
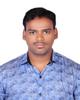
- Open Access
- [post-views]
- Authors : V.Yogeswara Rao, V.V.S. Kesava Rao, Ravindra Andukuri
- Paper ID : IJERTV13IS070053
- Volume & Issue : Volume 13, Issue 07 (July 2024)
- Published (First Online): 22-07-2024
- ISSN (Online) : 2278-0181
- Publisher Name : IJERT
- License:
This work is licensed under a Creative Commons Attribution 4.0 International License
Enhancing Reliability of Cryogenic Globe Valves Using Fuzzy AHP-TOPSIS Integrated FMEA Methodology
V.Yogeswara Rao, V.V.S. Kesava Rao,
Department of Mechanical Engineering, Andhra University College of Engineering, Visakhapatnam, Andhra Pradesh, INDIA
Ravindra Andukuri
Department of Mechanical Engineering, GITAM Deemed to be University, Visakhapatnam, Andhra Pradesh, INDIA
Abstract – This study combines the Fuzzy Analytical Hierarchy Process (FAHP) and Technique for Order Preference by Similarity to Ideal Solution (TOPSIS) techniques with the Failure Mode and Effects Analysis (FMEA) framework to prioritize failure modes of cryogenic globe valves used in oxygen plants. Conventional Failure Mode and Effects Analysis (CFMEA), which relies on Risk Priority Numbers (RPNs), has limitations because of inherent uncertainties and subjectivities. This study aims to overcome these constraints by integrating fuzzy logic, which allows for a more precise and dependable ranking of failure modes. Ten main failure modes are found in the cryogenic globe valves case study and graded using their proximity coefficients produced from the FAHP-TOPSIS method. The results highlight that "Disc wear and scratch" is the
most important failure mode; "Damaged and cracked valve body" and "Valve stem assembly failure" follow second importance failure categories. The research provides valuable insights and ideas for improving valve dependability by examining the importance of predictive maintenance techniques, premium materials, and regular inspections. In order to enhance operational efficiency and overall safety, it is recommended that a comprehensive framework be established for prioritizing failure categories that is pertinent to a wide range of industries.
Index Terms: AHP, Failure Mode, Reliability, Risk Priority Number, TOPSIS
-
INTRODUCTION
Failure Mode and Effects Analysis (FMEA) is a widely recognized systematic technique to identify and evaluate potential failures in products, processes, designs, and services. It aims to prioritize these failures based on risk levels, enabling organizations to implement corrective actions to mitigate them. The traditional FMEA relies heavily on the calculation of Risk Priority Numbers (RPNs), which are derived from three critical risk factors: occurrence (O), severity (S), and detection (D). Each factor is assigned a numerical value, and their product provides the RPN, which ranks the potential failures (Stamatis, 2003). However, this approach has notable limitations due to its reliance on precise numerical values, which may not always be feasible in practical scenarios due to inherent uncertainties and subjectivities (Bowles and Peláez, 1995).
In recent years, the integration of fuzzy logic with FMEA has garnered significant attention to address these uncertainties. Fuzzy logic incorporates expert judgment and linguistic terms, providing a more flexible and realistic approach to evaluating risk factors (Zadeh, 1965). This methodology facilitates the handling of vagueness and imprecision inherent in human assessments, thus enhancing the accuracy and reliability of FMEA. Specifically, the application of fuzzy logic techniques such as the Fuzzy Analytical Hierarchy Process (AHP) and the Fuzzy Technique for Order Performance by Similarity to the Ideal Solution (TOPSIS) has shown promise in improving the prioritization process in FMEA (Chan et al., 2008; Tseng et al., 2019).
This research focuses on the failure analysis of valves in industrial settings, a critical component in various manufacturing and processing industries. Valves are prone to multiple failure modes, leading to significant operational disruptions and safety hazards. By applying fuzzy logic- based FMEA, this study aims to prioritize valve failure modes more effectively, enhancing maintenance strategies and reducing the likelihood of failures. The motivation for this research extends to the broader application of the proposed methodology in other industries, such as construction, where safety and reliability are paramount. By demonstrating the effectiveness of fuzzy FMEA in a specific case study, this research provides a foundation for its application in other contexts, contributing to the overall improvement of risk management practices across different sectors.
The inherent subjectivity and uncertainty in traditional FMEA necessitate a more robust and flexible approach. Fuzzy logic offers a promising solution by allowing risk factors to be expressed as fuzzy variables and evaluated using fuzzy linguistic terms and ratings (Kahraman, 2008; Liu et al., 2019). This study integrates fuzzy AHP and fuzzy TOPSIS into the FMEA framework to enhance the evaluation and ranking of valve failure modes. The objective is to establish a more accurate and reliable methodology for FMEA, with potential applications extending beyond the industrial setting of valve failures to other sectors, such as construction, where safety and reliability are of paramount importance (Wang et al., 2020; Zhou et al., 2021).
This study aims to establish a more effective and reliable methodology for FMEA by addressing these limitations. The
research focuses on valve failures in industrial settings,
FPIS = (max v+), FNIS = (min v)
aiming to improve the accuracy and reliability of failure mode
i ij
i ij
prioritization by integrating fuzzy logic techniques. The broader goal is to provide a methodology that can be applied in various industries to enhance risk management practices and improve safety and reliability.
-
METHODOLOGY
-
FAHP and TOPSIS Methodology
In order to rank the possible causes of failure in the cryogenic globe valve of an oxygen plant, the suggested approach merges the Fuzzy Analytical Hierarchy Process (FAHP) with TOPSIS. By using the FAHP approach, experts' subjective evaluations can be translated into numerical metrics. TOPSIS
where Vij represents the weighted normalized fuzzy value for the i th failure mode and the j th criterion.
-
Distance Calculation:
The distance of each failure mode to the FPIS and FNIS is calculated using the Euclidean distance formula.
-
Closeness Coefficient (CC):
The closeness coefficient for each failure mode is calculated as:
Di
is then applied to rank the failure modes based on their
CCi =
D
i+
+ Di
closeness to an ideal solution.
-
-
FAHP Methodology
-
Establishment of Risk Assessment Team:
A team of experts is formed, and their experience levels are normalized to allocate contribution factors. The contribution
factor for the th expert is calculated as follows:
= 1
=1
where is the number of experts.
-
Linguistic and Fuzzy Scales:
Linguistic scales and corresponding triangular fuzzy scales are used to capture the relative importance of risk factors (severity, occurrence, and detection) through pairwise comparisons. These scales are essential for translating subjective judgments into quantitative measures.
-
Aggregation of Expert Opinions:
Expert opinions on the interrelationships between Severity (S), Occurrence (O), and Detection (D) are aggregated to obtain combined fuzzy values. This involves calculating the fuzzy synthetic extent for each pairwse comparison.
-
Normalization of Fuzzy Values:
The aggregated fuzzy values are normalized to derive weights for each risk factor. These weights are then used in the TOPSIS method to prioritize failure modes.
-
-
TOPSIS Methodology
-
Weighted Normalized Fuzzy Decision Matrix:
The normalized fuzzy values are multiplied by their respective weights to create a weighted normalized fuzzy decision matrix.
-
Positive and Negative Ideal Solutions:
The positive ideal solution (FPIS) and negative ideal solution (FNIS) are defined as follows:
where Di+ and Di are the distances to the FPIS and FNIS, respectively.
-
Ranking of Failure Modes:
Failure modes are ranked based on their closeness coefficient, with higher values indicating higher priority.
-
-
CASE STUDY: CRYOGENIC GLOBE VALVE
-
Overview of case study
Fluids like liquid oxygen and liquefied natural gas (LNG) are regulated by cryogenic globe valves, which are essential parts of oxygen plants. These valves work at extremely low temperatures, below -150°C. If the facility is to remain safe and efficient, the dependability of these valves is paramount. There are a number of ways in which cryogenic globe valves might fail under the extreme operating temperatures, reducing their performance and perhaps causing the system to fail. Figure 1 depicts the low temperature globe valve components and their accompanying trim.
Fig. 1. Cryogenic Globe valve trim and parts [23]
Cryogenic globe valve failure mechanisms are identified and prioritized in this case study using the combined FAHP and TOPSIS techniques. Our goal is to offer a systematic way to improve the valve's reliability by tackling the most important failure modes.
-
Identification of Failure Modes
A group of specialists with extensive knowledge in cryogenic systems undertook a thorough process of identifying failure modes. The team has identified ten main failure mechanisms that could impact the cryogenic globe valve's functioning. We classified these failure modes according to how they affected the valve's sealing and transmission mechanisms. Table 1 displays the failure modes that have been found.
Table 1: Failure Modes and Descriptions
Criteria
Failure Mode
Description
C1
Packing Wear and Loose
Degradation or loosening of the
packing material, leading to leaks and reduced sealing effectiveness.
C2
Steam
Deformation and Fracture
Structural damage to the valve stem
caused by thermal stresses, potentially leading to valve failure.
C3
Loose Disc Fixing Part
Loosening of the disc components, which affects the valve's ability to seal properly, causing leaks.
C4
Loose Body Bolts
Loosening of the bolts that secure the valve body, leading to structural integrity issues and potential leaks.
C5
Loose Lock Nut
Loosening of the lock nut, compromising the stability of the valve
assembly and leading to misalignment.
C6
Valve Stem Assembly Failure
Failure of the valve stem assembly, resulting in operational inefficiencies
and potential valve malfunction.
C7
Main Gasket Failure
Degradation or failure of the main
gasket, resulting in leaks and compromised sealing capabilities.
C8
Damaged and
Cracked Valve Body
Cracks or other damage to the valve body, compromising its integrity and
potentially leading to catastrophic failure.
C9
Steam Wear
Wear and tear on the valve stem due to friction and operational stresses,
reducing the valve's lifespan.
C10
Disc Wear and Scratch
Surface damage to the valve disc,
affecting its sealing capability and leading to leaks.
-
Calculation of RPN Values
For each detected failure mode, the Risk Priority Number (RPN) was calculated using the standard Failure Mode and Effects Analysis (FMEA) approach. Multiplying the severity (S), occurrence (O), and difficulty of detection (D) scores for each failure mode yields the RPN. Data from comparable systems in the past and expert opinion were the main factors in determining these grades.
Table 2: Categorization of failure scenarios according to their severity (S), frequency (O), and detection difficulties
(D).
Failure
Mode
Severity
(S)
Occurrence
(O)
Difficulty of
Detection (D)
C1
5
4
6
C2
7
5
6
C3
6
4
5
C4
8
6
2
C5
4
6
2
C6
6
4
6
C7
6
4
5
C8
9
1
2
C9
6
3
6
C10
5
8
5
Fig. 1. RPN values for Failure modes
According to the RPN values shown in figure 1, "Steam deformation and fracture (C2)" has the greatest priority failure mode at 210 RPN, followed by "Disc wear and scratch (C10)" at 200 RPN.
-
FAHP Analysis
The FAHP methodology was applied to further analyze the identified failure modes. This process involved the following steps:
-
Establishment of Risk Assessment Team
A team of four experts was formed, and their experience levels were normalized to allocate contribution factors as shown in Table 3.
Experts
Experience (Years)
Contribution Factor
E1
7
0.21875
E2
11
0.34375
E3
5
0.15625
E4
9
0.28125
Total
32
1
Table 3: Experts Experience and Contribution Factors
Table 3 shows the experience in years and the corresponding contribution factors for each expert in the risk assessment team. These factors are used to weight the expert opinions in the FAHP analysis.
-
Linguistic and Fuzzy Scales
Linguistic scales and corresponding triangular fuzzy scales were used to capture the relative importance of risk factors through pairwise comparisons. The scales are detailed in Table 4.
Table 4: Linguistic Scale and Corresponding Triangular Fuzzy Scale for FAHP
Linguistic Scale
Triangular Fuzzy Scale
Triangular Fuzzy Reciprocal Scale
Just Equal
(1, 1, 1)
(1, 1, 1)
Weakly Important
(2/3, 1, 3/2)
(2/3, 1, 3/2)
Strongly More Important
(3/2, 2, 5/2)
(2/5, 1/2, 2/3)
Very Strongly More Important
(5/2, 3, 7/2)
(2/7, 1/3, 2/5)
Absolutely More Important
(7/2, 4, 9/2)
(2/9, 1/4, 2/7)
This table 4 provides the linguistic scales and their corresponding triangular fuzzy scales, which are used to capture the relative importance of criteria (severity, occurrence, and detection) through pairwise comparisons in the FAHP analysis. The reciprocal scales are also provided for the inverse comparisons.
-
Aggregation of Expert Opinions
Expert opinions on the interrelationships between Severity
Table 6: Normalized Fuzzy Values of Severity (S), Occurrence (O), and Detection (D)
Criteria
Lower (L)
Middle (M)
Upper (U)
Severity (S)
0.203
0.310
0.471
Occurrence (O)
0.314
0.481
0.711
Detection (D)
0.151
0.209
0.315
These tables 5 and 6 represent the aggregated and normalized expert opinions on the interrelationships between Severity (S), Occurrence (O), and Detection (D), which are used to derive the weights for each criterion in the FAHP analysis.
-
-
TOPSIS Analysis
A weighted normalized fuzzy decision matrix was constructed using the weights derived from FAHP. After defining the positive ideal solution (FPIS) and the negative ideal solution (FNIS), the distances to these solutions were determined for each failure scenario.
-
Weighted Normalized Fuzzy Decision Matrix
As demonstrated in Table 7, a weighted normalized fuzzy decision matrix was generated by multiplying the normalized fuzzy values by their corresponding weights.
Table 7: Weighted Normalized Fuzzy Decision Matrix
Fail ure Mo
de
S_ L
S_ M
S_ U
O
_ L
O_ M
O_ U
D_L
D_ M
D
_ U
C1
0.0
96
0.
2
0.2
88
0
0.
3
0.6
7
0.00
275
0.0
07
0.
01
C2
0.0
96
0.
2
0.3
2
0.
2
0.
4
0.6
03
0.00
7
0.0
09
0.
01
C3
0.0
32
0.
1
0.2
24
0.
2
0.
5
0.6
7
0.00
6
0.0
09
0.
01
C4
0.0
32
0.
2
0.3
2
0.
07
0.
4
0.6
03
0.00
5
0.0
09
0.
01
C5
0.0
96
0.
2
0.3
2
0.
2
0.
4
0.6
03
0.00
8
0.0
09
0.
01
C6
0.0
96
0.
2
0.2
88
0.
2
0.
5
0.6
7
0.00
5
0.0
09
0.
01
C7
0.0
32
0.
2
0.3
2
0.
07
0.
3
0.6
03
0.00
5
0.0
07
0.
01
C8
0.0
9
0.
2
0.2
88
0.
2
0.
5
0.6
7
0.00
7
0.0
09
0.
01
C9
0
0.
1
0.3
2
0.
2
0.
4
0.6
03
0.00
6
0.0
09
0.
01
C10
0.0
96
0.
2
0.2
88
0.
34
0.
6
0.6
7
0.00
6
0.0
09
0.
01
(S), Occurrence (O), and Detection (D) were aggregated using fuzzy synthetic extent analysis. The aggregated values are shown in Table 5.
Table 5: Combined Expert Opinions on Severity (S),
Occurrence (O), and Detection (D) Interrelationships
Criteria
Severity
(S)
Occurrence
(O)
Detection
(D)
Severity (S)
(1, 1, 1)
(0.447,
0.581, 0.837)
(1.08, 1.58,
2.08)
Occurrence
(O)
(1.3, 1.8,
2.3)
(1, 1, 1)
(1.61, 2.11,
2.61)
Detection
(D)
(0.484,
0.64, 0.947)
(0.395,
0.497, 0.677)
(1, 1, 1)
(d) Normalization of Fuzzy Values
The aggregated fuzzy values were normalized to derive weights for each risk factor as shown in Table 6.
For each failure mode, these values are the weighted normalized fuzzy values for Severity (S), Occurrence (O), and Detection (D). The distances to the FPIS and FNIS and the closeness coefficients for rating the failure modes are determined using these values in the TOPSIS analysis.
-
Positive and Negative Ideal Solutions
A formula was utilized in order to determine the positive ideal solution (FPIS) and the negative ideal solution (FNIS).
-
Distance Calculation
After applying the Euclidean distance formula, we were able to determine the distance between each failure mode and the FPIS and FNIS. It is revealed in Table 8 that the distances are. Table 8: Distance to FPIS and FNIS for Each Failure Mode
Failure Mode
S
O
D
Distance
to FPIS (D+)
Distance
to FNIS (D-)
C1
0.15
0.4415
0.006
0.598
0.636
C2
0.137
0.3242
0.004
0.465
0.668
C3
0.207
0.2946
0.004
0.506
0.643
C4
0.182
0.3829
0.005
0.57
0.642
C5
0.141
0.3242
0.002
0.467
0.664
C6
0.15
0.2946
0.005
0.45
0.7
C7
0.175
0.4001
0.004
0.58
0.632
C8
0.146
0.2946
0.004
0.444
0.706
C9
0.215
0.3052
0.004
0.525
0.65
C10
0.155
0.1972
0.004
0.356
0.764
-
Closeness Coefficient (CC)
For every failure mode, the proximity coefficient was determined by dividing the distance to the FPIS (+) by the sum of the distances to the FNIS (), where + and are the corresponding distances.
Table 9: Closeness Coefficient and Ranking of Failure Modes
Criteria
Closeness Coefficient
Rank
C1
0.5154
10
C2
0.5897
4
C3
0.5596
6
C4
0.5298
8
C5
0.5869
5
C6
0.6086
3
C7
0.5214
9
C8
0.6137
2
C9
0.5535
7
C10
0.6822
1
Table 9 displays the proximity coefficients and rankings for each failure mechanism. The proximity coefficient measures the relative importance of each failure mode, with higher values suggesting a greater priority for taking corrective steps.
=
+
+
-
-
RESULTS AND DISCUSSIONS
-
Closeness Coefficient and Ranking
The proximity coefficients for each failure mode were computed using the FAHP and TOPSIS techniques. The coefficients indicate the relative importance of each failure mode, with larger values indicating a greater priority for corrective activities. The failure scenarios were evaluated and ordered according to their closeness coefficients, as presented in Table 9.
Fig 2. Radar Chart of Closeness Coefficients for Failure Modes
The radar map in figure 2 provides a clear and concise visualization of the relative importance of each failure mode. A higher proximity coefficient indicates that the failure mode is closer to the outer edge of the chart, which signifies a greater need for remedial action.
-
Analysis of failure modes based on priority
Figure 3 illustrates the hierarchy chart that classifies the failure scenarios of the cryogenic globe valve into three priority levels: high, medium, and low. This categorization is determined by the proximity coefficients of each failure scenario, which indicate their comparative influence on the performance and dependability of the valve. As the proximity coefficient increases, the priority for remedial action also increases.
Fig. 3 Hierarchy of Failure Modes for Cryogenic Globe Valves Based on Closeness Coefficients
-
Discussion on High-Priority Failure Modes
Several critical issues that influence the performance and reliability of the cryogenic globe valve are revealed by the analysis of high-priority failure modes. The valve's sealing is compromised, resulting in leakage and inefficiencies, as disc wear and scratch (C10) is the highest-ranked issue with a closeness coefficient of 0.6822. This is further exacerbated by rapid flow rates and extreme temperatures. Routine inspections, the application of protective coatings, and the use of wear-resistant materials are among the recommended solutions.
A substantial risk to system integrity is posed by the damaged and cracked valve body (C8), which has a closeness coefficient of 0.6137. This issue is highly severe and can result in catastrophic failure if not addressed, despite its rarity. The valve body is reinforced with high-strength materials, and preventive maintenance, non-destructive testing (NDT), and regular monitoring are part of the mitigation process.
With a closeness coefficient of 0.6086, valve stem assembly failure (C6) disrupts the transmission system, thereby impacting valve operation. Proper alignment during installation, the use of high-quality components, and consistent lubrication are all recommended actions.
Steam deformation and fracture (C2), which have a closeness coefficient of 0.5897, are the consequence of thermal stresses and mechanical loads, resulting in substantial operational disruptions and safety hazards. Thermal stress analyses, the utilization of high thermal resistance materials, and the implementation of temperature control mechanisms are all examples of mitigation strategies.
-
Discussion on Medium-Priority Failure Modes
The valve assembly can be destabilized by loose lock nuts, which have a closeness coefficient of 0.5869, which can lead to operational inefficiencies and potential misalignments. It is imperative to guarantee that all lock nuts are securely fastened during routine maintenance inspections and assembly in order to alleviate this issue. Furthermore, the implementation of vibration monitoring can assist in the detection of any loosening of lock nuts during operation, and the use of supplementary securing mechanisms or adhesives can further prevent loosening.
Similarly, the disc fixing element, which has a closeness coefficient of 0.5596, can impact the valve's sealing capability if it becomes loose, resulting in reduced performance and leaks. It is imperative to guarantee that all disc fixing components are securely fastened during installation and maintenance in order to resolve this issue. It is recommended that routine inspections be conducted to identify and rectify any loosening. The use of high-strength fasteners can mitigate the risk of loosening.
-
Discussion on Lower-Priority Failure Modes
Steam wear, although less critical than other failure modes, can still affect the valve's efficacy and longevity. It is advisable to employ materials with a high wear resistance for components that are exposed to steam, and to schedule regular maintenance to replace deteriorated components, as indicated by a closeness coefficient of 0.5535.
The valve's structural integrity may be compromised by loose body fasteners, which have a closeness coefficient of 0.5298, which may result in leaks and mechanical failures. In order to prevent this, it is recommended that torque monitoring instruments be implemented to guarantee that bolts are secured in accordance with the appropriate specifications. Additionally, routine maintenance inspections should incorporate bolt inspections.
The main gasket's failure, which has a closeness coefficient of 0.5214, can lead to breaches that compromise the valve's sealing capability and overall performance. It is advisable to replace gaskets as necessary and conduct regular inspections to identify signs of deterioration and use high-quality gaskets that are resistant to cryogenic temperatures and pressures.
Packing wear and loosening, which have a closeness coefficient of 0.5154, can result in operational inefficiencies and leakage. It is recommended that packing materials that are resistant to wear and degradation under cryogenic conditions be employed, and that packaging inspection and replacement be incorporated into the regular maintenance schedule.
-
-
General Recommendations and Conclusion
The integration of FAHP and TOPSIS methodologies has facilitated the prioritization of the failure modes of cryogenic globe valves. This method is structured and quantitative. The reliability and safety of the valve and th entire system can be substantially improved by addressing the highest priority failure modes. Several general recommendations are recommended in accordance with the analysis:
Initially, the implementation of predictive maintenance techniques can monitor the condition of the valve and its components in real time, allowing for proactive interventions prior to system failures. Secondly, it is imperative to guarantee that maintenance personnel are adequately trained and cognizant of the identified failure modes in order to implement effective maintenance practices. Finally, the continuous development of maintenance strategies can be facilitated by conducting regular reviews and updates that are informed by the most recent operational data and failure analysis.
The operational efficiency and safety of cryogenic globe valves in oxygen plants can be substantially improved by implementing these recommendations, resulting in reduced downtime and improved system reliability.
-
-
CONCLUSION
The failure modes of cryogenic globe valves utilized in oxygen plants were meticulously prioritized in this study through the application of the combined FAHP and TOPSIS methodologies. The integration of these methodologies enabled the development of targeted maintenance strategies by providing a quantitative and robust approach for evaluating and ranking the criticality of various failure modes.
The highest priority failure modes were identified as disc wear and scratch, damaged and fractured valve bodies, and valve stem assembly failures in the analysis. By conducting routine inspections, employing wear-resistant and high- quality materials, and employing predictive maintenance strategies, the valve's reliability and overall system safety can be substantially improved.
Furthermore, this investigation underscored the significance of ongoing enhancements to maintenance procedures. Organizations can achieve sustained improvements in valve performance by ensuring that maintenance personnel are adequately trained and routinely updating maintenance strategies based on the latest operational data and failure analysis.
Finally, the combined FAHP and TOPSIS methodologies not only offer a distinct framework for failure mode prioritization but also offer actionable insights for improving the operational efficiency and safety of cryogenic globe valves. The implementation of the recommendations derived from this study has the potential to result in increased safety, enhanced system reliability, and reduced downtime in oxygen plants. The approach can be further validated and refined by applying these methodologies to other critical components in similar operating environments in future research.
REFERENCES
-
J.B. Bowles and C.E. Peláez, "Fuzzy logic prioritization of failures in a system failure mode, effects and criticality analysis," Reliability Engineering & System Safety, vol. 50, pp. 203-213, Feb. 1995.
-
F.T.S. Chan, et al., "Integration of Fuzzy Logic and Analytic Hierarchy Process for Supplier Selection," International Journal of Production Research, vol. 46, pp. 381-400, Feb. 2008.
-
C. Kahraman, "Fuzzy Multi-Criteria Decision Making: Theory and Applications with Recent Developments," Springer Science & Business Media, 2008.
-
H. Liu, Q. Liu, and Q. Lin, "A modified FMEA approach for proactive prevention of failures based on multiple-criteria decision making," Journal of Intelligent Manufacturing, vol. 30, pp. 701-715, Jan. 2019.
-
D.H. Stamatis, "Failure Mode and Effect Analysis: FMEA from Theory to Execution," ASQ Quality Press, 2003.
-
M.L. Tseng, M.K. Lim, and W.P. Wong, "Sustainable supply chain management: A closed-loop network hierarchical approach," Industrial Management & Data Systems, vol. 119, pp. 671-688, Mar. 2019.
-
J. Wang, Q. Liu, and Z. Zhang, "A novel hybrid MCDM model combining DEMATEL, ANP, and Z-numbers for supplier selection," Applied Soft Computing, vol. 86, pp. 105887, 2020.
-
L.A. Zadeh, "Fuzzy sets," Information and Control, vol. 8, pp. 338-353, Mar. 1965.
-
Z. Zhou, Y. Yang, and H. Zhang, "Fuzzy FMEA based on a novel similarity measure of generalized fuzzy numbers and its application," Journal of Intelligent & Fuzzy Systems, vol. 40, pp. 2167-2179, 2021.
-
J.B. Bowles, "An assessment of RPN prioritization in a failure modes effects and criticality analysis," Proceedings Annual Reliability and Maintainability Symposium, 2004.
-
M. Braglia, "MAFMA: multi-attribute failure mode analysis," International Journal of Quality & Reliability Management, vol. 17, pp. 1017-1033, Sep. 2000.
-
D.Y. Chang, "Applications of the extent analysis method on fuzzy AHP," European Journal of Operational Research, vol. 95, pp. 649- 655, Dec. 1996.
-
C.T. Chen, "Extensions of the TOPSIS for group decision-making under fuzzy environment," Fuzzy Sets and Systems, vol. 114, pp. 1-9, Jan. 2000.
-
A.C.F. Guimaraes and C.M.F. Lapa, "Fuzzy inference to risk assessment on nuclear engineering systems," Applied Soft Computing, vol. 4, pp. 405-428, Oct. 2004.
-
A. Pillay and J. Wang, "Modified failure mode and effects analysis using approximate reasoning," Reliability Engineering & System Safety, vol. 79, pp. 69-85, Jan. 2003.
-
M. Ben-Daya and A. Raouf, "A revised failure mode and effects analysis model," International Journal of Quality & Reliability Management, vol. 13, pp. 43-47, Jan. 1996.
-
K.H. Chang, P.Y. Liu, and C. Wei, "Failure mode and effects analysis using grey theory," Integrated Manufacturing Systems, vol. 12, pp. 211-216, Sep. 2001.
-
Q. Zhou, et al., "Integrating risk assessment in sustainable supply chain management: A literature review and a new framework," Journal of Cleaner Production, vol. 287, pp. 125070, 2021.
-
Y.M. Wang and K.S. Chin, "Fuzzy analytic hierarchy process: A logarithmic fuzzy preference programming methodology," International Journal of Approximate Reasoning, vol. 50, pp. 1244- 1258, Aug. 2009.
-
V. Ebrahimipour, K. Rezaie, and S. Shokravi, "An approach for making risk-based maintenance decisions in marine systems," Journal of Marine Engineering & Technology, vol. 9, pp. 3-12, 2010.
-
C. Kahraman and S. Cebi, "A new multi-attribute failure mode analysis approach: An application to the landing system of a training plane," Engineering Applications of Artificial Intelligence, vol. 22, pp. 787- 795, Jul. 2009.
-
W. Gilchrist, "Modelling failure modes and effects analysis," International Journal of Quality & Reliability Management, vol. 10, pp. 16-23, Oct. 1993.
-
Website: www.ntgdvalve.com
IJERTV13IS070053
(This work is licensed under a Creative Commons Attribution 4.0 International License.)