
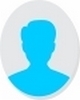
- Open Access
- Authors : Monther H Tobaigy, Osman Taylan, Mustafa Tahsin Yilmaz
- Paper ID : IJERTV13IS050073
- Volume & Issue : Volume 13, Issue 05 (May 2024)
- Published (First Online): 14-05-2024
- ISSN (Online) : 2278-0181
- Publisher Name : IJERT
- License:
This work is licensed under a Creative Commons Attribution 4.0 International License
Design and Analysis of a Response Surface Model to Realize the Mechanical Properties of Stainless Steel in Additive Manufacturing
Monther H Tobaigy
Department of Industrial Engineering King Abdulaziz University
Jeddah, Saudi Arabia
Osman Taylan
Professor, Department of Industrial Engineering King Abdulaziz University
Jeddah, Saudi Arabia
Mustafa Tahsin Yilmaz
Professor, Department of Industrial Engineering King Abdulaziz University
Jeddah, Saudi Arabia
AbstractAdditive manufacturing which is known as 3D printing, has revolutionized the production of complex structures, particularly in the field of materials science. This study investigates the impact of various manufacturing and post- manufacturing parameters on the mechanical properties and weight of stainless-steel specimens produced using additive manufacturing technology. The study provides insights into the effect of inner cell dimension, wall thickness, inner cells orientation angle, 3D printing orientation, surface roughness, and heat treatment on tensile strength, yield strength, Youngs modulus, strain, and weight. Also, it provides insights about the optimization of the factors to achieve the best mechanical properties with the least weight. The findings of this study contribute to the understanding of the additive manufacturing process and its optimization for stainless steel, paving the way for more efficient and cost-effective production in the future.
Keywords Stainless Steel, Response Surface Methodology, Additive Manufacturing, Design of Experiment
-
INTRODUCTION
The manufacturing landscape has been significantly transformed with the emergence of additive manufacturing, colloquially known as 3D printing. This innovative technology has unlocked new frontiers in the realm of materials science, enabling the fabrication of intricate structures with unprecedented precision and repeatability. Additive Manufacturing provides widespread applications across various industries, including aerospace, automotive, healthcare, and consumer goods. One of the key materials extensively utilized in AM processes is stainless steel, renowned for its superior mechanical properties, corrosion resistance, and versatility.
Stainless steel has been increasingly used in architectural and structural applications because of their superior corrosion resistance, ease of maintenance and pleasing appearance. The mechanical properties of stainless steel are quite different from those of carbon steel. For carbon and low-alloy steels, the proportional limit is assumed to be at least 70 % of the yield
point, but for stainless steel the proportional limit ranges from approximately 36 % – 60 % of the yield strength. [15]
Several applications already exist worldwide for structural and non-structural components made of SSs, all these steels are alloys of iron, chromium, nickel and to varying degrees molybdenum. The characteristic corrosion resistance of stainless steel is dependent on the chromium content and is enhanced by additions of molybdenum and nitrogen. Nickel is added, primarily, to ensure the mechanical properties and the correct microstructure of the steel. Other alloying elements may be added to improve particular aspects of the stainless steel such as high temperature properties, enhanced strength or to facilitate particular processing routes [1].
In stainless steel additive manufacturing, optimizing process parameters to achieve desired material properties and printing quality is vital. Response Surface Methodology (RSM) is a powerful tool in this endeavor, offering a systematic approach to experiment design, process optimization, and performance prediction.
RSM enables researchers and engineers to explore the complex interplay between multiple process variables and the desired outcomes. By statistically modeling these relationships and conducting systematic experiments, RSM facilitates the identification of optimal process settings that maximize material performance while minimizing production costs and time. [14]
Several studies have successfully applied RSM in the field of materials science. For instance, [10] the researcher used RSM to optimize the heat treatment process of stainless steel, resulting in improved hardness and tensile strength. Similarly,
[11] employed RSM to study the effect of welding parameters on the mechanical properties of stainless steel.This research enlightens the exploration of additive manufacturing, with a specific focus on the production of stainless-steel specimens. The core of the research is to understanding the influence of several adjustable manufacturing and post-manufacturing parameters on the
mechanical attributes and weight of the additive manufactured products to optimize the production by employing a well- recognized scientific methodology which is Response Surface Methodology.
The research pivots around several key parameters, including the inner cell dimension, wall thickness, inner cell orientation angle, surface roughness, and heat treatment. These parameters play a pivotal role in the manufacturing process and are anticipated to significantly impact the final products properties. The responses of the experiment, which serve as the dependent variables in this study, encompass tensile strength, yield strength, Youngs modulus, strain, and weight. These responses provide a comprehensive understanding of the mechanical behavior of the specimens under different conditions. The goal of the experiment is to reduce cost and weight of the manufactured objects by partially evacuating the inner structure via an inner net of structured cells.
Adopting a well-recognized scientific methodology such as response surface methodology which lies under the big umbrella of statistical design of experiment is a strong approach to study and optimize an industrial problem or process. This study will enlighten some areas for other researchers to examine and study furthermore parameters and factors that affect the manufacturing processes in the field of additive manufacturing specially 316L stainless steel manufacturing.
Targeting a metal additive manufacturing technology to apply an experiment on could be challenging because its a modern technology in comparison to conventional machining, its hard to obtain and operate because of its limited manufacturers and innovators worldwide and the rareness of powder raw material suppliers. Previous challenges should be taking into consideration before adopting such technologies. In addition, the journey of this research faced many obstacles during design in understanding the ability and adjustable parameters of the machine that produces the product and communication with the manufacturers of the machine to ensure eliminate any factor that may harm the manufacturing process. Also the machines availability and dedication to the research was a challenge since the study were conducted in a manufacturing environment not in a research institute.
-
LITERATURE REVIEW
The mechanical properties of stainless steel, such as tensile and yield strength, Youngs modulus, and strain are critical for many applications. These properties can be influenced by various factors, including manufacturing parameters and post- manufacturing treatments. Therefore, a comprehensive understanding of properties and factors affecting them is essential.
The stress-strain behavior of duplex and austenitic steels in a tensile test differs from that of carbon steels. Stainless steels are also characterized by:
A high degree of plasticity between the proof stress and the ultimate tensile stress.
-
Very good low-temperature toughness.
-
A degree of anisotropy
-
METHOD AND METHODOLOGY
The advent of additive manufacturing, colloquially known as 3D printing, has ushered in a new era in the field of materials
science. This innovative technology has revolutionized the production of complex structures and made a significant stride in the field of materials science, enabling the creation of intricate designs with a high degree of precision and repeatability.
Additive Manufacturing (AM) provides the capability to relax the design and manufacturing constraints by creating products with advanced geometrical complexity and without the need for extensive machining. [2-3].
The AM technique allows for the creation of three-dimensional shape structures through a layer-by-layer process [4]. Three- dimensional computer-aided design (CAD) programs are used to design components with complex shapes that could not be manufactured via conventional processes such as casting or forging [5]. Among the AM techniques, selective laser melting (SLM) is the most versatile, allowing for the creation of functional parts with mechanical properties similar to those of conventionally produced materials [6]. The wide variety of materials that can be produced, together with the low surface roughness achieved, are what differentiates this technique from other production methods [7]. The building process is achieved by the successive consolidation of molten powder layers. A high-temperature laser beam melts the powder of the first layer (from 0.02 mm to 0.1 mm) [1]. The second layer of metal powder is spread out over the surface, and the same operation is repeated until the building process is completed. However, this technique still presents some challenges that must be addressed in order to improve the performance of the manufactured parts [8].
Response Surface Methodology is a collection of statistical and mathematical techniques useful for developing, improving, and optimizing processes. It is a method used to model and analyze problems in which a response of interest is influenced by several variables and the objective is to optimize this response. The heart of RSM lies in the development of mathematical models that reflect the relationship between the factors and the responses. They are the keys to unlocking the optimal levels of the factors that maximize or minimize the responses [12].
To elaborate more about the impact and benefits of adopting RSM methodology in industrial applications some studies used RSM as a very beneficial tool. According to the researcher by applying Response Surface Methodology (RSM) the experiment had a structured framework for efficiently traversing the multidimensional parameter space, by this means augmenting the comprehension of the process dynamics and facilitating its optimization. On the other hand, leveraging predictive models derived from RSM successfully identified the optimal combination of culture conditions, yielding significant enhancements in both product yield and purity. A significant advantage is the graphical representations afforded by RSM, including contour plots and response surface plots, served as invaluable tools for visually interpreting the relationships between process variables and response variables, thereby aiding in informed decision-making and process optimization. By systematically varying culture parameters within the experimental domain defined by RSM allowed the researcher to uncover optimal operating conditions, maximizing desired biotransformation while minimizing undesirable by-products. [13]
According to the researcher by utilizing Response Surface Methodology, the study analytically optimized and enhanced the efficiency of the process. It functioned as the most valuable tool in the analysis, enabling to model the complex relationships between different factors and the quality of phenolic-rich cinnamon extracts. The study utilized Response Surface Methodology to methodically investigate how varying extraction parameters impacted both the quantity and biological activity of phenolic extracts from cinnamon, offering significant insights beneficial to the food industry. Also, RSM fine-tuned the ultrasound-assisted extraction technique to obtain high-quality cinnamon extracts rich in phenolic compounds, underscoring the effectiveness and efficiency of this sophisticated extraction method. The application of Response Surface Methodology steered the exploration into extracting phenolic compounds from cinnamon, providing a structured approach to designing experiments and refining processes, thus furnishing invaluable information crucial for the advancement of functional food and health product development. [14]
The experiment consists of five main phases starts with design, manufacturing and post-manufacturing activities, mechanical testing, data collection and data analysis.
-
Defining the factors and responses of the experiment
Factors that affect the manufacturing process and final product along with their types and levels as shown on Table 1 after realizing the machines capabilities.
Table 1: factors that have been obtained from the machines
capabilities.
No.
Factor
Type
Level
1
Inner Cell
Dimension
Numerical
0.25 1 mm
2
Wall
thickness
Numerical
2 4 mm
3
Inner Cells
Orientation Angle
Numerical
0° 45°
4
3D Printing
Orientation
Categorical
Vertical
Horizontal
Diagonal
5
Surface
Roughness
Categorical
No
Sand
blasting
Machinin
g
6
Heat
Treatment
Categorical
No
Annealing
Stress
Relief
Responses that will be obtained and recorded after performing a destructive test (tensile test) on the specimens of the experiment are as follow: Tensile Strength (MPa), Yield Strength (MPa), Young's Modulus (GPa), and Strain (%). While the only response to obtain prior to the destructive test is the weight (g).
The experimental design table is generated through design expert software based on the factors of the study, their types and levels as a response Surface design of experiment as shown table 2:
Table 2: Experimental design table
Runs
FACTORS
Factor
1
Factor
2
Factor 3
Factor 4
Factor 5
Factor
6
A:
Inner Cell Dimen sion
B:
Wall thickn ess
C: Inner Cells Orientati on Angle
D:3D
Printing Orientation
E:
Surface Roughne ss
F:
Heat Treat ment
1
1.00
2.00
45.0
Horizontal
Machini
ng
Stress
Relief
2
0.25
2.00
45.0
Diagonal
No
Anne
aling
3
0.58
2.00
45.0
Horizontal
No
Anne
ling
4
1.00
2.00
45.0
Vertical
No
Anne
aling
5
0.58
2.00
45.0
Vertical
No
Stress
Relief
6
1.00
4.00
44.0
Diagonal
Machini
ng
Anne
aling
7
0.25
4.00
44.0
Vertical
No
No
8
1.00
4.00
44.0
Horizontal
Machini
ng
No
9
0.25
4.00
44.0
Vertical
Sand
blasting
Anne
aling
1
0
1.00
4.00
44.0
Vertical
Sand
blasting
No
1
1
1.00
2.99
12.3
Diagonal
Machini
ng
Stress
Relief
1
2
0.40
2.99
12.3
Vertical
No
Anne
aling
1
3
1.00
2.99
12.3
Diagonal
Sand
blasting
No
1
4
0.85
2.99
12.3
Diagonal
Sand
blasting
Anne
aling
1
5
0.25
4.00
1.7
Vertical
No
Stress
Relief
1
6
0.25
4.00
1.7
Diagonal
Machini
ng
No
1
7
0.25
4.00
1.7
Diagonal
No
Stress
Relief
1
0.40
4.00
1.7
Vertical
Sand
Stress
0
ng
aling
4
1
1.00
2.00
0.0
Vertical
Sand
blasting
Anne
aling
4
2
1.00
2.00
29.8
Horizontal
No
No
4
3
1.00
2.00
29.8
Vertical
Sand
blasting
Stress
Relief
4
4
1.00
2.00
29.8
Vertical
Machini
ng
No
4
5
0.58
2.00
29.8
Diagonal
Machini
ng
Anne
aling
4
6
0.25
2.00
29.8
Horizontal
No
No
-
Designing, manufacturing and post manufacturing activities The CAD model of the specimens was created using the SolidWorks program. The CAD file was uploaded on a fabricate web browser of Desktop Metal to set the print parameters.
This section is crucial as it evolves the study from the theoretical design phase to the practical implementation phase. It involves the actual creation of the stainless-steel specimens and the subsequent post-manufacturing activities that are integral to the process.
The manufacturing process is carried out using a specialized stainless-steel additive manufacturing machine. This machine uses a high-power laser to melt and fuse fine metallic powders into a three-dimensional structure. The machine builds the specimens layer by layer. This process ensures a high level of precision and repeatability, which is crucial for the validity of the experimental results.
After the specimens are manufactured, they undergo a surface finishing process. This process is important because the surface quality of the specimens can significantly influence their mechanical properties. Surface finishing can involve various techniques such as sanding, polishing, or blasting. Each of these techniques can alter the surface roughness of the specimens, which can affect properties such as friction, wear resistance, and fatigue strength. The choice of surface finishing technique depends on the specific requirements of the study.
The final step in the post-manufacturing process is heat treatment. This process involves heating the specimens to a specific temperature and then cooling them at a controlled rate. Heat treatment can alter the microstructure of the stainless steel, which can significantly affect its mechanical properties. For example, annealing can increase ductility and reduce hardness, while stress relief can reduce residual stresses without significantly altering the other mechanical properties. The specific heat treatment process used depends on the desired properties of the specimens [9].
8
blasting
Relief
1
9
0.87
3.95
0.0
Horizontal
No
Stress
Relief
2
0
0.25
3.95
0.0
Horizontal
No
No
2
1
1.00
3.95
0.0
Vertical
Machini
ng
Anne
aling
2
2
1.00
3.95
0.0
Horizontal
No
Anne
aling
2
3
1.00
3.95
0.0
Diagonal
No
No
2
4
0.25
2.94
45.0
Horizontal
Machini
ng
Anne
aling
2
5
0.25
2.94
45.0
Diagonal
Sand
blasting
Stress
Relief
2
6
0.87
2.94
45.0
Diagonal
No
No
2
7
0.25
2.94
45.0
Vertical
Machini
ng
No
2
8
1.00
2.18
9.2
Horizontal
Machini
ng
Anne
aling
2
9
0.78
2.18
9.2
Horizontal
Machini
ng
No
3
0
1.00
2.18
9.2
Diagonal
No
Stress
Relief
3
1
0.25
2.18
9.2
Horizontal
Sand
blasting
Stress
Relief
3
2
1.00
3.60
32.6
Horizontal
No
Stress
Relief
3
3
1.00
3.60
32.6
Horizontal
Sand
blasting
Stress
Relief
3
4
0.25
3.60
32.6
Horizontal
Machini
ng
Stress
Relief
3
5
0.25
3.60
32.6
Horizontal
Sand
blasting
No
3
6
1.00
3.60
32.6
Vertical
Machini
ng
Stress
Relief
3
7
0.25
2.00
0.0
Vertical
Machini
ng
Anne
aling
3
8
0.25
2.00
0.0
Vertical
Sand
blasting
No
3
9
0.40
2.00
0.0
Vertical
Machini
ng
Stress
Relief
4
1.00
2.00
0.0
Diagonal
Machini
Anne
-
Mechanical testing and data collection
Performing tensile tests on all specimens after recording their weight. The mechanical tensile testing phase is a critical part of this study as it provides empirical data on the mechanical properties of the stainless-steel specimens. This phase involves subjecting each specimen to a tensile test after its weight has been accurately recorded. A tensile test, also known as a tension test, is a fundamental mechanical test where a sample is subjected to a controlled tension until failure. The purpose of this test is to measure the resistance of a material to a force that is trying to pull it apart. It provides fundamental information about the material, including its yield strength, ultimate strength, and ductility.
Recorded Results of each response as an average of 3 specimens for each experimental run are shown in table 3 below:
Table 3 Data collection for each experimental run
R
un s
Responses
Response
1
Response
2
Response
3
Response
4
Response
5
Tensile Strength
(MPa)
Yield Strength
(MPa)
Young's Modulus
(GPa)
Strain (%)
Weight (g)
1
675
519
176
29.4
160.7
2
634
389
205
31.1
180.1
3
600
360
181
28.7
172.1
4
548
337
170
35.9
138.0
5
598
437
172
35.4
157.3
6
623
391
191
42.9
177.5
7
635
461
193
33.1
183.1
8
686
549
187
31.1
177.2
9
589
382
201
45.3
182.9
10
634
474
187
39.6
168.1
11
682
552
197
27.4
161.1
12
561
386
192
18.2
178.1
13
671
526
200
29.6
157.1
14
596
387
199
29.1
163.0
15
627
477
197
29.6
182.7
16
698
565
198
30.8
189.2
17
691
536
206
33.1
183.8
18
655
500
200
30.4
180.5
19
707
561
187
31
177.3
20
708
551
213
30.6
183.1
21
617
396
207
40.9
177.4
22
634
404
214
41.2
179.0
23
664
517
194
37.8
168.4
24
649
401
216
32
182.4
25
686
532
204
25.7
182.5
26
614
468
174
36.4
155.2
27
662
510
196
22.5
186.0
28
601
424
213
10
168.9
29
685
563
196
7.4
167.3
30
667
527
193
21.7
138.5
31
696
547
192
23.7
181.6
32
681
535
187
31.8
175.8
33
683
541
192
31.3
175.6
34
705
559
191
28.9
184.2
35
688
551
179
30.5
183.0
36
651
508
196
31.7
169.4
37
645
420
216
26.4
185.7
38
688
505
199
21.6
179.6
39
746
594
232
11.3
176.9
40
649
427
217
18.7
142.6
41
589
379
209
23.2
139.2
42
630
464
168
25.4
159.4
43
590
453
168
25.3
134.5
44
609
468
175
24.8
142.4
45
558
376
191
12.6
168.5
46
668
520
176
23.9
180.7
-
Data Analysis:
-
General Statistics
Table 4 illustrates the type of experimental study of I-optimal response surface design with a quadratic model, comprising 46 runs. Its a split-plot subtype, indicating that different factors are applied to different parts of the experimental units. Blocks are unused in this design, suggesting that there are no distinct groups within the experimental structure. This design aims to efficiently explore the response surface while minimizing the average prediction variance of the estimated regression coefficients:
Table 4 type of experimental study
Study Type
Response Surface
Subtype
Split-plot
Design Type
I-optimal
Runs
46
Design Model
Quadratic
Blocks
No Blocks
Table 5 illustrates the general statistics of each factor separately. The range between min and max is essential in determining the targeted values of each variable in the upcoming optimization process for both the factors and responses in tables 5 and 6.
Table 5: Factors or independent variables of the experiment
Factor
Name
Type
Min.
Max.
Mean
Std.
Dev.
A
Inner Cell
Dimension
Numeric
0.2500
1.0000
0.6591
0.3452
B
Wall
thickness
Numeric
2.00
4.00
2.96
0.8556
C
Inner Cells
Orientation Angle
Numeric
0.0000
45.00
22.40
18.60
D
3D
Printing Orientation
Categoric
Vertica l
Diagon al
Levels:
3
E
Surface
Roughness
Categoric
Machi
ning
No
Levels:
3
F
Heat
Treatment
Categoric
Stress
Relief
No
Levels:
3
Table 6 illustrates the general statistics of each response separately.
Table 6: Responses or dependent variables of the experiment
Res
Name
Min.
Max.
Mean
Std.
Dev.
Ratio
Transf
orm
R1
Tensile strengt
h
547.60
4
746.1
92
647.2
2
45.14
1.36
None
R2
Yield
strengt h
337.29
5
593.9
61
476.7
1
69.80
1.76
None
R3
Youngs modulu
s
167.86
4
231.5
6
194.5
5
14.49
1.38
None
R4
Strain
7.4
45.3
28.46
8.29
6.12
None
R5
Weight
134.53
3
189.1
67
170.3
8
14.95
1.41
None
Since a ratio of max to min for a response of greater than 10 usually indicates that data transformation is required and as shown all ratios of responses are below 10 which indicate that no data transformation is required.
Figure 1: correlation matrix
Figure 1 shows the correlation matrix, the categorical factors are not represented by a value of correlation, meanwhile the numerical factors are represented.
Some observation after realizing the values presented in the matrix:
-
Inner cell dimension has a weak negative correlation with yield strength and Young's modulus. This means that as the inner cell dimension increases, the yield strength and Young's modulus tend to decrease slightly.
-
Wall thickness has a moderate positive correlation with tensile strength and yield strength. This means that as the wall thickness increases, the tensile strength and yield strength also tend to increase.
-
Inner cell orientation angle has a weak negative correlation with tensile strength and Young's modulus. This means that as the Inner cell orientation angle changes, the tensile strength, yield strength and Young's modulus also tend to change slightly in the opposite direction.
-
-
-
Analysis of Variance (ANOVA)
-
Results of ANOVA are illustrated in Table 7 which reflect the statically significant factors that affect each response depending on P-values less than 0.05 and their F-Values are relatively high.
Table 7: ANOVA Table for all responses
Res |
Source |
Term df |
Error df |
F-value |
p- value |
Tensile Strength |
Subplot |
8 |
32.43 |
11.03 |
< 0.0001 |
a-Inner Cell Dimension |
1 |
32.32 |
11.15 |
0.0021 |
|
C-Inner Cells Orientation Angle |
1 |
19.85 |
9.82 |
0.0053 |
|
D-3D Printing Orientation |
2 |
34.77 |
9.08 |
0.0007 |
|
E-Surface Roughness |
2 |
35.41 |
5.62 |
0.0076 |
|
F-Heat Treatment |
2 |
36.32 |
27.23 |
< 0.0001 |
|
Yield Strength |
Subplot |
17 |
19.30 |
40.85 |
< 0.0001 |
a-Inner Cell Dimension |
1 |
25.41 |
15.36 |
0.0006 |
|
C-Inner Cells Orientation Angle |
1 |
17.26 |
31.68 |
< 0.0001 |
|
D-3D Printing Orientation |
2 |
24.68 |
27.08 |
< 0.0001 |
|
E-Surface Roughness |
2 |
26.35 |
15.02 |
< 0.0001 |
|
F-Heat Treatment |
2 |
27.38 |
262.24 |
< 0.0001 |
|
BC |
1 |
24.89 |
8.10 |
0.0087 |
|
CE |
2 |
27.01 |
3.42 |
0.0476 |
|
EF |
4 |
26.10 |
2.92 |
0.0404 |
|
Young`s modulus |
Subplot |
11 |
34.00 |
10.62 |
< 0.0001 |
a-Inner Cell Dimension |
1 |
34.00 |
7.79 |
0.0086 |
|
C-Inner Cells Orientation Angle |
1 |
34.00 |
44.41 |
< 0.0001 |
|
E-Surface Roughness |
2 |
34.00 |
3.36 |
0.0466 |
|
F-Heat Treatment |
2 |
34.00 |
4.74 |
0.0153 |
BC |
1 |
34.00 |
8.60 |
0.0060 |
|
C² |
1 |
34.00 |
11.94 |
0.0015 |
|
Strain |
Subplot |
16 |
29.00 |
10.07 |
< 0.0001 |
a-Inner Cell Dimension |
1 |
29.00 |
6.85 |
0.0139 |
|
B-Wall thickness |
1 |
29.00 |
48.16 |
< 0.0001 |
|
C-Inner Cells Orientation Angle |
1 |
29.00 |
28.60 |
< 0.0001 |
|
E-Surface Roughness |
2 |
29.00 |
4.99 |
0.0137 |
|
aD |
2 |
29.00 |
4.34 |
0.0224 |
|
BC |
1 |
29.00 |
9.73 |
0.0041 |
|
a² |
1 |
29.00 |
10.07 |
0.0036 |
|
C² |
1 |
29.00 |
4.29 |
0.0474 |
|
Weight |
Subplot |
21 |
17.41 |
165.19 |
< 0.0001 |
a-Inner Cell Dimension |
1 |
19.72 |
1707.07 |
< 0.0001 |
|
B-Wall thickness |
1 |
18.80 |
200.09 |
< 0.0001 |
|
D-3D Printing Orientation |
2 |
23.44 |
32.81 |
< 0.0001 |
|
E-Surface Roughness |
2 |
20.87 |
40.69 |
< 0.0001 |
|
F-Heat Treatment |
2 |
20.80 |
8.59 |
0.0019 |
|
aB |
1 |
19.20 |
232.88 |
< 0.0001 |
|
aD |
2 |
19.81 |
99.27 |
< 0.0001 |
|
BD |
2 |
22.19 |
15.44 |
< 0.0001 |
|
DE |
4 |
22.17 |
6.99 |
0.0009 |
|
EF |
4 |
21.22 |
6.21 |
0.0018 |
According to ANOVA wall thickness has no significant impact on the tensile strength, yield strength, and Youngs modulus. While Inner Cells Orientation Angle has no significant impact on weight only. For 3D printing orientation, it has no significant impact on Youngs modulus, and strain. For heat treatment, it has no significant impact on only the strain.
All non-significant factors had been excluded from the model to enhance the software analysis and to obtain the ANOVA table.
Table 7 judges that the following factors have no significant impact on specific responses based on the p-values since they were excluded from the model during the analysis:
-
Wall thickness has no significant impact on tensile strength and yield strength.
-
3D Printing Orientation and wall thickness have no significant impact on Young`s modulus.
-
3D Printing Orientation and heat treatment have no significant impact on strain.
-
Inner Cells Orientation Angle has no significant impact on weight.
-
RESULTS AND FINDINGS
The experiment was conducted to examine the mechanical properties and the effect of the independent variables on the dependent variables of the experiment which are defined as factors and responses.
-
Prediction of responses
The following surface plots show the impact of two factors on a single response. The representation of factors on the surface plot includes only the continuous numerical factors of the experiment which are:
-
Inner cell dimension
-
Wall thickness
-
Inner cell orientation angle
-
-
-
While the other categorical factors are not represented because they are discrete variables. However, on each plot only two independent variables are represented and the other four independent variables took an assigned value by the software as presented in table 8:
Table 8: Assigned values to independent variable by the
software
These assigned values can be changed to further examine the model and have no statistical necessity to be valued as presented in the previous table. The aim of setting the values as presented is to analyze the impact of the factors on responses under similar conditions.
-
Tensile Strength Prediction
As shown in Figure 2, the tensile strength approaches higher values as the inner cell dimension approaches 0.25 and the wall thickness has no impact on tensile strength as it is a non- significant factor as discussed earlier in ANOVA.
Figure 2: Surface plot of tensile strength under the effect of wall thickness and inner cell dimension
-
Yield Strength Prediction
As shown in Figure 3, yield strength approaches higher values as the inner cell dimension approaches 0.25 and the wall thickness has no impact on yield strength as it is a non- significant factor as discussed earlier in ANOVA.
No.
Independent Variable
Assigned Value
1
Inner Cell Dimension
0.625 mm
2
Wall thickness
3 mm
3
Inner Cells Orientation Angle
22.5
4
3D Printing Orientation
Vertical
5
Surface Roughness
Machining Surface Roughness
6
Heat Treatment
Stress Relief
Yield strength
finish
600
550
500
450
400
350
300
50
40
finish
30
Strain
20
10
0
2
2.5
0.25
0.4
4
3.5
3
1
0.85
0.7
3
B: Wall thickness
3.5
0.85
0.7
0.55
a: Inner Cell Dimension/p>
B: Wall thickness
2.5
2 0.25
0.4
0.55
a: Inner Cell Dimension
4 1 Figure 5: Surface plot of strain under the effect of wall
Figure 3: Surface plot of yield strength under the effect of wall thickness and inner cell dimension
-
Young's Modulus Prediction
As shown in Figure 4, Young's modul3uDs aSpuprrfoaaccehes the higher
6 values as the wall thickness is in between (2.5-3) and the inner cell dimension decreases.
thickness and inner cell dimension
-
Weight Prediction
As shown in Figure 6, weight approaches the minimum value as the wall thickness approaches 2 and the inner cell dimension
sion
240
Factor Coding: Actual
Design Points
134.533 189.167
X1 = a: Inner Cell Dimension
approaches 1.
3D Surface
n Angle = 22.5
X2 = B: Wall thickness
on = Vertical 220
Youngs modulus
Machining Surface finish ss Relief
200
180
Actual Factors 2
C: Inner Cells Orientation Angle = 22.5 1
D: 3D Printing Orientation = Vertical
E: Surface Roughness = Machining Surface finish 1
F: Heat Treatment = Stress Relief
Weight
160
0.25
0.4
0.55
0.7
3
2.5
4
3.5
a: Inner Cell Dimension
0.85
1 2
B: Wall thickness
Figure 4: Surface plot of Youngs Modulus under the effect
of wall thickness and inner cell dimension
4 1
00
90
80
170
160
150
140
130
2
0.25
2.5 0.4
3
0.55
0.7
B: Wall thickness
3.5
0.85 a: Inner Cell Dimension
-
-
Strain Prediction
As shown in Figure 5, strain approaches higher values as the wall thickness increases and the inner cell dimension increases.
Figure 6: Surface plot of weight under the effect of wall thickness and inner cell dimension
-
Optimization
The aim of the experiment is to enhance and improve the status of the model, one of the improvement methods is to optimize the outputs. In the case of this study, the optimization of the outputs clears up the levels of the manufacturing and post- manufacturing activities.
Taking into consideration that all inputs are in range and no specific values are required. The aimed outputs of the optimization are illustrated in table 9:
Table 9: the aim of each response in optimization
Response
Aimed Value
Tensile strength
Maximum
Yield strength
Maximum
Youngs modulus
In range
Strain
In range
Weight
Minimum
The results of the optimization which should be the inputs of the manufacturing process to reach the desired output values are illustrated in table 10:
Table 10: the optimization results for each factor
Factor
Aimed Value
Optimized Value
Inner Cell Dimension
In range
1
Wall thickness
In range
2
Inner Cells Orientation Angle
In range
0
3D Printing Orientation
In range
Diagonal
Surface Roughness
In range
Machining Surface
Fininshing
Heat Treatment
In range
Stress Relief
The outputs of the manufacturing process depending on the statistical analysis should be as illustrated in Table 11:
Variable Type
Name
Aimed Value
Optimized Value
Response
Tensile strength
Maximum
697.9
Response
Yield strength
Maximum
582.7
Response
Youngs modulus
In range
214.4
Response
Strain
Maximum
19.2
Response
Weight
Minimum
143.9
Table 11: the predicted values of the optimized manufacturing process
-
-
CONCLUSIONS
This study has successfully demonstrated the potential of using a structured cell approach in designing and manufacturing stainless-steel specimens to reduce their cost and weight.
This study has made significant strides towards achieving its goal of reducing the cost and weight of manufactured objects by partially evacuating the inner structure via an inner net of structured cells. The findings of this research have broad implications for the field of materials science and can inform future work on the design and manufacturing of lightweight, cost-effective stainless-steel components.
The results of this study provide a solid foundation for further experimental investigations. Future studies could explore different design parameters, manufacturing conditions, or material types to expand the understanding of the relationship between the inner structure of manufactured objects and their mechanical properties.
This study focused on the immediate mechanical properties of the specimens after manufacturing. Future research could investigate the long-term performance of the specimens, such as their fatigue strength or corrosion resistance.
The ultimate goal of this research is to reduce the cost and weight of manufactured objects. Future studies could focus on applying the findings of this research to real-world applications, such as the design and manufacturing of lightweight structures for the automotive or aerospace industries.
REFERENCES
-
Graham Gedge, "Structural uses of stainless steel – buildings and civil engineering", Journal of Constructional Steel Research 64 (2008) 1194-
1198.
-
Tepylo, N.; Huang, X.; Patnaik, P.C. Laser-Based Additive Manufacturing Technologies for Aerospace Applications. Adv. Eng.Mater. 2019, 21, 20192020. [CrossRef]
-
Ziókowski, M.; Dyl, T. Possible applications of additive manufacturing technologies in shipbuilding: A review. Machines 2020, 8, 84. [CrossRef]
-
Fox, J.C.; Moylan, S.P.; Lane, B.M. Effect of process parameters on the surface roughness of overhanging structures in laser powder bed fusion additive manufacturing. Procedia CIRP 2016, 45, 131134. [CrossRef]
-
Sander, G.; Tan, J.; Balan, P.; Gharbi, O.; Feenstra, D.R.; Singer, L.; Thomas, S.; Kelly, R.G.; Scully, J.R.; Birbilis, N. Corrosion of additively manufactured alloys: A review. Corrosion 2018, 74, 1318
1350. [CrossRef]
-
Kruth, J.P.; Badrossamay, M.; Yasa, E.; Deckers, J.; Thijs, L.; Van Humbeeck, J. Part and material properties in selective laser melting of metals. In Proceedings of the 16th International Symposium on Electromachining (ISEM XVI), Shanghai, China, 1923 April 2010; pp. 314.
-
Vanmeensel, K.; Lietaert, K.; Vrancken, B.; Dadbakhsh, S.; Li, X.; Kruth, J.P.; Krakhmalev, P.; Yadroitsev, I.; Van Humbeeck, J. Additively manufactured metals for medical applications. In Additive Manufacturing: Materials, Processes, Quantifications and Applications; Elsevier: Amsterdam, The Netherlads, 2018; pp. 261309. ISBN 9780128121559. [CrossRef]
-
Branner, G.; Zaeh, M.F.; Groth, C. Coupled-field simulation in additive layer manufacturing. In Proceedings of the 3rd International Conference on Polymers and Moulds Innovations, Ghent, Belgium, 1719 September 2008; pp. 184193.
-
Wang, D.; Wu, S.; Yang, Y.; Dou, W.; Deng, S.; Wang, Z.; Li, S. The effect of a scanning strategy on the residual stress of 316L steel parts fabricated by selective laser melting (SLM). Materials 2018, 11, 1821. [CrossRef]
-
Response surface methodology (RSM) as a tool for optimization in analytical chemistry. Talanta, 76(5), 965-977. : Khuri, A. I., & Mukhopadhyay, S. (2010).
-
Response surface methodology. Wiley Interdisciplinary Reviews: Computational Statistics, 2(2), 128-149. : Anderson, M. J., & Whitcomb,
P. J. (2016).
-
Myers, R. H., Montgomery, D. C., & Anderson-Cook, C. M. (2016). Response surface methodology: process and product optimization using designed experiments. John Wiley & Sons
-
Nida Sezin Çolak, Engin ahin, Enes Dertli, Mustafa Tahsin Yilmaz & Osman Taylan (2019) Response surface methodology as optimization strategy for asymmetric bioreduction of acetophenone using whole cell of Lactobacillus senmaizukei, Preparative Biochemistry and Biotechnology.
-
Cebi, N., Sagdic, O., Basahel, A. M., Balubaid, M. A., Taylan, O., Yaman, M., & Yilmaz, M. T. (2018). Modeling and optimization of ultrasoundassisted cinnamon extraction process using fuzzy and
response surface models. In Journal of Food Process Engineering (Vol. 42, Issue 2). Wiley.