
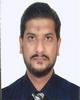
- Open Access
- Authors : Imran Hussain
- Paper ID : IJERTV13IS090045
- Volume & Issue : Volume 13, Issue 09 (September 2024)
- Published (First Online): 28-09-2024
- ISSN (Online) : 2278-0181
- Publisher Name : IJERT
- License:
This work is licensed under a Creative Commons Attribution 4.0 International License
Experimental and Ann-Gtm Based Optimization of Novel Environment-Friendly Natural Fuel Additives
Abstract
Authors; Imran Hussain
Mehran university of Engineering and Technology Sindh Pakistan- 76060 Pakistan
Corresponding Authors:
-
Imran Husain
of fossil fuels and the adverse effects of engine emissions like
Diesel engines available in various sizes, ranging from a few horsepower to 10,000 hp, provide a self-reliant energy source and are extremely reliable if maintained properly. However, they release toxic gases like nitrogen oxide, carbon monoxide, unburnt hydrocarbons, and particulate matter extremely harmful to the environment. Nowadays, diesel is blended with fuel-additives such as vegetable-based oils and metals oxides to control engine emissions. In the present study, the effect of fuel additives such as multi-walled carbon nanotubes, iron oxide, and graphite powder on controlled engine emissions in terms of the reduced quantity of toxic gases and un-burnt hydrocarbons have been investigated. Briefly, the emission of a single-cylinder diesel engine, operated at varying loads and speeds, fueled with plain-diesel and blends of diesel with fuel additives were measured using automobile emission analyser with and without using customed designed microfilter. The experimental quantitative data of engine emissions, i.e., total hydrocarbons, carbon mono and dioxide, obtained from automobile emission analyser was then analysed using the Grey Taguchi method with minimum the better criteria. Taguchi quality loss function and overall-grey-relation-grade indicated a set of inputs for minimum output responses.
The experimental and GTM optimized results were then validated using artificial neural network. The output responses were optimized at A=2, B=3, and C=4 for THCs, A=1, B=1, and C=1 for CO2 emission, and A=4, B=4, C=1. The experimental results were again measured at similar input factors and levels with the implementation of a novel HE-OB-MF after treatment retrofit. The results of THC and CO2 were abated by 18.5 % and 16 % respectively, and CO emissions were found a bit increased with heat exchanger oil bath micro filter retrofit (HE-OB-MF).
Keywords: Non-road diesel engine, Grey Taguchi method, Carbon nanotubes, Graphite powder, ANN
-
INTRODUCTION
Daily life facilities need some type of prime mover to supply mechanical power for pumping, electrical power generation, operation of heavy equipment, and to act as a backup electrical generator for emergency use during the loss of the regular power source. Several prime movers are available, i.e., gasoline engines, steam, and gas turbines, nevertheless diesel engines remain equally popular. They are small, inexpensive, powerful, fuel- efficient, and extremely reliable if appropriately maintained [1]. However, diesel engines are known to release toxic environmental gases like nitrogen oxide (NO), particulate matter (PM), carbon monoxide (CO), and unburned hydrocarbons (UHCs) [2]. In addition, the depleting reservoirs
THCs, PM and CO2 has forced researchers towards the experimental investigation of biodiesels as fuel alternatives. The use of biodiesels enhances combustion and performance of diesel engines, but nuclei-mode particulate emissions may increase substantially.
Recently, several attempts have been made to reduce engine emission by blending plain-diesel with a variety of fuel additives. For instance, a study has reported that nanoparticles of aluminum oxide (Al2O3) dosed in diesel fuel resulted in a greater reduction in NO, CO, UHC, and smoke emissions which ultimately boosted the brake-thermal efficiency of a diesel engine compared to that of plain diesel [3]. Similarly, cerium oxide (CeO2) nanoparticles mixed in bio-diesel fuel resulted in a significant improvement in engine efficiency and reduction of UHCs and NO emissions. The rationale behind using metal additives such as CeO2 and Al2O3 is that these metal oxides generally decompose on burning, thus releasing active metal ions, before the vaporization of fuel and water, reducing the formation of sunburnt carbon [4]. This also promotes low surface friction between the engine parts and improve engine fuel consumption. In contrast, metal additives are toxic to human health as they are also released as part of the combustion by-products [5] and hence must be controlled.
Therefore in lieu of metal oxides and metallic nanoparticles,
multi-walled carbon nanotubes (MWCNTs) and graphite powder can be environmentally friendly fuel additives because they form free-metal compounds. They provide high heat of combustion and boost chemically active sites for complete combustion [6], which effectively reduces diesel engine emissions, thereby improving engine fuel consumption [7]. Similarly, the exceptionally high thermal conductivity of CNTs can enhance nanofluids' thermal properties [8]. The addition of CNTs in diesel can also increase the fuel's cetane number as it acts as a catalyst to accelerate the burning rate. Tramp metals and tramp metal ions tend to bundle with impurities found in the fuel and form insoluble complexes, negatively affecting engine performance [8]. Carbon fibers in CNTs act as sequestering agents for tramp metals present in diesel fuel, thus resulting fuels will have fewer insoluble impurities [9]. Table 1 summarizes the use of fuel additives in conjunction with bio- diesel along with major findings.
Table 1. Literature review of fuel additives for bio-diesel.
Fuel Additives
Major Findings
MWCNTs
Optimised emission characteristics are obtained at a dose level of 30 mg/l where remarkable emission reduction is observed; NOx by 45 %, CO by 50 % [11].
Mahua oil
biodiesel +
alcohol blends
CO emissions were reduced by 7.4 %, HC emissions were reduced by 5.7 %, smoke emissions were decreased
by 2.9 % [10].
Papaya and watermelon
seed oil
Smoke emissions were reduced by 8.3 %. HC emissions were found to be 23.8 % lesser.CO emissions decreased
by 27.27 % [12].
Graphite Oxide and Single-Walled
Carbon Nanotubes
Reduced CO emission up to 23.4 %, and lowered UHC emissions up to 24.1 %. SWCNTs and GO additives could be an effective approach to lowering engine emissions [13].
Biodiesel
Results showed that 20% of waste cooking biodiesel reduced harmful emissions of an unmodified stationary diesel engine [14].
Coconut shell oil
The coconut shell was used as a nanoparticle (20 nm) along with diesel and biodiesel blends. NOx was reduced
by 18.56 %, and CO also decreased significantly [15].
Rice bran biodiesel and
n-butanol
Carbon monoxide emissions and smoke were found to be decreased with the inclusion of rice bran biodiesel in the
blends and were further decreased with n-butanol [16].
Mahua methyl ester +
nanoparticles
of titanium dioxide.
Biodiesel was prepared by transesterification processes. Heat rate is increased the adding of the nanoparticle because of an increase in more carbon combustions and therefore promote the complete combustions [17].
Hydrotreated vegetable oil
The results obtained from this study showed a significant reduction of carbon monoxide (52 %) and hydrocarbon (47 %) emissions. PM emissions were also reduced by 10
% by particulate mass [18].
Salvinia molesta (plant) oil
The emissions from this experimental setup showed a maximum reduction of CO, CO2, UBHC, NO, smoke as
14 %, 3.38 %, and 20.83 %, 12.86 %, 10.99 %,
respectively compared to diesel oil [19].
Poppy oil
Results described an increase in NOx but a decline in CO emissions [20].
Gasoline and kerosene
CO emissions were reduced up to a maximum of 45 %. NOx increased almost up to 7.5% [21].
Hydrogen
Due to the increase of hydrogen ratios NOx emission
was increased. Complete combustion of carbon reduced CO emissions [22].
Diesel- kerosene ethanol
The inclusion of ethanol in the adulterated diesel notably reduced engine exhaust emissions along with improvement in the performance and combustion
parameters [23].
Ethanol
Biodiesel with oxygen content increase NOx emissions.
Conversely optimum values of CO2, CO, and HC were obtained in the given experimental setup [24].
-
SCOPE OF THE MANUSCRIPT
Literature review, as summarized in table 1, has revealed a limited number of studies on the CNTs, graphite powder, and iron oxide as fuel additives with pure diesel oil. This work, thus, aims to dig out the effect of using different proportions of fuel additives in plain diesel on engine emissions. Additionally, this study intention is to examine the effects of graphite, iron oxide, and MWCNTs as a catalyst on the performance, characteristics of a single- cylinder direct injection diesel engine operated with plain diesel blends. Engine performance parameters, that is, the brake-thermal efficiency (BTE), brake power (BP), and heat release rate (HRR), would also be investigated.
The goal of the present experimental investigation is to investigate the effect of multi-walled carbon nanotubes (coal), graphite powder (lead pencil), and iron oxide (rust) on emissions of a single-cylinder non-road diesel engine at various operating conditions.
-
MATERIALS AND METHODS
The experiments were conducted at the internal combustion engine laboratory of Pakistan Institute of Engineering and Technology Multan, Punjab Pakistan. Single-cylinder non- road air cooled diesel engine with specifications in table 2 was used to conducted experiments as indicated in the DOE section. The specifications of dynameter, magnetic stirrer, fuels additives, and emission measurement, and emission control unit are provided in tables from 3 to 7.
Table 2. Specification of the diesel engine.
Engine Characteristics
Engine Specification
Engine Type
Single Cylinder, 4 Stroke, Air Cooled
Combustion System
Direct Injection
Bore x Stroke (mm)
70 x 55
Displacement (cc)
211
Engine Speed (rpm)
3000 3600
Maximum Output (HP)
3.8 – 4.2
Continuous Output (HP)
3.4 – 3.8
Fuel Tank Capacity
2.5
Lube Oil Capacity
0.75
Dimensions
420 x 360 x 460
Table 3. Specifications of magnetic stirrer
Properties
Values
Power Supply
AC 220 V/110 V 50 Hz/60 Hz
Speed
0-2400 rpm
Motor powers
25W
Heating power
200W
Temperature control range
Room temperature to 100 Celsius
Timer
0-120 min
Two-way rotation
Yes
Material
Steel
Table 4. Specifications of dynamometer
Properties
Values
Weight (Kg)
17
Frame Size
GF6054
HP
1
Poles
4
RPM
3000
Mounting
Foot
Type
Capacitor Start Capacitor Run
Table 5. Physical properties of carbon nanotubes
Properties
Values
Length
10-20 nm
Diameter
3-8 µm
Purity
>99 %
Density
1-2 g/cm3
Interlayer distance
0.34 nm
Table 6. Properties of iron oxide (FeO) and graphite powder.
Properties
Iron oxide
Graphite powder
Molecular weight
71.84
12.01
Melting point
1377 0C
3697°C
Boiling point
3414 0C
4200°C
Density
5.74 g/cm³
1.8g/cm3
Table 7. Specification of automobile emission analyser.
Measurements
CO2
CO
HC
Measuring Range
0 20 %
0 10 %
0 – 9999 ppm
Resolution
0.01 %
0.01 %
1 ppm
Warm-up Time
10 minutes
Display
LCD display
Response Time
TD + T90: 10 seconds (NDIR); ECD: 30
seconds
Power
110 V – 220 V±10 %, 50 Hz ± 1Hz
Operation Temperature
0 – 40
Dimension
260 mm × 180 mm × 360 mm
Net weight
6 kg
Flow rate
0.7 – 1.2 L/min
-
HEAT EXCHANGER OIL BATH MICRO FILTER
RETROFIT
The THC, CO2, and CO emission of single-cylinder diesel engine operated at varying loads and speeds fueled with plain diesel, and blends of diesel with MWCN tubes, Iron oxide and graphite powder as specified were measured using automobile emission analyser as specified in table 7 with and without custom designed heat-exchanger-oil bath- microfilter retrofit after treatment unit.
Figure 1. Heat exchanger-oil bath-micro filter retrofit.
The design of the experiment was performed in Minitab using Taguchi design of experiment method. Orthogonal array with L16 DOE model was selected based on three input variables (A: Fuel blend, B: Speed and C: Load) and four levels (LI, L2, L3, and L4) were used in orthogonal array design. Table 8 indicated input variables with their corresponding levels and table 9 indicates the number of experiments conducted with the defined inputs.
Table 8. Diesel engine inputs with levels.
Levels (L)
Input variables
Codes
L1
L2
L3
L4
Fuel additives
A
MWCNTs
G
FeO
D
Speeds
B
1800
2000
2200
2400
Loads
C
0%
33%
50%
100%
Table 9. Design of experiment.
Fuel Blends
A
Speed (RPM)
B
Load (%)
C
D+CNT
1
1800
1
0
1
D+CNT
1
2000
2
33
2
D+CNT
1
2200
3
50
3
D+CNT
1
2400
4
100
4
D+G
2
1800
1
33
2
D+G
2
2000
2
0
1
D+G
2
2200
3
100
4
D+G
2
2400
4
50
3
D+FeO
3
1800
1
50
3
D+FeO
3
2000
2
100
4
D+FeO
3
2200
3
0
1
D+FeO
3
2400
4
33
2
D
4
1800
1
100
4
D
4
2000
2
50
3
D
4
2200
3
33
2
D
4
2400
4
0
1
-
EXPERIMENTAL METHODOLOGY
-
Experiments with plain diesel
Experiment was performed on single-cylinder diesel engine with 100 ml plain diesel was poured it into a graded glass tube. The engine testbed was turned on and Set the apparatus at 0 % load and 1800 rpm. Turned the stopwatch on and noted the time the engine took to consume 10 ml of diesel. Noted the total hydrocarbon, CO2, and CO emission with and without HE-OB-MF retrofit from the digital indicators on the automobile emission analyser to be used for further analysis. Repeated same procedure at 1800, 2000, 2200 and 2400 rpm at 33 %, 50 %, and 100 % load.
-
Plain diesel and CNT
Took 80 ml Diesel in a beaker and poured 20 mg multi- walled Carbon Nano Tubes in it. Placed the beaker on a magnetic Stirrer and placed a stirring bar in it. Allowed the mixture to stir for 15-20 minutes at 2400 rpm. Poured the blend in the glass tube to be consumed by diesel Engine. Set the apparatus at 0% load and the speed at 1800 rpm. Turned the stopwatch on and noted the time the engine took to consume 10 ml of diesel blend. Noted the total hydrocarbon, CO2, and CO emission with and without HE-OB-MF retrofit from the digital indicators on the automobile emission analyser to be used for further analysis. The experiments were repeated with the procedure at 1800 rpm, 2000 rpm,2200 rpm, and 2400 rpm at 33 %, 50 %, and 100 % load.
Similar experimental methodologies were used for blends of graphite powder and iron oxide with a small proportion at specified stirring speed and temperature. The experimental emission results were measure for further analysis and discussions. The fuel was blended up to 20 minutes, and it had to be used within 20 minutes; otherwise, the nanoparticles would have been settled down in the beaker as they have large particle sizes. Particles suspended in liquids are prone to form aggregates that would finally lead to separation and settling due to gravity. On the other hand, aggregation is contemplated as a major mechanism responsible for nanofluids' enhanced thermal conductivity. A certain degree of aggregation in a nanofluid may be beneficial, but the ultimate settling would limit its practical use. In addition to thermal conductivity deterioration, separated large aggregates may clog filters and block the flow in narrow channels in heat transfer devices. However, for mineral extraction and effluent treatment industries, separation and settling are basic prerequisites of operation. To welcome or avoid it, one may need to understand the particle settling processes and settling rates.
indicates the number of experiments conducted fuels blends, speed (rpm) and load (%) are the defined inputs, whereas THC, CO2, and CO are the responses with and without HE- OB-MF retrofit.
Table. 10 Experimental outputs with defined inputs
Inputs
Output responses
Orthogonal arrays
Run #
Fuel blends
Speed (RPM)
Load (%)
Uncontrolled (without microfilter
retrofit)
Controlled (with microfilter retrofit)
THC (ppm)
CO2 (%)
CO (ppm)
THC (ppm)
CO2 (%)
CO (ppm)
1
D+CNT
1800
0
66
3
65
55
2.5
60
2
D+CNT
2000
33
46
7
60
38
5
56
3
D+CNT
2200
50
42
8
43
35
7
40
4
D+CNT
2400
100
40
10
36
33
8
32
5
D+G
1800
33
63
4
63
52
5
56
6
D+G
2000
0
56
5
52
46
4
48
7
D+G
2200
100
27
8
48
22
6
43
8
D+G
2400
50
31
11
41
26
2
37
9
D+FeO
1800
50
67
3
65
56
4
58
10
D+FeO
2000
100
56
5
60
46
3
53
11
D+FeO
2200
0
53
7
52
44
3
46
12
D+FeO
2400
33
47
9
45
39
5
40
13
D
1800
100
64
4
63
53
3.2
56
14
D
2000
50
42
4
51
35
3
45
15
D
2200
33
39
7
44
32
6
39
16
D
2400
0
36
9
38
30
7
35
5.4 Grey-Taguchi method (GTM)
The data series obtained from the experiment on a single- cylinder non-road diesel engine fueled with plain diesel, blends of diesel with multi-walled carbon nanotubes (coal), iron oxide (rust), and Graphite powder (Lead pencil) at specified operating loads and speeds were used to predict the optimised input and out comparable factors [25]. The prediction of optimised input factors and levels was performed in three steps. In the first step, grey relational generation of comparable data was obtained from experimental results using a normalising technique with smaller is the better as ideal methodology. In the second step, Taguchi quality loss function was used to determine the level of uncertainty in the predicted and original data series. In the end, in the third step, the overall grey relational grade was calculated to get optimised input factors for minimum output response and OGRG [26].
5.3.1 Grey relational generation
Grey relational analysis is majorly used to normalise the data series obtained from experiments into a comparable series of data with minimised uncertainty level. The experimental responses in the current study were then normalised using the grey relational model for the ideal case of smaller is the better by using equation 1 [27].
X (P )
max X
(P ) X 0 (P )
i i
i max X
i (P ) min X i
(P )
Fig.4. Experimental methodology.
Where Xi (P) is the normalised compareable to output for the
Xo (P) data value from table 10. Max X (P) and min X (P)
i i i
-
Experimental Results
The summary of results for the single-cylinder diesel engine under defined input levels and the designed number of experiments can be visualised in table 10. The run number
are the largest and smallest data point in the same table. The comparable data series of the experimental responses are provided in table 11. The run number indicates the number of data series rows and inputs, as well as smaller the better normalised responses, can be visualised in the same table.
Table 11. Gray relational generation
Table 12. Taguchi quality loss factor (0j) for emissions
Inputs
Smaller the better
Run number
Fuel blends
Speed (RPM)
Load (%)
THC (ppm)
CO2 (%)
CO (ppm)
Inputs
Smaller the better
2
1
2
2
0.5235
0.5000
0.1724
number
blends
(RPM)
(%)
(ppm)
(%)
(ppm)
3
1
3
3
0.6173
0.3750
0.7586
1
2
1
1
0.9753
0.0000
1.0000
4
1
4
4
0.6790
0.1250
1.0000
2
1
2
2
0.4765
0.5000
0.8276
5
2
1
2
0.0988
0.8750
0.0690
3
1
3
3
0.3827
0.6250
0.2414
6
2
2
1
0.2716
0.7500
0.4483
7
2
3
4
1.0000
0.3750
0.5862
4
1
4
4
0.3210
0.8750
0.0000
8
2
4
3
0.8889
0.0000
0.8276
5
2
1
2
0.9012
0.1250
0.9310
9
3
1
3
0.0000
1.0000
0.0000
6
2
2
1
0.7284
0.2500
0.5517
10
3
2
4
0.2716
0.7500
0.1724
7
2
3
4
0.0000
0.6250
0.4138
11
3
3
1
0.3457
0.5000
0.4483
8
2
4
3
0.1111
1.0000
0.1724
14
4
2
3
0.6173
0.8750
0.4828
10
3
2
4
0.7284
0.2500
0.8276
15
4
3
2
0.6914
0.5000
0.7241
11
3
3
1
0.6543
0.5000
0.5517
16
4
4
1
0.7654
0.2500
0.9310
12
3
4
2
0.5062
0.7500
0.3103
13
4
1
/td>
4
0.9259
0.0000
0.9310
14
4
2
3
0.3827
0.1250
0.5172
5.3.2
Taguchi quality loss function
15
16
4
4
3
4
2
1
0.3086
0.2346
0.5000
0.7500
0.2759
0.0690
1 2 1 1 0.0247 1.0000 0.0000
Run
Fuel
Speed
Load
THC
CO2 CO
12 3 4 2 0.4938 0.2500 0.6897
13 4 1 4 0.0741 1.0000 0.0690
9 3 1 3 1.0000 0.0000 1.0000
The difference between the experimental data and predicted normalised data termed as quality loss function 0. Model 2 was used to calculate the Taguchi QLF for the representation of correspondence between the original and comparable data series [28].
om= [ Xm(X, m = 1,2,3 . x) X* = 1.00 (2)
Where om is Taguchi quality loss function, Xm is the individual comparable data series from table 11, X* is the maximum value of data series, and x indicated the number
of experiments. The corresponding results of Taguchi
quality loss function for comparable data series are presented in table 12.
The overall grey relational grade and grey relational coefficient, as indicated in table 13 for the comparable data series indicated the minimum the best criteria for output responses [27]. It can be visualised that the results in run number 2 for A=1, B=2, and C=2 are the minima for all output responses in a combined way and the overall grey relation generation coefficient also minimum these inputs. Hence grey-Taguchi method has predicted that the total hydrocarbon, CO2, and CO emission may be minimum at an operating speed of 2000 rpm and 33% load with Diesel and Multi-walled carbon nanotubes as fuel. For all other input combinations, the emission results of all the output parameters could not optimise as a minimum. Individual
responses were minimum at some other input variables and levels.
min max
m
m max
1 y
(3)
Table 13. Grey relational coefficients and overall gray relational grade
ò (m
y m1
) m
Inputs
Smaller the better
Run number
A
B
C
THC (ppm)
CO2 (%)
CO (ppm)
1
1
1
1
0.3389
1.0000
0.3333
0.1045
2
1
2
2
0.5120
0.5000
0.3766
0.0868
3
1
3
3
0.5664
0.4444
0.6744
0.1053
4
1
4
4
0.6090
0.3636
1.0000
0.1233
5
2
1
2
0.3568
0.8000
0.3494
0.0941
6
2
2
1
0.4070
0.6667
0.4754
0.0968
7
2
3
4
1.0000
0.4444
0.5472
0.1245
8
2
4
3
0.8182
0.3333
0.7436
0.1184
9
3
1
3
0.3333
1.0000
0.3333
0.1042
10
3
2
4
0.4070
0.6667
0.3766
0.0906
11
3
3
1
0.4332
0.5000
0.4754
0.0880
12
3
4
2
0.4969
0.4000
0.6170
0.0946
13
4
1
4
0.3506
1.0000
0.3494
0.1063
14
4
2
3
0.5664
0.8000
0.4915
0.1161
15
4
3
2
0.6183
0.5000
0.6444
0.1102
16
4
4
1
0.6807
0.4000
0.8788
0.1225
(4)
An average GRC for each response is determined by the overall gray relation grade (OGRG). OGRG The whole thing Multi-response function of any process, using the Eq (iv)[26].
m is grey relational coefficient calculated by the model in equation (3) the value of coefficient = [0,1] was taken as
average 0.5 and
y
1
( m) 1 where y indicates the total
y m1
observations of the data.
-
-
RESULTS AND DISCUSSIONS
The single-cylinder non-road diesel engine was operated sixteen times with the combinations of the different levels of the influencing input factors, and output results are presented in Table 10. (A) Fuel Blends (B) Speed and (C) Load were selected as the three input factors for the engine. Four levels of variation for factor A are D, D+CNT,
D+FEO, and D+G and four levels of variation for factor B are 1800, 2000 2200, and 2400 rpm, and four levels of
divergence for factor C are 0% 33%, 50%, and 100%. Experimental data of output parameters, including THC, CO2 & CO was collected by using L16 (44) OA design as given in Table 10. The experimental data series were then normalised for conversion into comparable data series using Grey relational generation for minimum the better criterial. Taguchi QLF and OGRG methods were used to predict the most optimised set of input factors and levels for minimum emissions of the single-cylinder diesel engine. It can be visualised from table 12 that the results in run number 2 for A=1, B=2, and C=2 are the minima for all output responses in a combined way and the overall grey relation generation coefficient also minimum these inputs. Hence Grey-Taguchi method has predicted that the total hydrocarbon, CO2, and CO emission may be minimum at an operating speed of 2000 rpm and 33% load with diesel and multi-walled carbon nanotubes as fuel.
-
ANN Prediction
A computational model of the artificial neuron network (ANN) is based on the biological neural network's structure and functions. The information passing through the network influences the configuration of the ANN as it is dependent on this input and output by a neural network. ANNs are called nonlinear methods for statistical data processing, to model or track complex relationships between features [29]. The artificial neural network (ANN parallel) distributed processing Units function like human brain neurons, saving experimental information and supplying it at all times. Via a learning and training phase, these neurons may store required data from the experimental data. The synaptic weight of ANN neuron is associated with each other to operate based on the type of feature triggered (such as TANSIG or PURELIN) for deciding the output/response for each of the input signals. The most optimised set of inputs was predicted using the artificial neural network method in MATLAB[30]. The UGI widow used ANN prediction was obtained with the nnstart tool. The input and output matrixes were imported from PC into the nnstart window. The fitting tool was used to train ANN over defined inputs for target outputs and the predicted targets were obtained over the unknown inputs. Fig 5a, 5b, 6a, 6b, and 7 indicate the ANN Training and prediction results.
Fig. 5. (a) nnstart tool MatLab. (b) NN fitting tool window
Three input parameters with 20 hidden neuron layers and three output layers were used for training the ANN. By hit and trial method, the number of hidden layers is evaluated with 16 test data until the mean squared error between the real experimental data and the expected data is reduced. 'Trainlm' trains the ANN model and changes the value and weight by optimisation for the Levenberg-Marquardt model [31]. Experimental, predicted, and controlled output responses can be visualised in table 14.
Fig. 8. ANN Architecture
-
Optimisation of total hydrocarbon emissions The bar charts in figure 9(a) indicate the summary of output response of THC emissions for the most optimised input factors, as indicated in run number 7 table 14. The total hydrocarbons were minimum at input factors and levels as A=2, B=3, and C=4. The ANN predicted results for total hydrocarbon emissions at these input factors and levels were on the higher side up to 18 % as compared to the experimental results. The variation between experimental and ANN modelled results could be due to human, environmental, instrumental, and silent parameters in the simulation tool. The total hydrocarbon emission was reduced by 18.5 % by the implementation of HE-OB-MF retrofit. As the diesel engine exhaust passed through the HE- OB-MF retrofit, the temperature of the exhaust emissions was reduced in the Heat Exchanger section, and some amount of soluble organic fraction of HCs was dissolved in lube oil contained in the oil bath section of the retrofit the exhaust gasses were then passed through baghouse (microfilter) where insoluble organic fraction was controlled by some level. It can be concluded from the experimental, grey Taguchi and ANN simulation results that if a single- cylinder non-road diesel engine fueled with a blend of graphite power and plain diesel in the proportion of ¼ (mg of graphite powder/ml of plain diesel) at 2200 rpm with full load condition the total hydrocarbon emissions could be minimum.
-
Optimisation of CO emissions
CO emissions are more related to the rich burn engines where the amount of oxygen supplied for combustion in the cylinder is limited or the amount of fuel is much higher than the stoichiometric ratio. In the current experimental study as MWCNTs, G, and Iron Oxide were used as fuel enrichment mixtures and their effect on emissions was studied. The experimental, ANN validated and CO emissions with HE- OB-MF retrofit could be visualised in figure 9(b). As per GTM criteria of minimum the better, the CO emission was observed as a minimum for input factors A=4, B=4, C=1 when the diesel engine was operated with plain diesel at 2400 rpm and no-load condition. The experimental, ANN predicted and CO results with HE-OB-MF are presented in figure 9(b). The CO emission results were found slightly higher with the implementation of HE-OB-MF retrofit as the exhaust gasses were slowed down as they passed through the Oil bath and microfilter section of the retrofit and out cylinder pressure of exhaust gasses increased.
-
Optimisation of CO2 emissions
The CO2 was observed minimum at input factors and levels as A=1, B=1, and C=1. The ANN predicted results for CO2 emissions at these input factors and levels were on the higher side as compared to the experimental results. The bar charts in figure 9(c) indicate the summary of output response of CO2 emissions for the most optimised input factors as indicated run number 1 table 14. The validation of experimental and ANN modeled results could be Visualised in figure 9(c). The CO2 emission was reduced by 16 % by the implementation of HE-OB-MF retrofit. As the diesel engine exhaust passed through the HE-OB-MF retrofit the
temperature of the exhaust emissions was reduced in the heat exchanger section and some amount of soluble organic fraction of CO2 was dissolved in lube oil contained in the oil bath section of the retrofit the exhaust gasses were then passed through baghouse (microfilter). It can be concluded from the experimental, grey Taguchi and ANN simulation results that if a single-cylinder non-road diesel engine fueled with a blend of MWCNTs and plain diesel in the proportion of ¼ (mg of MWCNTS/ml of plain diesel) at 1800 pm with no load condition the CO2 emissions could be minimum. The reduction in CO2 with multi-walled carbon nanotubes as an additive was due to the transfer of lean burning of mixture towards rich burning due to the added amount of pure carbon.
Fig.9. Experimental, ANN, and Abated THCs
-
-
CONCLUSION
The experimental study was conducted with a motivation to investigate the effect of natural fuel additives like multi- walled carbon nanotubes, graphite powder, and iron oxide on THCs, CO2, and CO emissions of a single-cylinder non- road diesel engine at various load and speed conditions. The experimental study was started with L16 DOE in Minitab. 17 a statistical tool that suggested 16 experiments depending upon three inputs and fours levels. The experimental results of THC, CO2, and CO were then analysed using the grey Taguchi method to transform the data series into comparable data series with minimum better criteria. Taguchi QLF and OGRG indicted the optimised set of inputs for minimum output responses. The experimental and GTM optimised results were then validated by using a trained Artificial Neural Network model using the nnstart tool in MATLAB
17. The output responses were optimised over at A=2, B=3, and C=4 for THCs, A=1, B=1, and C=1 for CO2 emission, and A=4, B=4, C=1. The experimental results were again measured at similar input factors and levels with the implementation of a novel HE-OB-MF after treatment retrofit. The results of THC and CO2 were abated by 18.5% and 16% respectively and CO emissions were found a bit increased with HE-OB-MF.
-
ACKNOWLEDGMENT
The authors are grateful to the Pakistan Institute of Engineering and Technology Multan, Pakistan for providing the internal combustion engine laboratory facility for this study.
-
CONFLICT OF INTEREST
This experimental study was conducted and supported by the authors only authors declare no conflict of interest with any funding agency or individual.
-
POLICY STATEMENT
The fuel additives used in the current study are natural element resources and can be manufactured at the mass production level. The amount of natural additives used in this study was 0.025 % of the total plain diesel by weight. Total plain diesel imports of Pakistan are 5.6 million tones/year [32]. The replacement of diesel with these additives shall save the capital in billions. The sustainability of plain diesel for future endeavors shall be another outcome of this replacement. A policy could be devised at the national level to replace 0.025 % of the plain diesel with the most optimised natural fuel additive MWCN or Graphite powder as suggested in this study.
-
REFERENCES
-
-
S. Shuai, X. Ma, Y. Li, Y. Qi, and H. Xu, "Recent Progress in Automotive Gasoline Direct Injection Engine Technology," Automot. Innov., vol. 1, no. 2, pp. 95113,2018.
-
Y. Zhang, D. Lou, P. Tan, and Z. Hu, "Particulate emissions from urban bus fueled with biodiesel blend and their reducing characteristics using particulate after-treatment system," Energy, vol. 155, pp. 7786, 2018.
-
M. Zhang, W. Hong, F. Xie, Y. Su, H. Liu, and S. Zhou, "Combustion, performance and particulate matter emissions analysis of operating parameters on a GDI engine by traditional experimental investigation and Taguchi method," Energy Convers. Manag., vol. 164, no. November 2017, pp. 344352, 2018.
-
E. Khalife, M. Tabatabaei, A. Demirbas, and M. Aghbashlo, "Impacts of additives on performance and emission characteristics of diesel engines during steady state operation," Prog. Energy Combust. Sci., vol. 59, pp. 3278, 2017.
-
A. DomÃnguez-Sáez, G. A. Rattá, and C. C. Barrios, "Prediction of exhaust emission in transient conditions of a diesel engine fueled with animal fat using Artificial Neural Network and Symbolic Regression," Energy, vol. 149, pp. 675683, 2018.
-
F. Kim, J. Luo, R. Cruz-Silva, L. J. Cote, K. Sohn, and J. Huang, "Self- propagating domino-like reactions in oxidised graphite," Adv. Funct. Mater., vol. 20, no. 17, pp. 28672873, 2010.
-
B. S. Smitha, A. K. M. P, K. Adyanthaya, M. K. S. A, and D. M. Prajwal, "Experimental studies on fuel filter coated with nanoparticles on the exhaust emissions of 4-stroke engine," Int. Res. J. Eng. Technol., vol. 4, no. 7, pp. 3436, 2017.
Influence of Multi-walled Carbon Nanotubes Additives into Non-edible Biodiesel-diesel Fuel Blend on Diesel Engine Performance and Emissions," Energy Procedia, vol. 100, pp. 166172, 2016.
[12]M. A. Asokan, S. Senthur prabu, S. Kamesh, and W. Khan, "Performance, combustion and emission characteristics of diesel engine fuelled with papaya and watermelon seed oil bio-diesel/diesel blends," Energy, vol. 145, pp. 238245, 2018. [13]J. B. Ooi, H. M. Ismail, B. T. Tan, and X. Wang, "Effects of graphite oxide and single-walled carbon nanotubes as diesel additives on the performance, combustion, and emission characteristics of a light-duty diesel engine," Energy, vol. 161, pp. 7080, 2018. [14]M. Mofijur, M. Rasul, N. M. S. Hassan, and M. N. Uddin, "Investigation of exhaust emissions from a stationary diesel engine fuelled with biodiesel," Energy Procedia, vol. 160, pp. 791797, 2019. [15]K. Vinukumar, A. Azhagurajan, S. C. Vettivel, N. Vedaraman, and A. Haiter Lenin, "Biodiesel with nano additives from coconut shell for decreasing emissions in diesel engines," Fuel, vol. 222, pp. 180184, 2018. [16]G. Goga, B. S. Chauhan, S. K. Mahla, and H. M. Cho, "Performance and emission characteristics of diesel engine fueled with rice bran biodiesel and n-butanol," Energy Reports, vol. 5, pp. 7883, 2019. [17]M. Udaya Kumar, S. Sivaganesan, C. Dhanasekaran, and A. Parthiban, "Analysis of performance, combustion and emission parameters in di diesel engine by using mahua methyl ester along with nano metal additives titanium dioxide," Mater. Today Proc., 2020. [18]E. Dobrzyska, M. Szewczyska, M. Poniak, A. Szczotka, B. Puchaka,and J. Woodburn, "Exhaust emissions from diesel engines fueled by different blends with the addition of nanomodifiers and hydrotreated vegetable oil HVO," Environ. Pollut., vol. 259, p. 113772, 2020.
[19]M. W. G. Qureshi, Z. M. Khan, M. Hussain, F. Ahmed, M. Shoaib, andM. Qasim, "Experimental Evaluation of a Diesel Engine for Combustion, Performance and Exhaust Emissions with Fuel Blends Derived from a Mixture of Fish Waste Oil and Waste Cooking Oil Biodiesel," Polish J. Environ. Stud., vol. 28, no. 4, pp. 27932803, 2019.
[20]A. Uyumaz et al., "Experimental investigation on the combustion, performance and exhaust emission characteristics of poppy oil biodiesel- diesel dual fuel combustion in a CI engine," Fuel, vol. 280, p. 118588, 2020. [21]M. S. Gad and M. A. Ismail, "Effect of waste cooking oil biodiesel blending with gasoline and kerosene on diesel engine performance, emissions and combustion characteristics," Process Saf. Environ. Prot., vol. 149, pp. 110, 2021. [22]K. Bayramolu and S. Ylmaz, "Emission and performance estimation in hydrogen injection strategies on diesel engines," Int. J. Hydrogen Energy, 2020. [23]S. Bhowmik, A. Paul, R. Panua, and S. K. Ghosh, "Performance, combustion and emission characteristics of a diesel engine fueled with diesel-kerosene-ethanol: A multi-objective optimisation study," Energy, vol. 211, p. 118305, 2020. [24]V. Ayhan, Ç. Çangal, . Cesur, and A. Safa, "Combined influence of supercharging, EGR, biodiesel and ethanol on emissions of a diesel engine: Proposal of an optimisation strategy," Energy, vol. 207, p. 118298, 2020. [25]G. Pohit and D. Misra, "Optimisation of Performance and Emission Characteristics of Diesel Engine with Biodiesel Using Grey-Taguchi Method," J. Eng., vol. 2013, p. 915357, 2013. [26]M. Gul et al., "Grey-Taguchi and ANN based optimisation of a better performing low-emission diesel engine fueled with biodiesel," Energy Sources, Part A Recover. Util. Environ. Eff., vol. 0, no. 0, pp. 114, 2019. [27]S. Yessian and P. A. Varthanan, "Optimisation of Performance and Emission Characteristics of Catalytic Coated IC Engine with Biodiesel Using Grey-Taguchi Method," Sci. Rep., vol. 10, no. 1, pp. 113, 2020. [28]A. Kumar, "TAGUCHI LOSS FUNCTION AS OPTIMISED MODEL FOR SUPPLIER SELECTION AND EVALUATION Address for Correspondence TAGUCHI LOSS FUNCTION AS OPTIMISEDMODEL FOR," no. January, pp. 14, 2015.
[29]S. Agatonovic-Kustrin and R. Beresford, "Basic concepts of artificial neural network (ANN) modeling and its application in pharmaceutical research.," J. Pharm. Biomed. Anal., vol. 22, no. 5, pp. 717727, Jun. 2000. [30]S. Ozan, M. Taskin, S. Kolukisa, and M. S. Ozerdem, "Application of ANN in the prediction of the pore concentration of aluminum metal foams manufactured by powder metallurgy methods," Int. J. Adv. Manuf. Technol., vol. 39, no. 3, pp. 251256, 2008. [31]H. P. Gavin, "The Levenburg-Marqurdt Algorithm For Nonlinear Least Squares Curve-Fitting Problems," Duke Univ., pp. 119, 2019. [32]P. M. of Finance, "Energy outlook for year 2011-12," Pakistan Econ.Surv., pp. 193220, 2012.