
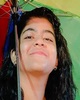
- Open Access
- Authors : Meedinti Gowri Namratha , Sankalp Chauhan , Swarnalatha P
- Paper ID : IJERTV11IS010208
- Volume & Issue : Volume 11, Issue 01 (January 2022)
- Published (First Online): 05-02-2022
- ISSN (Online) : 2278-0181
- Publisher Name : IJERT
- License:
This work is licensed under a Creative Commons Attribution 4.0 International License
Social Media use During Crisis Management, Disaster Response and Recovery Phases
Meedinti Gowri Namratha 1, Sankalp Chauhan 2, Swarnalatha P
Department of Computer Science and Engineering Vellore Institute of Technology,
Vellore
Abstract:- Social media plays a significant role in times of crisis. During disasters, affected people use social media to share their experiences. It enables the public to access information about the ongoing disaster and helps the public contribute to monitor and predict the next phases of the disaster by reporting the latest incidents. However, with the abundance of information available along with the heterogeneity of the information being generated, it becomes difficult to sort the actionable data from the mostly unimportant posts. In this paper, we first give an overview of the current popular social media platforms and their relevant features.
We then included the potential applications for disaster management. Response themes and recovery themes will also be mentioned. With the help of few papers, we also identified how researching on social media data can help during different phases of the crisis. Furthermore, we also proposed a simulation model that can help understand the spread of infectious diseases and conditions of public during disasters that ultimately lead to pandemics and epidemics byharnessing real time data related to the crisis from social media.
KeywordsDisaster management; social media; crisis; epidemic; pandemic;
-
KEYWORDS
Disaster management – the organisation and management of resources and responsibilities for dealing with all the humanitarian aspects of emergencies, in particular preparedness, response and recovery in order to lessen the impact of disasters.
Social media – Social media is a computer-based technology that facilitates the sharing of ideas, thoughts, and information through the building of virtual networks and communities
Crisis – A crisis is any event or period that will lead, or may lead, to an unstable and dangerous situation affecting an individual, group, or all of society. Crises are negative changes in the human or environmental affairs, especially when they occur abruptly, with little or no warning Epidemic – An outbreak of disease that spreads quickly and affects many individuals at the same time : an outbreak of epidemic disease.
Pandemic – A pandemic is basically a global epidemic, an epidemic that spreads to more than one continent,
-
INTRODUCTION
Disaster management has played a critical role in preventing and trying to minimize loss of life, property damage, and infrastructure damage. Intelligent infrastructure for the gathering, integration, management,
and analysis of a range of remote data sources, such as ground-based sensors, video streaming, and satellite imaging, is required for effective disaster management. The rise of social networks and crowd-sourcing has enabled the deployment of human- centric approaches that allow the public to give critical catastrophe-related information that may be utilised to improve disaster management's effectiveness in decreasing natural disaster impact. Geographic information scientists, computer scientists, and domain scientists can use social media data for data analysis since it contains rich information about human activities, environmental conditions, and public mood. Not only does social media generate large amounts of data, but it also generates a diverse range of data kinds, including text, photos, and videos. In 2020, there will be
3.5 billion social media users on the planet, accounting for roughly 45 percent of the global population. As of March 31, 2020, Facebook had over 2.6 billion monthly active users (MAUs) and 1.73 billion daily active users. In 2019, Twitter has 330 million monthly active users (MAUs) and 145 million daily active users (DAUs). Every day, Twitter users send 500 million tweets, which equates to 5787 tweets per second.
Furthermore, as of June 2018, Instagram had 1 billion monthly active users (MAUs), with 500 million daily activeusers updating their stories.
The enormous number of postings made by these active individuals shows the wide range of social media data characteristics. Account IDs, timestamps, user tweets (e.g., words, photos, videos), geolocation, retweets, and so on are all included in Twitter data. As a result of the volume, pace, and variety of data, catastrophe managers are finding it increasingly challenging to extract important and timely information from it.
While existing surveys mostly cover fresh approaches for analysing social media data, the classifications they provide do not accurately reflect the overall perspectives for using social media data in disaster management. Overall, this study presents a new way for comprehending the major components of social media data management and analysis for disaster management concerns, from data sources to social media applications. The key benefit of the taxonomical framework is that it can guide data management activities in the context of using social media data for disaster management.
The work described in this paper focuses on determining how social media data might help with disaster
management. We do so by looking through the literature for strategies for managing and analysing social media data for disaster management. We categorise social media data according to its sources, language, information dimension, data management, analysis, assessment, and application approaches. The goal of this project is to create a useful classification that can be used to improve decision-making by allowing disaster managers to select acceptable data sources and analysis and management approaches.
-
LITERATURE SURVEY
-
Different types of Social Media Users
Messages from different social media accounts have varying attributes and levels of credibility [8]. Official accounts used by government agencies, for example, are more likely to be trustworthy than personal accounts used by the general population. Despite the fact that government agencies in charge of disaster management use social media to communicate catastrophe-related information, they still play a little role in communities. Instead, it is the general population that contributes significantly to information networks during disasters. [20] demonstrated distinct proportions of Twitter users participating in various crisis events, with public users accounting for a significantly higher percentage of participation. To summarise, many categories of social media users play varied roles in disaster management, each giving different context, quality, and reliability of social media data. Government authorities, research/academic institutions, non-governmental organisations (NGO), and the general public are all sorts ofsocial media users in this article.
-
The term "government authority" refers to government agencies that are active in disaster relief and recovery. Theseorganisations, such as the National Disaster Management Authority (NDMA), are authorised to: I disseminate official announcements and actionable warning information to people in disaster-prone areas, and (ii) provide supporting information for disaster management, such as the Geological Survey of India (GSI), the British Geological Survey (BGS), and national meteorological offices.
-
Institutes or research organisations that do disaster management research are referred to as research institutions.
-
Non-Governmental Organization (NGO) defines private-sectororganisations that use social media to disseminate disaster-related information. When compared to information provided by individual users, this user type delivers a higher percentage of information than government agencies and gives a higher quality of information. Save the Hills, Cable News Network (CNN), and Asian News International are examples of non- governmental organisations (NGOs) (ANI).
-
Individuals with personal social media accounts are referred to as "public." By sharing disaster-related
information, this user type contributes the most to social media. When compared to other sorts of users, this category has the largest number of users and hence makes up the largest percentage of information networks.
The majority of research [1-6] rely on data provided by public users, despite the fact that the data may be of dubious quality and reliability. As a result, improving data quality and increasing the accuracy of social media data analysis using social media data preparation procedures (e.g., data filtering, data categorization, and data extraction) proves difficult.
-
-
Social Media Platforms
In general, social media data can be accessed immediately on social media platforms (e.g., Face- book, Twitter, and Instagram). These sites are important data sources for disaster management social media data analytics. For data users to access their social media services, most social media companies provide HTTP-based Application Programming Interfaces (APIs) (e.g., data service and analytics services). Data consumers can interface with the APIs using their tools to acquire and store social media data for their purposes [7]. Twitter, for example, has search APIs that allow users to search for historical or real-time data using keywords or hashtags. APIs to access social media data directly from the social media platforms are used in a lot of studies on leveraging social media data for disaster management [1-5]. The quality and reliability of the obtained social media data become key challenges due to the unstructured properties of social media data and the indeterminacy of the sources [8]. Additional data preparation operations (e.g., data filtering, data classification, and data extraction) are necessary as a result of this. Some social media sites (such as Facebook and Twitter) have implemented data access limitations due to privacy concerns.
-
Third parties
Organizations and institutions also gather and organise social media data for specialised purposes. Because of the advantages of Open Data, several of them have expressed an interest in sharing their social media data with others [8]. Alternative data sources for social media data are evaluated by these organisations. The many methods of acquiring social media data are discussed in this section. Alternative data sources for conducting research on social media data analytics for disaster management include third parties who donate their gathered social media data. This social media data is gathered and organised in a particular way in order to be used for a specific purpose. For example, CrisisLexT26 [21] collects crisis-related tweets from Twitter using crisis- specific keywords during emergency situations. The Figure Eight platform for free open datasets is provided by CrowdFlower [22]. These include tweets about several types of calamities. The majority of social media data acquired by third-party data sources is frequently processed further to provide higher-quality information. In addition to being utilised for disaster management, datasets from third-party data sources can be
used as training datasets and for data analysis in many disaster management studies. On social media, geographic data is expressed in a variety of ways:
-
When users send a message, social media systems attach location information automatically or manually (by users).
-
User-defined: The location is mentioned in the post by theuser, either as a name or as geographic coordinates.
-
Geographic coverage: Many posts merely describe the geographic scope, such as the Pre-even Real-time Post- event: town/village/locality, a district, a country/province,the continent, or similar data.
-
-
Social Media Data
-
Time frame events for social media Categorizing temporal information into three catogories: Pre-event: This refers to the period of time leading up to the event of interest. In general, a social media message released before to the occurrence of an event can be analysed to determine the following: I warningsfor example, a Met-Office bulletin about terrible weather before heavy rain, a cyclone alert, and so onserve as a warning message for an imminent natural disaster. (ii) temporal offsetpre-event posts from social media are analysed to determine the offset between the time of the post and the time of the actual event, such as the time taken after the leaning pole post and the actual landslide in that location.Pre-event social media posts can thus be used to support disaster management's mitigation and readiness phases.
Real-time: This refers to the duration of the occurrence. In the immediate aftermath of an event, social media may be widely used to disseminate information about the incident. In general, real-time social media posts during the occurrence of the event can be analysed for: Firstly, obtaining situational awarenessfor example, "trains cancelled, schools closed in Kerala due to heavy rains," or asocial media post about "roadblock due to landslides on NH-8, Secondly, determining/issuing warnings about the disaster's after-effects/impactsfor example, "high tides are expected in coastal areas after the tremors," and thirdly, response, relief, and recoveryfor example, "shortage of bubble wrap and ready-to-eat items in Sanskrit College Palayam" during the Kerala floods in 2018.
Post-event: This refers to the period of time following the occurrence of the relevant event. Following disasters, social media is frequently used to communicate about needed supplies, missing individuals, death tolls, property losses, government and non-governmental relief activities, preventive measures to be followed while returning home, cash donated by various agencies, and so on. As a result, the post-event data can be used to determine the temporal offset between the time of the post and the time of the actual event, as well as for warnings of future events, deriving information on the event's impact, identifying the relief and recovery measures required, and determining the
temporal offset between the time of the post and the time of the actualevent.
Spatial information can be used in order to implement effective disaster response, management, planning, and mitigation, it is critical to examine public/community behaviour before, during, and after disasters. We may use the time-stamped, geo-tagged data from social media for this purpose because it is the easiest and most prevalent approach to sample public opinion.
Chae et al. [30] describe a temporal analysis of Twitter data connected to Hurricane Sandy, in which they look at the Twitter user density distribution two weeks before and after the incident, as well as for a time period on the day of the event, just after the evacuation order was issued.
Kryvasheyeu et al. [31] conducted a similar study on the spatiotemporal analysis of Twitter data for the same disaster event, finding that the persistence of Twitter activity levels in the time frame immediately preceding the event (post- event) was a good indicator for determining which areas were likely to require the most assistance. Furthermore, normalised activity levels, rates of original content generation, and rates of material rebroadcasting must be taken into account during a disaster to identify the hardest- hit locations in real time. In [32], the number of tweets during the Christchurch earthquakes in New Zealand were analyse across time in a five-minute interval. According to the findings, when an earthquake with a magnitude of 4.2 or more struck at a specific time, it was associated with an increase in the number of tweets within that time period.
Another important thing to consider when deciding on a time frame for collecting social media data is the sort of crisis. We may be able to capture some of the warning signs for disasters like landslides, floods, and storms from social media posts before the actual occurrence of these events, whereas for other events, such as wildfires and earthquakes, relevant or related posts may only surface after the occurrence of these events. Wang et al. [33] analysed wildfire-related tweets from some of the significant wildfires that occurred in San Diego County, USA, in terms of place, time, content, and network by collecting Twitter data from the first wildfire to the date when most of these flames were completely contained.
The temporal evolution of wildfire-related tweets obtained using various keywords, with and without location, revealed the time lag required for information to circulate. Furthermore, as Granell and Ostermann pointed out in [34], the lengths of these events' impacts have an impact on the temporal and contextual variation in the data associated with these events. Real-time and post-event data, for example, can be used for disaster response and recovery, whereas pre-event data can be used for disaster preparation and planning. The authors used time-series decomposition of the data to identify the overall trend and variations with respect to different developmental stages of the event, as well as the cyclical trends of microblogging activity, in a
case study to analyse social media text during and after the 2012 Beijing rainstorm [3].
The categorization of these social media communications into several contextual categories, as well as their analysis over time, aid in identifying the transition between distinct phases of crisis management and promote better decision- making for disaster preparedness, response, and recovery.
[28] presents a classifier based on logistic regression that automatically classifies obtained social media data into multiple topic categories throughout various crisis phases, as well as the temporal patterns of these topic categories in different phases. The experiment with Hurricane Sandy tweets revealed that I tweets about preparedness peaked the day before the event when the emergency declaration was issued, (ii) a large proportion of tweets about impact peaked within a few days of the event's occurrence, and (iii) the largest peak of tweets about disaster recovery peaked five days after the event.
-
-
Language
The language used to make a social media post is referred toas language.
-
The term "global language" refers to English-language social media posts.
-
Social media posts in languages other than English are referred to as "local language."
-
Mixed Language refers to posts on social media that combine two or more languages.
-
Mixed Script refers to social media posts that combine two or more languages in a stylistic or linguistic variant. A Twitter user, for example, might utilise English script to tweet in Hindi.
-
-
Applications
-
Disaster Management Phases
-
In Disaster Management Phases, different stages of a disaster, information can be used to help in disaster management.
-
Mitigationactions taken to reduce the cause and impact of risks, preventing them from becoming disasters.
-
Preparednessaction plans and instructional programmes that help communities deal with unavoidable disasters.
-
Responsethe actions taken to protect people's lives andproperty in the event of a hazard or disaster.
-
Recovery refers to the efforts taken to repair damaged property and community infrastructures, as well as to treat persons who are unwell.
6.2. Disaster Management Types
Types of disaster management relate to categories of disasters that are classified depending on the disaster's root cause. Natural disasters and technical catastrophes are the two main types of disasters, according to the International Disaster Database (EM- DAT)
Natural disasters are events that occur as a result of natural processes or phenomena that can result in the loss of life and property. Biological, geophysical, climatological, hydrological, meteorological, and extraterrestrial disasters are the six sub-groups of natural disasters. Floods, landslides, earthquakes, and tsunamis are examples ofnatural hazards.
Disasters caused by technology processes or human activities are known as technical disasters. Industrial and transportation accidents are two instances of technology disasters.
IV . METHODOLOGY
The methodologies used (in order) are as follows:
Figure 1 : Methodologies
Publication search: Using the list of keywords we looked for publication candidates in many prospective repositories. IEEE Xplore (https://ieeexplore.ieee.org (accessed on 2 September 2020)), ACM Digital Library (https://dl.acm.org (accessed on 2 September 2020)), SpringerLink (https:// link.springer.com (accessed on 2 September 2020)), ScienceDirect (https://www.sciencedirect.com (accessed on 2 September 2020)), and Google Scholar (https:// scholar.google.com (accessed on 2 Furthermore, Google Scholar search results led to the publishing candidates' sources, which included ResearchGate, JMR, ScienceAdvances, PLOS, MDPI, and Tandfonline. The keywords were divided into three categories: Social Media (e.g., Social Media, Social Network, Crowd-sourcing, Twitter, Microblogs), Disaster (e.g., Disaster Management, Emergency Management, Landslide, Earthquake, Flood, Rainfall), and Data Management and Analysis (e.g., Disaster Management, Emergency Management, Landslide, Earthquake, Flood, Rainfall) (e.g., Data Analysis, Data Management, Data Mining).
Publication review: Based on the information supplied in the title, keywords, and abstract of the publications, the search results of articles obtained from repositories and search engines were examined and candidates were chosen. As a consequence, 20-30 publications were chosen as publication candidates from repositories and search engines.
Publication selection: We evaluated the contents of the publication contenders and chose the papers for this survey based on their relevancy. As a result, 20 papers were chosen from a variety of archives and search engines. In addition, significant problems raised in the chosen papers were used.
Methodologies Used for Data Management
Collecting, indexing, storing, and querying social media data for accessibility, reliability, and timeliness are all part of data management for social media. Every day, social media generates a significant amount of data. Facebook, for example, creates roughly four petabytes of data every day, according to. The sheer volume of data presents a huge hurdle in managing social media data, making it a Big Data issue. As a result, social media data management and analysis systems must be able to handle the "four Vs" of Big Data analytics: volume, variety, velocity, and veracity. The state of the art in various systems and research utilising social media analytics for disaster management is presented in this section. We looked at how data is gathered, filtered, pre-processed, localised, stored, indexed, and queried from a data management standpoint.
The data was collected for the evaluation of the framework using the Twitter streaming API. The methodologies of streaming and batch processing were both tested. Because it facilitates the execution of NLP pipelines with millions of documents, the GATE Cloud Paralleliser was employed to execute batch text processing. It performs the pre- processing and transformations required for loading into the GAE pipeline's principal information management system, Mmir (Multi-paradigm Information Management Index and Repository). Text indexing, annotations, and semantics are also supported. The Twitter client is used to grab data from the Twitter streaming API and feed it into a message queue in real-time stream analysis.Separate semantic analysis processors examine and annotate the text before sending it to Mmir, which allows semantic search utilising knowledge embodied in knowledge networks or ontologies. This allows the indexed documents to create semantic relationships, making complicated semantic searches over the indexed dataset much easier. In the Mmir system, GATE Prospector [14] is used to explore and search datasets.
Kim et al. established a conceptual framework [29] for collecting and evaluating social media data. The development, application, and validation of search filters are all part of the framework's strategy. The retrieval precision and recall are also measured. When analysing a significant amount of data, such as social media material, quality assessment in data acquisition is critical. This is especially important in the event of a tragedy. Keyword selection, which includes disambiguations and slang words, is used to construct search filters, and this technique is usually done manually by domain specialists. Standard logical operators like AND, OR, and NOT, as well as data preprocessing techniques like n-gram analysis and proximity operators, are used to create search filters. D- record [19] uses Twitter, Open-StreetMap, and satellite pictures as data sources.
Topic modelling, which was trained using an SVM-based classifier with Synthetic Minority Over-Sampling Technique, was used to expand a list of keywords for a needed notion (SMOTE). Goonetilleke et al. examined numerous open-source and commercial solutions for data collecting, management, and querying for Twitter in "Twitter Analytics: A Big Data Management Perspective" [13], several of which have been utilised in disaster management applications. Wang et al. (2013) [11,12] designed a scalable Cyber Infrastructure-based
Geographic Information System (CyberGIS) for analysing huge amounts of social media content in a natural dis- aster setting. To combine social media data with census data and remote-sensing pictures, the system uses data fusion techniques. Slamet et al. proposed a system architecture [9] for locating a secure place locator (SPL) that includes a system information engineering component.
Because it uses a relational database approach to store and process the data, it entails merging multiple data sources, such as location databases, governmental information, and community information. A case study on emergency knowledge management and social media technologies was conducted by Yates et al. [10]. The study looked into how to use social media and related tools to manage knowledge effectively. It looked at how US government agencies use social media data as an informal information disseminator, as well as how visual information layering aided disaster management.
Due to the variety and complexity of social media content, the use of multimedia data, such as photographs, audio, and video, in extreme event management [26] remains hard. To better understand severe occurrences, new ways for representing and analysing multimedia content are required. To manage the large amount of multimedia data produced by social media, the authors of [24] suggested a unique data model based on a hypergraph structure. To describe the variety and complexity of relationships between multimedia contents, the proposed data model includes three different entities: users, multimedia objects, and annotation objects. This method allows you to combine social media content from several networks into a unified data structure. The influence diffusion algorithm
[25] was presented in this study to look at social media users who have a lot of interactions with a specific social media object.Methodologies Used for Data Analysis
The researchers demonstrated an earthquake event and early warning utilising a social approach in [23]. This was accomplished by combining semantic analysis with Twitter real-time data. They made two main assumptions: that each Twitter user is a sensor, and that each tweet has a time and place linked with it. Positive and negative tweets were classified using semantic analysis. Positive tweets about earthquakes were categorised as positive, while negative tweets about earthquakes were labelled as negative. They also utilised a machine learning approach called support vector machine (SVM) to classify tweets.
In contrast, [13] used Latent Dirichlet Allocation (LDA), a topic modelling technique in the realm of information retrieval. The inherent subject structure of a set of social media communications was extracted using LDA, and the retrieved topic corresponded to an event (e.g., 2011 Virginia earthquake). The authors used an example of subjects and the proportions
of each topic to all messages to demonstrate how seismic occurrences were caught in a small percentage of messages. Meaningful subjects with multiple iterations were discovered using the LDA topic model technique. Abnormal occurrences acquired from extracted topics were rare, and only a small portion of the social media data stream was covered. The scientists employed seasonal trend decomposition based on locally weighted regression (Loess) to detect such aberrant episodes, which they dubbed STL.To confirm the anomalies, the observed anomaly events are compared to other social media data.
[14] employed a candidate retrieval technique to retrieve events from the database. The authors employed feature extraction to extract spatial, temporal, and textual data, and then scored and ranked the documents to determine which one related to which event. The technology employed in this paper for event detection was SVM-based classification.[16] provided an architecture for a public health surveillance mechanism based on SMART-C. The architecture describes the data sources, as well as their modalities, users, and services supplied by the underlying system, in order to improve situational awareness and inform decision-making during all phases of disaster management. The authors discussed the needs for implementing the following services: event classification/grouping, semantic reasoning, position identification, event extraction, speech, text, video, sensor, geographic analysis, reaction planning and production, and alert distribution service. They also discussed security and privacy, as well as event detection and correlation. [30] performed classification and information extraction from Twitter. The authors employed Weka data mining methods and free part-of-speech tagging software for Twitter. They divided the tweets into three categories for classification: personal, instructive, and other. They divided the useful tweets into five categories: I caution and advise, (ii) damage, (iii) donations, (iv) persons, and (v) others. To give a rich set of features in the classifier, they used a Naive Bayesian classifier and utilised unigram, bigram, and part-of-speech (POS) tagging. After a tweet was labelled, a sequence labelling task used conditional random fields to find important information. [27] discusses a participatory sensing-based strategy for mining spatial information of urban emergency occurrences. The researchers used Typhoon Chan-hom as a model for their simulations. They presented a three-layer hierarchical data model: a I social user layer, (ii) crowdsourcing layer, and(iii) spatial information layer. The proposed method collected data related to emergency events in the social user layer. The affirmative samples were collected in the crowdsourcing layer, and the address and Geographic
Information Systems (GIS) data were mined. In this layer, information about the same emergency incidents was grouped together. The spatial information of the emergency incident was mined in the spatial information layer.
Semantic analysis of the geo-tagged microblog data aided in obtaining a public opinion from a spatial standpoint, and assistance could be provided when needed. According to the statistics gathered, the risk was greatest in Beijing, Zhejiang, Jiangsu, and Shanghai.The authors suggested a probabilistic framework for determining a Twitter user's location based on the content of their tweet in [17]. The authors utilised a simple cart classifier to identify tweets with strong geo-scope, and then refined the user location using a lattice-based neighbourhood smoothing model. They also demonstrated that when the number of tweets increases, the location estimation algorithm converges. To overcome the problem of profiling users' home locations on Twitter, the authors of [18] suggested a unified discriminative influence model. To profile user location, they adopted probabilistic approaches for local and worldwide prediction. Local-prediction-based Methodology profiling employs the user's friends, follows, and tweets to accurately profile the user's location, whereas global prediction uses unlabeled persons as well. Text sentences are vectorised in D-record
[19] to capture their semantics. The text is pre-processed by stemming, case folding, and deleting loud words before being featured. Data Analysis and Management Term Frequency-Inverse Document Frequency (TF-IDF) vectors and gensim's word2vec embedding were used to evaluate lexical elements using an SVM classifier using a lexicon- based feature, TF-IDF vectors, and gensim's word2vec embedding.V. RESULTS AND DISCUSSIONS During natural disasters, social media may help with emergency response and provide a complete picture of situational awareness both during and after the crisis. Obtaining and extracting hazard-related information from social media presents various obstacles, including volume, unstructured data sources, signal-to-noise ratio, ungrammatical and multilingual data, and detecting and removing false messages. Because of the large numbers and variety of data generated by social media, several levels of information can be pulled from it. For example, a tweet about a roadblock on a mountainous route with geo- taggingprovides more contextual information than a similar tweet without geo-tagging. A tweet with photographs attached, on the other hand, may potentially provide additional situational awareness. A tweet with photographs of a roadblock on a mountainous road, for example, can enable those travelling close comprehend the present state of the roadblock and move to a new route away from the stopped area. Due to the volume and complexity of such vast volumes of social media data, it is critical to have tools and systems that can automatically identify and extract information, allowing people attempting to manage the situation to turn data into useful, actionable information.
This data must be consistently handled and made available on demand, as well as queried using various query conditions. The geo-location/geo-fence, keywords and their disambiguations, user type (e.g., government, non- governmental organisations (NGOs), news agencies, public, etc. ), and message type (e.g., warning, news, SOS, request for supplies, or general posts/tweets about an ongoing or impending situation) are the main dimensions of a query. As a result, the knowledge represented in an ontology enables machines to perform intelligent tasks such as interactively communicating with social media users to extract contextualinformation related to an event of interest, identifying the relationship between this information and the hazard of interest, and providing this information to the decision- maker as a complete picture to enable informed decision- making. As a result, an ontology-based strategy is more sophisticated than traditional data management systems, because it integrates the data model with associated domain knowledge that can be processed by machines to provide semantically rich and meaningful data [12]. Systematic extraction of significant information and semantic significance from free language in social media will aid in the development of intelligent systems capable of organising and presenting material in a usable fashion.
Natural language processing (NLP) is a critical technique for deciphering and extracting information from user- generated text content. Several natural language processing approaches and case studies were examined [1315].
Aspects derived from research
The sources of social media data and ancillary data provided by other sources (e.g., physical sensors, wireless sensor networks (WSNs), and web services) that are utilised to facilitate social media data analysis in disaster management
are referred to as data sources. Based on the similar qualities of data sources indicated in the selected papers, we divide the dimension of data sources into four sub-classes: Sensor, Social Media User, Social Media Platform, and Third Party. Social Media User class is divided into four categories: Government Authorities, Research/Academic Institutions, Non-Governmental Organizations (NGOs), and the General Public.
Figure 2 : Social Media User class
-
Information dimensionIn social media material, there are two fundamental dimensions of information: spatial and temporal. This category also includes approaches for extracting spatial and temporal information from social media content. The spatial dimension refers to how geographical information is represented in social media,
as well as the methodology for identifying and analysing geo-locations. Geo-tagging, User-defined, and Spatial Coverage are some of the ways the geographic data is presented. The use of temporal information representing disaster-related occurrences in the existing system for event detection is referred to as the temporal dimension. Based on the temporal categories of real occurrences, the temporal dimension is further divided as Pre-event, Real-time, and Post-event.
-
Languagethis relates to the language used to make a social media post. Global Language, Local Language, Mixed Language, and Mixed Script are the four types of language. English is also a common language for mixed- language social media posts. Furthermore, according to some study, social media posts in numerous local languages can be found. Understanding text-based information created by social media has gotten more difficult as a result of this variation. Natural language processing (NLP) has played a critical role in interpreting and extracting relevant information from text data and facilitating disaster management in this case.
Figure 3 : Language used to make asocial media posts
-
The approaches and algorithms used to analyse social media data, particularly the spatial and temporal information, are referred to as methodology. Methodologies for Data Management and Methodologies for Data Analysis are two types of methodologies that we categorise based on data analysis stages. Each methodology's evaluations are also summarised. There are two types of disasters, according to The Emergency Events Database (EM-DAT) , natural disasters and technological disasters, with several types of disasters within each of these groups.The disaster management phase denotes the step in the disaster management life cycle that social media applications contribute to, whereas the disaster management type denotes the kind of disaster apps. We analyse the present coverage of existing social media disaster management
applications and the overall picture of existing applications based onthese aspects.
Figure 4 : Two types of methodologies categorised based on data analysis stages
-
Applicationrefers to how social media data is now being used for disaster management, which is divided into two categories: Disaster Management Phases and Disaster Management Types, were also discussed. The Natural Disaster Disaster Management type falls under all the disaster management phases – Prepardness,
Response and recovery. While the technological type shall fall under preparedness and response phase alone. The authors Aulov, O., and Halem proposed a novel approach to using social media data as a human sensor to monitor technological disasters (oil sightings) and natural disasters (earthquakes and air quality). Geo-locations were extracted and used to anticipate an oil spill as a boundary. Guan, X.; Chen, C.; Chae, J.; Thom, D.; Jang, Y.; Kim, S.; Ertl, T.; Ebert, D.S. analysed Twitter data to discover public behaviour patterns during Hurricane Sandy from both spatial and temporal perspectives.
Wang, Y.; Wang, T.; Ye, X.; Zhu, J.; Lee, J. investigated the spread of emergency information using social media during an emergency incident. Using classification and location methods, this study examined the social media stream during the 2012 Beijing downpour. The majority of study has concentrated on the use of social media data for natural catastrophes rather than technical disasters, as can be observed. Most of these papers, however, propose techniques that can be used to many phases of crisis management. Surprisingly, the reaction phase is the most common way to use social media data, despite the fact that there are no publications that apply to the mitigationphase.
Figure 5 : Ways Social media data can be used for disastermanagement
-
THE PROPOSED SMS (SYSTEMATIC MAPPING STUDY)MODEL
Based on the survey and other findings, currently, two related research branches, social media mining and computational epidemiology, are working on the problem. To mimic the transmission of the flu, computational epidemiology models often use a social contact network. Each person in such a system will be allocated
geographical, social, behavioural, and demographic (e.g., age and wealth) traits [Bisset et al., 2009]. And each node (person) in the network is assigned daily activities and locations to replicate the social contact network [Bisset et al., 2009; Barrett et al., 2009]. The epidemic dynamics are then modelled as diffusion processes over the network, allowing all individuals' infectious time and location to be computed.
SNS (Social Networking Sites) Space
D = U uU ,tT Du,t is the formula for social media data. Du,t denotes the position of user u at time t. It's worth noting that several posts by user u during time interval t are combined into a single document. Each person is supposed to be in one of four states in the well-known SEIR model: susceptible (S), exposed (E), infectious (I), and recovered
-
(R). In general, the individual is asymptomatic in the susceptible (S) and recovered (R) states, infected but not yet infectious in the exposed (E) state, and has severe symptoms in the infectious (I) state. Because no symptoms are evident in state S and R, social media users will not publish anything relating to disease.
As a result, our research considers that a user is in one of three health states: healthy (S and R of SEIR), exposed (E), or infectious (I) (I).
Through a carefully designed Bayesian graphical model, the SMS model learns health status from social media data. In our model for social media posts, there are three steps to the creative process of words. First, the health status s is chosen from a multinomial distribution of per-document health statuses with prior: s = 0 denotes that the user of the corresponding post is healthy/ safe. s = 1 denotes that the user has been exposed but not confirmed as infected/ in danger zone (e.g., I am feeling weird"); and s = 2 denotes that the user has been infected/ danger. (e.g., house has been flooded ). Second, after selecting a s value, topic z is selected from a K-dimensional topic mixture s. Unlike other topic models, each document in this one is linked to S topic distributions.Based on the retrieved subjects, this technique allows for the prediction of health/safe state.
Generation process of words in SNS space
for each label s = 1,2,…,S dofor each topic z = 1,2,…,K do Draw s,z Dir(); endend
for each time stamp t = 1,2,…,T do
for each document Du,t = 1, 2, …, U do Draw µu,t Dir() ;
for each label s = 1,2,…,S doDraw u,t,s Dir() ; end for each word w in document Du,t do Draw s Multi(µu,t) ;
Draw z Multi(u,t,s) ;Draw w Multi(s,z) ; end endend
Simulation Space
The simulation space is a contact network G = (V, E, W), where V represents the targeted population, E represents
the edge set, and W represents the edge weights. In the network, node v1 V symbolises an individual who has a contact with another individual v2 via edge (v1,v2) E and has a contact time of w. (v1,v2). Person v2 can be infected/person in danger by person v1 in the contact network G with probability p(w(v1,v2),), where is the transmission probability per contact time unit. We assume that each person v in the simulation environment is connected with three states: healthy (S and R), exposed (E), and infectious (I), similar to the health status of social media users (I). The exposure time pE (v) and the infectious period pI (v) reflect the duration of person v's exposed and infectious condition, respectively.
The hidden health states computed by the simulation should be consistent with those derived from social media to decrease the inconsistency between social media and simulation space. Although mapping each person v in the simulation space to a single user u in the social media space is unfeasible, connecting the two spaces at the population level is practical and sufficient for our goal. We compare social media users to simulated people from the same region (for example, counties or states), which may be formalised using the following loss function:
The total infectious state of simulation results at time t is Iv,t(G,pE,pI,), while the associated incubation state is Ev,t(G,pE,pI,). The transmission probability is the parameter that must be maximised in order to attain the bestresults.
Interaction between the two spaces
Finding a way to combine individual-level social media posteriors into population-level characteristics is crucial to knowledge transmission from social media space to simulation space. pE and pI are input parameters required by the simulation space in the loss function. For each individual v, the specific incubation period pE(v) and infectious period pI(v) can be seen as observations from international distributions multi(pE) and multi(pI) (pI ).
Although linking each user u in social media space to each individual v in simulation space is unfeasible, the estimation based on population-level is sufficient for our goal. The expectation of social media users' incubation period ntE /|U
|, where ntE specifies the number of users whose incubation period is equal to t days, yields the maximum likelihood solutions for pE.
In a similar way, the estimation of parameter p can be done using IMSM.
The simulation outputs, on the other hand, can be used to increase learning performance in the SNS space. On the one hand, optimal values for Dirich let prior s of healthy status s in the social media arena should reflect the population's health state. The simulation outputs, on the
other hand, include information about the population's health. Two transition parameters, the incubation rate t,e and the infectious rate t,i, are defined to represent the proportion of exposed and infectious people in the population, respectively. These values are calculated as shown in thefollowing equations:
where E (G,p ,p ,) and I (G,p ,p ,) are the simulation space outputs v,tEI v,tEI, as described in the first Equation. The following is the gamma prior for a Dirichlet parameter of healthy/safe state s (s can be e or I at epoch t:
t,s Gamma(t,s, ),
where the mean is proportional to the simulation output parameter t,s, and the prior consistency is controlled by parameter.
-
-
ANALYZING A TWITTER DAA SET
We used a Covid-19 tweets dataset to derive the following results.
Figure 5.2.1: Categorizing a few missed objects in the columns of the dataset
Figure 5.2.2: Most popular users
Figure 5.2.3: Most popular user locations
Figure 5.2.7: Tweets distributions over the hours
Figure 5.2.4: Year Twitter accounts were created
Figure 5.2.5: Top 40 most popular locations by the number of tweets
Figure 5.2.6: Most popular users
Figure 5.2.8: Tweets distributions over the days
Figure 5.2.7: Length of the Tweets (in number of words)
-
USING THE SIR MODEL TO ANALYZE THE SCENARIO INITALY
SIR model is a simple mathematical model to understand outbreak of infectious diseases.
-
S: Susceptible (= Population – Confirmed)
-
I: Infected (=Confirmed – Recovered – Fatal)
-
R: Recovered or Fatal (= Recovered + Fatal)
Though R in SIR model is "Recovered and have immunity", I defined "R as Recovered or fatal". This is because mortality rate cannot be ignored in the real COVID-19 data.
Model:
: Effective contact rate [1/min]
: Recovery(+Mortality) rate [1/min]
Ordinary Differential Equation (ODE):
where
=++ is the total population,
is the elapsed time from the start date.
Non-dimensional SIR model
To simplify the model, we will remove the units of the variables from ODE.
This results in the ODE
is the total population and
is a coefficient ([min], is an integer to simplify).
The range of variables and parameters:
Basic reproduction number, Non-dimentional parameter, is defined as
where R0( R naught") means "the average number of secondary infections caused by an infected host" .
Scenario Analysis in Italy
Performing scenario analysis using the records of Italy:
Phases in Italy
We will use the change points as the start date of phases. For each phase, will apply SIR-F model.
values will be the same value.
Analyzing the effect of two parameters Effect of school closure and lockdown
According to first report of COVID-19 Mobility Monitoring project on 13Mar2020, the government of Italy declared a national lockdown on 09Mar2020 and all peole are asked to remain home. This resulted in average reduction of potential encounters of 19% during week 3 (from 07Mar2020 to 10Mar2020).
Here, we will predict the effect of school closure (started
before 04Mar2020), lockdown on 13Mar2020 with assumption that the effect will be shown from the start date of 3rd phase.
Predicting the future with the last parameters:
In a week,
In a month,
In long term,
Effect of expected new medicines
New drugs are required so that people can recover from their illnesses more quickly. COVID-19 medications are being developed using a drug repositioning technique (i.e., discovering effective candidates from a library of current pharmaceuticals for various disorders). Remdesivir (USAN) and Favipiravir (AVIGAN) are two examples of potential options.
-
KEGG DRUG DATABASE: Remdesivir (USAN), Antiviral.
-
KEGG DRUG DATABASE: Favipiravir (AVIGAN), efficancy is Antiviral, RNA replicase
-
inhibitor.
Favipiravir (AVIGAN) can cause a variety of dangerous side effects, and it should not be given to expecting mothers, according to the KEGG database (Sorry, this is written in Japanese). However, it has the potential to save tens of thousands of lives.
Remdesivir will be studied in an uncontrolled clinical trial from January 25th to March 7th, 2020.
According to Grein, Jonathan, et al., 2020, a 10-day Remdesivir regimen (200 mg on day 1 followed by 100 mg daily) was completed with a median follow-up of 18 days.
The whole study set included 53 individuals who had a confirmed illness and had an oxygen saturation of 94% or less when inhaling ambient air or receiving oxygen supplementation. They come from the United States (22 patients), Europe/Canada (22 patients), and Japan (22 patients) (9 patients). Clinical improvement was seen in 36 individuals (68 percent). 25 patients (47%) were discharged,
while 7 patients (13%) died.
For evaluation, a clinical experiment with placebo is
required, although we can assume and
as follows. (This estimate isn't included in the report.) This is only a rough estimate.)
In 90 days,
In long-term,
VII. CONCLUSION
In this study, we looked at research papers to see what role social media data plays in disaster management, as well as data management and analytic strategies. Based on our proposed taxonomy, which comprises data sources, languages, spatial and temporal information, techniques, and applications, we investigated the many dimensions of the contributions. Human-centric initiatives (such as social media, blogs, and crowdsourcing) have emerged as important data sources for observing real-world occurrences and aiding catastrophe management. Several articles have suggested using social media data for disaster management, with Twitter being one of the most popular social media data sources. The temporal and spatial data retrieved from Twitter is crucial for disaster management decision- making.Geo-location identification and analysis are major research concerns in catastrophe management from a spatial perspective. Despite the fact that a number of techniques have been offered in the literature, these issues remain unsolved. However, social media content, as well as temporal data such as posting and event times, can be used to aid disaster management in a variety of ways. Many studies have used this type of data to detect precursor occurrences or to aid decision-making during disasters. In addition, the literature has recommended numerous ways for managing, analysing, and assessing social media data.
Due to the large volume of created social media data, Big Data technology is clearly a significant technology for social media data management. Furthermore, machine learning and information retrieval methods are routinely utilised to collect, classify, and extract critical data from social media. Finally, evidence from this survey's application perspective has demonstrated that social media plays a key role in every phase of disaster management, and the data generated has been extensively employed in such management.
Figure 6 : Pictorial representation of the proposed model
VII. REFERENCES
-
Yin, J.; Lampert, A.; Cameron, M.; Robinson, B.; Power, R. Using Social Media to Enhance Emergency Situation Awareness. IEEE Intell. Syst. 2012, 27, 5259. [CrossRef]
-
Rogstadius, J.; Vukovic, M.; Teixeira, C.A.; Kostakos, V.; Karapanos, E.; Laredo, J.A. CrisisTracker: Crowdsourced social media curation for disaster awareness. IBM J. Res. Dev. 2013, 57, 4:14:13.[CrossRef]
-
Middleton, S.E.; Middleton, L.; Modafferi, S. Real-Time Crisis Mapping of Natural Disasters Using Social Media. IEEE Intell. Syst. 2014, 29, 917.
-
Kuriakose, S.L.; Sankar, G.; Muraleedharan, C. History of landslide susceptibility and a chorology of landslide-prone areas in the Western Ghats of Kerala, India. Environ. Geol. 2009, 57, 1553 1568.
-
Olteanu, A.; Castillo, C.; Diaz, F.; Vieweg, S. CrisisLex: A Lexicon for Collecting and Filtering Microblogged Communications in Crises. In Proceedings of the Eighth International Conference on Weblogs and Social Media (ICWSM), Ann Arbor, MJ, USA, 14 June 2014.
-
To, H.; Agrawal, S.; Kim, S.H.; Shahabi, C. On Identifying Disaster- Related Tweets: Matching-based or Learning-based? In Proceedings of the 2017 IEEE Third International Conference on Multimedia Big Data (BigMM), Laguna Hills, CA, USA, 1921 April 2017; pp. 330337.
-
Lomborg, S.; Bechmann, A. Using APIs for Data Collection on SocialMedia. Inf. Soc. 2014, 30, 256265. [CrssRef]
-
Immonen, A.; Pääkkönen, P.; Ovaska, E. Evaluating the Quality of Social Media Data in Big Data Architecture. IEEE Access 2015, 3, 20282043. [CrossRef]
-
Slamet, C.; Rahman, A.; Sutedi, A.; Darmalaksana, W.; Ramdhani, M.A.; Maylawati, D.S. Social Media-Based Identifier for Natural Disaster. In Proceedings of the IOP Conference Series: Materials Science and Engineering 2018, Kuala Lumpur, Malaysia, 1314 August 2018.
-
Yates, D.; Paquette, S. Emergency knowledge management and social media technologies: A case study of the 2010 Haitian earthquake. Int. J. Inf. Manag. 2010, 31, 613. [CrossRef]
-
Wang, S.; Anselin, L.; Bhaduri, B.; Crosby, C.; Goodchild, M.F.; Liu, Y.; Nyerges, T.L. CyberGIS software: A synthetic review and integration roadmap. Int. J. Geogr. Inf. Sci. 2013, 27, 21222145. [CrossRef]
-
Wang, S. A CyberGIS framework for the synthesis of cyberinfrastructure, GIS, and spatial analysis. Ann. Assoc. Am. Geogr. 2010, 100, 535557. [CrossRef]
-
Chae, J.; Thom, D.; Bosch, H.; Jang, Y.; Maciejewski, R.; Ebert, D.S.; Ertl, T. Spatiotemporal social media analytics for abnormal event detection and examination using seasonal-trend decomposition. In Proceedings of the Visual Analytics Science and Technology (VAST), Seattle, WA, USA, 1419 October 2012; pp. 143152.
-
Reuter, T.; Cimiano, P. Event-based classification of social media streams. In Proceedings of the 2nd ACM International Conference onMultimedia Retrieval, Hong Kong, China, 1 June 2012; p. 22.
-
Becker, H.; Naaman, M.; Gravano, L. Learning similarity metrics for event identification in social media. In Proceedings of the Third ACM International Conference on Web Search and Data Mining, New York,NY, USA, 36 February 2010; pp. 291300.
-
Adam, N.R.; Shafiq, B.; Staffin, R. Spatial computing and social media in the context of disaster management. IEEE Intell. Syst. 2012, 27, 90 96. [CrossRef]
-
Cheng, Z.; Caverlee, J.; Lee, K. You are where you tweet: A content- based approach to geo-locating twitter users. In Proceedings of the 19th ACM International Conference on Information and Knowledge Management, Toronto, ON, Canada, 2630 October 2010; pp. 759768.
-
Li, R.; Wang, S.; Deng, H.; Wang, R.; Chang, K.C.C. Towards social user profiling: Unified and discriminative influence model for inferring home locations. In Proceedings of the 18th ACM SIGKDD International conference on Knowledge Discovery and Data Mining, Beijing, China, 1216 August 2012; pp. 10231031.
-
Kar, S.; Al-Olimat, H.S.; Thirunarayan, K.; Shalin, V.; Sheth, A.; Parthasarathy, S. D-record: Disaster Response and Relief Coordination Pipeline. In Proceedings of the ACM SIGSPATIAL International Workshop on Advances in Resilient and Intelligent Cities (ARIC) 2018, Seattle, WA, USA, 69 November 2018.
-
Steinberg, A.; Wukich, C.; Wu, H. Central Social Media Actors in Disaster Information Networks. Int. J. Mass Emerg. Disasters 2016, 34, 4774.
-
Olteanu, A.; Castillo, C.; Diaz, F.; Vieweg, S. CrisisLex: A Lexicon for Collecting and Filtering Microblogged Communications in Crises. In Proceedings of the Eighth International Conference on Weblogs and Social Media (ICWSM), Ann Arbor, MJ, USA, 14 June 2014.
-
Eight, F. Data For Everyone. Available online: https://www.figure- eight.com/data-for-everyone/ (accessed on 22 January 2019).
-
Sakaki, T.; Okazaki, M.; Matsuo, Y. Tweet analysis for real-time event detection and earthquake reporting system development. IEEE Trans. Knowl. Data Eng. 2013, 25, 919931. [CrossRef]
-
Amato, F.; Moscato, V.; Picariello, A.; SperlÃ, G. Multimedia Social Network Modeling: A Proposal. In Proceedings of the 2016 IEEE Tenth International Conference on Semantic Computing (ICSC), Laguna Hills,CA, USA, 46 February 2016; pp. 448453.
-
Amato, F.; Moscato, V.; Picariello, A.; SperlÃ, G. Diffusion Algorithms in Multimedia Social Networks: A Preliminary Model. In Proceedings of the 2017 IEEE/ACM International Conference on Advances in Social Networks Analysis and Mining, Sydney, Australia, 31 July3 August 2017; pp. 844851.
-
Amato, F.; Moscato, V.; Picariello, A.; Sperliì, G. Extreme events management using multimedia social networks. Future Gener. Comput. Syst. 2019, 94, 444452. [CrossRef]
-
Xu, Z.; Zhang, H.; Sugumaran, V.; Choo, K.K.R.; Mei, L.; Zhu, Y. Participatory sensing-based semantic and spatial analysis of urban emergency events using mobile social media. EURASIP J. Wirel. Commun. Netw. 2016, 2016, 28. [CrossRef]
-
Huang, Q.; Xiao, Y. Geographic situational awareness: Mining tweets for disaster preparedness, emergency response, impact, and recovery. ISPRS Int. J. Geo. Inf. 2015, 4, 15491568. [CrossRef]
-
Kim, Y.; Huang, J.; Emery, S. Garbage in, garbage out: Data collection, quality assessment and reporting standards for social media data use in health research, infodemiology and digital disease detection. J. Med Internet Res. 2016, 18, e41. [CrossRef] [PubMed]
-
Chae, J.; Thom, D.; Jang, Y.; Kim, S.; Ertl, T.; Ebert, D.S. Public behavior response analysis in disaster events utilizing visual analytics of microblog data. Comput. Graph. 2014, 38, 5160. [CrossRef]
-
Kryvasheyeu, Y.; Chen, H.; Obradovich, N.; Moro, E.; Van Hentenryck, P.; Fowler, J.; Cebrian, M. Rapid assessment of disaster damage using social media activity. Sci. Adv. 2016, 2, e1500779. [CrossRef] [PubMed]
-
Yin, J.; Karimi, S.; Lampert, A.; Cameron, M.; Robinson, B.; Power,
R. Using social media to enhance emergency situation awareness. In Proceedings of the Twenty-Fourth International Joint Conference on Artificial Intelligence, Buenos Aires, Argentina, 2531 July 2015.
-
Wang, Z.; Ye, X.; Tsou, M.H. Spatial, temporal, and content analysis of Twitter for wildfire hazards. Nat. Hazards 2016, 83, 523540. [CrossRef]
-
Granell, C.; Ostermann, F.O. Beyond data collection: Objectives and methods of research using VGI and geo-social media for disaster management. Comput. Environ. Urban Syst. 2016, 59, 231243. [CrossRef]
AUTHORS PROFILE
1. Meedinti Gowri Namratha- Vellore Institute of Technology, Vellore; Computer Science with Business Systems
2. Sankalp Chauhan – Vellore Institute of Technology, Vellore; Computer Science with Business Systems