
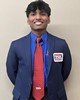
- Open Access
- Authors : Mihir Gannavarapu, Avaneesh Mohapatra, Nishant Gadde, Sudhanva Vasista
- Paper ID : IJERTV13IS090060
- Volume & Issue : Volume 13, Issue 09 (September 2024)
- Published (First Online): 16-10-2024
- ISSN (Online) : 2278-0181
- Publisher Name : IJERT
- License:
This work is licensed under a Creative Commons Attribution 4.0 International License
A Dual-Sensor Frame Work for Predictive Maintenance in Additive Manufacturing Integrating Acoustic Emission and Infrared Thermography for Enhanced Defect Detection
Mihir Gannavarapu |
Avaneesh Mohapatra |
Nishant Gadde |
Jordan High School |
West Forsyth High School |
Jordan High School |
Fulshear, USA |
Atlanta, USA |
Fulshear, USA |
Sudhanva Vasista
Jordan High School Fulshear, USA
AbstractAdditive manufacturing, popularly known as 3D printing, is the breakthrough process coming up for making components, which particularly helps high-performance industries such as aerospace, which rely largely upon performance optimization and ma-terial efficiency. The possible geometrical intricacies and minimal material waste have greatly improved the performance profile concerning fuel efficiency and power output. With these benefits, however, aerospace manufacturing is very cautious about full exploitation because of issues in process consistency, part reliability, and defect detection. This paper introduces a new framework for improving the predictive maintenance of AM processes, meeting the unique needs of aerospace manufacturing. The integration of AE sensors with infrared thermography provides a dual-sensor setup for complete internal and external anomaly monitoring in real-time during production. Data from these sensors can be fed into sophisticated machine learning algo-rithms to further enable earlier detection of defects in the process, increasing the overall consistency of the manufacturing run. Besides, the proposed research methodology intends to formulate a deep learning-based data analysis framework that can help with more accurate defect prediction and prevention. Such a dual-sensor system and predictive maintenance framework might open the route for much wider industrial con- sequences than within the aerospace sector per se; extension toward automotive and even medical device manufacturing could also be interesting. This presented work highlights the opening of opportunities for defect detection and process control due to the integration of AE sensors, infrared thermography, and machine learning into the AM process. An approach like this opens the door for improved reliability of AM parts for mission-critical applications, which in turn leads to the increased adoption of these parts in industries where the stake is high, such as aerospace.
Index TermsAdditive Manufacturing, Acoustic Emission, Infrared Thermography, Predictive Mainte-nance, Defect Detection
-
INTRODUCTION
Additive Manufacturing (AM), often referred to as 3D printing, represents a transformative shift in the way components and systems are designed and manufactured. Unlike traditional subtractive manu-facturing processes that remove material to create a part, AM builds components layer by layer, allow-ing for unprecedented design flexibility and material efficiency [1]. This capability has made AM increas-ingly valuable across various industries, particularly in aerospace, where the demand for lightweight, high-performance components is critical. The ability to manufacture complex geometries, reduce material waste, and produce parts on demand offers significant advantages, such as reducing lead times and enabling rapid prototyping [2]. In the aerospace industry, the potential of AM is particularly compelling. The use of AM in aerospace has already demonstrated re-markable benefits, such as in the case of the Cessna Denali aircraft engine, where AM reduced the number of components from 855 to just 12, resulting in a 10However, despite these advantages, the aerospace industry remains cautious about fully integrating AM into the production of mission-critical components. This hesitancy stems from several persistent chal-lenges associated with AM processes, particularly the lack of process consistency and the significant variation in the physical properties of parts [5]. Vari-ability in material properties, dimensional accuracy, and surface finish can lead to defects such as porosity,
distortion, and residual stresses [6]. These defects are especially concerning in aerospace applications, where the failure of a single component can have catastrophic consequences. As a result, ensuring the defect-free production of parts is paramount to in-creasing the adoption of AM in the aerospace in-dustry [7]. To address these challenges, advanced monitoring and predictive maintenance strategies are crucial. Predictive maintenance involves the use of data-driven approaches to predict when equipment or processes will fail, allowing for timely intervention before defects manifest in the final product [8]. In the context of AM, predictive maintenance can significantly enhance the reliability and consistency of the manufacturing process by detecting incipient faults early in the production cycle [9]. Recent ad-vancements in computational modeling and sensor technologies offer promising avenues for improving predictive maintenance in AM [10]. For instance, real-time monitoring using in-situ sensors has been successfully employed in various industries to track critical process parameters and detect anomalies be-fore they result in defects [11]. In theory, com-bining these sensor data with advanced computa-tional models, such as digital twins, could provide a powerful tool for enhancing process control in AM. A digital twin, which is a virtual representation of the physical manufacturing process, can simulate the process in real-time, allowing for the predic-tion of temperature distribution, material behavior, and potential defects with high accuracy [12]. This hybrid approachintegrating physics-based models with real-time sensor datahas been shown to im-prove fault detection and process optimization in other manufacturing domains, making it a promising candidate for overcoming the challenges faced in AM
[13]. The methods explored in this paper build on these advancements by applying them to the specific context of AM processes used in aerospace appli-cations. The goal is to develop a robust predictive maintenance framework that leverages the strengths of digital twins and in-situ sensor data to enhance process consistency, reduce defects, and ultimately increase the adoption of AM for mission-critical aerospace components [14].A. Novel Contribution/Motivation
This paper introduces a novel approach to improving predictive maintenance in Directed Energy Deposition (DED) and other metal-based AM processes through the integration of an advanced dual-sensor system combining Acoustic Emission (AE) sensors with infrared thermography. This dual-sensor system represents a significant advancement in process monitoring, offering a more comprehensive solution for detecting both internal and external anomalies during the AM process [15].
-
Dual-Sensor Integration and Advanced-Data Analysis Framework for Enhanced Predictive Maintenance: Application- Specific Adaptation for Aerospace Manufacturing: The proposed sensor integration is tailored to meet the stringent reliability and precision requirements of aerospace
ISSN: 2278-0181
Vol. 13 Issue 9, September 2024
manufacturing. Aerospace components, particularly those produced using AM, must withstand extreme operational conditions and adhere to strict safety standards [21]. The dual- sensor system is designed to address these needs by providing real-time monitoring that aligns with the high-rliability standards of the aerospace industry. For example, in the production of 3D-printed rocket engines or other critical aerospace components, early detection of internal material stresses and thermal anomalies is crucial [22]. The AE sensors enable the detection of microstructural changes that could compromise the parts integrity, while infrared thermography ensures optimal thermal management during the build process [23].
-
Potential for Broader Industry Impact and Scal-ability: While the proposed system is specifically designed for aerospace applications, its principles of dual-sensor integration and advanced data analysis can be adapted to other industries where AM is crit-ical. The methodologys adaptability and scalability make it a valuable contribution to the broader field of AM, with potential applications in industries ranging from automotive to medical devices [24]. The novel contribution of this paper lies in the introduction of a dual-sensor system combining AE sensors and in-frared thermography, the development of an advanced data analysis framework using machine learning, and the application-specific adaptation for aerospace man-ufacturing. This comprehensive approach addresses key challenges in predictive maintenance for AM, offering a robust solution that enhances the reliability, efficiency, and scalability of 3D printing technologies in high- stakes industries like aerospace [25].
-
-
METHODOLOGY
-
3.1 Dual-Sensor Data Acquisition
3.1.1 Acoustic Emission Sensing: To effectively capture the acoustic emissions during the additive manufacturing (AM) process, a fiber Bragg grating (FBG) sensor should be selected for its high sensitivity and broad frequency response. The chosen FBG sensor should have a sensitivity range of -60 to
+60 dB and a frequency response from 100 kHz to 1 MHz. This range ensures the capture of a comprehensive spectrum of acoustic emissions relevant to the AM process. A custom- designe mounting bracket for the FBG sensor should be employed to secure the sensor to the build platform while minimizing interference from vibrations. The optimal positioning of the sensor is crucial; it should be placed as close as possible to the build areatypically within 50-100 mm from the melt poolwithout obstructing the laser or powder delivery system. Furthermore, a high-speed data acquisition system, capable of sampling at 2 MHz, is necessary to accurately capture rapid acoustic events.
2) 3.1.2 Infrared Thermography: For thermal monitoring, a high-resolution infrared camera is es-sential. A camera with a resolution of 640×480 pixels or higher, operating within the spectral range of 3-5 µm or 8-14 µm, is suitable for metals. The camera should be mounted at an optimal angle (e.g., 45° to the build platform) to capture the entire build area without obstruction. The system should be capable of high frame rates (e.g., 100 Hz or higher) to record rapid thermal changes throughout the build process. To ensure accurate temporal alignment of data streams, a triggering system must be imple- mented to synchronize infrared image capture with acoustic signal collection.
-
3.2 Experimental Design
-
3.2.1 Process Parameter Tuning: Identifying key process parameterssuch as laser power, scan speed, hatch spacing, and layer thicknessis crucial for influencing part quality. A design of experiments (DOE) approach should be used to systematically vary these parameters and their interactions. Process modeling software can assist in predicting parameter combinations likely to achieve the target porosity levels for each quality category. Preliminary builds should validate these predictions, and parameter sets should be iteratively refined to consistently achieve the desired quality levels.
-
3.2.2 Sample Production: To ensure statistical significance, a minimum of 30 samples should be fabricated for each quality category. Test specimens must be designed to include features relevant to typical AM parts, such as overhangs, thin walls, and complex internal structures. Consistency in en-vironmental conditionssuch as oxygen levels and chamber temperaturemust be maintained across all builds. Detailed documentation of process parame-ters, environmental conditions, and any deviations or anomalies observed during each build is essential for reliable results.
-
-
3.3 Data Preprocessing and Feature Extraction
-
3.3.1 Acoustic Signal Processing: Raw acoustic signals should be processed by applying a band-pass filter to remove low-frequency machine noise and high-frequency electromagnetic interference. The continuous acoustic signal must be segmented into discrete time windows corresponding to individual laser scans or layers. A continuous wavelet transform (CWT) using an appropriate mother wavelet, such as Morlet or Daubechies, should be implemented to han-dle transient signals. Spectrograms generated from the CWT coefficients will provide time-frequency-amplitude information. A spectral clustering algo-rithm, such as k-means or DBSCAN, can be used to group similar acoustic signatures and create encoded label representations.
-
3.3.2 Thermal Image Processing: For ther-mal image processing, flat-field and non-uniformity corrections must be applied to raw images to ad-dress camera sensor variations. Adaptive thresholding should be utilized to isolate the melt pool from the surrounding area in each frame. Features such as the melt pool area, aspect ratio, centroid position, and maximum temperature should be extracted. Tempera-ture gradients can be calculated using finite difference methods, and cooling rates should be estimated by tracking temperature
changes of fixed points over consecutive frames.
-
-
3.4 Data Fusion and Advanced Analysis Frame-work
-
3.4.1 Sensor Data Integration: A temporal alignment algorithm must be developed to precisely match acoustic events with corresponding thermal images. A unified feature vector combining acoustic and thermal features for each time step should be created. Feature scaling and normalization are neces-sary to ensure all inputs are on a comparable scale for machine learning models.
-
3.4.2 Machine Learning Model Development: The deep convolutional neural network (CNN) ar-chitecture should include separate input branches for acoustic and thermal data, with convolutional layers for feature extraction. Fusion layers will combine features from both branches before classification lay-ers. Transfer learning, utilizing weights from pre-trained models on related tasks, can enhance perfor-mance. Data augmentation techniquessuch as ran-dom cropping, rotation, and noise additionshould be employed to improve model generalization.
-
3.4.3 Classification and Anomaly Detection: The CNN
should be trained using a stratified k-fold cross-validation approach to ensure robustness across all quality categories. Ensemble methods, like bagging or boosting, can be applied to improve clas-sification accuracy and reduce overfitting. Anomaly detection modules, using techniques such as autoen- coders or one-class SVMs, should be developed to identify deviations from expected signal patterns. Calibration of anomaly detection thresholds should be conducted using a separate validation set to balance sensitivity and specificity.
-
-
3.5 Real-time Monitoring and Predictive Mainte-nance System
-
3.5.1 Online Data Processing Pipeline: A multi-threaded data processing architecture must be developed to handle parallel streams of acoustic and thermal data. A circular buffer system will man-age continuous data input without memory overflow, while feature extracion algorithms should be opti- mized for GPU acceleration to minimize processing latency. A queuing system will ensure that all samples are processed in order.
-
3.5.2 Dynamic Model Updating: An online learning framework should allow for incremental updates to the model as new data becomes available. A concept drift detection algorithm will identify sig-nificant changes in the underlying data distribution. A versioning system for model updates will enable rollback in case of performance degradation, and a feedback mechanism will incorporate post-build quality assessments into the training dataset.
-
3.5.3 Predictive Maintenance Integration: A rule-based expert system should translate model out-puts and detected anomalies into specific maintenance recommendations. Time series forecasting models, such as ARIMA or LSTM, will predict future quality trends based on historical data. A user interface should display real-time quality predictions, anomaly alerts, and maintenance suggestions, while a reporting system will generate detailed build quality reports and maintenance logs for each production run.
-
-
-
RESULTS
-
Overview of Model Performance
Additive manufacturing (AM) processes, particu-larly in aerospace, demand highly accurate defect prediction systems to ensure structural integrity and operational safety. In this study, we implemented two machine learning models Random Forest and Logistic Regressionon a dataset containing features related to defect detection in AM-produced parts. Both models underwent a thorough hyperparameter tuning process via RandomizedSearchCV, optimizing their configurations to achieve the best performance in predicting defects. The Random Forest model achieved a final accuracy of 85.96%, with an F1-score of 0.92 for the defective class. This indicates that the model is highly proficient in identifying defec-tive parts, which is crucial in aerospace applications where even small defects can lead to catastrophic failure. The Logistic Regression model performed similarly, with an accuracy of 85.34% and an F1-score of 0.92 for the defective class. However, Lo-gistic Regression had slightly higher false positives in the non-defective category.
Detailed Confusion Matrix Analysis
Figure 1. Random Forest Confusion Matrix
Figure 2. Logistic Regression Confusion Matrix
Figures 1 and 2 show the confusion matrices for both the Random Forest and Logistic Regression models. The confusion matrix is a critical tool for understanding not only the accuracy of the models but also their weaknesses in misclassifying defective and non-defective parts.
-
The Random Forest model successfully pre-dicted 509 defective parts out of 546, while mis-classifying 37 defective parts as non-defective. It also correctly identified 48 non- defective parts, but misclassified 54 non-defective parts as de- fective. This relatively high number of false positives in the non-defective category could be a result of the models sensitivity to noise or minor variations in the manufacturing process that resemble defects. This high false positive rate, while not ideal, is less critical than false negatives in aerospace applications because it errs on the side of caution mislabeling a part as defective prompts further inspection rather than allowing a defective part to pass unnoticed.
-
Logistic Regression exhibited a somewhat dif-ferent behavior. It identified 535 defective parts correctly but made
11 false negative predic-tions, where defective parts were classified as non-defective. This is particularly concerning for aerospace applications, as undetected de-fects could compromise part integrity during operation. Additionally, it misclassified 84 non-defective parts as defective, which is a higher false positive rate than Random Forest. The models tendency to overpredict defects could be attributed to its linear nature, which struggles with capturing complex interactions among fea-tures that non-linear models like
Random Forest can better exploit.
-
Feature Importance and Model Interpretability: The
importance of interpretability in defect detection models cannot be understated, particularly in safety-critical industries such as aerospace, where under-standing why a model made a certain prediction is as important as the prediction itself. One of the advantages of using Random Forest is its ability to provide feature importance metrics, which allow us to identify the most significant predictors of defects in the dataset.
Figure 3.Random Forest Feature Importance
Figure 3 illustrates the importance of the top 10 principal components (PCs) extracted using PCA (Principal Component Analysis). It is evident from the figure that PC6 contributes the most to the models decision-making process, followed by PC5 and PC3. These principal components likely encap-sulate complex relationships between various features in the dataset, such as temperature variations, material composition, and layer adhesionkey factors known to influence the presence of defects in AM processes.
-
PC6: The high importance of this component suggests that it might be capturing thermal anomalies during the AM process, which are often precursors to defects such as warping, cracking, or residual stresses. The integration of real-time thermal data, as suggested in the
methodology section of this paper, could further improve the models predictive power by allow-ing for the early detection of thermal defects.
-
PC5 and PC3: These components could be re-lated to material deposition consistency or laser power fluctuations, both of which are known to significantly impact the structural integrity of AM parts. The importance of these features highlights the need for continuous monitoring and fine-tuning of process parameters during production to minimize the risk of defects.
The interpretability of these results aligns with existing knowledge in the AM field, where ther-mal management and material consistency are well-established factors influencing part quality. The Ran-dom Forest modelâs ability to prioritize these factors gives it an edge in defect detection, especially when used in conjunction with real-time monitoring tools like infrared thermography.
Figure 4. Random Forest Feature Importance
-
-
Model Comparison and Implications for Aerospace Manufacturing: Figure 4 presents a side-by-side comparison of the accuracy of the Random Forest and Logistic Regression models. Although both models achieved similar overall accuracy, the Random Forest models more balanced approach to predicting both defective and non-defective parts makes it the preferable choice for real-world implementation in AM defect detection systems. The slightly higher accuracy of Random Forest (85.96% compared to Logistic Regressions 85.34%) may seem negligible, but its higher precision in predicting defective parts (F1-score of 0.92 compared to Logistic Regression 0.92) and its capacity to handle non-linear feature interactions provide significant advantages in high- stakes industries. In the context of predictive maintenance, both models offer promising avenues for defect detection in AM processes. However, the Random Forest model
demonstrates better resilience to false negatives (defective parts being misclassified as non-defective), which is of paramount importance in aerospace applications. Undetected defects can compromise component performance, leading to catastrophic failures, as seen in past incidents involving metal fatigue or structural weaknesses in aircraft components. The slight trade-off of higher false positives in Random Forest can be mitigated by implementing additional quality checks, ensuring that the occasioal false alarm does not disrupt the production flow unnecessarily. Furthermore, the Logistic Regression model, while slightly less accurate, provides a more interpretable framework for understanding how linear relationships between process variables contribute to defect formation. This could be useful for identifying straightforward correlations, such as the impact of layer thickness or laser power on defect rates, which can help refine AM process parameters.
-
-
Broader Industry Impact and Scalability
The findings from this comparison between Ran-dom Forest and Logistic Regression models are di-rectly applicable to predictive maintenance systems in AM, especially in aerospace manufacturing. As discussed in the papers background, the aerospace industry has stringent requirements for part qual-ity and reliability. The ability to detect defects in real-time using machine learning models trained on data from in-situ sensors offers a revolutionary ap-proach to process monitoring and control. The dual-sensor system described in the paper, which inte-grates Acoustic Emission (AE) sensors with infrared thermography, complements the model predictions by providing real-time data that can further improve the accuracy and reliability of these predictions. The insights gained from the Random Forest models feature importance analysis can guide the placement and optimization of sensor arrays in AM machines. For instance, since thermal anomalies are a signif-icant predictor of defects (as suggested by PC6s importance), optimizing infrared camera placement to capture a broader temperature range or improve spatial resolution could lead to even more accurate defect predictions. Similarly, the AE sensors could be tuned to detect microstructural changes that correlate with the principal components identified as important by the Random Forest model.
-
Future Research Directions
While this study demonstrates the effectiveness of Random Forest and Logistic Regression models for defect detection in AM, there is still significant room for improvement. Future research could focus on integrating deep learning models, such as Con-volutional Neural Networks (CNNs), with the dual- sensor system described in this paper. CNNs could be particularly effective in analyzing thermal images and acoustic signals in real-time, providing a more nuanced understanding of defect formation. Addi-tionally, exploring ensemble methods that combine the strengths of both Random Forest and Logistic Regression could result in even more robust predic-tive maintenance systems, reducing false positives while maintaining high recall for defective parts. The integration of digital twins, as discussed earlier, could further enhance the predictive power of these models by simulating various AM scenarios and training the models on synthetic data generated from these sim-ulations. By continuously updating the digital twin with real-time sensor data, the models can adapt to changes in the manufacturing environment, ensuring consistent quality even as production scales.
-
-
CONCLUSION
The proposed dual-sensor framework for real-time monitoring by combining Acoustic Emission sensors with infrared thermography is a great stride in the field of AM. This allows early detection of inter-nal and external defects, enhancing process consis-tency and reliability by tackling some of the critical challenges in high-stake industries like aerospace. The integration of machine learning algorithms en-hances predictive maintenance further through dy-namic, data-driven insights into defect formation. This innovative approach surely will find its niche in enhancing not only safety but also performance for mission-critical components within aerospace; meanwhile, it will be possible to adapt it to a wide range of industries, from automotive to medical device manufacturing. Because of this fact, growth in adoption may increase in the future, especially in those applications where defect prevention and process optimization bear paramount importance.
REFERENCES
-
N. Anwer and L. Mathieu. From geometry to topology: a step toward automated additive manufacturing process planning. CIRP Annals, 65(1):912, 2016.
-
S. Berumen, F. Bechmann, S. Lindner, J.-P. Kruth, and
T. Craeghs. Quality control of laser- and powder bed-based additive manufacturing (am) technologies. Physics Procedia, 5:617622, 2010.
-
L. N. Carter, C. Martin, P. J. Withers, and M. M. Attallah. The influence of the laser scan strategy on grain structure and cracking behaviour in slm ti-6al-4v. Materials & Design, 66:315324, 2014.
-
S. Cattaneo, L. Ciani, and G. Maccarini. Predictive main-tenance architecture for additive manufacturing equipment. IEEE Access, 8:172670172682, 2020.
-
P. Colegrove, H. Coules, D. Fairchild, R. Martukanitz,
R. Griffiths, A. Cashman, and F. Sciammarella. Microstruc-tural analysis and modelling to understand the relationship between process parameters and internal defects in laser powder bed fusion. Materials & Design, 182:108052, 2019.
-
T. DebRoy, H. Wei, J. Zuback, T. Mukherjee, J. Elmer,
J. Milewski, A. Beese, A. Wilson-Heid, A. De, and W. Zhang. Additive manufacturing of metallic componentsprocess, structure and properties. Progress in Materials Science, 92:112224, 2018.
-
R. Elkharoua.Predicting manufacturing defects
dataset. https://www.kaggle.com/datasets/rabieelkharoua/ predicting- manufacturing-defects-dataset/data, 2021.
-
C. Galy, E. Le Guen, F. Lacan, C. Arvieu, and T. Perdrizat. Microstructure and mechanical properties of titanium parts fabricated by additive manufacturing: A review. Materials Science and Engineering: A, 759:339 361, 2019.
-
F. He, Z. Zhang, and H. Hu. Real-time prediction of weld penetration during laser welding using an infrared sensor and deep learning. Journal of Intelligent Manufacturing, 30(2):487497, 2018.
J.Ma, I. Karaman, and R. Arroyave´. Numerical and experi-mental analysis of heat distribution in the laser powder bed fusion of ti-6al-4v. IISE Transactions, 51(2):136152, 2019.
[11]T. DebRoy, H. Wei, J. Zuback, T. Mukherjee, J. Elmer,J. Milewski, A. Beese, A. Wilson-Heid, A. De, and W. Zhang. Additive manufacturing of metallic componentsprocess, structure and properties. Progress in Materials Science, 92:112224, 2018.
[12]R. Elkharoua.Predicting manufacturing defectsdataset. https://www.kaggle.com/datasets/rabieelkharoua/ predicting- manufacturing-defects-dataset/data, 2021.
[13]C. Galy, E. Le Guen, F. Lacan, C. Arvieu, and T. Perdrizat. Microstructure and mechanical properties of titanium parts fabricated by additive manufacturing: A review. Materials Science and Engineering: A, 759:339361, 2019. [14]F. He, Z. Zhang, and H. Hu. Real-time prediction of weld penetration during laser welding using an infrared sensor and deep learning. Journal of Intelligent Manufacturing, 30(2):487497, 2018. [15]K. Karayagiz, A. Elwany, G. Tapia, B. Franco, L. Johnson,J.Ma, I. Karaman, and R. Arroyave´. Numerical and experi-mental analysis of heat distribution in the laser powder bed fusion of ti-6al-4v. IISE Transactions, 51(2):136152, 2019.
[16]T. Kellner. Fired up: Ge successfully tested its advanced turboprop engine with 3d-printed parts. GE Reports, 2018. [17]M. Khanzadeh, L. Bian, J. Luo, and K. Palaniappan. Real-time defect prediction for additive manufacturing processes using machine learning. In 25th Annual International Solid Freeform Fabrication Symposium, 2017. [18]M. Khanzadeh, S. Chowdhury, M. Tschopp, and L. Bian. Insitu laser speckle imaging for detection of defects in additive manufacturing. Optics & Laser Technology, 102:220228, 2018. [19]J. H. Martin, B. D. Yahata, J. M. Hundley, J. A. Mayer, T. A. Schaedler, and T. M. Pollock. 3d printing of high-strength aluminium alloys. Nature, 549(7672):365369, 2017. [20]S. Mehdi, M. Shokouhfar, and A. Abdollahi. A novel data fu-sion approach for process monitoring and diagnosis in laser-based additive manufacturing. Journal of Manufacturing Processes, 56:571582, 2020. [21]T. D. Ngo, A. Kashani, G. Imbalzano, K. T. Q. Nguyen, and-
Hui. Additive manufacturing (3d printing): A review of materials, methods, applications and challenges. Composites Part B: Engineering, 143:172196, 2018.
79(1-4):307316, 2015.
[24]W. J. Sames, F. List, S. Pannala, R. R. Dehoff, and S. S. Babu. The metallurgy and processing science of metal additive manufacturing. International Materials Reviews, 61(5):315 360, 2016. [25]H. Taheri, M. M. Shoaib, L. W. Koester, T. A. Bigelow,P. C. Collins, and L. J. Bond. Powder based additive manufacturinga review of types of defects, generation mech-anisms, detection, property evaluation and metrology. Int. J. Addit. Subtractive Mater. Manuf, 1(2):172209, 2017.
[26]V. Thombare, N. Dahotre, and N. B. Dahotre. A new acoustic emission monitoring system for laser assisted additive man-ufacturing of titanium alloy. Journal of Materials Processing Technology, 285:116785, 2020. [27] L. A. Verhoef, B. W. Budde, C. Chockalingam, B. Garc´a Nodar, andA. J. M. van Wijk. The effect of additive manufacturing on global energy demand: An assessment using a bottom-up approach. Energy Policy, 112:349360, 2018.
[28]Z. Yang. Model-based Predictive Maintenance of Manufac-turing Systems. PhD thesis, University of Massachusetts, 2018. [29]Z. Yang, D. Eddy, S. Krishnamurty, I. Grosse, P. Denno,Y. Lu, and P. Witherell. Investigating grey-box modeling for predictive analytics in smart manufacturing. In ASME, International Design Engineering Technical Conferences and Computers and Information in Engineering Conference, pages V02BT03A024034, 2017.
[30]Z. Zhang, F. He, Z. Han, Q. Huang, and H. Hu. Real-time monitoring of laser welding using an acoustic emission sen-sor. The International Journal of Advanced Manufacturing Technology,102(9-12):37013711, 2019.
[1] [2] [4] [3] [5] [6] [7] [8] [9] [10] [11] [12] [13] [14] [15] [16] [18] [19] [20] [21] [17] [22] [24] [23] [25]IJERTV13IS090060
(This work is licensed under a Creative Commons Attribution 4.0 International License.)