
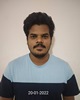
- Open Access
- Authors : Yugandhar Neelapu , K. Venkata Subbaiah
- Paper ID : IJERTV11IS030100
- Volume & Issue : Volume 11, Issue 03 (March 2022)
- Published (First Online): 24-03-2022
- ISSN (Online) : 2278-0181
- Publisher Name : IJERT
- License:
This work is licensed under a Creative Commons Attribution 4.0 International License
Application of Kansei and Fuzzy Analytical Hierarchy Process (FAHP) to Determine the Most Effective Minicar Model
Yugandhar Neelapu1
Department of Mechanical Engineering, A.U college of Engineering, Andhra University, 530003 Visakhapatnam, India
Prof. K. Venkata Subbaiap
Department of Mechanical Engineering, A.U college of Engineering, Andhra University, 530003 Visakhapatnam, India
Abstract Today's product development trends imply that buyers will struggle to differentiate between a large number of items owing to functional equivalence. As a result, customers' choices will be influenced by more subjective variables. Additionally, in the future, items will be composed of a physical and ethereal component. Kansei Engineering is a technology that converts client sentiments into tangible product specifications and serves as a guide for future product design. Kansei is a strategy in which a product attributes vocabulary is accessible; the appropriate characteristics may then be selected from the vocabulary. These terms may be classified as grouped or clustered. This grouping approach is based on factor analysis. On this factor, Multiple-criteria decision-making (MCDM) and Fuzzy Analytical Hierarchy Process (FAHP) approaches are used to create a hierarchical structure and determine the component's relative relevance. On the basis of the Kansei approach and FAHP, 18 criteria are identified for examination and rated in order of importance.
Keywords Kansei; MCDM; FAHP;
-
INTRODUCTION
Most of the world's well-known multinational vehicle firms operate across the globe, and their consumers come from many nations with diverse perspectives and lifestyles. What Americans consider to be the ideal vehicle might be inappropriate for Asians. In other words, globally running automotive firms would not know what are trends in the industry or what may be improved or added to the vehicle if consumer concerns were not taken into account. When car companies act as customers of a supplier company, the added value for the customers is that they get a good product because they work together, "two brains are better than one," the quality is better, the cost price is lower, and the development and production process lead time may be shortened. When it comes to suppliers, they learn about their customers' reliance on them and get fresh ideas for certain items. Car businesses bring in their ideas and collaborate with suppliers, allowing us to be more innovative. When clients are pleased with a supplier's work, they spread the word to other businesses, expanding the supplier's member channel.
This demonstrates that the customer-producer relationship is based on active involvement rather than passive contact. And it adds value to the product development process every time by following this active contact.
The goal of this study is to find out why consumer engagement in the automotive development process is so vital.
Customer interaction was discovered to be a vital aspect of the automotive development process. Customers' suggestions and concerns, which are assessed by marketers and sent to engineers for further development, are what bring value to the car business. Of course, the kind of consumer matters: in the case of light automobiles, where the customer's emotions determine the product, a car expert's ideas and views are more valuable than an average outsider. In this situation, the customer's choice is influenced by both brains and technology. The stage of the business when the client is generally involved is the earlier stage (market research only). Car makers, for example, display their concept vehicles to end consumers at exhibitions or road shows and then seek input from them. We may witness active interactions between the client and the corporation in this manner. Naturally, this provides value for both buyers and producers. The additional value is that the client contributes to making the product better, more useful, and marketable, as well as sometimes assisting car technology. The insights gained through consumer contact and involvement, continual feedback, customer pleasure, and, most importantly, customer devotion to the automobile brand contribute value to
the firm.
-
Customer Integration
Customer participation is most often employed in the early phases of product development when organisations are looking for fresh ideas and concepts to include into an existing product. Many businesses operate a programme to find inventive consumers and innovators from their marketing department or other divisions to introduce a fresh wave into research activity. It is insufficient to communicate with customers just via the website or other tools. It's often helpful to meet a client face to face to hear and see what he's thinking about your product, as well as what responses and emotions he's displaying on his face when he's talking about it.
-
Development Process
Several manufacturers have slightly varied development cycles for designing an automobile, but in practice, these are the following:
-
Design and consumer research
-
Concept development sketching
-
CAS (Computer Aided Styling)
-
Clay Modeling
-
Interior buck model
-
Vehicle ergonomics
-
Class-A Surface Development
-
Colour and trim
-
Vehicle graphics
Other product engineers will be engineering the styling data to fulfil performance, manufacturing, and safety requirements simultaneously with the design process. Back and forth discussions between designers and product engineers begin in the middle phase and conclude in a final product that is production ready.
Aside from that, the engineering team is working on the following projects at the same time. Product Engineering (Body in White Sheetmetal Design and Plastic Engineering), NVH Development Team, Prototype Development, Powertrain Engineering, Physical Vehicle Validation, Tool and Die Development, Manufacturing Process Design.
-
-
Development Team
A head designer, as well as an exterior and interior designer, make up the design team for a certain model. In certain circumstances, a single designer is responsible for all three responsibilities. A number of junior designers are also engaged in the development process, making particular contributions that are all monitored by the chief designer. Aside from that, the colour and trim designer collaborates closely with the rest of the team. The clay model team and digital model team, all of whom are based in the studio, collaborate closely with the styling team. Apart from that, the studio would have studio heads, studio managers, and prototype engineers who would collaborate with all of the studio's teams. The complete team size for designing a whole automobile typically runs from 25 to 40 persons, and the development process takes more than 24 months from start to finish, including tooling and production approval. Following that, a smaller crew would labour until the vehicle was launched.
-
Definition of Quality
Quality comes from the Latin term 'qualitas,' which meaning "of what." It denotes the quality or character of things in general (Cicero). The notion of quality in a technical environment varies depending on the situation and time:
The ISO 9000 definition establishes a clear link between product quality and its properties:
Quality is defined as "the sum of a product' or activity's traits and attributes that pertain to its appropriateness to meet specified standards."
Even more affective-oriented definitions appear, revealing links to the study of ergonomics: "A product/service is of quality when it contributes to the health and pleasure of all persons engaged in its manufacture, use, destruction, and reuse to the greatest extent possible" (Aune 1999).
Tribus describes quality in even more emotional terms: "Quality is what allows a consumer to fall in love with your product or service." Love is a fickle beast. You must always be on the lookout for what pleases the consumer, since quality is defined only by the customer." (1990, Tribus).
Focusing on product development processes two a aspects of the above presented definitions are of importance:
-
Affective aspects (emotional impact of the new product)
-
Design aspects (product properties)
Affective impact on the user is consequently a result of the composition of the different product properties. Hence, the goal must be to choose the best combination possible in order to maximize the customer satisfaction. Figure 2 lists some quality dimensions of an article.
Figure 1: Quality dimensions
E. Objective of the Study
Selecting semantic criteria for a product that can meet the needs of consumers seems to be critical, and this has a direct impact on the form element of mapping goods. The author uses the minicar as the experimental subject in this study, and the procedure can be divided into two stages: the first stage uses factors analysis to group the 18 Kansei adjectives into four main factors, and the second stage uses the FAHP to calculate the relative weight of customers' Kansei meaning evaluations. Such a methodical approach may assist designers and relevant manufacturers in ensuring the consumers' genuine emotional attractions.
-
-
METHODOLOGY
-
Kansei Engineering
Kansei is a subjective personal perception derived from various illusions, surroundings, or events. Kansei makes use of all of the sensory organs of the human body, including vision, hearing, touch, gustation, and intellect. Kansei Engineering is a customer-oriented technology that dedicates itself to the creation of innovative products.
It is based on ergonomics and computer science, and it may translate a customer's psychological wants or mood into design aspects for a product. Category Identification, Kansei Engineering System, Hybrid Kansei Engineering System, Kansei Engineering Modeling, Virtual Kansei Engineering, and Collaborative Kansei Engineering Designing are the six forms of Kansei Engineering [2, 3]. Expanding semantic attributes (SA) and product characteristics' space is critical in the Kansei Engineering process (Fig. 1) [4], with the formation of semantic attributes (SA) being the first step toward Kansei design success. In past studies, the final Kansei terms were mostly derived from targeted interviews or consumer scoring, resulting in the highest score. Such a strategy is quite simple to implement. The goal of this study is to use factor analysis in conjunction with a fuzzy analytic hierarchy approach to discover the best Kansei Vocabulary technique, allowing designers to better understand their clients' inner hearts and enhancing Kansei meaning accuracy.
-
Category Classification Method: First decide the new product domain: (e.g., online market place, wireless accelerometer). Imagine the product and write the customers emotion, for instance, imagine what kinds of property the customers need, and then write down them on a piece of paper. Then, create a tree structure
using the cards, like the first category, second category and so on. A Fish-born diagram can be used in this stage.
-
Psychological Scaling Method: After collecting Kansei (emotional) words, you create a Likart-type scale using those Kansei words (or SD scale). In this case, the 5- or 7-scale are very popular. In this method, we usually look at the real samples of the product and check the feeling on the scale. Next, analyze these records using the multi-variable statistical methods.
-
Analysis using the multi-variable statistical method: Calculate Factor Analysis to get a similar meaning group as a factor structure and delete the meaningless Kansei words from the research. Principal Component Analysis, data mining method, and others are useful. The JMP (SAS) analysis is most useful to find the design items from customer emotion.
-
Kansei Rough Set Model Kansei Rough Set Model is a unique mathematical model developed by Professor Zdzislaw Pawlak in Poland and it is able to make clear the relation between hidden emotion and design items.
In case of prediction model failure, it is necessary to update the Space of Properties and the Semantic Space, and consequently refine the model. According to Nagamachi (2001) there are three focal points of Kansei Engineering.
-
How to accurately understand consumer Kansei
-
How to reflect and translate Kansei understanding into product design
-
How to create a system and organization for Kansei orientated design
Figure 2: Nagamachi 3 focal points of Kansei Engineering
Kansei Engineering is confined to assessing the emotional effect of words on a human's psyche. As a result, Kansei is not quantified in its whole; just the parts that may be articulated in words are employed. Adjectives are most common; however nouns may also be found (see even Fig.2 depicts this visually).
Thoughts:
Figure 3: Visual representation of adjectives in mind
KE is based on subjective product and idea property evaluations, and it assists consumers in expressing their expectations on items, even those they are unaware of. As a result, semantic tools are used, such as the Semantic Differential Method created by (Osgood et al. 1969). It is feasible to quantify emotions such as feeling, spatial perception, and impression in this manner. The choice to target a certain market group means that certain product characteristics must be understood by the development team and included into the new product's design. Kansei Engineering Type I begins with a notion known as the zero-level idea, which is divided into various sub concepts. These sub ideas may be assessed at various levels until the product design specifications are readily identified. In more complex circumstances, mini surveys may be essential to assist the selection of a final idea.
Figure 4: Kansei and AHP process
A Kansei word is a term used to describe a product. These terms are often adjectives, although they may also have other grammatical forms. Adjectives such as effective, robust, rapid, and others, as well as verbs and nouns (acceleration), may be used to describe a "tv" (Schütte and Eklund 2001).
All accessible sources are utilized to get a comprehensive list of terms, even if the emerging words seem to be similar or identical. Sources that are appropriate include:
-
Magazines
-
Pertinent Literature
-
Manuals
-
Experts
-
Experienced Users
-
Relating Kansei Studies
-
Ideas, visions
-
-
-
-
Factor Analysis
Factor analysis is based on the idea of lowering dimensions; numerous indices may be reduced into a few comprehensive ones under the condition of losing minimal information.
Figure 5: Kansei engineering process (Source: Schütte, 2002)
Factor analysis is a method for condensing a large number of variables into a smaller number of components. This method takes the largest common variance from all variables and converts it to a single score. We may use this score as an index of all variables for future investigation. Factor analysis is a subset of the general linear model (GLM), and it makes certain assumptions: there is a lnear connection, no multicollinearity, relevant variables are included in the analysis, and there is real correlation between variables and factors. There are other ways to choose from, but principal component analysis is the most popular.
As a result, a product style assessment criterion may be constructed, which will be employed in the next research. Chang, Yu-Ming Three sorts of characteristics determining customer emotional perfection toward automobile steering wheels were found by factor analysis extraction: aesthetic considerations, operational strength factors, and modernity aspects.
-
Chih-Fang Huang by applying factor analysis extraction, 14 pairs of adjectives about Chinas melody intention are extracted, thus getting three intention factors.
-
Qianru Qiu in the design of name card, the factor analysis is applied to identify the five semantic groups and the extractive factors which uses the orthogonal rotation method, thus effectively distinguishing the original variables.
-
Achmad Shergian adopting the factor analysis is to divide the originality alarm clocks 8 Kansei adjectives into two main factors, thus meeting
the demand of Kansei Engineerings utilization of mapping process to establish the correlation between Kansei intention and physical design elements.
-
Simon Schütte adopts the factor analysis to process the candy products ordered correlation Kansei words in the AVI scale and get the 4 principle factors: attraction, lifestyle, familiarity and snacks.
-
-
Minitab Statistical Software
Figure 6: Factor analysis through inserting desired variables
-
Analytic Hierarchy Process
Professor Thomas L. Saaty of the University of Pittsburgh in the United States created the Analytic Hierarchy Process in 1971.
One of the most famous and commonly used multicriteria procedures is the analytic hierarchy process (AHP). The methods of evaluating alternatives and aggregating to discover the most relevant alternatives are combined in this methodology. The approach is used to rank a collection of options or to choose the best from a group of choices. The rankings are determined by a series of criteria that are broken down into an overall aim. AHP is a multi-criteria decision- making analysis approach that is used to create quantitative analysis for qualitative situations. It is easy, convenient, and practical.
It may break down complicated concerns into systematic level pieces and then compare the relevance of each one, resulting in a weighted value for each element and a priority ranking for each project.
-
Basic stages of analytical hierarchy process
Pair-wise comparisons (PWC) using a structured questionnaire generate relative priorities (local weights) on the selected criteria; and synthesis of the relative priorities (local weights) into global priorities (global weights) that lead to the selection of the final option. A synthesis of same-level priorities within the hierarchical structure, going up to the end aim, is used to calculate general priorities. The concept of hierarchical composition is the name given to this process (Lam and Zhao, 1998).
Figure 7: Step by step Analytical Hierarchy Process
Table 1: Nine-point scale suggested by Saaty
The PCM is a positive square matrix Ax, where xij > 0 is the subjective ratio betweenwi, and wj, and wx is the weight score from the Ax. The following equation can calculate Saaty's eigenvector method, which is defined for the PCMs:
Awx = max wx wx(A max I)
where the maximum eigenvalue of the A matrix is max.
-
Benefits of AHP in Making Collaborative Decisions Scientists have come up with many methods you can use to make the right decision, but the Analytic Hierarchy Process still stands out of the crowd because of:
-
A proven formula
It is one of the oldest and most trusted decision-making methodologies that many companies have been using to carry out their decisions.
-
Many uses
If you look at the uses of the Analytic Hierarchy Process, you will find that various organizations have used different decision-making problems. There are many applications of
the AHP available. Some of the key uses of the Analytic Hierarchy Process are
-
Project prioritization and selection
-
Site selection (Where you should design your new warehouse / road / airport?) Procurement
-
Technology selection
-
Evaluating different design options
-
Strategy development
-
Stakeholder engagement (for deciding product roadmap, for finalizing the location of an airport or for assisting the government in making policy)
-
Hiring
-
Trade studies
-
-
Easy to use
The software is simple to use. Most essential, you should be able to explain how the programme works and persuade others to trust the judgments it makes.
Let's have a look at some of the more modern approaches.
In such case, you'll notice that the most of them feature a complicated functioning mechanism that makes it difficult to comprehend how the results were obtained.
-
Offers many criteria
When selecting between two issues, you often use a number of multi-criteria to arrive at a decision. However, the majority of software does not have the ability to set various application criteria. Because Analytic Hierarchy Process has the Multi- criteria option, you won't have to worry about this.
-
Choosing the importance of particular criteria When dealing with the multi-criteria functionality, setting the priority value to certain criterion might be difficult. The Analytic Hierarchy Process has made this simple by asking each person which criteria are more important to him and by how much you may evaluate the comparisons and debate the values you can provide to a criterion after the results of the diverse preferences of people have been obtained. The algorithms are then created using your accepted values for the various criteria. As a result, the programme assists you in reaching a consensus on the importance of a criteria.
-
Checks on consistency
We can sometimes be inconsistent in providing the data to the AHP software. But the software works efficiently in checking out the inconsistent data and making you aware of the same. Thus, you can see that the analytic hierarchy process can be beneficial for people working in projects and collaborative firms. But there are many more features of the analytic hierarchy process than just project prioritization. The Analytical Hierarchy Process AHP technique is based on expert judgment method to perform pairwise comparisons between all implemented criteria. However, AHP suffers from a critical criticism. AHP is unable to deal with the impression and subjectiveness of the expert judgment when performing the pairwise comparisons method.
-
-
Fuzzy Analytic Hierarchy Process
The AHP is unable to resolve the problem of fuzziness in decision-making quality. To address the disparities in interviewees' subjective perception, appraisal, and group decision-making, as well as the fuzziness problem, Laarhove and Pedrycz included fuzzy theory into the AHP, resulting in the FAHP. The combination of fuzzy theory and AHP may successfully handle the issue of experts' inaccurate decision- making.
-
Fuzzy Sets, Triangular Fuzzy Numbers and Linguistic Terms
The Fuzzy Set Theory was first published in the journal of Information and Control in 1965 by Professor L.A. Zadeh of the University of California, Berkeley. Over the course of 50 years of Research on the Design Method of Extracting 197 growth and development, the theoretical basis and application technologies of the Fuzzy Sets Theory have become more complex. Fuzzy Set Theory can best explain and emulate human thinking style, as well as synthesise and reflect humansentiments and experiences, enabling for fuzzy measurement, fuzzy identification, fuzzy deduction, fuzzy control, and fuzzy decision for complicated items and systems. The triangle fuzzy membership function is used to define a fuzzy set's membership function across the whole domain X, proving that element X is a member of the membership degree of this fuzzy set. The input variables are x, and the real-value parameters are a, b, and c. The most common triangle membership function is described as follows:
Zadeh (1975) coined the phrase linguistic value, which replaces definite value with linguistic value and uses natural language to communicate the link between the two criteria. It is beneficial for the participants in the experiment to evaluate things objectively. This research use 9-point linguistic values to distinguish between various degrees of attention (Table-1). It has a membership function that ranges from 0 to 1. If it is more important, its membership is closer to 1; otherwise, it is closer to 0.
Figure 8: Triangle membership function
Table 2: Semantic variable used in FAHP
-
Overall Product Evaluation Framework
When evaluating a product in the past, consumers mainly concentrated on three dimensions: "aesthetics," "function," and "commerce." However, as people's material lives have gotten more satisfying, "human-orientation" has become a worry, and whether a product is developed from a "human" viewpoint has become more essential [47,48]. The fourth dimension of "humanity" in design, according to Arthur J. Pulos, a well-known American design professor, is more significant than the other dimensions.
-
Weight
To achieve the overall product evaluation system, the AHP is used to compute the weight of each element compared to the element above it, as well as the weight of each element in the lowest level related to the element in the highest level. The steps are as follows:
1. Construct judgment matrix
Several experts use pairwise comparisons of elements of the same level in the overall product evaluation framework to determine the relative importance of each element and create judgement matrices Aesthetic Attribute; at the evaluation index level, the weights of "Spiritual Demand," "Basic Function," and "Social Influence" are 0.1995, 0.1901, and 0.1000, respectively, ranking the top three in the weight ranking, while the weights of "Material Texture," "Structure and
-
Subjective Product Evaluation System
The overall product evaluation system includes not only subjective evaluation indexes that can be scored based on subjective feelings, but also objective evaluation indexes that can only be scored based on objective product information and data, rather than a customer's visual impression and subjective feelings of products during the purchase stage. As a result, in order to create a realistic subjective product evaluation system, we must first remove objective and subjective evaluation indexes from the total product assessment system, keeping just the subjective evaluation indexes.
According to the weight connections between these factors, the weights of the elements in the subjective product assessment system are further computed by the weights of the elements in the overall product evaluation system.
-
Subjective Product Evaluation System
The overall product evaluation system includes not only subjective evaluation indexes that can be scored based on subjective feelings, but also objective evaluation indexes that can only be scored based on objective product information and data, rather than a customer's visual impression and subjective feelings of products during the purchase stage. As a result, in order to create a realistic subjective product evaluation system, we must first remove objective and subjective evaluation indexes from the total product assessment system, keeping just the subjective evaluation indexes. According to the weight correlations between these components technique used, the weights of the elements in the subjective product assessment system are further determined by the weights of the elements in the overall product evaluation system.
-
determination of Kansei factors,
-
determination of weights using FAHP,
-
determination of composite weights and
-
selection of product.
-
-
-
CASE STUDY
Consumers' ratings of minicar features on a scale of 1 to 5 for each of the 18 sub-criteria listed below were collected from 24 randomly selected customers looking to purchase a new car.
Table 3: 1-5 rating of 24 persons for 18 sub-criteria
Table 4: 1-5 rating of 24 persons for 18 sub-criteria (Contd)
Stage One: Extract Product Image.
Semantic Screening is a kind of semantic screening. The semantic characteristics and property space of goods are two crucial components for expanding the products field. This study conducted a large survey of minicar-related journals, magazines, and networks, resulting in the extraction of 18 relevant adjectives that have a strong relationship with the minicar's perceptual cognition level (concise, free, leisure, smooth, lively, romantic, transparent, curvilinear, enthusiastic, strong, practical, rounded, vigorous, feminine, eye-catching, harmonious, luxurious, and wild).
Stage Two: Weight of Measuring Criterion.
Factor Analysis:
C1, C2, C3, C4, C5, C6, C7, C8, C9, C10, C11, C12, C13, C14, C15, C16, C17, C18
Principal component factor analysis of the correlation matrix. Unrotated factor loadings and communalities
Table 5: Factor analysis of the input values
Table 6: Weights of measured criterion through factor analysis
FAHP's main goal is to provide a hierarchical structure. Objective hierarchy, criterion hierarchy, and sub-criteria hierarchy are the three hierarchies used in this study. The criteria hierarchy is characterized as contemporary factor, appealing factor, elegant factor, and dynamic factor; the objective hierarchy is defined as the minicar's Kansei semantic
choice. The Kansei adjectives are used to define the sub-
The following takes the first expert evaluations Modern Factor, subset P1 as an example:
criteria hierarchy.
(1,1,2) (1,1,2) (0.2,0.25,0.33) (2,3,4)
I
(0.5,1,1)
(1,1,2)
(0.25,0.33,0.5)
(1,1,2)
= I
(3,4,5)
(2,3,4)
(1,1,2)
(1,1,2)
P1
(2,3,4)
(1,1,6)1
I (2,3,4)I
A. Evaluation Value after Calculating and Integrating the Groups Opinions
After getting each experts paired comparison value, the experts fuzzy positive reciprocal matrix is set up. Then the matrixs original accurate value aij is transformed into aij = (aijl, aij, aiju) in accordance with the semantic value. Each experts relative importance evaluation value for No. i and No. j items is aij. The triangle fuzzy number will transform aij into three numbers, aijl, aij and aiju; the aijl represents the experts evaluation minimum value of item i to js comparative judgment. aiju represents the experts evaluations maximum value.
The following takes the first expert evaluations attractive
P1=
I(0.25,0,0.5) (0.5,1,1) (0.5,1,1) (1,1,2) (2,3,4)I
[(0.25,0,0.5) (0.17,1,1) (0.25,0.33,0.5) (0.25,0.33,0.5) (1,1,2)]0.8 2.25 21.33
0.125 0.333 12 1
12 36 320
I 0.125 1 4 I
Geometric Mean :
Z1 =(0.95635, 1.17608, 1.8442)
Z2 =(0.65975, 0.80274, 1.64375)
Z3 =(1.64375, 2.04477, 3.16979)
Z4 =(0.65975, 1.00000, 1.31951)
Z5 =(0.30418, 0.51728, 0.75786)
According to formula (3), each criterions fuzzy weight can be :
W1 =(0.10948, 0.21214,0.43663)
W2 =(0.07553, 0.1448, 0.38916)
W3 =(0.18818, 0.36936, 0.75046)
W4 =(0.07553, 0.18038, 0.3124)
W5 =(0.03482, 0.09331, 0.17943)
factor, subset P1 as an example:
In order to get each criterions fuzzy weight, the formula (2) should be used to get each lines geometric mean. The following is the value of calculating Zi:
Geometric mean
Z1 = (2.4644, 2.9938, 4.4246)
Z2 = (0.2387, 0.2806, 0.4175)
Z3 = (0.4807, 0.6177, 0.9012)
Z4 = (0.9635, 1.3077, 1.9786)
Z5 = (2.6889, 3.4085, 4.2339)
Z6 = (0.3574, 0.4228, 0.4533)
According to formula (3), each criterions fuzzy weight can be:
W1 = (0.2016, 0.3314, 0.5898)
W2 = (0.0195, 0.0311, 0.0580)
W3 = (0.0393, 0.06384, 0.1253)
W4 = (0.0788, 0.1448, 0.2750)
W5 = (0.2200, 0.3773, 0.5885)
W6 = (0.0292, 0.0468, 0.0630)
In order to get each criterions optimum defuzzification value, each defuzzification value can be worked out in accordance with the centroid method. And the normalization method can be applied to get the relative weight. P1s defuzzification matrix DFP1 and the normalization value NWP1 are as follows:
In order to get each criterions optimum defuzzification value, each defuzzification value can be worked out in accordance with the centroid method. And the normalization method can be applied to get the relative weight: P1s defuzzification matrix DFP1 and the normalization value NWP1 are as follows:
0.25275
0.203161
DFP1= 0.43623
I0.18944I [0.10252]
0.2135
0.1716 1
NWP1= 0.36828
I0.16001I [0.08661]
The following takes the first expert evaluations Elegant Factor, subset P1 as an example:
(1,1,2)
(1,1,2)
(1,1,2)
(2,3,4)
(0.5,1,1)
(1,1,2)
(2,3,4)
(1,1,2)
(0.5,1,1)
(0.25,0.33,0.5)
(1,1,2)
(1,1,2) ]
P1= [
Z1 =(1.18921, 1.31607, 2.37841)
Z2 =(1, 1.31607, 2)
Z3 =(0.5946, 0.75984, 1.18921)
Z4 =(0.5, 0.75984, 1)
According to formula (3), each criterions fuzzy weight can be :
W1 =(0.18107,0.31699,0.72428)
W2 =(0.15226,0.31699,0.60905)
W3 =(0.09054,0.18301,0.36214)
W4 =(0.07613,0.18301,0.3042)
0.3743
0.0362
0.0777
DFP1
0.1662
0.3953
0.0463
0.3145
0.0330
0.0709
NWP1
0.1516
0.3607
0.0422
Table 7: 8 Experts weight value for each evaluation criterion
Table 8: Sequence of order though relative weight
-
CONCLUSIONS
It is critical for Kansei Engineering to extract consumers' emotional demands in particular goods. The choice of modelling elements has a direct impact on the Kansei image. A technique is suggested that combines components analysis and the fuzzy analytic hierarchy process to extract the products' high correlation meaning, so pointing the designer's modelling creativity in the right path. In this article, the minicar is used as the object, and it is discovered that the contemporary aspects of "concise" and "smooth" are the easiest to captivate the customer's inner heart, resulting in emotional resonance. Meanwhile, the study approach might be used to improve the design of other industrial products.
-
REFERENCES