
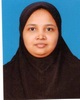
- Open Access
- Authors : J. Yamuna Bee , Dr. M. Vargheese
- Paper ID : IJERTV10IS110137
- Volume & Issue : Volume 10, Issue 11 (November 2021)
- Published (First Online): 03-12-2021
- ISSN (Online) : 2278-0181
- Publisher Name : IJERT
- License:
This work is licensed under a Creative Commons Attribution 4.0 International License
A Review of Biomedical Imaging using Deep Learning Approaches
J. Yamuna Bee1, Dr. M. Vargheese2
CSE Department, Assistant Professor, PSN College of Engineering and Technology,Tirunelveli CSE Department ,Professor, PSN College of Engineering and Technology, Tirunelveli
Abstract:- Web of Medical Things (WoMT) is the collection of medical devices and related applications which link the healthcare IT systems through online computer networks. In the field of diagnosis, medical image classification plays an important role in prediction and early diagnosis of critical diseases. Modern hospitals and clinics depend upon scientific imaging technology to diagnose patients. Soft computing has performed a vast position in latest scientific imaging era breakthroughs. It can manage ambiguity and enhance photo quality. The scientific discipline has been provided many gentle computing approaches. We will have a take a observe scientific imaging strategies and gentle computing strategies which includes fuzzy logic, synthetic neural networks (ANNs), genetic algorithms, system learning, and deep learning. We as compared and contrasted every technique used for the opposite imaging modalities primarily based totally at the device evaluation parameter. Various studies alternatives for similarly development are offered on the stop of the paper. No earlier studies has checked out this to our knowledge.
INTRODUCTION:
Using scientific imaging, clinicians can also additionally examine the shape and feature of interior organs while not having to carry out any intrusive operations. The subject of scientific imaging presently employs a extensive variety of various picture modalities. While conventional radiography makes use of x-rays, those modalities use non-invasive strategies to present the radiologist a third-dimensional attitude of the anatomical and useful conduct of organs inclusive of the coronary heart, kidneys, liver, and spleen in evaluation to standard radiography. In the future, extra checks could be finished to evaluate coronary heart rate, blood supply, chemical composition, and blood absorption adjustments as imaging technology grow to be extra useful.) Medical imaging modalities consist of a extensive variety of techniques, inclusive of CT, ultrasound, Positron Emission Tomography (PET), SPECT, optical coherence tomography (OCT), and Mammography, among others, as shown in Figure 1.
-
Computerized Tomography (CT):
The term computed tomography, or CT, refers to a computerized x-ray imaging procedure in which a narrow beam of x-rays is aimed at a patient and quickly rotated around the body, producing signals that are processed by the machines computer to generate cross-sectional imagesor slicesof the body. These slices are called tomographic images and contain more detailed information than conventional x-rays. Once a number of successive slices are collected by the machines computer, they can be digitally stacked together to form a three-dimensional image of the patient that allows for easier identification and location of basic structures as well as possible tumors or abnormalities. Data from the projection is continuously recorded during the operation. A sequence of overlay sinusoidal pictures seems to be created when all of the one- dimensional projections from the camera system have finished spinning.) These raw CT scanning data are known as sonograms, and this picture is the most often utilized source for businesses across the country Finally, an image reconstruction approach is used to create a tomographic picture of the patient's internal organs from the ultrasound data.
-
Particle Emission Tomography (PET):
Positron emission tomography (PET) is a type of nuclear medicine procedure that measures metabolic activity of the cells of body tissues. PET is actually a combination of nuclear medicine and biochemical analysis. Used mostly in patients with brain or heart conditions and cancer, PET helps to visualize the biochemical changes taking place in the body, such as the metabolism (the process by which cells change food into energy after food is digested and absorbed into the blood) of the heart muscle.PET differs from other nuclear medicine examinations in that PET detects metabolism within body tissues, whereas other types of nuclear medicine examinations detect the amount of a radioactive substance collected in body tissue in a certain location to examine the tissue's function.
-
Single-photon emission computed tomography (SPECT):
A single photon emission computed tomography (SPECT) scan is an imaging test that shows how blood flows to tissues and organs. It may be used to help diagnose seizures, stroke, stress fractures, infections, and tumors in the spine. SPECT is a nuclear imaging scan that integrates computed tomography (CT) and a radioactive tracer. The tracer is what allows doctors to see how blood flows to tissues and organs.Before the SPECT scan, a tracer is injected into your bloodstream. The tracer is radiolabeled,
meaning it emits gamma rays that can be detected by the CT scanner. The computer collects the information emitted by the gamma rays and displays it on the CT cross- sections. These cross-sections can be added back together to form a 3D image of your brain. ASPECT scanner rotates around you as you lie down on a table.) Your inside organs and other tissues are photographed using a SPECT computer. Images are then transmitted to a computer, which utilizes the data to construct a 3D model of your body. Because of the low radiation dose used in the SPECT scan, you should see your physician if you are worried about being exposed to radiation. The use of this imaging technology was determined to have no long-term health implications.
1.3 Magnetic Resonance Imaging (MRI)
Magnetic Resonance Imaging (MRI) is a non-invasive imaging technology that produces three dimensional detailed anatomical images. It is often used for disease detection, diagnosis, and treatment monitoring. It is based on sophisticated technology that excites and detects the change in the direction of the rotational axis of protons found in the water that makes up living tissues. MRIs employ powerful magnets which produce a strong magnetic field that forces protons in the body to align with that field. When a radiofrequency current is then pulsed through the patient, the protons are stimulated, and spin out of equilibrium, straining against the pull of the magnetic field. When the radiofrequency field is turned off, the MRI sensors are able to detect the energy released as the protons realign with the magnetic field. The time it takes for the protons to realign with the magnetic field, as well as the amount of energy released, changes depending on the environment and the chemical nature of the molecules. Physicians are able to tell the difference between various types of tissues based on these magnetic properties.
1.5. Optical Coherence Tomography (OCT):
Optical coherence tomography (OCT) is an emerging imaging modality that has been widely used in the field of biomedical imaging. In the recent past, it has found uses as a diagnostic tool in dermatology, cardiology, and ophthalmology. In this paper we focus on its applications in the field of ophthalmology and retinal imaging. OCT is able to non-invasively produce cross-sectional volumetric images of the tissues which can be used for analysis of tissue structure and properties. Due to the underlying physics, OCT images suffer from a granular pattern, called speckle noise, which restricts the process of interpretation. This requires spcialized noise reduction techniques to eliminate the noise while preserving image details. Another major step in OCT image analysis involves the use of segmentation techniques for distinguishing between different structures, especially in retinal OCT volumes. The outcome of this step is usually thickness maps of different retinal layers which are very useful in study of normal/diseased subjects. Lastly, movements of the tissue under imaging as well as the progression of disease in the tissue affect the quality and the proper interpretation of the acquired images which require the use of different image
registration techniques. This paper reviews various techniques that are currently used to process raw image data into a form that can be clearly interpreted by clinicians.
-
Ultrasound (US):
Ultrasound imaging (sonography) uses high-frequency sound waves to view inside the body. Because ultrasound images are captured in real-time, they can also show movement of the body's internal organs as well as blood flowing through the blood vessels. Unlike X-ray imaging, there is no ionizing radiation exposure associated with ultrasound imaging.In an ultrasound exam, a transducer (probe) is placed directly on the skin or inside a body opening. A thin layer of gel is applied to the skin so that the ultrasound waves are transmitted from the transducer through the gel into the body. The ultrasound image is produced based on the reflection of the waves off of the body structures. The strength (amplitude) of the sound signal and the time it takes for the wave to travel through the body provide the information necessary to produce an image.
-
OVERVIEW:
To see if researchers have discovered Deep Learning (DL) approaches, go to the PubMed website and search for "biomedical image application using SC techniques" with the keyword "article" selected as the text type. The number of articles published has increased significantly since 2010 (Figure 2), reaching over 1231 publications in 2019. The rise of DL approaches has had a crucial role in its spread (Figure 2). Once the sources were chosen, they were then consulted. Web of Science, Google Scholar, PubMed, and Springer were the four well-known databases used in this investigation. CT, PET, reconstruction, and segmentation, as well as (genetic algorithm) OR (machine learning), were all combined to create the search string. Other relevant terms included: (fuzzy logic), and (deep machine learning). To narrow down the results, similar items were combined into groups. After that, they went through a screening procedure in which titles and abstracts were assigned to them based on their performance. A thorough review of the research articles selected for analysis was conducted before any conclusions could be drawn. This included a close examination of each paper's primary goal, anatomical focus, methodology, evaluation criteria, and dataset properties utilized throughout the experiments. As a consequence of the structuring of these data, the present piece of writing was able to be completed. In most publications, the focus is on reconstructing an image, then segmenting it, and then denoising it. This is important to note. Others have gotten far less attention than they deserve. According to the statistics, CT is the most common imaging test, followed by PET and finally the United States.) Figure 2 shows the year-to-year growth in the number of publications published.
Figure 2: Over the period 20102020, the number of papers published per year related to biomedical application work utilizing DL approaches (a), the distribution by article target (b), and the distribution per modality form (c)
-
DEEP LEARNING(DL)
Deep learning (DL) is a machine learning method that allows computers to mimic the human brain, usually to complete classification tasks on images or non-visual data sets. Deep learning has recently become an industry- defining tool for its to advances in GPU technology.Deep learning is now used in self-driving cars, fraud detection, artificial intelligence programs, and beyond. These technologies are in high demand, so deep learning data scientists and ML engineers being hired every day. Deep learning and other ANN methods allow computers to learn by example in a similar way to the human brain. This is accomplished through passing input data through multiple levels of Neural Net processing to transform data and narrow the possible predictions each step along the way.
-
SOFT COMPUTING (SC)
-
Due to its ability to effectively cope with the ambiguities inherent in collected image data, SC has been used in medical imaging [5]. Soft computing approaches are used in a variety of sectors, including scientific research, medical science, management, and engineering.) With soft computing, artificial intelligence may be achieved by simulating human brain function to handle real-world situations that are ambiguous. For a more dynamic, skillful, and optimal solution, statistical computation (SC) may be a combination of computer procedures and biological methods. SC was initially proposed in the 1960s by Lotfi
A. Zadeh. Fuzzy logic, artificial neural networks, and evolutionary algorithms are among the important soft computing technologies examined in this review [5, 7]. SC approaches, in contrast to hard computing, are more tolerant of ambiguity, imprecision, partial truth, and estimations. They work better when they have greater freedom to do what they need to do within the parameters of their job. Soft computing technology is the most commonly used and recommended by scientists due to its adaptability and accuracy. It also has the advantages of being cost-effective, efficient, and capable of resolving complex problems. A variety of SC approaches are shown in Figure 3.
SOFT COMPUTING APPROACHES
-
Genetic Algorthm
-
Fuzzy Logic
-
Artificial Intellience
-
Machine Learning
-
Neural Network
4.1. Genetic Algorithm:
Genetic Algorithm (GA) is a search-based optimization technique based on the principles of Genetics and Natural Selection. It is frequently used to find optimal or near- optimal solutions to difficult problems which otherwise would take a lifetime to solve. It is frequently used to solve optimization problems, in research, and in machine learning.Nature has always been a great source of inspiration to all mankind. Genetic Algorithms (GAs) are search based algorithms based on the concepts of natural selection and genetics. GAs are a subset of a much larger branch of computation known as Evolutionary Computation.GAs were developed by John Holland and his students and colleagues at the University of Michigan, most notably David E. Goldberg and has since been tried on various optimization problems with a high degree of success.In GAs, we have a pool or a population of possible solutions to the given problem. These solutions then undergo recombination and mutation (like in natural genetics), producing new children, and the process is repeated over various generations. Each individual (or candidate solution) is assigned a fitness value (based on its objective function value) and the fitter individuals are given a higher chance to mate and yield more fitter individuals. This is in line with the Darwinian Theory of Survival of the Fittest.In this way we keep evolving better individuals or solutions over generations, till we reach a stopping criterion.
-
Fuzzy Logic
Fuzzy Logic (FL) is a method of reasoning that resembles human reasoning. The approach of FL imitates the way of decision making in humans that involves all intermediate possibilities between digital values YES and NO.The conventional logic block that a computer can understand takes precise input and produces a definite output as TRUE or FALSE, which is equivalent to humans YES or NO.The inventor of fuzzy logic, Lotfi Zadeh, observed that unlike computers, the huma decision making includes a range of possibilities between YES and NO.
-
Survey of Fuzzy Logic:
A fuzzy-based technique for iterative image reconstruction in Emission Tomography has been developed, according to Mondal and Rajan [20]. (ET). There are two simple stages in this procedure: fuzzy filtering and smoothing. To rebuild edges, we use fuzzy filtering and fuzzy smoothing algorithms. (Fuzzy smoothing, on the other hand, is used to penalize only those pixels where the edges are missing in the immediate vicinity. Once a suitable degree of convergence is achieved, these operations are repeated until they are no longer necessary. When it came to picture segmentation algorithms, Bose [21] started with a fuzzy artificial bee colony as a starting point (FABC). To identify a better cluster century, this research has used fuzzy c- means (FCM) and artificial bee colony (ABC) optimization techniques. The recommended approach FABC is more reliable than other optimization methods like GA and PSO. FABC (particle swarm optimization). Grayscale photographs were used in an experiment that produced a slew of other images, including some fabricated medical and textural images. Fast convergence and low computing resource requirements are two advantages of the presented approach To increase picture reconstruction accuracy in capacitance tomography systems, Debas et al. [22] developed an improved Fuzzy Inference System (FIS) image reconstruction technique. With the proposed paradigm, outcomes are more precise while processing costs remain the same or even go down. For ECT structure reconstruction, the suggested approach's accuracy and computing cost make it an excellent choice. The "single- stage fuzzy" image reconstruction approach improves picture reconstruction in terms of both time and resolution, making it a promising paradigm for ECT.)
-
-
Artificial intelligence
Artificial intelligence is a science and technology based on disciplines such as Computer Science, Biology, Psychology, Linguistics, Mathematics, and Engineering. A major thrust of AI is in the development of computer functions associated with human intelligence, such as reasoning, learning, and problem solving.Out of the following areas, one or multiple areas can contribute to build an intelligent system.
4.3 Machine Learning:
For the first time, in the late 1980s and early 1990s, the idea of machine learning was floated. Arthur Samuel [30] coined the phrase "machine learning" in 1959. An AI subset lets robots behave and make data-driven decisions to complete certain tasks. To learn and evolve, these programs must be built using particular methods that allow them to be exposed to new data over time. There have been several applications of machine learning (ML) in recent years, including the reconstruction of medical images, segmentation and classification, and the recognition of human body parts from images.
-
Neural network:
Neuronal networks have gained great traction in recent years, notably for a method known as deep learning, which makes use of enormous, sophisticated neural networks. Deep learning and neural networks, two artificial intelligence techniques, have created a new framework for inverse problems that have the potential to alter the area.
-
Survey of Neural Networks:
Kartheeswarn [40] used PSO-ANN (particle swarm optimization with artificial neural networks) to develop a quick and accurate sequential and parallel data decomposition method. To save training time, the author divides the dataset into smaller subsets and uses PSO to give weights to each one.) By decomposing and assigning weights similarly, the training time may be decreased. As a result, using the sequential technique will require more practice time.
A computer vision system was used by Souza et al. [41] to do automatic CXR lung segmentation. When pulmonary defects lead to "closed" lung sections, it's used to reduce the reconstructive issue. The proposed approach utilizes a two-DNN convolution method and is broken down into four stages: picture acquisition, initial segmentation, reconstruction, and final segmentation (see Figure 1). Based on results from tests conducted in Montgomery
County, it had an average sensitivity, specificity, accuracy, and dice coefficient, as well as a Jaccard index.) A DNN model-based reconstruction phase is used in the lung segmentation method to solve dense abnormality in chest X-rays, according to our findings. The first efficient and convergent INN framework was Chan et al. [42]'s Momentum-Net. INN was added to the block-wise MBIR approach that used the momentum and NN-regression majorizers. Using majorizers in each layer, Momentum-Net estimates components for quick MBIR and noniterative MBIR components using momentum terminology. It's important to note that Momentum-other Net's layers are also made up of these three components (multi-layer backpropagation inversion). The convergence to a fixed point is ensured in two asymptomatic situations, for certain nonconvex MBIR variables and convex optimal sets, by guaranteeing that they converge. A regularization parameter selection approach based on the statistical radius of primary matrices is presented to better understand the differences in data fit between training and testing sets. Because of this, the resulting MBIR is quicker and more accurate than conventional CNN systems. To recreate CT images, Wu et al. [40] built a deep convolutional neural network (DCNN).) To make CT reconstruction network training more realistic for modern processors, this research intends to minimize memory and time consumption while keeping the quality of rebuilt pictures. They employed DeepUNet as a CNN to deal with greedy learning's local minimum problem and created independent quadratic substitutes with aggregate data fidelity subsets to avoid simple local minima and improve picture quality.) In both two- and three-dimensional problems, our technique beats iterative reconstruction based on total variation and dictionary learning. Table 4 gives a quick review of the work of several researchers in the field of biomedical image processing using neural networks.
-
-
Generative Adversarial Network (GAN):
An ever-evolving group of opponents is a threat. GAN is a family of artificial intelligence algorithms extensively used in machine learning and was first proposed by Ian Goodfellow et al. in 2014 [50]. Two neural networks compete with each other in a conventional GAN configuration.) It is possible to use two different GAN systems to produce different types of results. The effectiveness of these two networks continues to grow throughout the approach. The discriminator network allows GAN to tackle far more complicated data production difficulties as compared to typical simple neural networks. Because of its widespread usage in image processing, its data-generating capabilities are regularly called upon. Picture synthesis, semantic image processing, and design transmission through multiple networks are all made easier with this new technology. One network is used to generate clean images from low-dose, limited-angle, or sparse view data, while the other is a discriminator network used to evaluate the results. When using a generative model, such as GAN, new data is generated based on the input rather than old data being removed [51]. used to evaluate the results. When using a generative model, such as GAN, new
data is generated based on the input rather than old data being removed [51].
-
A Survey of the Generative Adversarial Network:
An integrated low-dose CT reconstruction technique coupled with various algorithms was developed by Pathak et al. [52].) "Global Dictionary-based Statistical Iterative Reconstruction" and "Adaptive Dictionary-based Statistical Iterative Reconstruction" approaches are combined in this methodology. D has already been determined in this instance, and GDSIR can be used in addition to D if it is amended (in this case). To eliminate artifacts in low-dose CT, ADSIR can be used as a suitable replacement for selecting and using a gain-based intervention filter. The first input is CT scans, followed by dictionary learning, GDSIR, or ADSIR. You may use this method for a wide range of problems including too much smoothing, artifacts, and noise. Deora et al. [53] developed a unique generative adversarial network (GAN) architecture for reconstructing CS-MRI images using their technique. It improves the output's overall quality by employing a patch-based GAN discriminator and a structural similarity index loss. The authors were particularly concerned with preserving high- frequency information and adequate textural data in the reconstructed picture. To allow more direct data transfer and adjustable network length, dense and residual connections were introduced into U-Net-based generator architecture previously unattainable. According to their findings, the proposed method surpasses alternative reconstruction procedures in terms of efficiency and resistance to noise.
-
-
Deep Learning:
Because it learns features and tasks directly from input, it's a development of neural network or machine learning (ML) technology. Data includes things like text, images, and music. High-performance GPUs employ DL, which is a complicated approach because of the vast quantity of datasets accessible everywhere.) Medical imaging challenges including image reconstruction, segmentation, super-resolution, and classification are among the many applications for deep learning techniques that are now garnering a lot of attention. Deep learning approaches such as iterative and cascaded neural networks have been claimed to deliver the best possible results for a variety of quantitative quality metrics, including PSNR, NRMSE, and SSIM, spanning imaging modalities and imaging systems, for numerous quantitative quality metrics. DL-based approaches have been used successfully in many image processing applications, including image reconstruction, denoising, segmentation, classification, and others.
-
Survey of Deep Learning:
An Examination of the Situation Alder et al. [60] employed a partially learned method to deal with a poorly stated problem. This solution resolves the issue by doing a series of gradient descents. Using the inverse issue and prior knowledge, deep learning is used to improve the PSNR (signal-to-noise ratio) by 5.4 dB while reconstructing the variance. The error function selection does not become any better by using another iterative strategy. With the DL
method, Kang and colleagues created a new low-dose computed tomography (CT) technique [61]. To find and eliminate different noise patterns from CT data, a novel CNN architecture appropriate for denoising CT was presented. I broke the project down into three sections: To help train deep networks, the counter-let transform can effectively evaporate the directional noise component; to remove this noise, CNN has a lot of promise; and to gather different types of data from a big amount of data, DNN is appropriate. Reconstruction is now completed in a fraction of the time it took with earlier MBIR techniques.
CONCLUSIONS:
As well as examples of their applications, this article includes a brief review of fuzzy logic, evolutionary algorithms, neural networks, machine learning, and generative adversarial networks. There was also an investigation and comparison of the application of each approach, the different techniques/methods used, the various imaging modalities used, the system used, and each parameter examined As we discovered after exhausting all other options, the deep learning algorithm is garnering a lot of attention these days due to its ability to solve a wide range of medical imaging difficulties. Medical imaging scientists are intrigued by these qualities and have begun to examine them. As an example, image reconstruction, segmentation, detection, and classification have all seen rapid adoption in both classic and new applications. The findings of this survey, which may be used to motivate biomedical researchers, can have an impact on future CT and PET research. DL with image input is likely to be the industry standard in medical imaging technology in the future.
REFRENCES:
-
A. C. Kak and M. Slaney, 0 Content – Principles of Tombgraphic Imaging, )e Institute of Electrical and Electronics Engineers, Inc., New York, NY, USA, 1988.
-
K. Herrmann and P. R. Ros, Computed tomography, in Textbook of Clinical Gastroenterology and Hepatology, pp. 10061013, Blackwell Publishing Ltd, Hoboken, NJ, USA, 2012.
-
D. W. Townsend, J. P. J. Carney, J. T. Yap, and N. C. Hall, PET/CT today and tomorrow, Journal of Nuclear Medicine, vol. 45, no. 1, pp. 4S14S, 2004.
-
R. J. Jaszczak, R. E. Coleman, and C. Bin Lim, Spect: single photon emission computed tomography, IEEE Transactions on Nuclear Science, vol. 27, no. 3, pp. 11371153, 1980.
-
S. Kobashi, L. G. Ny´ul, and J. K. Udupa, Soft computing in medical image processing, Computational and Mathematical Methods in Medicine, vol. 2016, Article ID 7358162, 1 page, 2016.
-
A. Madan, A. Kaura, N. Verma, and S. Jindal, Fuzzy techniques in image processing, in Proceedings of the Climate Change 2013: #e Physical Science Basis, pp. 130, September 2005, https://www.cambridge.org/core/product/identifier/ CBO9781107415324A009/type/book_part.
-
L. A. Zadeh, Soft computing and fuzzy logic, IEEE Software, vol. 11, no. 6, pp. 4856, 1994.
-
K. Deb, Introduction to genetic algorithms, SadhanaAcademy Proceedings in Engineering Sciences, vol. 24, no. 4, pp. 293315, 1999.
-
S. Mirjalili, J. Song Dong, A. S. Sadiq, and H. Faris, Genetic algorithm: theory, literature review, and application in image reconstruction, in Nature-Inspired Optimizers. Studies in Computational Intelligence, S. Mirjalili, D. J. Song, and A. Lewis, Eds., Vol. 811, Springer International Publishing, NY, USA, 2020.
-
M. Changhua, P. Lihui, D. Yao, and D. Xiao, Image reconstruction using a genetic algorithm for electrical capacitance tomography, Tsinghua Science and Technology, vol. 10, no. 5, pp. 587592, 2005.
-
A. M. T. Gouicem, K. Benmahammed, R. Drai, M. Yahi, and A. Taleb-ahmed, Multi-objective GA optimization of fuzzy penalty for image reconstruction from projections in X-ray tomography, Digital Signal Processing, vol. 22, no. 3, pp. 486496, 2012.
-
M. J. D. Cruz, A. Jadhav, V. Chavan, and M. A. Dighe, Detection of lung cancer using backpropagation neural networks and genetic algorithm, International Journal Advance Research on Computer and Communication Engieering, vol. 5, no. 4, pp. 963967, 2016, http://www.ijcta.com.
-
P. Liu, Y. Li, M. D. E. Basha, and R. Fang, Expedited genetic algorithm for medical image denoising, in Proceedings of the International Conference on Medical Image Computing and Computer-Assisted Intervention, Springer International Publishing, Granada, Spain, September 2018.
-
N. B. Bahadure, A. K. Ray, and H. P. )ethi, Comparative approach of MRI-based brain tumor segmentation and classification using genetic algorithm, Journal of Digital Imaging, vol. 31, no. 4, pp. 477489, 2018.
-
S. A. Qureshi, S. M. Mirza, and M. Arif, Determination of optimal number of projections and parametric sensitivity analysis of operators for parallel-ray transmission tomography using hybrid continuous genetic algorithm, International Journal of Imaging Systems and Technology, vol. 17, no. 1, pp. 1021, 2007.
-
-