
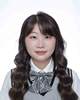
- Open Access
- Authors : Tianqiu Mao , Yidan Zhang , Xiaofeng Zhang
- Paper ID : IJERTV12IS050104
- Volume & Issue : Volume 12, Issue 05 (May 2023)
- Published (First Online): 17-05-2023
- ISSN (Online) : 2278-0181
- Publisher Name : IJERT
- License:
This work is licensed under a Creative Commons Attribution 4.0 International License
Influence of Pattern Composition Factors on Clothing Preference based on PAD Emotion Model
TianQiu Mao
College of Textiles and Clothing Engineering Soochow University
Suzhou215021China
Yidan Zhang
College of Textiles and Clothing Engineering Soochow University
Suzhou215021China
Xiaofeng Jiang
College of Textiles and Clothing Engineering Soochow University
Suzhou215021China
AbstractIn the current study, based on PAD emotion model, the type, size and density of clothing patterns were used as variables to explore the influence of pattern composition factors on individual emotional activation and apparel preference evaluation. The results showed that: Clothing pattern type has a significant effect on individual emotion and preference. The more positive the pattern type, the higher the stimulation of emotional pleasure and evaluation of the clothing preference; Clothing pattern size has a significant effect on individual emotion and clothing preference. The larger the pattern, the higher the arousal of emotional pleasure and evaluation of the clothing preference; Clothing pattern density has a significant effect on individual emotion and preference. Emotional arousal intensity shows an inverted U-shaped trend with the pattern variation from denseness to sparseness, and clothing preference evaluation is consistent with trends of pleasure; Emotional response is highly correlated with preferred evaluation, and emotional pleasure is highly positively correlated with clothing preference, that is, the higher the pleasure induced by the pattern, the higher the individual preference for clothing. This conclusion can help clothing designers to achieve a more scientific and emotional operation to meet the needs of consumers.
Keywordsclothing pattern; pattern composition; PAD emotion model; clothing preference
. INTRODUCTION
The ability of product design to adequately meet consumer needs has become a key to business success (Nagamachi 2002). Generally speaking, when consumers buy a new product before, their minds must have a psychological expectation of the product, such as practical, beautiful, exquisite attributes and so on. If the consumer's psychological expectations can be reflected in the new product, then the product will stimulate the consumer's emotional identity and will lead to the consumer be more satisfied with it. For example, Gan had drawn on the emotional needs of adolescents as a method for styling optometry products and found that using the emotional needs of users as a guide for product design can make it easier to meet their needs (Gan
2021). As with other product designs, the emotional needs of consumers are combined with design to promote the era of "emotional design" in apparel design.
In recent years, emotional demands are widely used in the field of clothing design (Gao 2010; Lv 2014; Chen 2015; Xu 2018; Kweon et al. 2004). In menswear design, the design elements of men's sports tops were divided, the PAD emotion model and ERPs were employed, and Lv obtained the relationship between the design elements of men's tops and the subjects' emotional feelings (Lv 2014); Kweon based on kansei engineering found that gender differences in users can lead to differences in the evaluation of different pajama fabrics (Kweon et al. 2004). Not much research has been done on the impact of clothing patterns based on emotional needs, mainly involving simple patterns such as stripes, polka dots and
checks (Zhu 2011; Li 2020; Shang 2020; Cao 2019)For
example, Shang used eye-tracking techniques and questionnaires to study subjects' preference for polka dot patterns and showed that subjects' gaze duration is highly correlated with clothing preference (Shang 2020).
From a cognitive psychology perspective, the PAD emotion model and E-Prime psychology experiment software were used to explore the influence of pattern composition factors (type, size and density) on consumer emotions and preferred evaluation in this paper, which facilitates clothing designers to design garments that better meet consumer needs.
. EXPERIMENT
-
Participants
Sixty school students participated in the experiment (30 males and 30 females), aged between 20 and 27 years. All subjects had normal vision and were in good physical and mental health, were right-handed, volunteered to participate in this experiment, and had never participated in any similar experiment before.
-
Stimulus materials
The patterns for this experiment were collected through the Vogue software for major clothing brand launches from 2021-2022. In this phase, 316 clear samples of garment
patterns were collected, 300 from garments and 16 from garment accessories. The collected samples were analyzed for single-element pattern composition factors as well as element frequency statistics to determine the three angles of pattern composition factors (type, size and density), and finally the rose, polka dot, butterfly and wolf patterns were selected as experimental design elements, as shown in Figure 1.
There are five pattern types: T1 (rose), T2 (polka dot), T3 (butterfly), T4 (wolf 1) and T5 (wolf 2), with three levels of pattern size: a (80 × 80 pixels), b (160 × 160 pixels) and c (240
×240 pixels), with a fixed pattern density of 1; and four levels of density: 1 (dense), 2 (medium dense), 3 (medium sparse) and 4 (sparse), with a fixed pattern size of a. All images were processed by CLO3D. A total of 30 stimulus images were obtained for this experiment, as shown in Figure 2 and Figure 3, with an image size of 600 × 900 pixels.
Fig.1 Elemental samples
Fig.2 Sample of pattern size variation
Fig.3 Sample of pattern density variation
-
Evaluation vocabulary
Chinese version of the PAD three-dimensional emotion scale (Li 2008) was chosen to assess the subjects' emotional responses to the experimental stimulus pictures, while preference items were added as an assessment of the subjects' degree of preference for clothing patterns, as shown in Table 1. The pleasure of the scale reflects the degree of polarization (positive-negative) of individual's emotion; the arousal reflects the individual's level of physiological activation and alertness; and the dominance reflects the individual's level of subjective control in different emotional states.
Tab.1 Evaluation items
Excited
Irritated
Sober
Sleepy
Excited
Clam
Interested
Relaxed
Surprised
Relaxed
Dominant
Controlled
Dominant
Submissive
Condescending
Humble
Influential
Influenced
Preference
Liked
Disliked
Arousal
Dominance
Each subdivision is a 9-point scale (-4-4, with 0 in the middle), with positive values greater than 0 and negative values less than 0. The greater the absolute value of its value indicates the stronger the identity of this emotion.
-
Experimental procedure
Subjects started the experiment after reading the instructions. Six pictures were used for practice before the formal experment to help subjects become familiar with the experiment. A 100ms "+" gaze point is displayed on the screen, then a 400ms white screen is presented, after the white screen disappears a 500ms random evaluation word pair appears, and after a 500ms white screen, a 900ms random picture of the clothing experiment stimulus is then presented. Subjects were asked to rate the degree of conformity of the pictures to the previously presented word pairs on a -4 to 4 keystroke scale, and a random 500ms-700ms white screen was presented after the response, and then they moved on to the next trial. A total of 408 clothing stimulus pictures were presented and the experiment lasted about 30 minutes. At the end of the experiment, the E-Prime program automatically stores detailed response data for the subjects.
. RESULES AND ANALYSIS
-
The PAD values and the categories analysis of the samples Based on the positive and negative data of the three dimensions of emotion, the subjects' emotions could be classified into eight different categories, and these eight different emotions were further classified into two categories, positive emotions and negative emotions, based on the positive and negative relationship of the emotional pleasure scores. The data obtained from the E-prime experiment were calculated to obtain the mean values of each evaluation item, and finally the PAD values for each sample were obtained and labelled with the emotion category to which they belonged, as shown in
Table 2.
Sample number
Pleasure
Arousal
Dominance
Emotional categories
T1a1
0.36
-0.10
0.12
Relaxed
T1a2
0.68
0.03
-0.28
Dependent
T1a3
0.83
-0.05
-0.22
Docile
T1a4
0.71
-0.20
-0.77
Docile
T1b1
1.14
0.20
0.35
Exuberant
T1c1
1.18
0.17
0.68
Exuberant
T2a1
-0.58
-0.40
-0.14
Bored
Negative
T2a2
0.17
0.01
-0.28
Dependent
Positive
T2a3
0.40
-0.24
-0.25
Docile
Tab.2 The PAD values and results of categories of 30 samples
Positive
Segmented indicators
Evaluation items
Positive
Negative
Energetic
Angry
Pleasure
Friendly
Dismissive
Exuberant
Pained
1.(C)
-0.971
0.146
-6.647
0.000**
-1.270
-0.672
Type
0.415
0.044
0.872
9.417
0.000**
0.325
0.505
T2a4
0.30
-0.24
-0.32
Docile
T2b1
0.26
-0.13
0.23
Relaxed
T2c1
0.27
0.01
0.28
Exuberant
T3a1
0.30
0.05
-0.01
Dependent
T3a2
1.10
0.42
0.10
Exuberant
T3a3
1.24
0.28
0.16
Exuberant
T3a4
1.01
0.05
-0.36
Dependent
T3b1
1.19
0.66
0.29
Exuberant
T3c1
1.28
0.78
0.81
Exuberant
T4a1
-0.60
0.23
0.32
Hostile
Negative
T4a2
0.04
0.82
1.17
Exuberant
T4a3
0.17
0.70
0.99
Exuberant
T4a4
0.40
0.58
0.62
Exuberant
Positive
T4b1
0.29
0.78
0.78
Exuberant
T4c1
0.17
0.86
0.85
Exuberant
T5a1
-0.90
0.44
0.98
Hostile
T5a2
-0.72
1.18
1.38
Hostile
T5a3
-0.40
1.13
1.24
Hostile
Negative
T5a4
-0.49
0.87
0.72
Hostile
T5b1
-0.73
0.92
1.30
Hostile
T5c1
-0.87
1.12
1.34
Hostile
-
Dependent: Pleasure; b. Predictors:Constant, Type
Combining the above information, the unstandardized coefficients were chosen as regression coefficients to establish the regression equation, as shown in Equation 1, with a correlation coefficient of 0.872 for the model, which be named as the fitness equation of pleasure and pattern composition factors. From the equation, it can be seen that the more positive the pattern type, the higher the pleasure score.
Pleasure=0.415Type-0.971 (1)
-
Analysis of pattern composition factors on arousal
The results of the evaluation of the arousal for the different pattern composition factor conditions were subjected to a repeated measures ANOVA, as shown in Table 6. Type, size and density all have significant main effects. (F Type=16.332, F Size= 10.979, F Density=6.402; P=0.000)
-
-
Analysis of patten composition factors on emotional evaluation
-
Analysis of pattern composition factors on pleasure
The results of the evaluation of the pleasure for the different pattern composition factor conditions were subjected to a repeated measures ANOVA, as shown in Table 3. Type, size and density all have significant main effects. (F Type=21.568, F Size=15.050, F Density=15.791; P=0.000)
Tab.3 Pleasure evaluation ANOVA table of different pattern composition
Source
SS
df
MS
F
Sig.
Type
109.433
2.662
41.107
21.568
0.000**
Size
23.195
1.647
14.084
15.050
0.000**
Density
20.080
1.997
10.058
15.791
0.000**
factors
Source
SS
df
MS
F
Sig.
Type
49.622
2.642
18.779
16.332
0.000**
Size
10.058
1.347
7.468
10.979
0.000**
Density
6.472
2.132
3.163
6.402
0.000**
Tab.6 Arousal evaluation ANOVA table of different pattern composition factors
**indicates significant correlation at the 0.01 level.
Correlation analysis was conducted between the arousal and pattern composition factors, as shown in Table 7. The table showed a moderate negative correlation between arousal
and type.|r|=0.551>0.5
Type
Size
Density
Pleasure
Type
1
Size
0.000
1
Density
0.000
-0.567**
1
Pleasure
-0.551**
0.270
-0.103
1
Tab.7 Correlation analysis between arousal and pattern composition factors
**indicates significant correlation at the 0.01 level.
Correlation analysis was conducted between the pleasure and pattern composition factors, as shown in Table 4. The table showed a high positive correlation between pleasure and
type.r=0.8720.8
Tab.4 Correlation analysis between pleasure and pattern composition factors
**indicates significant correlation at the 0.01 level.
Regression analysis was used to establish the relationship between arousal and pattern type. The regression model coefficients are shown in Table 8, where the contribution of type to pleasure is 0.551.
Tab.8 The model coefficient analysis table
Type
Size
Density
Pleasure
Type
1
Size
0.000
1
Density
0.000
-0.567**
1
Pleasure
0.872**
0.137
0.143
1
UC SC
Model
95%
Confidence
**indicates significant correlation at the 0.01 level.
Regression analysis was used to establish the relationship between pleasure and pattern type. The regression model coefficients are shown in Table 5, where the contribution of type to pleasure is 0.872.
UC
SC
95%
Confidence
Model
B
Error
Beta
t
Sig.
Lower
Upper
Tab.5 The model coefficient analysis table
B Error Beta t Sig. Lower Upper
1.(C) 0.902 0.170 5.300 0.000** 0.554 1.251
Type -0.179 0.051 -0.551 3.494 0.002** -0.284 -0.074
a. Dependent: Arousal; b. Predictors:Constant, Type
Combining the above information, the unstandardized coefficients were chosen as regression coefficients to establish the regression equation, as shown in Equation 1, with a correlation coefficient of 0.551 for the model, which be named as the fitness equation of arousal and pattern composition factors. From the equation, it can be seen that the more positive the pattern type, the lower the arousal score.
Arousal=-0.179Type+0.902 (2)
c. Analysis of pattern composition factors on dominance The results of the evaluation of the dominance for the
different pattern composition factor conditions were subjected to a repeated measures ANOVA, as shown in Table 9. Type, size and density all have significant main effects. (F Type=13.012, F Size=5.473, F Density=3.909; P Type=0.000,
P Size=0.014, P Density=0.024)
Tab.9 Dominance evaluation ANOVA table of different pattern composition
Source
SS
df
MS
F
Sig.
Type
71.219
2.842
25.064
13.012
0.000**
Size
8.834
1.334
6.622
5.473
0.014*
Density
7.298
1.932
3.778
3.909
0.024*
factors
Source
SS
df
MS
F
Sig.
Type
104.854
2.997
34.992
13.474
0.000**
Size
31.414
1.609
19.529
16.361
0.000**
Density
31.422
2.432
12.918
8.577
0.000**
Tab.12 Preferred evaluation ANOVA table of different pattern composition factors
**indicates significant correlation at the 0.01 level.
Correlation analysis was conducted between the preference and pattern composition factors, as shown in Table 13. The table showed a high positive correlation between preference
and type.r=0.802>0.8
Type
Size
Density
Pleasure
Type
1
Size
0.000
1
Density
0.000
-0.567**
1
Pleasure
0.802**
0.163
0.195
1
Tab.13 Correlation analysis between preference and pattern composition factors
**indicates significant correlation at the 0.01 level; *indicates significant correlation at the 0.05 level.
Correlation analysis was conducted between the
dominance and pattern composition factors, as shown in Table
10. The table showed a moderate negative correlation between dominance and type.|r|=0.674>0.5
Tab.10 Correlation analysis beteen dominance and pattern composition
factors
**indicates significant correlation at the 0.01 level.
Regression analysis was used to establish the relationship between preference and pattern type. The regression model coefficients are shown in Table 14, where the contribution of type to preference is 0.802.
Tab.14 The model coefficient analysis table
Type
Size
Density
Pleasure
Type
1
Size
0.000
1
Density
0.000
-0.567**
1
Pleasure
-0.674**
0.359
-0.319
1
UC SC
Model
95%
Confidence
1.(C)
-1.167 0.192
-6.080 0.000** -1.560
-0.774
Type
0.411 0.058
0.802
7.094 0.000** 0.292
0.529
B Error Beta t Sig. Lower Upper
**indicates significant correlation at the 0.01 level.
Regression analysis was used to establish the relationship between dominance and pattern type. The regression model coefficients are shown in Table 11, where the contribution of type to dominance is 0.674.
Tab.11 The model coefficient analysis table
95%
-
Dependent: Preference; b. Predictors:Constant, Type
Combining the above information, the unstandardized coefficients were chosen as regression coefficients to establish the regression equation, as shown in Equation 1, with a correlation coefficient of 0.802 for the model, which be named as the fitness equation of preference and pattern composition
factors. From the equation, it can be seen that the more
Model
UC SC
Confidence
positive the pattern type, the higher the preference score.
B Error Beta t Sig. Lower Upper
1.(C)
1.242 0.192 6.470 0.000** 0.849
1.635
Type
-0.280 0.058 -0.674 -4.834 0.000** -0.398
-0.161
-
Dependent: Dominance; b. Predictors:Constant, Type
-
Combining the above information, the unstandardized coefficients were chosen as regression coefficients to establish the regression equation, as shown in Equation 1, with a correlation coefficient of 0.674 for the model, which be named as the fitness equation of dominance and pattern composition factors. From the equation, it can be seen that the more positive the pattern type, the lower the dominance score.
Dominance=-0.280Type+1.242 (3)
-
-
-
Analysis of pattern composition factors on preferred evaluation
The results of the evaluation of the preference for the different pattern composition factor conditions were subjected to a repeated measures ANOVA, as shown in Table 12. Type, size and density all have significant main effects. (F Type=13.474, F Size=16.361, F Density=8.577; P=0.000)
Preference=0.411Type-1.167 (4)
-
Analysis of the association between PAD and preferred evaluation
Correlation analysis was conducted between PAD and preference, as shown in Table 15. The table showed a high
positive correlation between pleasure and preference r=0.932>0.8 , and a low positive correlation between dominance and preference.|r|=0.489>0.3
Tab.15 Correlation analysis between PAD and preference
Type |
Size |
Density |
Pleasure |
|
Type |
1 |
|||
Size |
-0.346 |
1 |
||
Density |
-0.496** |
0.888** |
1 |
|
Pleasure |
0.932** |
-0.296 |
-0.489** |
1 |
**indicates significant correlation at the 0.01 level; *indicates significant correlation at the 0.05 level.
Regression analysis was used to establish the relationship
between pleasure and preference. The regression model
coefficients are shown in Table 16, where the contribution of type to Pleasure is 0.932.
Tab.16 The model coefficient analysis table
95%
[9] Nagamachi, Mitsuo. 2002. Kansei engineering as a powerful consumer-oriented technology for product development, Applied Ergonomics, 33: 289-94. [10] Shang LihuaZhao YuxiaoRen HaidongLiu XiaoyiGeng Xianghuan,.Model
UC SC
Confidence
B Error Beta t Sig. Lower Upper
2020. Research on Visual Evaluation of Knitted Skirt Pocar Dot Based on Eye Tracker, Journal of Beijing Institute of Fashion
1.(C) -0.210 0.053 -3.992 0.001** -0.319 -0.100
Type 1.003 0.074 0.932 13.636 0.000** 0.853 1.154
a. Dependent: Preference; b. Predictors:Constant, Pleasure
Combining the above information, the unstandardized coefficients were chosen as regression coefficients to establish the regression equation, as shown in Equation 1, with a correlation coefficient of 0.932 for the model, which will be named as the fitness equation of PAD and preference. From the equation, it can be seen that the more score of the pleasure, the higher the preference score.
Preference=1.003Pleasure-0.210 (5)
. CONCLUSION
The PAD emotion model was employed, and pattern composition factors (type, size and density) were used as variables in this study to systematically explore the influence of pattern composition factors on individual emotional activation and apparel preference evaluation. The results showed that pattern composition factors influence individuals' emotions and clothing preferences. In particular, the type of pattern has the most significant effect on emotion and preference evaluation; Emotional response is highly correlated with preferred evaluation, and emotional pleasure is highly positively correlated with clothing preference, i.e., the higher the pleasure induced by the pattern, the higher the individual preference for clothing. In short, designers can design garments that better match consumers' emotional needs and aesthetic preferences through a scientific scheme of pattern composition factors.
REFERENCES
[1] Chen ting. 2015. the perceptual study of women's suit lapel types based on psychological experiment, [d], soochow university. [2] Gan yan, ji yingrui, shi yuzhe, liu xinxiong. 2021. method and application of user kansei perception and product kansei design, packaging engineering. [3] Gao chuan. 2010. the study on emotional design and kansei engineering system's development of office suit, [d] ,shanghai university engineering science. [4] Zhu jiani ,shi jianping. 2011. a study of striped knitwear based on the perception of the wearer, knitting industry: 53-56. [5] Kweon, Soo-Ae, Eun-Kyung Lee, and Jong-Myoung Choi. 2004. A comparative study on the subjective fabric hand according to gender for winter sleepwear fabrics, Fibers and Polymers, 5: 6-11. [6] Li Xiaoming, Fu Xiaolan, Deng Guofeng.2008.Preliminary Application of the Abbreviated PAD Emotion Scale to Chinese Undergraduates,Chinese Journal of Mental Health, 22: 327-29.
[7] Li Yang, Wu Jing. 2020. Correlation between consumers perceptual cognition of garment polka dot patterns and design elements, Journal of Textile Research, 41: 132-26. [8] Lv Jia. 2014. A Study of Event-Related Potentials Technique Applied on Clothing Emotion, [D], Jiangnan University.Technology( Natural Science Edition), 40: 69-75.
[11] Xu Bing. 2018. The Study of Clothing Image Affecive Computing Based on Emotion Measyrements –Taking Costume Patterns in Ming And Qing Dynasty As An Example , [D], Donghua University. [12] Cao Zhen, Su ZhaoweiWei Yuhui. 2019. Application of eye movementtracking technology in the pattern design of sweater for middle-aged and elderly person, Wool Textile Journal, 47: 71-74.