
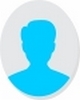
- Open Access
- Authors : K. Bendou , M A. Abrache , C. Cherkaoui
- Paper ID : IJERTV10IS120110
- Volume & Issue : Volume 10, Issue 12 (December 2021)
- Published (First Online): 31-12-2021
- ISSN (Online) : 2278-0181
- Publisher Name : IJERT
- License:
This work is licensed under a Creative Commons Attribution 4.0 International License
Towards An Intervention Agent based on Trace Analysis to Support and Motivate As Part of Eals (LMS, MOOC)
K. Bendou |
M A. Abrache |
C. Cherkaoui |
IRF-SIC, Laboratory, IbnZohr |
IRF-SIC, Laboratory, IbnZohr |
IRF-SIC, Laboratory, IbnZohr |
B.P.37/S Agadir Morocco |
B.P.37/S Agadir Morocco |
B.P.37/S Agadir Morocco |
Abstract- The work we present in this paper is a part of the general problem of drop-out in online learning environments (LMSs and MOOCs). It deals in particular with the motivation issue of learners to finish their courses. In this sense, we defend the idea of the interest of animated pedagogical agents to motivate learners and to adapt content, presentation and navigation to their profile. We therefore propose the first specifications of the PAOLE agent. The design of this agent is based on a new concept which we have called the Pedagogical Intervention. An intervention may be of different kinds, but it is more precisely used to overcome the current problem of abandonment of learners. The PAOLE agent combines characteristics of intelligent agents like: autonomy, ability to perceive, to interact, to reason and to act; and some other characteristics of pedagogical agents as: observing, evaluating, adapting content, recommending, engaging, motivating, etc.
Keywords_Learning;Environment;Adaptivity;Recommendation; Feedback; Pedagogical Intervention; Pedagogical Agent.
I.INTRODUCTION
The quality of Online Learning Environments (OLEs) depends essentially on their flexibility, the capacity to adapt the delivery and ability to provide feedback and recommendations to maintain students engaged on courses.
Most OLEs currently suffer from the drop-out problem which constitutes the most studied problem in the last ten years. Recent Studies point out a significant dropout rate estimated at 90% and shared by most authors in the field [6]; [18]. The causes of abandonment are related in particular to the lack of motivation and commitment of learners, other reasons are associated to occupation, lack of time, isolation, etc. The new generation of students needs to be connected, and wants to have a continuous human presence. In that direction, the opportunities of using pedagogical agents in OLE become more and more important. Pedagogical agents are mainly reactive, autonomous and proactive [20]. They can improve interactions and support learning. Indeed, agents have other important characteristics such as ability to perceive, to communicate reason and act in specialized fields [21]. They also have the ability to cooperate with other agents, which makes them effective in the context of learning environments. Research in that domain is not new. Pedagogical agents have already made their proof to simulate collaborative and adaptive behaviors as they appear in some particular works. Different Intelligent Tutoring System (ITS) used agents as virtual entities emulating a human tutor adapting content to the learners needs, profiles, preferences, rhythm, style [26]; [13]; [19]. Pedagogical agents are also used as learning aids
and recommender agents to adapt content to user profiles [28]. In this paper, we discuss the contribution of pedagogical agents, which are visible characters in learning environments designed to facilitate learning, to motivate learners and to engage them in an effective learning process. We present in particular an agent who proposes alternatives and specific intervention strategies. This agent should be able to adapt learning pathways to a learner by recommending pedagogical interventions strategies, according to a number of indicators compiled in the learner model.
In this paper, we first discuss the limitations of traditional MOOCs and in the second part we present the advantages of integrating an animated educational agent into these e- learning environments. The last part will present our first specifications and developments of an animated pedagogical agent that takes into account our approach of improvement of the OLE in order to increase the motivation of learners.
-
LIMITATION OF TRADITIONAL MOOCS
A detailed review of the literature shows that current MOOCs suffer from four main limitations, namely:
-
MOOCs Teach to a Certain Percentage of the learners Hill [18] identified different types of MOOC participants: (1) No shows register, but dont even login, (2) Observers log in and read content, but do not engage, (3) Drop-Ins want to achieve a specific goal, which once satisfied, ends the course for them, (4) Passive Participants consume content, but dont do assignments, (5) Active Participants fully intend to complete the course and all activities. In this classification, we can immediately see that only the fifth class is likely to complete a course. In addition, latest research demonstrates that among learners who complete courses; most of them seek tangible benefits such as getting a new job, starting a business, or completing prerequisites for an academic program.
-
Students Need Assistance and Immediate Feedback Engaging students in the learning process is a big challenge for online learning environments. Designers and teachers must develop appropriate methods of engagement for online education. Research has shown that the higher the levels of interaction in a course, the more students develop positive attitudes towards courses. The feedback, encouragement and assistance are crucial in the success of a Learning Process. The instructor feedback reinforces the course material and encourages the students to become more engaged in the learning process. Thus as mentioned before, some authors
show that this is possible simply by adding some encouragement sentences above the statement of mathematics exercises such as "Remember, the more you practice, the more you become intelligent, or this could be a difficult problem, but we know that you can do it easily", etc. At the same moment, appropriate assistance and help can avoid student disappointment, anxiety, and confusion and learning can be increased. Situations where learners require help and assistance are many; it may be such as help to solve problems, to manage their time, to retrieve best resources, etc.
-
Students Want a Great Adaptive Instruction
Even today, most of learning environments are still delivering the same educational content in the same way to learners with different profiles. Everyone nowadays knows that the learners are different, by their needs, expectations, interests, preferences, prerequisites, difficulties, facilities, performance, styles, etc. This difference is generally felt both by learners who are well advanced and at risk of being bored, but also by learners who have problems with their acquisition and are at risk of dropping out. In the context of MOOCs, learners are encouraged to read carefully the resources and participate in activities [10].
However, it is very difficult to track all activities and interactions in these tools because of the massiveness number of enrolled students. What is sought learners is a tool which adapts its delivery and enhance motivation in different dimensions, ways and levels, namely the adaptation of content, presentation, navigation; but also through individual dimensions and/or collaborative, the fun and the massive one. The learner is also looking for tools that allow for a great openness (even massive and therefore very social), and that takes into account thei different daily practices.
-
Students Need Continuous Presence
In online learning environments, learners are more likely to feel that "someone is there" when needed [14] and in general, they like to enjoy a strong interaction and feel that there is a "human presence" [17].
For Hersh [10], the more the exchanges that occur within an OLE have common features with those that occur in classrooms, the more students will feel connected and engaged in their learning tasks. [23] found that the presence of teachers or tutors in an online course is an important factor influencing their success. Above all, he emphasized the presence that manifests itself through the various interventions such as: frequent feedback, clear communications, organizing and maintaining actions, providing students with clear goals, and strong direct instruction, etc.
-
-
BACKGROUND OF PEDAGOGICAL AGENTS
-
Pedagogical Agents in Traditional Learning Environments
The Intelligent Tutoring System (ITS) is an historical precursor of the new learning environments, with promising results. The ITSs have previously relied on artificial intelligence techniques and had as the main objective to simulate the trainer (or the interactions between the learner and the tutor). They constitute the first generation of learning
environments which set up pedagogical agents. Pedagogical agents are agents whose function is educational or pedagogical and whose aim is to improve learning.
A profound analysis of these pedagogical agents shows that they are very complex and efficient. We can underline for example, the agent STEVE [25], a personified agent working in a virtual training environment; or BAGHERA [24] which relies on a distributed multi-agent system where each agent can act as tutor, learner-assistant or as a teacher-assistant. Another famous intelligent agent is "AutoTutor" Graesser
[16] which helps students learn new notions in Newtonian mechanics, computer science, or scientific reasoning through a natural language dialogue that it establishes with learners. Three types of educational agents have marked the history of ITSs: pedagogical agents, assistant agents and recommendation agents. Without going into the details of the differences between these agents, we will simply say that the main objective of agents is to play different and important roles in a learning environment such as being present and reactive in order to maintain the motivation of learners. Research suggests that pedagogical agents have the ability to play many roles in the multimedia learning environment, such as demonstrating, scaffolding, coaching, modeling and testing [7]. Animated educational agents are supposed to play a playful role in guiding, reflecting and interacting with learners. Recent research shows that these characters can support the commitment and motivation of the learners [10]; [2]; [9]. -
Pedagogical Agents in New Learning Environments (LMSs and MOOCs)
To our knowledge, little research has been carried out on the integration of pedagogical or recommendations agents in MOOCs and LMSs. As a well-known agent in the context of LMS, we mention the agent ABITS (Agent Based Intelligent Tutoring System) [5]. [27] developed an extensible collaborative learning agent that was used to promote interaction among learners. Another example is the one proposed in the work of Lin [22], who has developed several agents communicating with the platform MOODLE using JADE. Other work are in the process of emergence in the context of MOOC learning environments, we so emphasize the work of [10].
Additional research, although independent of LMS and MOOC platforms, claim that Pedagogical Agents foster Engagement, Motivation, and Responsibility [1];[28]. They also suppose that Pedagogical Agents are adaptable and versatile and can Address learners sociocultural meeds. Similar research suggests integrating agents into MOOCs to adapt learning resources to the learner based on his preferences and learning style [12] Research made in [15] proposes a Recommendation System for MOOCs based on the concept of generating predictions according to other learners experiences.
Finally, although this research on the integration of agents in OLEs (LMS and MOOC) is rare, we find some attempts almost similar to the ITSs, but with the new vision and the new characteristics of the OLE. Interest is not the least, and the rest of this paper proposes the specifications of a pedagogical agent for these environments based on the
concept of educational intervention and other characteristics of agents raised before.
-
-
THE PAOLE PROJECT
-
PAOLE Project
PAOLE (Pedagogical Agent for Online Learning Environments) is a project of the IRF-SIC Laboratory, university IBN ZOHR in Morocco, whose main goal is to examine what agent technologies can bring to the motivation of learners and to minimize the Drop-Out problem. It is a continuation of the various work carried out on personalization and adaptivity in OLE. In the first development of this project, a roadmap has been drawn up to take into account a number of constraints, including the fact of taking account agent characteristics in general, but also the characteristics of pedagogical agents. It must also, as we will present next, address the problems highlighted at the beginning of this article on MOOCs.
-
PAOLE Specifications
A first review of the literature allowed us to distinguish some strategies of the pedagogical intervention of an agent, as we described above. First, we have mentioned four principles to be taken into account in MOOCs and OLE in general, namely: (1) the need to take into account the different profiles of learners, (2) continuous presence,
(3) assistance and feedback, and (4) adaptation. Other general strategies can be emphasized, such as: rewarding the effort constantly for failures findings, add frequent and clear comments. We add to this the encouragement of collaboration in forums and social media. It is also necessary to reinforce the observation of traces of the learner and their analysis in order to determine a better intervention strategy. But it goes much better when the intervention is playful and in our case played by an animated agent, which sometimes can distract and consequently distress the learner in failure or in a demotivation state.
The work we present in this section is concerned with an attempt of specifications of an animated pedagogical agent, having the general capacities of the agents and the abilities that we have just described. From an educational point of view, he is classified in the category of a facilitator, but with an additional role of guide and companion. In this context, the pedagogical agent must interact with learners to minimize learner frustration, and enhance learning.
We propose a design based on the concept of pedagogical intervention which we describe in the following. The rest of the paper addresses an area, which to our knowledge is relatively unexplored in the field of OLE. This article draws inspiration from the general importance of the design of the intervention, situating it within the broader landscape of learning analysis, and then examines the specific issues of intervention design for OLEs. In the following, we refer to our agent by the abbreviation PAOLE (Pedagogical Agent for Online Learning Environments).
-
The Pedagogical Intervention
-
Structure of the Pedagogical Intervention: Although this notion of intervention is not very common in the literature, we have chosen it to refer to the different actions of an agent related to the process of help, cooperation, collaboration, adaptation, observation, etc. We note that these actions are not of the same level of intervention and therefore the notion of intervention concerns different levels of learning. Lenoir [21] analyzes this notion in the context of traditional learning, and states that edagogical intervention is a set of interactions between "the learner, learning objects and the teacher, in connection to the purposes underlying these reports". Following this research, the notion of intervention in PAOLE is the interaction between the learner, the agent and the contents.
Our approach is to determine the importance of the intervention design, placing it in the largest landscape of educational agents. The pedagogical intervention design in this context is concerned with addressing questions such as: why intervene in learning and the teaching process? how should the agent intervene and why? (Figure 1).
Fig 1. Structure of the Pedagogical Intervention.
To carry out its task, the pedagogical agent PAOLE will have to put in place an effective pedagogical strategy and, if possible, be able to change strategy according to the situation. The pedagogical agent here uses this model to select and adapt his role, his pedagogical strategy, and choose the activities he will put in place. As shown in the figure above, this model allows the answer to three main questions:
-
Why: for this question, a pedagogical intervention occurs for several reasons, such as: motivating and retaining a learner, helping him to understand a concept or in order to solve a problem, etc.;
-
When: the moment when the pedagogical intervention is carried out is important in learning. Intervention can take place before, during or after the learner's actions, i.e., the agent must choose the appropriate time either by taking the initiative or responding to the needs and questions of the learner;
-
How: the answer to this question involves the selection of the most adapted intervention strategy to execute thereafter among a set of strategies.
-
-
-
Process of Educational Intervention
In this section, we present a set of processes that can be used by the agent PAOLE to design pedagogical interventions that support a productive learning. The different processes related to a pedagogical intervention that we have modeled are represented in the figure 2. We can distinguish six important processes, namely: (i) execute the session (ii) observe learners, which can lead to analysis and update of the learner profile (iii) the process of detecting a problem (vi) the proposal and calculation of intervention strategies, (v) the choice of an adapted intervention and its implementation, and
(vi) the operationalization of the strategy in an animated behavior of the agent.
Fig 3. Algorithm of Intervention
For lighting reasons, we propose the following diagram summarizing the steps of thealgorithm (cf. figure 4).
Fig 4. Process of the intervention mechanism
Fig 2. The General Use Case of PAOLE
-
An Intervention Mechanism
The algorithm of intervention proposed in this article aims at calculating an intervention of the PAOLE agent according to:
-
the learner's traces, (2) a set of motivational indicators and their weighting and (3) high-level indices called Thematic Coefficients.
We propose in the figure 3 a preview of the algorithm capturing this mechanism.
The first step of this algorithm consists in collecting the different traces of the learner during his/her interaction with the platform.
In the second step, we calculate a set of motivation indicators based
on the collected traces. After words, we determine the different thematic coefficients by aggregating the indicators and their weights corresponding to each coefficient. Finally, the last step aims to choose the intervention of the agent according to the determined coefficients.
We emphasize that the interventions covered in this article relate to the beginning of a learning session. For the indicators we are based on standardized indicators proposed in [4].For example, the motivational coefficient "Coefficient of Presence" is calculated according to three indicators, namely: (1) the frequency of connection, (2) the date of the last connection, and (3) the time spent in line.
-
-
Modeling Elements of Paole
-
The Architecture of PAOLE
These capabilities are intervention strategies and can be summarized as follows:
PAOLE is an autonomous Pedagogical Agent that supports human The Adapting strategy: by observing and analyzing the
learning in online Learning Environments with the main objective of keeping them motivated.
LMS or MOOC
The PAOLE
Agent
different behaviors and outcomes of the learner, the PAOLE agent can propose different types of adaptations: content, presentation, navigation, etc.;
-
The Supporting strategy: a learner may at some point need help and support. Two situations are possible: either at the demand of the learner or at the initiative of the agent. The support that can be offered by the agent
Pedagogical Intervention Database
Gestual and Verbal Database
Communication Manager
Reasoning Engine
Behaviour Engine
Presentation Manager
Fig 5. The Architecture of PAOLE
PAOLE may be at the level of: homework, solving a problem, providing additional resources, modifying the interface, or at another level such as the emotional support;
-
The Engaging strategy: the engagement strategy involves engaging a learner in the proposed courses. This strategy will, for example, engage the learner in these courses by offering him varied and advanced content. Involvement can also be at the flexibility of the course agenda by proposing additional weeks to finish homework;
-
The Motivating strategy: motivation can be expressed in questions addressed to the learner by the agent, by offering encouragement, providing feedback, encouraging collaboration, encouraging the learner to make other
From a computer architecture perspective, PAOLE consists
of four components: (i) a reasoning engine, which monitors the students interactions and generates appropriate pedagogical interventions (ii) a behavior engine responsible for generating behavior from primitive animations, sounds and speech elements (iii) a presentation manager, which enables to present generate and present agents animations, and (iv) a communication module for interactions with the other components of the learning environment as : the learner model and the domain model (see Figure 5). PAOLE is currently under development. It is not created from scratch; we use the interface of the Microsoft agent, which was grafted for the first tests to the platform Moodle. An animated agent action of PAOLE allows combining several elementary actions of the same character: messages, highlights of components, animations (show a component, applaud, greetings, etc.), and movements on the screen. Other high- level actions based in particular on intervention strategies are represented in the figure 4, in particular the strategies: adaptation, support, engagement and motivation that we present in the following.
-
Some Use Cases of PAOLE
Research suggests that pedagogical agents have the ability to play many roles in the multimedia learning environment, such as demonstrating, scaffolding, coaching, modeling and testing [8].
The contributions referenced in this paper give more insight into the concepts and methods we've implemented, which we'll go through in more depth below. Some of these contributions were produced by us, while others were elaborated by researchers in the field of pedagogical agents. In this context, our agent can be characterized by four capabilities that guide its pedagogical intervention (Figure 4).
attempts in multiple-choice questions, or providing memorable examples.
Fig 6. A Use Case of PAOLE Strategies
-
-
-
IMPLEMENTATION
-
PAOLE Experimentation and Evaluation
In this section, we present the experimentation process that we hav set up and we will give the analysis and interpretation of the data collected.
-
Methodology
Our approach was tested with students attending the second year of the MSI Master, class of 2021. The participants in the experiment consisted of 20 students. The students did not have any pre-requisites or particular problems, it was a homogeneous group made up of students with almost the same educational level, apart from two students who came from a management background.
The students had 8 weeks to complete all the activities offered to them. A first theoretical course was offered face- to-face over a period of 5 weeks. Then, an introductory meeting to the project of the face-to-face online course was organized for a preliminary explanation of the task, its objectives and the importance of the added value for research and for the teaching of technologies of training technologies.
In the rest of the process, the following days were reserved for reading documents, videos, multiple-choice questions and individual work on a project to create an electronic shopping cart.
More explicitly, the experiment focused on the course of E- commerce. The first part or theoretical part is carried out in person. The second part was organized, completely online, through the Moodle platform and the PAOLE agent. As the students had no particular problems with the content, we chose a pedagogy by project, where the learner should work individually and alone in front of his machine, with very little contact with the tutor. The learner must then be motivated and committed to finish his project. The project to create an electronic shopping cart in PHP requires knowledge of PHP and E-commerce. We made this choice of "individual project without help" to test the autonomy, motivation and commitment of learners, but also their perseverance while experimenting with the role of the PAOLE agent in maintaining this motivation.
-
Assumptions Chosen for the Experiment
To achieve the objective of our research, we decided to use a set of hypotheses to focus on some aspects more than others. Thus, as the students did not have any particular problems, we proposed a project-based pedagogy so that the students feel involved in heir earning and to allow them to work independently. Indeed, as presented before, the students had no help or human intervention: the only possible interventions are those of the PAOLE agent.
We ignored a number of variables so as not to detract from the purpose of the experiment. Remember that the variables that can cause dropping out are: (1) demographic variables (age, sex, family situation, etc.), (2) environmental variables (changes in living conditions), (3) institutional variables (course characteristics, supervision, e-learning experience, etc.) and (4) personal characteristics of learners (motivation, cognitive strategies, educational background, etc.).
Regarding demographic variables, we ignored them because the students have almost homogeneous profiles: they are almost the same age, the number of boys and girls is balanced, the students are single, etc. Indeed, a synthesis of studies carried out by Beal and Sauer (1980) notes, for example, that we cannot show the link between the female and male genders and the decision to give up.
For the institutional variables, and in particular related to the course, we chose a simplified course which would correspond to what is done in the MOOCs (readings, videos, multiple- choice questions and a project). For this same variable, there is no guidance to try to create demotivation. The only interventions are those of agent PAOLE. Regarding the e- learning experience, the students had already completed a MOOC on databases the year before.
Finally, for personal characteristics, the issue of initial motivation was not addressed because it is rewarded with a grade. Finally, the monitoring of learners is carried out using trace analysis to try to measure: the quantity and the quality of the efforts invested through the four indicators of BOVO.
-
-
The Tools used in the experiment
-
Data gathering
To measure the effects of the proposed program (Moodle course and PAOLE agent) on student motivation, we used four additional sources of information:
-
The data contained in the student file, data from a MOOC training in Semester S2 allowed us to establish the respective rates of perseverance and dropout;
-
Data from the trace extracted from the Moodle platform, and calculations made by the PAOLE agent concerning the quality and quantity of the efforts made;
-
Data from a plugin that we installed in Moodle to measure the progress of learners;
-
A questionnaire to assess their impression of the course, their motivation, their perseverance, and the quality of the interventions of the PAOLE agent
-
Measures sought
The scarcity of data on the motivation and abandonment of learners in the EAL invites us to wonder about what is actually happening with the phenomenon by integrating a motivation maintenance agent.
This is the question that this experiment addresses, the result of a case-by-case research aimed not only at discovering the proportions of perseverance and dropping out of learners in EAL, but also:
-
To measure the quantity and the quality of the efforts invested by the students, through the five indicators of the Bovo model;
-
Analyze the perseverance and dropout rates of these learners;
-
To identify the impact of a Multimedia agent on maintaining motivation;
-
To validate the importance of the concept of intervention developed.
-
Some Results of Interactions with the System
To measure the effects of the PAOLE agent on student perseverance, we used the trace data contained in the Moodle platform as a source of information. The data we are presenting is spread over 5 weeks of face-to-face lessons and another 8 weeks at a distance. These data result from the raw traces that we have processed. They measure the indicators that have been chosen: the Presence indicator (IPR), the studies indicator (IE), the activities indicator (IA), the results indicator (IR), the social indicator (IS).
Ressources/Activités
Number of Consultations
By number of users
Section 1 – Introduction
Chapitre 1 – Introduction au PHP
39
16
Quiz 1 – Généralités PHP
640
22
Section 2 – Variables
Chapitre 2 – Les variables en PHP
26
13
Quiz 2 – Variables et commentaires
753
22
Quiz 3 – Variables et leurs fonctions
797
22
Ressources/Activités
Number of Consultations
By number of users
Section 1 – Introduction
Chapitre 1 – Introduction au PHP
39
16
Quiz 1 – Généralités PHP
640
22
Section 2 – Variables
Chapitre 2 – Les variables en PHP
26
13
Quiz 2 – Variables et commentaires
753
22
Quiz 3 – Variables et leurs fonctions
797
22
TABLEAU 1: USE OF RESOURCES AND ACTIVITIES BY STUDENTS
Section 3 – Variables
Chapitre 3 – Les instructions de contrôle
25
13
Quiz 4 – Instructions de contrôle
461
20
Section 4 – Variables
Chapitre 4- Gestion des bases de données
x
x
Quiz 5 – Bases de données
1337
23
Devoir – Gestion des bases de données
483
22
TP – Panier électronique (PDF)
60
18
Section 3 – Variables
Chapitre 3 – Les instructions de contrôle
25
13
Quiz 4 – Instructions de contrôle
461
20
Section 4 – Variables
Chapitre 4- Gestion des bases de données
x
x
Quiz 5 – Bases de données
1337
23
Devoir – Gestion des bases de données
483
22
TP – Panier électronique (PDF)
60
18
because the strong interactions concerned the first course of E
-Commerce, presented in hybrid.
Connection frequency
Students
%
No connection (less than 14) 0 0%
Very weak or weak connection (from 15 to 20) 4 20%
Average connection (from 21 to 35) 9 45%
High connection (from 36 to 40) 5 25%
Very high connection (from 41 to 48) 2 10%
Total 20 100%
Connection frequency
Students
%
No connection (less than 14) 0 0%
Very weak or weak connection (from 15 to 20) 4 20%
Average connection (from 21 to 35) 9 45%
High connection (from 36 to 40) 5 25%
Very high connection (from 41 to 48) 2 10%
Total 20 100%
TABLEAU 2: FREQUENCY OF STUDENT CONNECTIONS (INDICATOR PRESENCE)
Table 1: presents the different uses of the resources of the practical work part in PHP on E-commerce. As we have already presented in the previous chapter, the contents are composed of sections, and on each one a textual content, a quiz and videos. As the students already knew the PHP language, we can see that the textual contents are less frequented by the students. The great interactions and viewings were more relevant to the quiz. In chapter 2, quiz 3 recorded 797 visits by 22 users (20 students and two teachers). Quiz 5 which is final, 1337 consultations. In section 4, the project (due date) was opened more than 483 times. This shows the distribution of access to the most popular resource blocks and quizzes. We will see the use of videos below. Figure 7 shows an aspect of the practical work in PHP under Moodle.
Fig 7. An aspect of interactions in the content of practical work in PHP
Regarding the presence (Presence Indicator) of the students on the platform, Table 2 shows the behavior of the students in terms of connections. We can therefore report that over 8 weeks, more than a third of the students (35%) were strongly connected. For medium connections, 45% of participants are counted. Only 20% of students, or 4 students out of a total of 20, are weakly connected.
Table3 shows the frequency of use (Study Indicator) of the different course blocks. These blocks are essential to complete the final project of creating an electronic shopping cart with PHP. This study shows us that 50% of the students interacted with the proposed resources, 40% had an average interaction and 10% (i.e., 2 students) a weak interaction. We can note, however, that the scale of the number of interactions that we took is low, and this is explained by the fact that the students knew the language PHP, but also
TABLEAU 3: FREQUENCY OF INTERACTION WITH RESOURCES (STUDIES INDICATOR)
Frequency of use of blocks (Resources, Activities, Videos)
Students
%
No interaction (less than 5)
0
0%
Very weak or weak Interaction (from 6 to 7)
2
10%
Medium interaction (8 to 10)
8
40%
High and / or very high interaction (from 11 to 12)
10
50%
Total
20
100%
Table 4 shows the distribution of the different consultations and interactions carried out by the students on the QUIZ. This table shows the difference in use of the course blocks and justifies that the QUIZs were placed in the foreground. From there, we can emphasize that the participants optimized their uses by giving more importance to certain blocks. We will come back to this point in the analysis of the final questionnaire.
The effort made indicator presented in Table 5 identifies a combination of factors including, the time spent on the platform, the quantity of returns, the activity participation index and the social participation in the forums. In Table 5, we see the efforts made according to the number of students. Almost two thirds of the participants (high and very high effort), or 60% made very good efforts; 15% made an average effort. Finally, 25% were satisfied with the minimum and this can be explained either by the fact that they knew the language well, or by an optimization of use, which is reflected in the marks obtained in the QUIZ and the final project.
TABLEAU 4: FREQUENCY OF INTERACTION WITH QUIZ (STUDIES INDICATOR)
Quiz
Consultations
%
Quiz 1
640
16%
Quiz 2
653
17%
Quiz 3
800
21%
Quiz 4
463
12%
Quiz 5
1339
34%
Total Consultations
3895
100%
TABLEAU 5: STUDENT EFFORT (EDUCATION INDICATOR)
Effort Made (Resources, Quiz, time spent, connections, forums)
Students
%
Not much effort (less than 40)
0
0%
Very low or low effort (from 41 to 60)
5
25%
Average effort (from 61 to 80)
3
15%
High effort (81 to 100)
3
15%
Very high effort (from 101 to 166)
9
45%
Total
20
100%
-
-
The risk of abandonment
We also tried to seek representation from students about the risk of dropping out. In this sense, item 11 shows that 65% of students thought to quit, 30% answered no and 5% said yes. The risk was there because the students were not used to self- study and autonomy was not sufficiently developed. We recall that during the entire test period the only interventions were those of the PAOLE agent.
Students also tended to leave everything until the end and the regularity of the work was not up to the expected level (Fig 8). Indeed, on item 15, we can note that only 65% of students worked regularly, 20% a little less, and 15% felt a little demotivation and therefore less commitment.
Fig 8: Question on regularity and demotivation
-
Synthesis
The analysis we carried out during this experimentation of a pedagogical agent helps to maintain the motivation of learners within the framework of the EAL, in order to avoid abandonment problems.
The objective was to understand the aspects related to the motivation of learners and their practices in an online environment by analyzing their traces, from the indicators that we have retained, which can be grouped into two main categories: the quality of the efforts made by participants or performance (cognitive engagement), but also the amount of effort made (or behavioral engagement), measured using indicators: online presence, content study, participation in activities, etc.
In this article, we have presented the methodology pursued for the experiment, touching on the services and tools preferred by learners. These preferences concern both the content and the proposed scenario, but also the various interventions of the PAOLE agent.
After analyzing the traces and the questionnaire, we can underline that the risks of abandonment were present, even if we did not identify any. This is due to demotivation mainly due to the independent work offered, as the students were not used to self-study and working in autonomy.
We can also see that the organization of work was essential to finish the requested project. Learners emphasized that their commitment, persistence, effort made and task expectations
were major elements in maintaining motivation and commitment. The interventions offered by the PAOLE agent played an important role in the finish, including advice on organization, gratification and some anecdotal aspects, which were somewhat lacking in the learner profiles we had.
Regarding the interactions, we have shown that different participants were satisfied with the minimum and this is explained either by the fact that they know the PHP language well, or by an optimization of use, which is reflected a little on the marks obtained. For the final project, and not on the QUIZ. Thus, and as it appears clearly on the traces and on the questionnaire, we can underline that the MCQs were one of the preferences of the learners.
We therefore tried to touch on the factors that influence the evolution of the different components of motivation, which we draw up as follows: organization, regularity, commitment, perseverance, efforts made, expectations in relation to task and the presence of an agent. This design gives us a clear idea of the nature of interventions to target for those who are at risk of dropping out due to reduced motivation.
-
-
CONCLUSION
Following the various issues presented, and within the few limits to be respected in this thesis report, we hope to put a first draft of the current problems related to the issue of the abandonment problem in online learning environments. After reviewing the literature, we have given a characterization of different solutions to keep learners motivated. We first examined the interest of the animated educational agents in order to have a presence, to offer rapid assistance and feedback, etc. Other intervention strategies for agents were also specified, such as: encouraging learners, encouraging collaboration in forums, strengthening the observation of learner traces and their analysis in order to determine a better intervention strategy. We applied all these elements to give the first specifications of the PAOLE agent, emphasizing intervention strategies, to increase learner engagement and motivation. The concept of intervention was central throughout the process followed. Indeed, we were able to design an intervention model based on three main questions: Why intervene? When? and how to intervene? We then proposed a more in-depth reflection on the intervention structure as well as on its design using UML language but also a conceptual map summarizing the concept. To our knowledge, this is a personal work that is unique in the literature, which combines both pedagogical aspects and certain behaviors of pedagogical agents. This work drew on the general importance of the concept of intervention, situating it within the larger landscape of learning analysis, examining specific questions of intervention design for EALs communicating with educational agents. We have carried out an implementation of our system and we have experienced it. We have indeed validated the importance of the intervention, by distinguishing the best interventions preferred by learners. It is clear that several problems remain to be solved for the expected system. Our work continues in this direction to try to complete a complete and stable model of the PAOLE Agent, which will be tested and validated across a board spectrum.
REFERENCES
-
Baylor, A. & Kim, Y. (2003). Validating pedagogical agent roles: Expert, Motivator, and Mentor. In D. Lassner & C. McNaught (Eds.), Proceedings of EdMedia: World Conference on Educational Media and Technology 2003 (pp. 463-466). (AACE).
-
Baylor, A. L., & Chang, S. (2002). Pedagogical agents as scaffolds: The role of feedback timing, number of agents, and adaptive feedback. In International Conference of the Learning Sciences, Seattle, WA.
-
Belanger, Y., & Thornton, J. (2013). Bioelectricity: A quantitative approach Duke Univer-sitys first MOOC.
-
Bovo, A., (2014). Apprentissage automatique pour l'assistance au suivi d'étudiants en ligne: approches classique et bio-inspirée (Doctoral dissertation).
-
Capuano, N., Marsella, M. and Salerno, S., (2000). ABITS: An agent based Intelligent Tu-toring System for distance learning. In Proceedings of the International Workshop on Adaptive and Intelligent Web-Based Education Systems, ITS.
-
Cherkaoui, C., et al. (2015). Un modèle d'adaptation dans les environnements d'apprentissage en ligne (LMS et MOOC). (SITA2015), 10ème Conférence internationale sur les systèmes Intelligents. IEEE, 2015
-
Chang, S.H. (2008). Effectiveness of personal interaction in learner – centered paradigm distance education class based on student satisfaction. Journal of Research on Technology in Education . 40 (4).
-
Clarebout, G., Elen, J., Johnson, W. L., & Shaw, E. (2002). Animated pedagogical agents: An opportunity to be grasped?. Journal of Educational multimedia and hypermedia, 11(3), 267-286.
-
Craig, S.D., Driscoll, D.M. and Gholson, B., (2004). Constructing knowledge from dialog in an intelligent tutoring system: Interactive learning, vicarious learning, and pedagogical agents. Journal of Educational Multimedia and Hypermedia, 13(2), p.163.
-
Daradoumis, T., Bassi, R., Xhafa, F. and Caballé, S., 2013, October. A review on massive e-learning (MOOC) design, delivery and assessment. In P2P, Parallel, Grid, Cloud and Internet Computing Conference (3PGCIC), IEEE.
-
Douglas E. Hersh, 2016 – The Human Element https://www.insidehighered.com/news/2010/03/29/lms
-
El Mhouti, A., Nasseh, A., & Erradi, M. (2016). Stimulate Engagement and Motivation in MOOCs Using an Ontologies Based Multi-Agents System. International Journal of Intel-ligent Systems & Applications, 8(4).
-
Frasson, C., Mengelle, T., & Aimeur, E. (1997, August). Using pedagogical agents in a multi-strategic intelligent tutoring system. In Workshop on Pedagogical agents in AI-ED (Vol. 97, pp. 40-47).
-
Galan J-P., Sabadie W. (2001). Evaluation du site Web: une approche par l'expérience de service, 17ème Congrès International de l'Association Française de Marketing, Deauville, 1-26.
-
Garg, V., Tiwari, R. and Gwalior, A.I.(). Hybrid Massive Open Online Course (MOOC) Recommendation System using Machine Learning (2016), In International conference on soft computing techniques in engineering and technology, http://asctet.co.in/papers/OR0033.pdf
-
Graesser, A. C., Lu, S., Jackson, G. T., Mitchell, H. H., Ventura, M., Olney, A., & Lou-werse, M. M. (2004). AutoTutor: A tutor with dialogue in natural language. Behavior Re-search Methods, 36(2), 180-192.
-
Gulz, Agneta. (2004). Benefits of virtual characters in computer based learning environ-mnts: Claims and evidence." International Journal of Artificial Intelligence in Educa-tion 14.3, 4): 313-334.
-
Hill, P. (2013). The most thorough summary (to date) of MOOC completion rates, e-Literate, February 26th..
-
Johnson, W. L., Rickel, J. W., & Lester, J. C. (2000). Animated pedagogical agents: Face-to-face interaction in interactive learning environments. International Journal of Artificial intelligence in education, 11(1), 47-78.
-
k. Bendou, El. Megder and C Cherkaoui. Animated Pedagogical Agents to Assist Learners and to keep them motivated on Online Learning Environments (LMS or MOOC). International Journal of Computer Applications 168(6):46-53, June 2017.
-
Lenoir, Y., (1991). Relations entre interdisciplinarité et intégration des apprentissages dans l'enseignement des programmes d'études du primaire au Québec (Doctoral dissertation, Paris 7).
-
Lin, F.O., (2010). Integrating JADE agents into MOODLE.
-
McCluskey, F., Kupczynski, L., Ice, P., Wiesenmayer, R. (2010). Student Perceptions of the Relationship between Indicators of Teaching Presence and Success in Online Courses. Journal of Interactive Online Learning, 9, 2343.
-
Pesty, S., Webber, C. and Balacheff, N., (2001). Baghera: une architecture multi-agents pour l'apprentissage humain. Agents Logiciels, Cooperation, Apprentissage et Activité Humaine ALCAA, pp.204-214.
-
Rickel, J. and Johnson, W.L., (1999). Animated agents for procedural training in virtual reality: Perception, cognition, and motor control. Applied artificial intelligence, 13(4-5), pp.343-382.
-
Stoilescu, D. (2009). Modalities of using learning objects for intelligent agents in learning. International Journal of Doctoral Studies, 4, 49-64.
-
Suh, H. and Lee, S., (2006). Collaborative learning agent for promoting group interac-tion. ETRI journal, 28(4), pp.461-474.
-
Veletsianos, G. and Russell, G.S., (2013). What do learners and pedagogical agents discuss when given opportunities for open-ended dialogue?. Journal of Educational Computing Research, 48(3), pp.381-401.
-
ZaÃane, O. R. (2002, December). Building a recommender agent for e-learning systems. In Computers in Education, 2002. Proceedings. International Conference on (pp. 55-59). IEEE.