
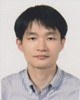
- Open Access
- Authors : Ki-Seok Choi
- Paper ID : IJERTV12IS080039
- Volume & Issue : Volume 12, Issue 08 (August 2023)
- Published (First Online): 26-08-2023
- ISSN (Online) : 2278-0181
- Publisher Name : IJERT
- License:
This work is licensed under a Creative Commons Attribution 4.0 International License
Effective Demand Forecast in Supply Chain Management: Methodology and Measure
Ki-Seok Choi
Department of Industrial and Management Engineering Hankuk University of Foreign Studies
Yongin, Korea
Abstract Due to the importance of demand forecasting to provide basic data for a series of activities for matching demand and production in supply chain management, several methodologies have been adopted for improving effectiveness of demand forecasting. In general, the demand forecasting error measure is widely used as a method to measure how accurate demand forecasting is. In this paper, we look at the points to be considered when evaluating the performance of demand forecasting methodologies based on the demand forecasting error measure.
Keywords supply chain management; demand forcast
-
INTRODUCTION
Demand forecasting is one of the key activities that enable other supply chain operation activities, such as production planning and raw material supply planning, by providing basic data for sales and production. Depending on demand forecasts, manufacturers decide how many products to make, how much production capacity they need, and how many raw materials to purchase. The activity of forecasting demand, which is highly influenced by external factors beyond our control, is inherently imprecise. Inaccurate demand forecast results result in excessive inventory costs or lost sales opportunities. These costs must be reduced to remain competitive in today's business environment, where customer needs change rapidly and product life cycles are short. Therefore, supply chain managers put a lot of effort into monitoring and controlling forecasting errors to improve forecasting accuracy. In supply chain management, it is common to measure the accuracy or error of demand forecasts using a single measure. Several methods have been proposed to manage forecast errors by quantifying the extent to which actual sales volume deviate from forecast. In this paper, we first classify the difference between demand forecast and actual sales into several types. Distinguishing demand forecasting errors by cause can help you find areas to focus on and improve to improve demand forecasting. Next, problems that may occur when calculating the demand error using the demand forecast error measure are examined. As mentioned earlier, when a single measure is used for the ease of managing demand forecasting activities, unexpected problems may occur by comprehensively calculating demand forecasting errors by product, period, and cause. Demand forecasting activities should be managed with this in mind.
In order to establish a frequency allocation policy targeting radio resources owned by the state, for example, first we need to clarify the target time, derive information and communication services using radio resources expected to be provided at the target time, and consider the characteristics of the service and the scale of demand. Therefore, it is necessary to calculate the
optimal frequency band and frequency requirements. Globally, individual companies, industries, and countries recognize radio resources as an indispensable element in enhancing productivity, economic growth, and securing national competitiveness through communication facilities and services, and due to this importance, the demand for radio spectrum is rapidly increasing. It has been growing rapidly and is expected to increase even more rapidly in the future. This growth is attributable to the rapid growth in demand for mobile communication services driven by technological development and economic growth that can provide a variety of services. Demand has already exceeded supply in some bands, and similar situations are expected to occur in other bands in the near future. Therefore, it is urgently needed to develop a frequency band calculation method suitable for the characteristics of the services and a methodology for predicting long-term frequency demand. In particular, it is urgently required to prepare objective standards in setting frequency bands by predicting the market size according to the type of wireless communication service and evaluating the value of each service at the same time.
-
DEMAND FORECASTING METHODOLOGY
-
Delphi technique
The Delphi technique is a qualitative demand forecasting methodology proposed by O. Helmer in 1950. It is based on the
hypothesis that "two people's opinions are more accurate than one". It is mainly used for mid- to long-term forecasts where related data are insufficient, such as new product development, new market development, and new equipment acquisition.
It is suitable for forecasting the future and estimating conflicts among interest groups, collects opinions from a large number of people, and has the advantage of being easy and simple to implement. However, it has the disadvantage that it takes a lot of time and the variation according to the composition of experts is severe.
Key considerations when applying the Delphi technique include defining the problem to be estimated as narrowly and specifically as possible and preventing panelists from leaving due to repetition of the procedure. In addition, when selecting a panel, the representativeness of the panel, appropriateness and qualifications, responsible participation, independence of response, individual differences in panel, and characteristics of non-responders should be considered. As a scale for measuring survey responses, a Likert scale, a 5-point/7-point scale, a median, a percentile, and a rank are used.
-
Market research
Market research means to study the entire group by selecting and examining samples from large and small groups in order to discover the existence, distribution, and correlation of social or psychological variables. This includes phone/interview surveys, questionnaire surveys, opinion gathering at consumer meetings, and trial sales.
It is useful when it is difficult to present objective data, such as new business or new product development, and can be easily predicted by synthesizing the opinions of a small number of experts or members. It has advantages such as being able to obtain a large amount of information from the system and obtaining generally accurate information and being easy to apply to small and medium-sized businesses due to its low cost. On the other hand, there is a possibility that superficial results may be derived depending on the subjectivity of members, different prediction results are presented according to individual abilities, insights, and experiences, and it is difficult to accurately judge between study variables, and accuracy is poor due to insufficient objective data analysis. It has disadvantages such as high reliance on investigators' abilities/skills, and in some cases requiring a lot of time and money.
There are unstructured open-response questionnaires and structured non-free-response questionnaires. The open-response questionnaire is used when the researcher has limited knowledge on a specific question and wants to obtain a wide range of responses from the respondent, when the range of responses is expected to be large, and when the respondent's voluntary response is of interest. On the other hand, there are disadvantages such as difficulty in handling questionnaires, difficulty in recording all of them, and difficulty in analyzing data.
-
Quantitative forecasting
Quantitative prediction methods include micro-model and macro-model methods. The micro-model method creates a causal model that mathematically represents a causal relationshi, predicts demand, and constructs a causal prediction model by relating the internal and environmental factors(variables) of the company that affect demand change to demand.
Macro model methods include time series model, diffusion model, and Bass model. Time series analysis predicts future demand by identifying trends or trends from past data(demand, sales, etc.) presented along time series(time intervals such as years, months, weeks, days, etc.). In this case, the independent variable is time and the dependent variable is quantity demanded. The focus of time series analysis is the time series related to the demand generated in the past, and it is used when time series data collection is easy, has a clear tendency to change, and is stable.
-
-
TYPES OF DEMAND FORECASTING ERRORS
In general, demand forecasting is handled by the marketing/sales department, which has frequent contact with customers and has a good understanding of the market. Depending on product type, market size, and sales volume, marketing/sales departments typically have multiple segments for demand forecasting. For example, demand forecasting can be performed by configuring segments for each product, or by
grouping several products into product groups and then configuring segments for each product group to perform demand forecasting. How to construct demand forecast segments is usually closely related to a company's marketing strategy. Once the demand forecast segments are created, the marketing/sales department conducts periodic forecasts for each segment. Since demand forecasting is one of the input data for production planning, the demand forecasting period is generally chosen to be the same as the production planning period.
Although it may be different depending on the situation of each company, in this paper, we try to classify the demand forecast error by type considering the situation in which the manufacturing department establishes a production plan by considering demand forecast information and other information such as production process capacity. The production plan established by the manufacturing department is notified to the marketing/sales department in the form of a ReTurn of Forecast(RTF). The RTF can sometimes be less than the demand forecast because there are other constraints(production process
capability, for example) taken into account in production
planning. After receiving the RTF, the marketing/sales department will develop a marketing and sales plan to sell as much as the RTF. At the same time, the manufacturing department makes an effort to produce as much as RTF, which is the production plan quantity, and provide it to the sales department. If the manufacturing department is unable to produce the target volume for some reason, the marketing/sales department may not have enough product to sell. Here, two cases can arise where demand forecasts differ from actual sales volume. The first case is when the RTF is smaller than the demand forecast(Type 1), and the second is when the actual production is smaller than the RTF(Type 2). Here, the actual sales volume means the available quantity (Available To Sale: ATS) that the marketing/sales department can actually sell. In reality, there are cases where the actual production volume and ATS of the manufacturing department are different due to reasons such as transportation delays and carryover inventory.
Looking at a common cause of this forecast error, the difference between demand forecast and RTF(Type 1) is that the manufacturing department's production capacity is not sufficient to meet all the demand forecast. This is common for popular products with very high aggregate demand and creates a tricky allocation problem to determine how to distribute limited production quantities to different marketing/sales segments(usually regional). Whichever allocation rule is used, it is inevitable that there will be marketing/sales segments that receive less RTF than demand forecast. If the manufacturing department produces fewer quantities than the RTF, and thus a demand forecasting error occurs(Type 2), it is because various uncertainties occur during the manufacturing process. If it is not possible to produce as planned by the promised time due to factors such as equipment failure, discontinuous supply of materials, or problems with the distribution network, a quantity less than the RTF will be supplied for the amount of insufficient production. Even in this case, an allocation problem may arise in determining which marketing/sales segment to supply and how much less than the RTF.
ATS is the quantity of product available that the marketing/sales department can satisfy customer orders. Actual sales volume cannot exceed this ATS, even if there is a greater demand for the product. On the other hand, if the demand is not
as great as the ATS, a different type of forecasting error occurs. This forecasting error(Type 3), which occurs when ATS exceeds actual sales, is usually caused by the marketing/sales department making higher-than-actual demand forecasts. In particular, if the marketing/sales department does not pay attention to demand forecasting and makes a demand forecast under the assumption that it can sell all the supplied quantity, and the manufacturing department has sufficient production process capacity to produce as much as the demand forecast, this demand forecasting error type arises.
Unlike Types 1, 2, and 3 of demand forecast error discussed so far, the last Type 4 occurs when demand is underestimated, and actual sales are higher than expected. Such underforecasting, depending on management's attitude, may receive less attention than overforecasting, which forecasts more volume than actual demand. This is because they are satisfied that actual sales have exceeded forecasts and that sales have increased. On the other hand, if the supply chain is designed and operated to maintain a low level of safety stock, the error in demand forecasting by Type 4 will not be large. The reason is that this type of demand forecasting error occurs when there is an underestimation of demand, i.e., when the actual sales volume is greater than the forecasted demand. This is because the maximum level of ATS is the sum of demand forecast and safety stock. In other words, in this case, since the actual sales volume exceeding the demand forecast amount is about the maximum safety stock quantity, the demand forecast error will be limited to the safety stock quantity. If the Type 4 error due to underestimation of demand is large, the current supply chain performance should be closely evaluated, and production and inventory policies should be revised.
-
MEASURES OF DEMAND FORECASTING ERROR
In companies paying attention to supply chain management, the accuracy of demand forecasting is one of the key indicators to measure the performance of the marketing/sales department, the department in charge of forecasting demand. The ultimate goal of supply chain management activities is to match demand and supply, and demand forecasting activities are the starting point for all efforts to achieve this goal. It is important for marketing/sales departments to establish sales/sales plans and carry out marketing activities according to the available supply (RTF) presented by the manufacturing department, but demand forecasts, which are used as basic data for all production/supply plans, are more reliable. It would be desirable in terms of overall supply chain management to make the utmost effort in demand forecasting so that data is available. As mentioned in the introduction, quantified measures are often used to measure the performance of a department for the convenience of business management. These performance measures or indicators will have to be carefully designed to measure how effectively the task being measured is working to achieve its intended goals.
As discussed in the previous section, demand forecast errors can be caused by a combination of various types of errors, and the responsibility for each type of error cannot all be attributed to the marketing/sales department. However, in most companies, demand forecasting is recognized as a major task of the marketing/sales department, so the fact that all departments in the supply chain must work together to improve demand forecasting accuracy is overlooked. It is common to use the performance of only the marketing/sales department as an evaluation index. Among the errors classified in the previous
section, in the case of the types corresponding to excessive demand forecasting (Types 1, 2, and 3), forecasting accuracy may be reduced due to factors outside the marketing/sales department. For example, even if the marketing/sales department accurately identifies customer needs and requests the manufacturing department to supply the exact amount of demand, if the supply is not sufficient from the manufacturing department, Type 2 or 3 demand error occurs, resulting in a demand forecast error. Accuracy is low and consequently degrades the performance metrics of the marketing/sales department. A desirable demand forecasting error measure should be designed so that the responsibility for the error can be easily recognized, and the exact reason can be found among the possible causes of the error.
In this regard, the Absolute Deviation Rate (ADR), which is widely used as a measure of demand forecast error, has several problems. The demand forecast error using the ADR measure is defined as the absolute difference between the actual demand and the demand forecast divided by the demand forecast.
The most prominent problem with the ADR measure is that it does not distinguish between excessive demand forecast errors (types 1, 2, and 3) and under-demand forecast errors. In other words, even if the same measure of demand forecasting error is observed, it is impossible to distinguish whether the error is caused by more or less than actual sales volume, so it is an activity to recognize the cause of the demand forecasting error and prepare a direction for improvement. The usability for This problem can be solved simply by using the simple difference value instead of the absolute value of actual sales volume and forecast demand used when calculating the ADR measure. In this case, an error of a positive value indicates an underestimation of demand, and an error of a negative value indicates an overestimation of demand. However, as can be easily expected, the error measure using the simple difference value has limitations when evaluating various demand forecasts comprehensively. For example, if an error of +10% occurs in the first demand forecast and an error of -10% occurs in the second demand forecast, the two demand forecast error measures are used to evaluate the two demand forecasts as a whole. A simple summation measures 0% error, or 100% demand forecasting accuracy achieved. Because of these offsetting effects, the ADR measure is more widely used despite the disadvantages described above.
In addition to the disadvantage of being unable to distinguish between overestimation and underestimation, ADR measures have other disadvantages that make it difficult to measure the performance of demand forecasting activities efficiently. As explained in the introduction, companies that sell multiple products in multiple regions perform demand forecasting by dividing them into multiple segments according to the type of sales organization. For example, if there are two segments for each product, two demand forecasting errors are calculated for each segment. Each segment will evaluate performance using the corresponding demand forecasting error, but in order to measure the performance of the entire marketing/sales organization, a method of calculating demand forecasting error by integrating demand forecasting by segment must be prepared. An easily conceivable method is to use a simple average of segment-specific demand forecasting errors as the overall demand forecasting performance indicator. In this case, an error in a segment with a small amount of demand forecast can have a significant effect on the overall performance. For example,
suppose that the forecasted demand for product A is 1 and the actual sales volume is 0, and the forecasted demand for product B is 100 and the actual sales volume is 90. At this time, the demand forecasting error for product segment A is 100%, the demand forecasting error for product segment B is 10%, and the simple averaged overall demand forecasting error is 55%. As can be seen through the example, an undesirable phenomenon can occur in which the demand forecasting error of a segment, which has a much smaller demand forecasting volume and generally accounts for a smaller portion of the total sales volume, greatly affects the overall demand forecasting performance. Therefore, in general, weights according to the ratio of demand forecasts are used when summing the demand forecasting errors of several segments. In the example above, the total demand forecasting error considering weights is (1/101)x100% + 100/101 x 10% = 10.9%. However, when the demand forecasting errors for each segment are added using weights according to the demand forecasting quantity, another disadvantage arises in that the difference between the demand forecasting for each segment and the actual sales volume is not considered. For example, if there are 10 differences between demand forecast and actual sales volume, the same demand forecast error is measured regardless of which segment and how many of these 10 differences occur. Considering the ultimate goal of supply chain management, which is to match supply and demand, this demand forecasting error measure that does not identify which product or region has a discrepancy between demand and supply has become less useful.
-
CONCLUDING REMARKS
The goal of reducing demand forecasting errors is to reduce overall costs by achieving efficiency throughout the entire supply chain. If customer demand is accurately predicted and ideal supply chain management in which products are produced and supplied in a timely manner is achieved, the error in demand forecasting will be maintained at a very low level. In this case, the measure used to measure the demand forecasting error will not have a significant impact on the level of demand forecasting. However, it is not easy to judge that the supply chain has ideal efficiency just because the demand forecasting error level is measured low when a specific measure is used, and additional analysis is needed to make an accurate judgment. This is because there are limitations in representing the performance of all demand forecasting activities with simple indicators due to the problems of the demand forecasting error measures as discussed in this paper.
If the performance of demand forecasting activities is evaluated using only measures that can be simply expressed for the convenience of management, it can remain only in one- dimensional management activities ignoring interdependencies among departments in the entire supply chain. If a serious error occurs in demand forecasting, the type and cause must be quickly and clearly identified so that appropriate measures can be taken by the appropriate department to improve demand forecasting activities. In this respect, it may be considered to design a separate performance indicator that can lead each department to play its proper role so that better demand forecasting can be achieved at the level of the entire supply chain. It is not possible to identify and measure the different types of errors discussed in this paper with a single measure, nor is it desirable to measure the performance of multiple supply chain functions with the same measure. However, from a management perspective, designing and applying multiple performance indicators can be cumbersome and inefficient. Analyzing the pros and cons of each method and finding ways to tailor each supply chain function to achieve optimal demand forecasting are areas for further research.
REFERENCES
[1] J. S. Armstrong, Error easures for generalizing about forecasting methods: empirical comparisons, Int. J. of Forecasting, vol. 8, 1992, pp.69-80. [2] Y. Aviv, The effect of collaborative forecasting on supply chain performance, Management Sci., vol. 47, 2001, pp. 1326-1343. [3] D. Bovet and Y. Sheffi, The brave new world of supply chain management, Supply Chain Management Review, Spring 1998, pp. 14- 22. [4] R. Fildes and B. Kingsman, Incorporating demand uncertainty and forecast error in supply chain planning models, J. of Oper. Res. Society, vol. 62, 2011, pp.483-500. [5] M.L. Fisher, What is the right supply chain for your product? Harvard Business Review, March-April 1997, pp. 105-116. [6] R. J. Hyndmana and A. B. Koehler, Another look at measures of forecast accuracy, Int. J. of Forecasting, vol. 22, 2006, pp.679-688. [7] C. L. Jain, How to measure the cost of a forecast error, J. of Business Forecasting Methods & Sys., vlo. 22, 2004, pp.2-30. [8] G. Stalk, P. Evans, and L.E. Shulman, Competing on capabilities: the new rule of corporate strategy, Harvard Business Review, March-April 1992, pp. 57-69. [9] T. Troyer and D. Denny, Quick-response evolution, Discount Merchandiser, vol. 32, 1992, pp. 104-107. [10] C. Troyer and R. Cooper, Smart moves in supply chain integration, Transportation & Distribution, vol. 36, 1995, pp. 55-62.