
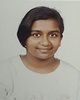
- Open Access
- Authors : Achshah R M , Dr. T. Ruban Deva Prakash
- Paper ID : IJERTV11IS040094
- Volume & Issue : Volume 11, Issue 04 (April 2022)
- Published (First Online): 21-04-2022
- ISSN (Online) : 2278-0181
- Publisher Name : IJERT
- License:
This work is licensed under a Creative Commons Attribution 4.0 International License
Design of Complete Intelligent Power Quality Preserver for Smart Micro-Grid
Achshah R M
Department of Artificial Intelligence & Data Science SRM Easwari Engineering College
Chennai, India
Dr. T. Ruban Deva Prakash
Principal
Govt. College of Engineering Munnar, India
AbstractReliability and quality are the prime requirements of electrical consumers. Consumers are annoyed by frequent shut down and failure of smart equipments due to voltage flicker, voltage sag, voltage unbalance, harmonic pollution and frequency deviation. The main objective of this work is to design a novel power quality conditioning device Complete Intelligent Power Quality Preserver which automatically senses, diagnose and predicts the type of power quality problems which will prevails in smart micro grid and initiates proper control action to mitigate the same, thus imparting self healing feature to micro grid. The Complete Intelligent Power Quality Preserver (CI-PQP) is designed with the capacity to mitigate voltage flicker, voltage sag, harmonics, voltage unbalance and frequency deviation simultaneously, provide both real and reactive power support, sense data from remote locations to estimate power quality, predict and diagnose the type of power quality problem, select necessary control technique automatically without human intervention and learn by experience. CI-PQP has compensating unit (CU), wide area monitoring system (WAMS) and central power conditioning unit (CPCU). The WAMS has phasor measurement unit (PMU), phasor data concentrators (PDC), and fiber optic communication channel with transmission control protocol / internet protocol (TCP/IP). The compensating unit consists of converter controller, battery energy storage system (BESS), wind-up battery charger, shunt converter, matrix converter and series converter. The converter controller has three dsPIC Digital Signal Controllers and one FPGA controller which generate gate drive signals based on reference signals. The CPCU has cloud computing system with Artificial Neural Network (ANN) and Fuzzy Logic Control based multi- agents which continuously analyses and predicts the power quality problems that will prevail at various regions of the micro-grid and activate necessary control actions automatically by communicating with the compensating device. Prediction of power quality problems and proper selection of control for mitigating is achieved by data base agent, manager agent, power quality agent and several intelligent control agents who act together towards common objective as in multi-agent system. The control strategies used by control agents are fuzzy bang- bang control, two neuron control, adaptive ANN control, frequency control using co-ordinate transformation and voltage balancing using sequence components. Incorporation of intelligent behaviour of multi-agent system and efficient use of computing infrastructure feature of cloud computing system in CPCU makes the system highly effective and efficient. This work benefits the industries by reducing field service costs, manufacturing costs, productivity costs, product damage and loss of revenue. It increases competitiveness and opportunity. This also helps the power sector in supplying clean and green power, reducing energy wastage and increasing life time of power system components.
KeywordsPower quality; compensators; ANN; fuzzy logic; multi-agent; cloud computing.
-
INTRODUCTION
The main concern of consumers of electricity is the continuity of the supply, i.e. the reliability. However, nowadays consumers not only want reliability, but quality too. For example, a consumer that is connected to the same bus that supplies a large motor load may face sudden voltage fluctuations (flicker) every time the motor is started. Depending on the sensitivity of the consumers load this voltage fluctuation may lead to a failure or disconnection of the entire plant. Although the supply is not interrupted the consumer experiences a disturbance in the form of voltage flicker that causes an outage of the plant. There are also very sensitive loads such as hospitals, processing plants, air traffic control, and financial institutions, etc. that require uninterrupted and clean power. Increased automation in homes and factories has increased the impact of power quality deviations. Power quality has been defined as any problem manifested in voltage, current, or frequency deviations that result in failure or mal-operation of utility or end-user equipment. Several reasons have been given to explain the current interest in power quality.
-
Equipment has become less tolerant to voltage disturbances. Industrial customers are much more aware of the economical losses that power quality problems may cause in their processes.
-
Equipment causes voltage disturbances. Often the same equipment that is sensitive to voltage disturbances will itself cause other voltage disturbances. This is the case with several power converters.
-
There is a need for performance criteria. An increasing need for performance criteria to assess how good the power companies do their job is realized. This is especially important for the monopolistic part of the chain formed by generation, transmission, and distribution of electricity. The natural monopoly that transmission and distribution companies possess, even in the deregulated markets, requires a quality framework where compulsory quality levels are given. Regulator bodies will have to create such a quality framework in terms of power quality indices.
-
Power quality can be measured. The availability of power quality monitors means that voltage and current quality can actually be monitored on a large scale.
Hence power quality conditioning gains importance particularly in smart micro grid with more RESs [1]. There are
three general approaches to solve power quality problems: eliminate or modify the source of the disturbances, eliminate or modify the path for the disturbances between the source and the affected equipment, protect the affected equipment. Generally, consideration of all three options is necessary to develop a cost-effective solution. The least-cost approach to mitigate power quality problem is to be adopted [2]. Earlier studies have indicated that energy storage can compensate for the stochastic nature and sudden deficiencies of RESs for short periods without suffering loss of load events and without the need to start more generating plants. Upgrading the power system to mitigate power quality problem is often uneconomic. Instead, the inclusion of power quality conditioning device for power smoothing and voltage correction at the remote point of connection would allow utilization of the power and could offer an economic alternative to upgrading the power system and energy storage [3].
-
-
RELATED WORK
Mitigation of power quality can be done through reactive power compensation. High power quality can be maintained if real power support is provided along with reactive power. In micro grid the inverters used along with PV cell, fuel cell or micro turbine are controlled to improve power quality. Even though it is economic, it is not an effective mean of power quality mitigation in micro-grid with Custom Power Park (CPP). CPP contains highly unbalanced nonlinear loads along with distributed renewable generation [4]. Conventionally, Static Var Compensators (SVCs) have been used in conjunction with passive filters at the distribution level for reactive power compensation and mitigation of power quality problems. Though SVCs are very effective system controllers use to provide reactive power compensation at the transmission level, their limited bandwidth, higher passive element count that increases size and losses, and slower response make them inapt for the modern day distribution requirement [5]. Static Compensator (STATCOM) and Distribution Static Compensator (DSTATCOM) are used which has low harmonic emission and shorter response time than that of an SVC, mainly due to the fast switching times provided by the IGBTs of the voltage source converter [6]. The STATCOM also provides better reactive power support at low AC voltages than an SVC, since the reactive power from a STATCOM decreases linearly with the AC voltage. On the other hand STATCOMs typically exhibit higher losses and may be more expensive than SVCs, so the technology is not widespread. Static Synchronous Series Compensator (SSSC) works in the same way as the STATCOM [6]. It has a voltage source converter serially connected to a transmission line through a transformer. It needs an energy source to provide a continuous voltage through a condenser and to compensate the losses of the VSC. A SSSC is able to exchange active and reactive power with the transmission system. Unlike Thyristor Controlled Series Compensator (TCSC), it does not suffer from sub-synchronous resonance problems. The SSSC version applied in distribution systems is the Dynamic Voltage Regulator (DVR). This requires large dc link capacitor, coupling transformer and energy source for real
power transfer. Hence it is not economical [8]. Unified Voltage Controller (UVC), is a cheap version of the DVR because it doesnt have the energy storage device, it is made to operate in network points with a high level of short circuit [7]. Interline Dynamic Voltage Restorer (IDVR) is used for compensation in parallel feeders. It comprises of two DVRs linked by common dc link capacitor, when one DVR compensates, the DVR in the other line replenishes the dc link energy storage. Hence it does not require large dc link capacitor, but when both the lines demands compensation simultaneously, the device is not effective [8]. IDVR is the version of Interline Power Flow Controller (IPFC) used for controlling voltage quality rather than power flow. Generalized Universal Power Flow Controller (GUPFC) overcomes this drawback, which has two DVRs and one STATCOM for replenishing dc link energy storage. GUPFC cannot be used for frequency deviation compensation [9]. Another universal and effective device is the Unified Power Flow Controller (UPFC) which is able to control transmission voltage, line impedance and phase angle independently and concurrently, and to internally generate controllable reactive power. Thus UPFC operats as a static synchronous compensator, static synchronous series compensator and phase angle regulator. UPFC used in distribution side for power conditioning is called Unified Power Quality Controller (UPQC). The drawbacks are high cost, high loss and large harmonic injection. The capacitor in UPFC brings with it several disadvantages which affects the overall reliability of the UPFC [10]. The Matrix Converter (MC) topology comes without any such bulky reactive element except for lighter components as filters. Matrix converter is highly controllable and allows independent control of the output voltage magnitude, frequency, phase angle as well as an input power factor. The main drawback of the matrix converter is the presence of current harmonics in input and voltage harmonics in the output. The matrix converter input voltage variation directly affects the matrix converter output voltage. The matrix converter voltage transfer ratio is 0.866. These drawbacks are rectified by a Unified Power Line Manager (UPLM) which is a combination of STATCOM, SSSC and matrix converter [11]. Due to limitation in size of dc link capacitor and its energy storage, long duration disturbances cannot be compensated. The device can provide only reactive power compensation, but it cannot provide real power compensation. Real power compensation along with reactive power compensation can give a better power quality solution. In order to overcome this drawback the proposed device has BESS and LVM-WEG along with UPLM. The control strategy adopted for generating reference signal for the converters of FACTS device plays a key role in deciding the dynamic behavior of the system. All the conventional control algorithms are designed for mitigating only one or two power quality issues. Conventional control algorithms lack intelligence such as learning, creativity, intuition and inference. Hence there is a need for control technique which automatically sense and diagnose the power quality problem prevailing in the system and mitigate the same without human intervention. The challenges in the area of PQ monitoring may be better addressed using agent technology. Agent technology is one of the recent developments in the
Fig. 1 Block Diagram of CI-PQP
field of distributed artificial intelligence. In the past few years, multi-agent system (MAS) technologies have been proposed for applications in power systems such as substation automation, fossil-fuel power unit, power system reconfiguration, power market, and transmission expansion planning. The advantages of MAS over client-server systems are in the areas of network bandwidth requirement and computation time, improved scalability, and the ability to create an intelligent system. Artificial Neural Networks (ANN) and Fuzzy Logic Controllers (FLC) are extensively used in multi-agents. Cloud computing is the most recent results of the advancement of several computer technologies both from the hardware side, such as virtualization and multi- core architectures, and from the software side like cluster computing, Grid computing, Web services, service-oriented architectures, autonomic computing, and large-scale data storage. In particular, virtualization in Cloud computing is the key element that separates system functionality and implementation from physical resources. By exploiting virtualization techniques, a Cloud infrastructure can be partitioned in several parallel virtual machines, dynamically configured according to the user requirements and devoted to run independent applications concurrently. Virtualization
separates applications from hardware and users from other users giving them the feeling that a large-scale computing infrastructure is devoted to their applications by meeting a given quality of service (QoS). Virtualization is also used to isolate applications avoiding that if one fails that other can fail too. Finally, virtualization is a way to improve security and privacy of concurrent applications running on the same Cloud. An agent is a computer system that can act flexibly in dynamic and uncertain environments. A multi-agent system is a system with multiple problem solving entities, and it consists of a number of agents which interact with one another. To successfully interact, agents require the ability to cooperate, coordinate, and negotiate with each other. Research in multi-agent systems is concerned with the development of methods and algorithms for multiple interacting agents. A Cloud computing system consists of a collection of inter-connected and virtualized computers dynamically provisioned as one or more unified computing resource(s) through negotiation of service-level agreements (SLAs) between providers and consumers. Agent-based Cloud computing is concerned with the design and development of software agents for bolstering discovery, negotiation and composition of Cloud services. Hence our
PMU
V I
Garmin GPS 72H GPS receiver
USB 6009 OEM data
acquisition module
PIC
Microcontroller
Personal Computer for Synchro-phasor calculations
Modem
Dell power edge server 12G T420 with Intel Xeon E5
processor
Modem & Router
Other PMUs
PDC
Fig. 2 Block Diagram of PMU and PDC in Wide Area Monitoring System (WAMS)
project uses agent based cloud computing which will be very useful for implementing IQC in a highly interconnected smart grid with more number of PMUs, CUs and control areas. The present research will result in the development of power quality conditioning device and effective control technologies for power quality mitigation. Unlike other existing devices, this product uses Phasor Measurement Unit for measurements and gathers the remote measurement data using Phasor Data Concentrators. Collected data are analyzed for power quality assessment and prediction and the required control technique is automatically selected using ANN and FLC based multi-agent system implemented in a cloud computing system of central power conditioning unit and mitigation of power quality is executed using the proposed compensating device. This product is named as Complete Intelligent Power Quality Preserver (CI-PQP) as it self-heal all power quality problems associated with smart micro grid intelligently.
-
DESCRIPTION OF CI-PQP
The block diagram of CI-PQP is shown in Fig.1. CI-PQP has compensating unit (CU), wide area monitoring system (WAMS) and central power conditioning unit (CPCU). The WAMS has three PMUs, PDC, and fiber optic communication channel with TCP/IP communication protocol. The PMU and PDC are designed on the basis of open source PMU design for
research purpose by National Instruments which has enough channels to measures the three phase voltages and currents. The PMU designed by us has USB 6009 OEM data acquisition module, Garmin GPS 72H type GPS receiver, PIC microcontroller to serve as Phase Locked Loop (PLL), PC to do synchro-phasor calculation using open ware VC++ program and fibre optic modem (FOM). Phasor Data Concentrators (PDC) collects the information from PMUs using optical fibre communication with TCP/IP communication protocol and transmits them to Central Power Conditioning Unit (CPCU). The PDC designed by us has a fibre optic router, fibre optic modem and Dell power edge server 12G T420 with Intel Xeon E5 processor for performing data consolidation, data analysis and visualization and temporary data storage using open ware provided by National Instruments. The PMU and PDC setup are shown in Fig.2. The compensating unit consists of converter controller, battery energy storage system (BESS), wind-up battery charger, shunt converter, matrix converter and series converter. The shunt converter is connected in the input side of the matrix converter and series converter is connected in the output side. The shunt converter and series converter shares a common dc link. The dc link energy is replenished using BESS charged by wind-up battery charger. The wind-up battery charger consists of a three blade, small permanent magnet three phase alternator with internal rectifier producing 48V DC output.
Data base agent
Power quality agent Manager agent
Control agent – I
Control agent – II
Control agent – III
Series agent
Series agent
Series agent
Shunt agent
Shunt agent
Shunt agent
Matrix
Matrix
Matrix
PDC
PMU – I PMU – II
Communication Channel
Converter Control
PMU – III
Fig. 3 Architecture of CPCU
The converter controller has three dsPIC Digital Signal Controllers and one FPGA controller which generate gate drive signals based on reference signals. The shunt and series converters are controlled by dsPIC and the matrix converter is controlled by the combined action of FPGA and dsPIC.
The CPCU has cloud computing system with Artificial Neural Network (ANN) and Fuzzy Logic Control based multi-agents which continuously analyses and predicts the power quality problems that will prevail at various regions of the micro-grid and activate necessary control actions automatically by communicating with the compensating device. The functions of CPCU are complicated and cannot be performed efficiently by single processor. Hence they are
performed by a cloud computing system which is a collection of inter-connected and virtualized computers with efficient infrastructure. Prediction of power quality problems and proper selection of control for mitigating the same requires intelligence. Thus the interconnected and virtualized computers in the cloud computing system are replaced by intelligent agents who act together towards common objective as in multi-agent system. Incorporation of intelligent behaviour of multi-agent system and efficient use of computing infrastructure feature of cloud computing system in CPCU makes the system highly effective and efficient. The CPCU has data base agent, manager agent, power quality agent and several control agents as shown in Fig.3. The data
1
Y
2
CPCU
PDC
Communication Channel
A
3
5 B D 4
TSC1
F TSC2
Load C
6
E Load
8 7
BESS
PMU &
Compensating Unit-1
TSC3 BESS
Load3
PMU &
Compensating Unit-3
PMU &
Compensating Unit-2
Wind Power Generating System
Fig. 4 Microgrid Test Setup with CI-PQP
collected by PMU reaches database agent through PDC. The data base agent stores the data and communicate the same to power quality agent. The power quality agent has ANN to predict the nature of Power Quality (PQ) problem that may occur in the next instant and its location (control area). The set of measurements taken from the considered network with its power quality analysis results using S-transform at various loading conditions for quite long period is used as training data for ANN. The power quality agent communicates the result to data base agent and manager agent. The manager agent selects the compensating unit that should be activated to solve the PQ problem depending on location information. It also has fuzzy rule base to select the control algorithms for the same. The manager agent communicates to data base agent and respective control agents. Each and every control area of a micro grid has a control agent. The operations of control agent are complicated for software development. Hence the same is decomposed into three subagents called shunt agent, series agent and matrix agent. The shunt agent has specific control strategies for generating references to solve harmonic and
voltage unbalance problems. The series agent has specific control strategies for generating references to solve voltage sag and voltage flicker problems. The matrix agent has specific control strategy for generating references to solve frequency deviation problems. The output of control agent is given to PDC which communicates though fiber optics to converter controller. Each and every intelligent agent or subagent is a programmed processor/PC with artificial intelligence. The agents are interconnected by using the concepts of cloud computing system.
-
CONTROL ALGORITHMS
The control strategies used by control agents are fuzzy bang-bang control, two neuron control, adaptive ANN control, frequency control using co-ordinate transformation and voltage balancing using sequence components. For voltage flicker mitigation using series converter of CI-PQP, the magnitude of injected voltage is kept constant and its angle is varied. When the angle is varied the real and reactive power changes. Flicker mitigation can be achieved by reactive power control. The reactive power flow is maximum at 90o, medium
at 0o and minimum at 270o of injected voltage. The angle of injected voltage can be switched between these three values for flicker mitigation. Fuzzy Logic Controller (FLC) is used for proper switching. Thus the output of CI-PQP is bang-bang controlled between three values. The design of FLC is based on Lyapunav function analysis. In case of voltage sag, a new two neuron control algorithm based on simplest pre-sag supply voltage boosting technique is activated. According to this, the series converter of CI-PQP injects a voltage in series with line such a way that the supply voltage becomes equal to pre-sag supply voltage. The resulting voltage at the load bus bar equals the sum of the grid voltage and the injected voltage from the CI-PQP. Adaptive ANN control is used for generating reference control signal for shunt converter of CI- PQP to filter harmonics. A Newton-Raphson based algorithm is proposed for ANN to extract flicker components. In case of voltage unbalance, sequence components of voltages are generated and the shunt converter of the CI-PQP filters negative and zero sequence components. In order to eliminate frequency deviation, the supply frequency is sensed and compared with reference frequency. The frequency error is given as input to PI controller and the co-ordinate transformation angle is calculated according to the output of PI controller by PLL, which is used for co-ordinate transformation to generate reference signal for the matrix converter of CI-PQP.
-
TEST SYSTEM AND RESULTS
The micro-grid setup of the selected campus network is shown in Fig. 4. Specifications of the micro-grid setup of the selected campus network are given in table 1. The prototype micro grid test system has eight buses and the same is divided into three control areas. Control area -1 has busses 1, 2, 3 and
8, control area 2 has busses 3, 4 and 7 and control area 3 has busses 3, 5 and 6. PMUs and compensating units are located in three busses (Bus-6, Bus-7 & Bus-8) and the CPCU
& PDC are located at Bus – 2. Each control area has one PMU and CU. Selection of nodes for locating compensating unit and Phasor Measurement Unit (PMU) is out of the scope of our project.The designed device CI-PQP based on ANN, FLC and multi-agent system includes measurement of data required for analyzing power quality; predict and diagnose the power quality problem using measured data and implements solution using inbuilt control algorithms suitable for various power quality issues.
TABLE I SPECIFICATIONS OF THE MICRO-GRID SETUP
Load – 2
476.6KW, 205KVAR
Load – 3
109.8KW
Wind power generating simulator
3.7KW
Transmission Line A (12 meter)
4 x 3.5 x 400 Sq.mm, AYFY
cable
Transmission Line B (10 meter)
3.5 x 400 Sq.mm, AYFY cable
Transmission Line C (10 meter)
2 x 3.5 x 185 Sq.mm, AYFY
cable
Transmission Line D (10 meter)
3.5 x 150 Sq.mm, AYFY cable
Transmission Line E (350 meter)
3 x 3.5 x 400 Sq.mm, AYFY
cable
Transmission Line F (12 meter)
3.5 x 240 Sq.mm, AYFY cable
Bus-8 from control area-1, bus-7 from control area-2 and bus-6 from control area-3 are selected for power quality analysis with and without compensator. Voltage variation, variation in frequency, flickers, THD in voltage and current are used as the element of power quality analysis. Values of all elements are recorded at interval of five minute except flicker. The short time flickers are recorded at interval of 10 minutes.
Case 1: Bus-8
Over the period of measurement from 9 am to 10 am, the load current is recorded between 130A to 160A.The voltage variation on the terminal of load is shown in Fig 5. The maximum value of variation is recorded as 8.2% of rated rms phase voltage without CI-PQP and 2.1% with CI-PQP. It is observed that voltage unbalance is also corrected. The next quality parameters, the frequency variation and flickers are also in the defined range of good power quality after using CI- PQP. As in Fig.6, the maximum value flicker is only 0.52volts in B-phase of the supply without CI-PQP and 0.25volts with CI-PQP. Fig. 7 depicts the graphical representation of average value of THD over an interval of 5 min, for voltage without and with CI-PQP which are 12% and 1.5% respectively.
Case 2: Bus-7
Over the period of measurement from 9 am to 10 am, the load current is recorded between 90A to 60A.The voltage variation on the terminal of load is shown in Fig 8. The maximum value of variation is recorded as 12.2% of rated rms phase voltage without CI-PQP and 3.5% with CI-PQP. The next quality parameters, the frequency variation and flickers are also in the defined range of good power quality after using CI-PQP. As in
Item
Specification
Point of Common Coupling (PCC)
11 KV
Transformer
630KVA, 11KV/433V
Capacitor at bus-2
50KVAR
Distributed Generator (DG) at bus-4
125KVA, 415V,3, 50Hz
Distributed Generator (DG) at bus-5
500KVA, 415V,3, 50Hz
Battery Energy Storage System (BESS) at bus-7
60KVA
Battery Energy Storage System (BESS) at bus-8
40KVA
Thyristor Switched Capacitor (TSC) at bus-6
120KVAR
Thyristor Switched Capacitor (TSC) at bus-7
205KVAR
Thyristor Switched Capacitor (TSC) at bus-8
150KVAR
Load – 1
375KW, 142HP, 13.5KVA,
120KVAR
Flicker without CI-PQP
Fig. 12 (a) Flicker without CI-PQP at Bus 6
Flicker with CI-PQP
Fig. 12 (b) Flicker with CI-PQP at Bus 6
Fig. 10 (a) THD without CI-PQP at Bus 7
Fig. 10 (b) THD with CI-PQP at Bus 7
Fig. 11 (a) Variation in voltage without CI-PQP at Bus 6
Fig. 11 (b) Variation in voltage with CI-PQP at Bus 6
Fig. 9 (a) Flicker without CI-PQP at Bus 7
Fig. 9 (b) Flicker with CI-PQP at Bus 7
Fig. 6 (a) Flicker without CI-PQP at Bus 8
Fig. 6 (b) Flicker with CI-PQP at Bus 8
Fig. 8 (a) Variation in voltage without CI-PQP at Bus 7
Fig. 8 (b) Variation in voltage with CI-PQP at Bus 7
Fig. 5 (a) Variation in voltage without CI-PQP at Bus 8
Fig. 5 (b) Variation in voltage with CI-PQP at Bus 8
Fig. 7 (a) THD without CI-PQP at Bus 8
Fig. 7 (b) THD with CI-PQP at Bus 8
Fig.9, the maximum value flicker is only 0.9volts in B-phase of the supply without CI-PQP and 0.35volts with CI-PQP. Fig. 10 depicts the graphical representation of average value of THD over an interval of 5 min, for voltage without and with CI-PQP are 14% and 1.28% respectively.
Case 3: Bus-6
Over the period of measurement from 9 am to 10 am, the load current is recorded between 60A to 90A.The voltage variation on the terminal of load is shown in Fig 11. The maximum value of variation is recorded as 9% of rated rms phase voltage without CI-PQP and 1.8% with CI-PQP. The next quality parameters, the frequency variation and flickers are also in the defined range of good power quality after using CI-PQP. As in Fig.12, the maximum value flicker is only 1.5volts in B-phase of the supply without CI-PQP and 0.55 volts with CI-PQP. Fig. 13 depicts the graphical representation of average value of THD over an interval of 5 min, for voltage without and with CI-PQP are 10% and 0.9%..
Fig. 13 (a) THD without CI-PQP at Bus 6
Fig. 13 (b) THD with CI-PQP at Bus 6
-
PROJECT IMPACT
This project has number of social benefits in the three levels of individual, organization and community. It contributes towards the supply of clean power which increases the life time and performance of end use equipments. It provides national benefit by reducing power quality cost. Association with pollution free environment can enlarge mans imagination and revive his spirit. Polluted atmosphere can demean the people who live among it. Most of today's
generation capacity relies on fossil fuels and contributes significantly to the increase of carbon dioxide in the world's
amosphere, with negative consequences for the climate and society in general. Generation of electrical energy, however, is currently the largest single source of carbon dioxide emissions, making a significant contribution to climate change. To mitigate the consequences of climate change, the current electrical system needs switching towards renewable energy. One of the major problems associated with the integration of Renewable Energy Sources (RESs) to grid is the power quality problem. Our project solves this problem and paves way for the development of smart micro grids with green energy sources. This has positive effect in climate change and global warming caused by fossil fuels fired power plants. We know that resources are limited, and due of this, in 21st century all developing and developed countries are promoting the studies, and researches that address the concerns of depleting resources. This project gives a solution to depleting fossil fuels by paving way for the integration of RESs. This project also contributes indirectly towards reduction in energy cost. The research output is important for
-
Supplying clean power in smart micro-grids
-
Improving the productivity of industries by reducing the impact of power quality.
-
Reduces down time cost and lost product in industries.
-
Unexplained equipment trips or shutdowns
-
Reduces equipment damage, component failure and process disruptions.
-
Reduces random lockups and data errors.
-
Reduces over heating of power system components.
-
Improving the Energy Cost/Value of electricity by reducing process inefficiency associated with power quality disturbances
Thus, this project benefits the industries by reducing field service costs, manufacturing costs, productivity costs, product damage and loss of revenue. It increases competitiveness and opportunity. This project helps the power sector in supplying clean and green power, reducing energy wastage and increasing life time of power system components. In lighting systems, voltage flicker values as low as 0.5% can be perceptible to the human eye. Flicker values of 3% are downright irritating. This project helps in reducing flicker in lighting system and to improve the performance and life time of household equipments.
VII CONCLUSION
The economic growth of 1.4 billion population in India depend on modernization of electric grid, because the grid is so unreliable and power is polluted. Nearly 14% of India's electricity demand is met by backup diesel generators which is both very expensive and polluting. Hence every step taken to provide clean and reliable power contributes to modernization of power sector In India, every development in power sector already has a micro-grid of renewable sources to supply communities, malls, and office complexes.This project designed a novel power quality conditioning device which automatically senses, diagnose and predicts the type of power quality problems which will prevails in smart micro grid and initiates proper control action to mitigate the same,
thus imparting self healing feature to micro grid. Since most process in industries are controlled by microcontrollers, power quality problems leads to outages. Loss encounter with a momentary outage due to power quality problem ranges in lakhs of rupees and loss encounter due to voltage deviation event is approximately fifty thousand rupees. The initial cost of installing power quality conditioners can be get back within a simple payback period of two years. The simulation results obtained from analysis of the designed product can be used for future improvements in design of prototype model and implementation.
ACKNOWLEDGMENT
We thank the Director, Centre of Continuing Education, Kerala, India who permitted us to utilize the research facilities of College of Engineering, Munnar to complete the design of Complete Intelligent Power Quality Preserver.
REFERENCES
[1] Mukesh Pushkarna, Haroon Ashfaq, Rajveer Singh, Anurag Priyadarshi, Power Quality Problems due to Upgrades in the Power System with RES and Front-line Solutions, 2022 2nd International Conference on Power Electronics & IoT Applications in Renewable Energy and its Control (PARC), IEEE Xplore, pp. 529-551, March 2022. [2] A. Sannino, J. Svensson and T. Larsson, "Power-electronic solutions to power quality problems", Electric Power Systems Research, vol. 66, pp. 71-82, 2003.. [3] Mohit Bajaj, "Design and Simulation of Hybrid DG System Fed Single-Phase Dynamic Voltage Restorer for Smart Grid Application", Smart Science, vol. 8, no. 1, pp. 24-38, 2020.. [4] A. N. Abdalla, M. S. Nazir, Z. Tiezhu, M. Bajaj, P. Sanjeevikumar andL. Yao, "Optimized Economic Operation of Microgrid: Combined Cooling and Heating Power and Hybrid Energy Storage Systems", ASME. J. Energy Resour. Technol, May 2021.
[5] M. Bajaj, F. Aymen, M. Alowaidi, N. K. Sharma, S. Mishra and S. K. Sharma, "A Lyapunov-Function based Controller for 3-phase Shunt Active Power Filter and Performance Assessment Considering Different System Scenarios", IEEE Access, vol. 9, pp. 66079-66102, 2021.. [6] Mohit Bajaj and Ankur Singh Rana, "Harmonics and Reactive Power Compensation of Three Phase Induction Motor Drive by Photovoltaic- based DSTATCOM", Smart Science, vol. 6, no. 4, pp. 319-329, 2018. [7] Püvi. V, Lehtonen. M. Convex Model for Estimation of Single-Phase Photovoltaic Impact on Existing Voltage Unbalance in Distribution Networks. Applied Sciences. , vol.10, No.24, 2020. [8] Dr. Arun K. Mitra , Mr. Vinay Keswani, 2019, A Review Paper on Power Quality Improvement Techniques in Power System with Renewable Distributed Generation Source, International Journal of Engineering Research & Technology (IJERT) Volume 08, Issue 11, November 2019. [9] Xu Tao, Design of power grid quality detection terminal for smart grid Chengdu University of Technology, 2017 [10] Jingyue Zhang, Research on Power Quality Problems Based on Smart Grid and New Energy Generation, AIP Conference Proceedings 2066, 020022 (2019), Jan.201. [11] D..Amaripadath, R.Roche, L..Joseph-Auguste, D.Istrate, Power quality disturbances on smart grids: Overview and grid measurement configurations, 2017 52nd International Universities Power Engineering Conference (UPEC), IEEE Xplore: 21 December 2017.