beaufort, Eastern Cape Province, South Africa using a ten year period wind data. Introduction of wind energy technologies in residential areas was the primary focus of their study. The results obtained showed that the maximum power density was 123.1 W/m2 at 15m height and concluded that small scale wind power generation projects can be established. Shonhiwa et al [21] used a five year weather data recorded at 10m height to evaluate wind potential at six sites which included Fort Beaufort. The wind power densities for the weather stations ranged from 34.7 W/m2 for Fort Beaufort to 207.8 W/m2 for Port Elizabeth. They concluded that Fort Beaufort area had low wind potential therefore suitable for standalone applications and recommended electricity generation in Port Elizabeth. Ayodele et al [22] assessed ten different sites at 20 and 60 m heights for wind potential in South Africa. It was established that at one of the sites the wind resource was very good and the power density of 694 W/m2 was recorded at 60 m AGL.
-
Fitting Distributions to Wind Data
2.3.1 Weibull distributions functions with two parameters.
This distribution has been widely applied to myriad of scientific researches to describe wind speed data because it is simple, adaptable and precise when compared to other distribution functions [15], [23][25].
Weibull Cumulative distribution function (WCdf) is given:
v k
c
G(v) 1 e
Differentiating G(v) with respect to v leads to a Weibull probability distribution function (WPdf) (two-parameter):
(1)
g(v) k
v
k 1
v k
e
(2)
dG(v)
dv c c
c
and with no units k is Weibull shape, c ( 1) represents weibull scale parameters, v represent wind speed and
dG(v) dv
is the
derivative of Weibull Cumulative distribution function with respect to v . If c increases then wind speed will also follow the same increasing trend and k values shows wind stability. Equation 1 becomes cumulative Rayleigh distribution once k 2 . Also calm conditions must be excluded since c ( 1) (m/s) [26].
-
Estimation algorithms of scale and shape parameters.
Although WPdf is generally endorsed, it is important to note that the best technique to estimate its scale and shape parameters remains a challenge [27], [28]. Hence the performance of Weibull distribution in fitting the data depends on the correct choice of the method to calculate c and k parameters. Bearing in mind that every method has its own unique limitations the following four numerical algorithms were used, and compared, to calculate k and c. Description of each algorithm is given below.
-
Maximum likelihood method (ML)
It is a widely used mathematical procedure to fit WPdf to a set of actual wind data in time series format. The numerical algorithm requires extensive iterations to estimate c and k parameters [4], [10], [28][30]. Equation 3 is used to calculate k and then Equation 4 is used to calculate c as indicated.
k 1
N V k In(V )
N
In(V )
(3)
i i i
N
N
i 1 i 1
V k N
i
i 1
1
c 1 N V k k
(4)
N i
i 1
where, N 0 represent total data points and Vi
-
Least Squares Method (LS)
represents wind speed (m/s) for ith time step.
Least Square method commonly known as Graphical method is also used to match Weibull distribution to a set of actual wind data. The c and k parameters are obtained through use of cumulative distribution function. Firstly, Equation 1 is transformed to Equation 5 by applying logarithmic transformation two times [28], [31]:
1
In In kIn U kInc
(5)
1 G(U )
Equation 5 is in the form of linear function y mx d , where x = In U
and y =
In In 1 and therefore the slope
1 G(U )
m k and the y-intercept d kInc . The values of x and y are then computed using actual wind data. The gradient m and y-
intercept d are computed by the standard least squares regression algorithm. Weibull shape (k) and scale (c) are given by Equation 6.
k m and c expd / k . (6)
-
WAsP method
WAsP algorithm has two specific conditions listed below that needs to be satisfied first [30], [32]:
-
Average WPd of the matched Weibull distribution (WPdwbl ) is the same as the WPd of the distribution of actual data ( WPdobs ).
-
Proportion of WPdwbl
average wind speed.
values greater than average wind speed must be equal to the proportion of WPdobs values greater than
Using first requirement c is given as in Equation 7:
N 3
V i
c i 1
(7)
3 3
k
k
N 1
In satisfying condition two, Y is proposed to denote a number of observed wind speeds that are higher than average wind speed. The WCdf G(V ) is the number of values that are belowV , so 1 G(V ) is the number of values higher thanV . Equation 8 gives the value Y .
Y 1 G(V )
Rewriting G(V) in terms of Equation 1 and then applying natural logarithms both sides to Equation 8 give:
(8)
1 N k
N Vi
InY
i 1
c
(9)
Combining Equation 7 and Equation 9 yields:
k
1 N V
N
N
In(Y )
i
i 1
N 3
(10)
V i
i 1
3 3
k
k
N 1
Coefficient Y should be computed first and Equation 10 through iterative procedure is utilized to determine k and then c evaluated using Equation 7.
-
-
Openwind Method
The Openwind Method similar to WAsP method has also two specific conditions for matching the Weibull distribution to actual wind data stated below [33] :
-
WPdwbl should be equal to WPdobs .
-
Mean wind speed of the fitted WPdf ( V wbl ) must be equal to the mean of observed wind speeds (V obs ).
WPdwbl is written as:
WPd
1 c3 3 1
(11)
wbl 2 k
Similarly, WPdobs is written as:
WPd
N
1
1
V 3
(12)
obs
i
2N i1
Equating Equation 11 and Equation 12 according to the first requirement and solving for c gives Equation 13
1
1
N
V 3
N
N
i
3
3
c i 1
3
(13)
k 1
V wbl is written as:
V wbl c 1 1
(14)
k
and V obs is written as:
V 1 N V
(15)
N
N
obs
i i1
Considering the second requirement Equation 14 and Equation 15 are equated and then solved to obtain Equation 16:
N
N
1 N
V
i
c i 1
(16)
1 1
k
Equating Equation 13 to Equation 16 gives Equation 17 with only one unknown variable k:
N
N
N
N
1
1
N V 3 1 N V
i i 1
3
3
3
i
i 1
1
(17)
k 1 k 1
k is obtained by Equation 17 through an iterative procedure of Brent Method and then Equation 13 is used to determine c [34].
-
-
-
Statistical indicators for performance test.
Assessing efficiency of techniques that are utilized in estimating c and k, the following seven statistical indicators listed below were considered in this study.
-
Coefficient of determination (COD)
-
COD depicts how well actual data is matched by a model. COD is found in many ways and in this study the windographer calculated COD based on Equation 18 [35], [36].
N
i i
i i
(a z )2
N
N
COD 1 i 1
i
i
(a a )2
(18)
i 1
where, ai is the actual data values of a , a is average of all a -values and zi represent predicted values obtained from WPdf. N is total data points.
2.5.2 Mean absolute Bias error (MAE)
The MAE gives mean number of all absolute bias errors between Weibull function computed values and values obtained from actual wind speed data [29], [37]. The formula is indicated in Equation 19:
MAE 1
N WPd
WPd
(19)
N
N
i1
i,wbl i,obs
2.5.3 Root mean square error (RMS)
It is considered superior than COD in terms of precision. Its function is to report the WPds precision in fitting data by calculating the discrepancies between the values obtained by Weibull function and actual data values. A very small value is a good indicator that the distribution is well matched to the data. The RMS value is always positive [23], [29], [38], [39]. The formula is indicated in Equation 20:
RMS 1
N
(WPd
WPd
1
)2 2
(20)
N
i,wbl i,obs
i 1
-
Relative root mean square error (RRMS)
RRMS is found by dividing RMS by mean WPdobs . The formula is indicated in Equation 21:
N
N
1 (WPd
0.5
WPd )2
N i 1
i,wbl i,obs
RRMS 100
(21)
N
N
1 n
WPd
i 1
i,obs
The accuracy of the model was regarded as poor when RRMS 30%; fair if 20 < RRMS < 30%; good if 10% < RRMS < 20% and lastly if the RRMS is less than 10% it is classified as excellent [29], [40].
-
Mean absolute percentage error (MPE)
MPE depicts mean absolute percent deviation between the values of power density obtained by Weibull function and actual data values [17], [23]. The MPE formula is indicated in Equation 22:
MPE
N
N
N
1
i 1
WPdi,wbl WPdi,obs
WPdi,obs
100
(22)
2.5.6 Correlation coefficient (R)
This statistical indicator describes the strength of the linear relationship existing between the wind power density values obtained using Weibull function and those from actual wind speed values. The values of R are from 0 up to 1.If values of R are high it represent a strong agreement, whereas zero values shows that the compared two data sets are totally different [39]. R is given by:
WPd WPd WPd WPd
N
i,obs obs,avg i,wbl wbl ,avg
R i 1
(23)
WPd
WPd
WPd
i ,wbl i ,obs
2.5.7 Index of agreement (IOA)
The IOA resembles COD statistic but with some improvements, therefore it is more effective in showing the degree of accuracy between predicted values and observed data values. The values of IOA are from 0 up to 1 and If values of IOA are high it represent best match between the model and the observed data values [41]. It is given by:
N
WPdi,wbl WPdi,obs
IOA 1 i1
(24)
WPd WPd WPd WPd
N
i 1
i,obs obs,avg i,wbl obs,avg
In above Equations (19)-(24), WPdi,obs and WPdi,wbl
are the ith calculated wind power density using actual data and the ith
calculated Weibull function power density respectively. WPdobs,avg and WPdwbl ,avg are the mean of WPdi,obs and WPdi,wbl values
respectively. Also
-
and
WPd
WPd
i ,wbl
-
are the standard deviations of Weibull function power density and actual data power
WPd
WPd
i ,obs
density respectively and N is total data points.
2.6 Turbulence intensity (TI)
TI is a dimensionless number that measures the variations in wind speed that are time-dependent. High turbulence levels impacts negatively on the wind turbine components as it reduces the efficiency as well as operating life of wind turbine [42].The turbulence intensity is given by:
TI i
Vi
where i , is the standard deviation of wind speed and Vi
(25)
is the average wind speed [17]. A small value of TI means greater
stability of wind speed at the site [43].The third edition of International Electrotechnical Commission 61400-1 was used in this study to give four turbulence classes of average TI at 15 m/s wind speed. A comparison is made between obtained values with representative TI for third edition of International Electrotechnical Commission standard values [30].
-
METHODOLOGY
-
Wind Project description.
The Eastern Cape Government, through the Department of Economic Development, Environmental Affairs and Tourism (DEDEAT) is collaborating with South Africa Wind Energy Project (SAWEP) to add the wind component into the Upper Blinkwater mini-grid project. SAWEP, that is supported by the United Nations Development Programme (UNDP with resources from the Global Environment Facility (GEF), and implemented by the South African National Energy Development Institute (SANEDI) on behalf of the Department of Minerals Resources and Energy (DMRE), will fund the integration of the wind component to the mini-grid. The Council for Scientific and Industrial Research (CSIR) Energy Centre partnering with University of Fort Hare will lead the wind integration phase of the project and is responsible for the resource assessment, energy and power system modeling and knowledge transfer to the community.
-
Site description
The proposed site is a small, remote village called Upper Blinkwater located near Fort Beaufort town and about 15 km from Balfour in Raymond Mhlaba Municipality, Eastern Cape Province, South Africa. The choice of the area was motivated by a quest to provide electricity to this small off-grid community by the Eastern Cape government in partnership with the Ministry of environmental science and climate change in Lower Saxony State of Germany. It has approximately 70 households with limited infrastructure. The position of the site is latitude 32°34'31.70"S, longitude 26°33'3.30"E, and an elevation of 924 m. This site is an open-land which consists of lush grasslands on rolling hills and mountains and it has unimpeded access by all modes of transport. A value of 0.000741 m for surface roughness was calculated using windographer and therefore area classified as open land with low dwellings and trees. The ZephIR Lidar was installed at the site of interest for wind data collection from April 2019 up to Nov 2019 at 10 min average of time. The wind data was collected at 11, 20, 25, 30, 39, 45, 50, 60, 70, 80 and 100 m heights. However, for the current study wind data at heights of 11, 20 and 30 m were considered in the detailed analysis
because small scale wind turbines are intended to be installed. The geographical map of Upper Blinkwater is depicted as Figure
1.
1.
Upper Blinkwater village
Figure 1. Position of Upper Blinkwater village in South Africa on the map.
-
Wind assessment techniques.
A lot of studies have proposed different approaches of calculating Weibull parameters [4], [14][16], [44]. In this work ML, LS, WAsP and Openwind algorithms are used, and their results are compared [28].
-
Wind direction
In this study windographer software created a 16 sector Windrose diagram. The findings on how often wind blows in a particular direction were presented in section 5.4.
-
-
Performance indicators
This current work uses wind power density (WPd) as a performance indicator. This is because it only determines available wind power based only on wind speed without including the wind turbine characteristics [45]. It is classified among the best suitable quantifier of available wind resource of a site. It shows quantity of energy present at a site and also describes the strength of the winds throughout a time period [13], [28].
-
Wind power density (WPd)
WPd is expressed in two forms using parameters Weibull scale and shape or average wind speed derived from collected data as given by Eq. (26) and Eq. (27):
WPd
obs
1 v3
2
(26)
WPd
1 c3 3 1
(27)
wbl 2 k
where , WPdwbl is the Weibull wind power density (W/m2), WPdobs is the wind power density (W/m2) of actual wind speed
data, v is wind speed (m/s) and is the air density expressed in kg/m3 (assumed to be 1.225kg/m3).
For this current study to determine the wind resource availability using wind power densities, the Fazelpour et al. [13], [32],
[46] classification is used as presented in Table 1.Table 1. Classification of wind resources
Class
Fair
Fairly Good
Good
Very good
P (W/m2)
P < 100
100 P < 300
300 P < 700
P 700
-
-
RESULTS AND DISCUSSION
-
Assessment of wind speed properties.
Table 2 presents a statistical report of the average wind speed, standard deviation (StDev), minimum, maximum, range, kurtosis (Kur) and skewness (Sk) of the recorded wind speed values. The monthly average wind speed varied between 3.53 m/s in April and 7.49 m/s in July at 30 m above the ground level (AGL). An upward trend for the monthly average was observed from April to July and then starts to decrease towards the later part of the year. This trend is also similar to the observations made at 11 and 20 m heights AGL. The overall average wind speed measured at the site was 4.36, 4.61and 4.79 m/s at heights 11, 20 and 30 m, respectively and their corresponding standard deviations were 3.38, 3.23 and 3.04 at 11 m, 20 m and 30 m respectively. The standard deviation values in all the months show an increasing trend with an increase in height above the ground. However it can
be envisaged that this variation is significantly small when compared to the variation in the average wind speeds. This could be due to more stable winds experienced at greater heights. The skewness for all the months is positive. This implies that values of measured wind speed data are higher than the mean wind speed and depicts a better wind performance at this location. In addition to this the skewness depicts a noticeable decreasing trend as the height increases. This could be due to more stable winds experienced at greater heights. It is also interesting to note that the same pattern is observed for kurtosis, as it also decreases as the height is increased. The values of kurtosis for all the months are below 3, which indicate that the wind speed data has few extreme values. In July and November Kurtosis values are negative for all the heights which indicate lighter tails and a flatter distribution.
Table 2. Statistical wind data at Upper Blinkwater.
Month
Height [m]
Total Count
Mean
StDev
Minimum
Maximum
Range
Sk
Kur
April
30m
3175
3.5278
2.3093
0.6370
13.3940
12.7570
1.19
1.40
20m
3175
3.4051
2.1840
0.6640
13.0130
12.3490
1.25
1.61
11m
3175
3.2100
2.0208
0.6670
12.5770
11.9100
1.34
1.99
May
30m
3916
4.2657
3.3526
0.6600
16.4400
15.7800
1.20
0.75
20m
3916
4.1130
3.1600
0.6480
15.8620
15.2140
1.27
0.97
11m
3916
3.8784
2.9556
0.6530
15.0470
14.3940
1.36
1.27
June
30m
4195
5.4609
3.7603
0.6670
22.7900
22.1230
0.91
0.75
20m
4195
5.2596
3.6090
0.6600
21.8310
21.1710
0.95
0.97
1m
4195
4.9632
3.4118
0.6700
21.1550
20.4850
1.00
1.27
July
30m
4185
7.4899
4.1165
0.6730
20.1490
19.4760
0.19
-0.67
20m
4185
7.1848
3.9837
0.6660
19.9770
19.3110
0.23
-0.67
11m
4185
6.7750
3.7970
0.6430
19.0580
18.4150
0.27
-0.66
Aug
30m
4267
4.2671
3.0399
0.6690
21.6980
21.0290
1.25
1.83
20m
4267
4.1445
2.9012
0.6390
20.6770
20.0380
1.31
2.05
11m
4267
3.9297
2.7194
0.6700
20.0360
19.3660
1.40
2.37
Sept
30m
3817
4.0638
2.4819
0.6570
13.6180
12.9610
0.69
-0.01
20m
3817
3.9251
2.3561
0.6630
13.3580
12.6950
0.75
0.19
11m
3817
3.7146
2.1937
0.6510
12.9010
12.2500
0.83
0.39
Oct
30m
4009
4.2704
2.7509
0.6710
16.5110
15.8400
0.95
0.51
20m
4009
4.1345
2.6387
0.6710
16.0530
15.3820
0.99
0.66
11m
4009
3.9132
2.4647
0.6700
15.1830
14.5130
1.03
0.78
Nov
30m
2228
4.2048
2.2907
0.6690
12.1930
11.5240
0.47
-0.52
20m
2228
4.1050
2.1975
0.6690
11.7530
11.0840
0.49
-0.50
11m
2228
3.9229
2.0531
0.6390
10.8540
10.2150
0.51
-0.51
Overall
30m
29792
4.7787
3.3765
0.6370
22.7900
22.1530
1.07
0.87
20m
29792
4.6132
3.2273
0.6390
21.8310
21.1920
1.12
1.01
11m
29792
4.3612
3.0360
0.6390
21.1550
20.5160
1.19
1.19
-
Daily and monthly values of wind speed.
In Figure 2, daily average values of wind speeds are shown at three heights 11, 20 and 30 m AGL. During the morning before 8 am, a stable wind speed is experienced. The wind speed then increases steeply to attain greatest values of 5.9, 6.2 and 6.4 m/s at 11, 20 and 30 m heights AGL respectively, between 12:00 and 13:00. In the afternoon, average wind speed profile is dome shaped and between 21:00 and 22:00 in the evening the mean wind speed has lowest values of 3.3, 3.5, and 3.8 m/s at 11, 20 and 30 m heights AGL respectively. It can be concluded from this analysis that Upper Blinkwater is windy between 10:00 and 17:00.
Figure 2. Average daily wind speed measured at different heights of Upper Blinkwater.
Figure 3 illustrates that in July the highest wind speeds recorded were 6.8, 7.2 and 7.5 m/s at 11, 20 and 30 m heights respectively. In all months and heights the mean wind speeds were always above 3.2 m/s.
Figure 3. Mean wind speed for each month measured at different heights of Upper Blinkwater.
-
Wind speed frequency distribution of Upper Blinkwater.
The form of Weibull distribution curve is determined by k and c [31], [47]. The values of c are responsible for the distribution curve and range hence they depict amount of wind available at a site. If the values of c are high the weibull curve becomes broader. The value of k is responsible for the width of the curve. The values of k between 1 and 2 shows a shift to small values of wind speeds whilst k values within the range of 2.5 to 3 illustrates a distribution which is more skewed to high values of wind speed [31], [48].The monthly and overall c and k values given in Table 3 were computed using four methods and data collected at 11, 20 and 30 m heights AGL. At 30 m, the monthly c values were within the range of 3.814 and 8.943 m/s and the k values between 1.176 and 2.386. At 20 m height AGL, the c values changed their range to between 3.654 and 8.539 m/s and with k values moving to a range between 1.179 and 2.305. Lastly, at the 11 m height c was found between 3.88 and 8.01 m/s and their corresponding k values fell within the range between 1.149 and 2.220. Overally the k values ranged from 1.389 to 1.727 for all heights and the Weibull scale parameters were also within the range between 4.667 and 5.308 m/s at all the heights AGL. Therefore from these results a conclusion is made that high wind speed values led to the k and c at their respective heights to also increase. Figure 4 (a)-(c) also show a graphical representation of the monthly k and c values.
Table 3. Algorithms used and monthly weibull parameters and wind power densities for Upper Blinkwater at 11, 20 and 30m heights.
Method
Maximum likelihood
Least square
WAsP
Openwind
Month
Height [m]
k
c
WPdwbl
k
c
WPdwbl
k
c
WPdwbl
k
c
WPdwbl
WPdobs
April
11
1.706
3.620
47.059
1.997
3.559
36.763
1.446
3.388
51.094
1.580
3.576
51.125
51.111
20
1.670
3.835
57.742
1.916
3.769
45.704
1.471
3.654
61.998
1.562
3.789
62.007
61.983
30
1.630
3.964
66.197
1.841
3.899
53.078
1.472
3.814
70.412
1.539
3.919
70.400
70.4484
May
11
1.423
4.300
107.871
1.671
4.210
76.322
1.149
3.730
119.631
1.319
4.211
119.542
104.618
20
1.394
4.541
132.582
1.601
4.449
96.327
1.179
4.054
142.498
1.319
4.466
142.601
142.573
30
1.348
4.678
155.932
1.522
4.589
115.286
1.176
4.234
163.517
1.299
4.618
163.464
163.3810
June
11
1.524
5.533
201.596
1.668
5.448
165.842
1.454
5.405
205.221
1.492
5.494
205.168
205.214
20
1.514
5.854
241.608
1.626
5.773
205.274
1.489
5.799
242.178
1.500
5.826
242.241
242.211
30
1.496
6.064
274.511
1.582
5.992
240.016
1.527
6.116
271.315
1.499
6.049
271.470
271.3350
July
11
1.819
7.607
400.202
1.709
7.643
441.782
2.220
8.010
379.016
1.921
7.638
379.245
379.080
20
1.833
8.064
472.143
1.689
8.126
540.135
2.305
8.539
445.335
1.949
8.103
445.481
445.425
30
1.843
8.402
530.415
1.673
8.490
624.809
2.386
8.943
498.445
1.972
8.449
498.552
498.4613
Aug
11
1.557
4.402
97.770
1.808
4.318
73.768
1.326
4.086
107.875
1.437
4.329
107.917
107.822
20
1.526
4.629
117.772
1.734
4.545
91.002
1.353
4.384
127.283
1.432
4.563
127.266
127.313
30
1.485
4.746
133.429
1.655
4.669
105.639
1.367
4.577
141.589
1.415
4.690
141.586
141.4976
Sept
11
1.786
4.191
68.537
1.937
4.140
59.826
1.737
4.159
69.561
1.747
4.170
69.558
69.571
20
1.745
4.420
82.964
1.848
4.374
74.584
1.785
4.468
83.106
1.730
4.404
83.062
83.082
30
1.701
4.565
94.774
1.770
4.527
87.422
1.804
4.670
93.587
1.709
4.556
93.577
93.5703
Oct
11
1.685
4.406
86.403
1.890
4.336
70.708
1.541
4.254
89.835
1.616
4.368
89.795
89.855
20
1.656
4.647
104.057
1.831
4.576
86.393
1.544
4.519
107.325
1.601
4.612
107.307
107.327
30
1.632
4.791
116.648
1.784
4.723
98.236
1.549
4.687
119.073
1.592
4.761
119.145
119.1224
Nov
11
2.026
4.439
70.268
2.129
4.398
65.110
2.053
4.454
70.037
2.017
4.427
70.021
70.043
20
1.971
4.641
82.674
2.037
4.605
78.017
2.049
4.691
81.983
1.975
4.631
81.960
81.956
30
1.925
4.748
90.883
1.965
4.718
87.147
2.047
4.829
89.521
1.944
4.742
89.541
89.5133
Overall
11
1.526
4.869
137.056
1.727
4.782
106.599
1.389
4.667
145.027
1.453
4.811
144.919
144.993
Overall
20
1.506
5.138
164.942
1.672
5.053
131.845
1.412
4.991
171.381
1.453
5.089
171.521
171.473
Overall
30
1.480
5.308
187.855
1.617
5.226
153.631
1.426
5.221
192.266
1.446
5.268
192.079
192.1895
In Table 4a the error analysis using coefficient of determination parameter COD is presented. The test shows that a method matches well observed data when the values of COD approach one. The COD was found to be varying between 0.8680 and 0.9196. This showed that Weibull distributions fitted well with the observed data. The Least square method performed poorly when compared to other methods.
Table 4a. Statistical Error Analysis for Good Fit for Upper Blinkwater at 11, 20 and 30m heights
Method
Coefficient of determination (COD)
Heights
11
20
30
MLE
0.8932
0.9093
0.9142
Least square
0.8680
0.8845
0.8908
WAsP
0.8893
0.9127
0.9196
Openwind
0.8946
0.9126
0.9179
The performance of WAsP and Openwind algorithm was so excellent in all heights and a further goodness of fit examination was done to identify best algorithm that would represent correctly wind regime of Upper Blinkwater. To carry out the analysis, WPdwbl for all four algorithms was calculated utilizing monthly k and c values and then WPdobs values computed using actual wind data as indicated in Table 3. Table 4b shows results of six indicators used namely; MPE, MAE, RMS, RRMS, R and IOA for performance evaluation. This approach is considered to be more accurate and reliable as each statistical indicator gives an alternative view when comparing the four methods [29]. In Table 3, the results depicted that the calculated WPdwbl from the distribution was always very close to WPdobs computed from the actual data. This is evident in Table 4b because indicators for errors, MPE, MAE, RMS and RRMS had small values whilst precision indicators, R and IOA had values close to one. Open wind algorithm is ranked first at 11 and 20 m heights and second at 30m height. Therefore overally it is a superior method that must be utilized to determine k and c values. In contrast, at all heights the least square algorithm was found to be least effective in finding k and c values.
Table 4b. Monthly based assessment of four algorithms with six error analysis indicators for Upper Blinkwater at 11, 20 and 30m heights.
Method
Height [m]
MAE
RMS
RRMS
MPE
R
IOA
Rank
MLE
11
6.9028
9.5254
6.9769
4.9993
0.998514643
0.95727
3
20
6.9000
10.8069
6.6923
3.9570
0.998921719
0.96347
3
30
7.4932
12.1409
6.7108
3.5963
0.999220330
0.96446
3
Least square
11
28.4396
33.9131
24.8394
21.7365
0.982782960
0.83675
4
20
32.9819
42.6946
26.4389
19.7843
0.983534296
0.83996
4
30
36.0489
51.6296
28.5378
17.8843
0.984108040
0.84475
4
WAsP
11
0.0338
0.0455
0.0333
0.0281
0.999999917
0.99978
2
20
0.0368
0.0464
0.0287
0.0245
0.999999976
0.99980
2
30
0.0468
0.0630
0.0348
0.0348
0.999999900
0.99977
1
Openwind
11
0.0527
0.0733
0.0537
0.0381
0.999999861
0.99966
1
20
0.0285
0.0324
0.0201
0.0208
0.999999979
0.99984
1
30
0.0627
0.0748
0.0414
0.0383
0.999999950
0.99969
2
Figure 4(a)-(c) show the mean of monthly values of k and c at 10, 20 and 30 m heights for the Upper Blinkwater. It is observed from all the heights that weibull scale parameter values have very small deviations from each other in all algorithms shown. However there is a small noticeable deviation in May for LS. The values of k parameter for each month are also in phase except for the values of the LS. The LS performed poorly in calculating wind power density as a result of its k and c parameter values which were different from the values of the other three methods.
Figure 4a. Mean monthly weibull scale and shape values at 11m
Figure 4b. Mean monthly weibull scale and shape values at 20m
Figure 4c. Mean monthly weibull scale and shape values at 30m
Figure 5(a)-(c) shows pictorial representation of comparison between wind speed frequency distribution function and actual data frequency distribution. The four algorithms at all the three heights portrayed a better fitting of the data with the Openwind, WAsP and MLE method leading in that order. The least square method was the least performer overally and this was also indicated in error analysis results.
Figure 5a. Weibull distributions matched to actual data histogram at 11m of Upper Blinkwater.
Figure 5b. Weibull distribution matched to actual data histogram at 20m of Upper Blinkwater.
Figure 5c. Weibull distribution matched to actual data histogram at 30m of Upper Blinkwater.
-
Windrose diagrams.
Figure 6(a)-(c) depicts Windrose diagrams at 11,20 and 30 m AGL. The main role of a wind rose is to display data for both wind speed and direction occurrences [28]. This information is used for siting purposes so that best position for installing wind turbines is established that will maximize fully the use of wind power [49]. All measurements of wind direction are done clockwise direction with the north as the zero degrees bearing. A 22.5o degrees arc divides the whole 360o polar diagram into 16 sectors [50]. It is worth noting that most widespread winds are from the 15th direction sector between 303.75° and 326.25° degrees clockwise. This means that the dominating wind at all heights mostly comes from the north-west direction (NW). The corresponding bin frequencies for this NW direction sector are 22.64 %,21.04 % and 20.07% at 11, 20 and 30 m heights AGL It can also be observed from wind rose diagrams that 54.86 %, 54.21 % and 53.46 % of wind occurs between 258.75° and 348.75° at heights 11, 20 and 30 m respectively. This indicates that between the North North West (NNW) and West (W) direction is the most ideal for the wind turbines because in this direction they have the capacity to harness most of the available wind energy. The wind turbine will also be more efficient in this direction because they will not be frequently changing their direction.
Figure 6a. Windrose diagram at a height of 11m of Upper Blinkwater.
Figure 6b. Windrose diagram at a height of 20m of Upper Blinkwater.
Figure 6c. Windrose diagram at a height of 20m of Upper Blinkwater.
-
Turbulence intensity of Upper Blinkwater.
Figure 7 presents the turbulence intensity at 11, 20 and 30 m heights AGL. The third edition of International Electrotechnical Commission classes A, B and C are used to classify turbulence intensity in this study [30]. TI average value of 0.16 was calculated in all heights and with corresponding representative TI of 0.19. According IEC3 classification the turbulence category is A. The obtained TI value of 19% is above the recommended 16% TI at 15 m/s wind speed [17], [42].It is therefore suggested that the site requires wind turbine that can sustain higher TI as it decreases its life span. As observed in Figure 7, the value of TI decreases as the height increases, though the difference is very small. The decrease of TI with height is caused by wind speed reaching stability and becoming smoother at greater heights.
Figure 7. Turbulence intensity measured at various heights of Upper Blinkwater.
-
-
Wind power density
Overall mean wind power densities and also wind power densities for each month at 11, 20 and 30 m heights for Upper Blinkwater are depicted in Table 3. The values were obtained by using actual wind data and Weibull parameters. Scale and shape parameters computed by most accurate algorithm, Open wind were used in this analysis. It can be observed that there is slight difference between the actual power densities and Weibull power densities. This observation endorses a well known fact that Weibull distribution function is appropriate for fitting wind speed data with precision [37], [51] .The site registered highest monthly average wind power densities of 379.25, 445.48 and 498.46 W/m2 in July at 11, 20 and 30 m heights AGL respectively, followed by a recording of 205,17, 242.24 and 271.47 W/m2 in June at 11, 20 and 30 m heights AGL respectively. Furthermore the lowest monthly average power densities were recorded in April. The obtained values for this month were 51.11, 62.00 and
70.40 W/m2 at 11, 20 and 30 m heights respectively. From the results in Table 3, the power density shows an upward trend as the height is changed from 11 to 30 m. This trend is expected because power density depends on average wind speed which also increases with height. The overall wind power density for Upper Blinkwater was 144.92, 171.52 and 192.08 W/m2 at 11, 20 and 30 m heights respectively. According to the classification in Table 1, Upper Blinkwater is considered as having a fairly good wind resource therefore the site is only suitable for Stand-alone applications but using small scale wind turbines.
-
CONCLUSION
The findings from this research led to the following conclusions to be reached: The skewness for all the months is positive. This implied that values of measured data are above the average wind speed and therefore depicted a better wind performance at this location. At all the heights AGL, mean wind speeds were between 4.36 and 4.78 m/s. The diurnal and monthly average wind speed for Upper Blinkwater was over 3 m/s at all heights. In July the highest observed mean wind speed for the month was 7.49 m/s at 30 m height whilst April registered least value of 3.21 m/s at 11 m height. This period of July concurs with the winter time when it is cold and there is high power demand to provide warmth. It was also observed that Upper Blinkwater is windy between 10:00 and 17:00 reaching the maximum wind speeds in the afternoon between 12:00 and 13:00 in all the heights AGL.
In the analysis of Weibull parameters of shape and scale the results revealed that the Open wind algorithm was the best. Overally the k values ranged from 1.389 to 1.727 for all heights and the Weibull scale parameters were also within the range between 4.667 and 5.308 m/s at all the heights AGL. The COD done using windographer was found to be varying between 0.8680 and 0.9196. This showed that Weibull distributions fitted well with the observed data. A further goodness of fit test carried out utilizing six statistical indicators proved that Open wind was the most efficient algorithm, followed by WAsP and Maximum likelihood algorithms. Least Square algorithm was last in terms of performance. The Windrose diagram was constructed using Windographer software. The directional data analysis for the overall wind data set at heights 11, 20 and 30 m showed that dominating wind mostly comes from the north-west direction (NW).
The turbulence intensity results showed that the mean value was 0.16 and the value of the representative TI was also 0.19. According IEC3 classification the turbulence category is A. The results from the data analysis depicted that Upper Blinkwater site had overall wind power density of 144.92, 171.52 and 192.08 at heights of 11, 20 and 30 m AGL respectively. The obtained maximum mean monthly wind power densities for the site were 379.25, 445.48 and 498.46 W/m2 in July at heights of 11, 20 and 30 m AGL respectively. The minimum monthly mean power density were recorded in April with values of 51.11, 62.00 and
70.40 W/m2 at heights of 11, 20 and 30 m AGL respectively. According to classification of wind power densities, Upper Blinkwater should implement Stand-alone applications using small scale wind turbines.
ACKNOWLEDGMENT
The authors would like to thank the Ministry of environmental science and climate change in Lower Saxony State of Germany, Deutsche Gesellschaft für Internationale Zusammenarbeit (GIZ) both in Germany and South Africa , The Eastern Cape Government, Department of Economic Development, Environmental Affairs and Tourism, South Africa Wind Energy Project (SAWEP) , United Nations Development Programme (UNDP) , Global Environment Facility (GEF), South African National Energy Development Institute (SANEDI), Department of Minerals Resources and Energy (DMRE) who has funded and supported this project. We also want to give a special thanks to the Council for Scientific and Industrial Research (CSIR) who helped us with data collection at the site and financial support as well as Govan Mbeki Research and Development Centre at University of Fort Hare also for their support.
REFERENCES
-
J. Yu et al., Assessment of offshore wind characteristics and wind energy potential in Bohai Bay, China, Energies, vol. 12, p. 2879, 2019.
-
W. Document, S. January, J. Wright, and T. Bischof-niemz, Least Cost Electricity Mix for South Africa Optimisation of the South African power sector until 2050 CSIR Energy Centre Dr Tobias Bischof-Niemz, 2017.
-
M. Shoaib, I. Siddiqui, S. Rehman, S. Khan, and L. M. Alhems, Assessment of wind energy potential using wind energy conversion system, J. Clean. Prod., vol. 216, pp. 346360, 2019.
-
M. A. Baseer, J. P. Meyer, S. Rehman, and M. M. Alam, Wind power characteristics of seven data collection sites in Jubail, Saudi Arabia using Weibull parameters, Renew. Energy, vol. 102, pp. 3549, 2017.
-
C. Y. Wright et al., Gathering the evidence and identifying opportunities for future research in climate, heat and health in South Africa: The role of the South African Medical Research Council, South African Med. J., vol. 109, pp. 2024, 2019.
-
IRENA, Renewable Capacity Statistics 2020, Abu Dhabi, 2020.
-
S. Ouedraogo, K. Lolo, K. Attipou, A. Senah, A. Ajavon, and S. Tiem, Assessment of Wind Potential in the Perspective of Water Pumping in Sahelian Area of Burkina Faso, Int. J. Eng. Res. Technol., vol. 9, pp. 231243, 2020.
-
J. Li and X. (Bill) Yu, Onshore and offshore wind energy potential assessment near Lake Erie shoreline: A spatial and temporal analysis, Energy, vol. 147, pp. 10921107, 2018.
-
S. Ali, S. M. Lee, and C. M. Jang, Statistical analysis of wind characteristics using Weibull and Rayleigh distributions in Deokjeok-do Island Incheon, South Korea, Renew. Energy, vol. 123, pp. 652663, 2018.
-
Y. Kassem, H. Gökçeku, M. M. Mizran, and S. M. Alsayas, Evaluation of the wind energy potential in Lebanons coastal regions using weibull distribution function, Int. J. Eng. Res. Technol., vol. 12, pp. 784792, 2019.
-
M. Benmedjahed, R. Maouedj, and S. Mouhadjer, Wind energy resource assessment of desert sites in Algeria: energy and reduction of CO2 emissions, Int. J. Appl. Power Eng., vl. 9, pp. 2228, 2020.
-
P. K. Chaurasiya, V. K. Kumar, V. Warudkar, and S. Ahmed, Evaluation of wind energy potential and estimation of wind turbine characteristics for two different sites, Int. J. Ambient Energy, vol. 0, pp. 111, 2019.
-
O. Assowe Dabar, M. O. Awaleh, D. Kirk-Davidoff, J. Olauson, L. Söder, and S. I. Awaleh, Wind resource assessment and economic analysis for electricity generation in three locations of the Republic of Djibouti, Energy, vol. 185, pp. 884894, 2019.
-
J. Galarza, D. Condezo, B. Camayo, and E. Mucha, Assessment of Wind Power Density Based on Weibull Distribution in Region of Junin, Peru, Energy Power Eng., vol. 12, pp. 1627, 2020.
-
N. N. Sadullayev, A. B. Safarov, S. N. Nematov, and R. A. Mamedov, Statistical Analysis of Wind Energy Potential in Uzbekistans Bukhara Region Using Weibull Distribution, Appl. Sol. Energy (English Transl. Geliotekhnika), vol. 55, pp. 126132, 2019.
-
M. El Yazidi, A. Redouane, M. Benzirar, and M. Zazoui, Analysis of Wind Data and Assessment of Wind Energy Potential in Lamhiriz Village, Morocco, Appl. Sol. Energy (English Transl. Geliotekhnika), vol. 55, pp. 429437, 2019.
-
K. Singh, L. Bule, M. G. M. Khan, and M. R. Ahmed, Wind energy resource assessment for Vanuatu with accurate estimation of Weibull parameters, Energy Explor. Exploit., vol. 37, pp. 18041832, 2019.
-
C. Fant, B. Gunturu, and A. Schlosser, Characterizing wind power resource reliability in southern Africa, Appl. Energy, vol. 161, pp. 565573, 2016.
-
H. C. Tshimbiluni and P. Y. Tabakov, Wind Energy Potential for Small-scale WEC Systems in Port Elizabeth, in IOP Conference Series: Earth and Environmental Science, 2019, vol. 342.
-
T. Manyeredzi and G. Makaka, An assessment of the wind power generation potential of built environmentwind turbine (BEWT) systems in Fort Beaufort, South Africa, Sustain., vol. 10, 2018.
-
C. Shonhiwa, G. Makaka, and K. Munjeri, Estimation of Wind Power Potential of Six Sites in Eastern Cape Province of South Africa, Phys. Sci. Int. J., vol. 6, pp. 209218, 2015.
-
T. R. Ayodele, A. A. Jimoh, J. L. Munda, and J. T. Agee, A statistical analysis of wind distribution and wind power potential in the coastal region of South Africa, Int. J. Green Energy, vol. 10, pp. 814834, 2013.
-
P. K. Chaurasiya, S. Ahmed, and V. Warudkar, Study of different parameters estimation methods of Weibull distribution to determine wind power density using ground based Doppler SODAR instrument, Alexandria Eng. J., vol. 57, pp. 22992311, 2018.
-
A. I. Idriss, R. A. Ahmed, R. K. Said, and A. I. Omar, Suitability and Evaluating Wind Speed Probability Distribution Models in a Hot Climate: Djibouti Case Study, Int. J. Renew. Energy Res., vol. 9, 2019.
-
H. Bidaoui, I. El Abbassi, A. El Bouardi, and A. Darcherif, Wind Speed Data Analysis Using Weibull and Rayleigh Distribution Functions, Case Study: Five Cities Northern Morocco, Procedia Manuf., vol. 32, pp. 786793, 2019.
-
A. F. Lopez-Rodriguez, J. C. Serrano-Rico, and E. G. Florez-Serrano, Statistical methodologies for wind resource analysis , case: Catatumbo region
– Norte de Santander , Colombia, in 18th International Conference on Renewable Energies and Power Quality, 2020.
-
M. Gul, N. Tai, W. Huang, M. H. Nadeem, and M. Yu, Assessment of wind power potential and economic analysis at Hyderabad in Pakistan: Powering to local communities using wind power, Sustain., vol. 11, 2019.
-
A. Allouhi et al., Evaluation of wind energy potential in Moroccos coastal regions, Renew. Sustain. Energy Rev., vol. 72, pp. 311324, 2017.
-
K. Mohammadi, O. Alavi, A. Mostafaeipour, N. Goudarzi, and M. Jalilvand, Assessing different parameters estimation methods of Weibull distribution to compute wind power density, Energy Convers. Manag., vol. 108, pp. 322335, 2016.
-
D. Solyali, M. Altunç, S. Tolun, and Z. Aslan, Wind resource assessment of Northern Cyprus, Renew. Sustain. Energy Rev., vol. 55, pp. 180187, 2016.
-
M. Khalid Saeed, A. Salam, A. U. Rehman, and M. Abid Saeed, Comparison of six different methods of Weibull distribution for wind power assessment: A case study for a site in the Northern region of Pakistan, Sustain. Energy Technol. Assessments, vol. 36, p. 100541, 2019.
-
F. Fazelpour, N. Soltani, S. Soltani, and M. A. Rosen, Assessment of wind energy potential and economics in the north-western Iranian cities of Tabriz and Ardabil, Renew. Sustain. Energy Rev., vol. 45, pp. 8799, 2015.
-
T. Lambert, Openwind Algorithm, Windographer, 2017. https://www.windographer.com/ for Weibull Fitting.
-
S. Rehman, N. Natarajan, M. Vasudevan, and L. M. Alhems, Assessment of wind energy potential across varying topographical features of Tamil Nadu, India, Energy Explor. Exploit., vol. 38, pp. 175200, 2020.
-
T. Lambert, Windographer, Mistaya Engineering. https://aws-dewi.ul.com/windographer/.
-
T. B. M. J. Ouarda, C. Charron, and F. Chebana, Review of criteria for the selection of probability distributions for wind speed data and introduction of the moment and L-moment ratio diagram methods, with a case study, Energy Convers. Manag., vol. 124, pp. 247265, 2016.
-
A. A. Teyabeen, F. R. Akkari, and A. E. Jwaid, Comparison of Seven Numerical Methods for Estimating Weibull Parameters for Wind Energy Applications, in 2017 UKSim-AMSS 19th International Conference on Modelling & Simulation Comparison, 2017, pp. 173178.
-
I. Tizgui, F. El Guezar, H. Bouzahir, and B. Benaid, Comparison of methods in estimating Weibull parameters for wind energy applications, Int. J. Energy Sect. Manag., vol. 11, pp. 650663, 2017.
-
A. A. Teyabeen, F. R. Akkari, and A. E. Jwaid, Comparison of Seven Numerical Methods for Estimating Weibull Parameters for Wind Energy Applications, Proc. – 2017 UKSim-AMSS 19th Int. Conf. Model. Simulation, UKSim 2017, pp. 173178, 2018.
-
M. F. Li, X. P. Tang, W. Wu, and H. Bin Liu, General models for estimating daily global solar radiation for different solar radiation zones in mainland China, Energy Convers. Manag., vol. 70, pp. 139148, 2013.
-
J. Zhou, E. Erdem, G. Li, and J. Shi, Comprehensive evaluation of wind speed distribution models: A case study for North Dakota sites, Energy Convers. Manag., vol. 51, pp. 14491458, 2010.
-
T. Aukitino, M. G. M. Khan, and M. R. Ahmed, Wind energy resource assessment for Kiribati with a comparison of different methods of determining Weibull parameters, Energy Convers. Manag., vol. 151, pp. 641660, 2017.
-
G. Ren, J. Wan, J. Liu, and D. Yu, Characterization of wind resource in China from a new perspective, Energy, vol. 167, pp. 9941010, 2019.
-
I. PoboÃková, Z. Sedliaková, J. imon, and D. Juráová, Statistical analysis of the wind speed at mountain site Chopok, Slovakia, using Weibull distribution, IOP Conf. Ser. Mater. Sci. Eng., vol. 776, pp. 19, 2020.
-
A. T. Abolude and W. Zhou, Assessment and performance evaluation of a wind turbine power output, Energies, vol. 11, 2018.
-
F. Fazelpour, E. Markarian, and N. Soltani, Wind energy potential and economic assessment of four locations in Sistan and Balouchestan province in Iran, Renew. Energy, vol. 109, pp. 646667, 2017.
-
Z. H. Hulio, W. Jiang, and S. Rehman, Techno – Economic assessment of wind power potential of Hawkes Bay using Weibull parameter: A review, Energy Strateg. Rev., vol. 26, p. 100375, 2019.
-
C. Shonhiwa and P. Mukumba, An Assessment of Wind Power Generation Potential for Margate Town in South Africa, Int. J. Energy Power Eng., vol. 4, p. 32, 2015.
-
M. Nedaei, Wind resource assessment in Hormozgan province in Iran, Int. J. Sustain. Energy, vol. 3, pp. 650694, 2014.
-
M. Gul, N. Tai, W. Huang, M. H. Nadeem, and M. Yu, Evaluation of wind energy potential using an optimum approach based on maximum distance metric, Sustain., vol. 12, 2020.
-
B. Olomiyesan, Performance evaluation of Weibull function for wind data analysis in two selected locations in North-Western, Nigeria, Int. J. Phys. Res., vol. 6, p. 18, 2018.