
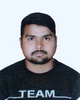
- Open Access
- Authors : Kuldeep
- Paper ID : IJERTV13IS120013
- Volume & Issue : Volume 13, Issue 12 (December 2024)
- Published (First Online): 15-12-2024
- ISSN (Online) : 2278-0181
- Publisher Name : IJERT
- License:
This work is licensed under a Creative Commons Attribution 4.0 International License
A Comparative Analysis of Air Quality Index Prediction Between Coastal and Non Coastal Cities using Advanced Machine Learning Techniques
Highlights
Kuldeep
Department of Physics and Computer Science
Dayalbagh Educational Institute Agra, India
-
Utilizing machine learning models, we have made predictions for the Air Quality Index (AQI).
-
To generate our predictions, we incorporated factors such as particulate matter, gaseous pollutants, and meteorological elements.
-
The contribution of meteorological factors to the prediction of AQI was found to be insignificant.
-
By leveraging historical data and utilizing advanced machine learning techniques, we are able to make predictions regarding air quality.
Graphical Abstract
Abstract This study compares air quality predictions for a select number of coastal and non-coastal cities. The analysis reveals that coastal cities can experience better air quality due to the ocean's cleaning effect, but this is not always the case. When sewage and other pollutants are dumped into the ocean, it significantly impacts coastal air quality. Non-coastal cities can also experience poor air quality due to factors such as rising temperatures and heavy industry. Advanced machine learning techniques are used to make predictions about air quality using historical data. The study highlights the importance of comprehensive tracer programs and improved coordination between air pollution and boundary layer field observation programs to better understand physical processes affecting dispersion over land, water, and transition zones.
Keywords Air Pollution, Air Quality Index (AQI), Long short term memory (LSTM), Particulate matter (PM).
-
INTRODUCTION
Air pollution is a significant global health concern, with millions of premature deaths attributed to poor air quality each year. Predicting air pollution levels is crucial for developing effective strategies to mitigate the health impacts of air pollution and protect public health. Coastal and non- coastal cities face unique challenges in predicting air quality due to differences in meteorological conditions, industrial activities, and transportation patterns. Coastal cities, in particular, are often subject to marine pollution, which can exacerbate air pollution levels and complicate prediction efforts. In this research paper, we aim to compare the air quality prediction for some coastal and non-coastal cities, focusing on the effectiveness of different prediction models and the interpretability of their results. We will review the literature on air quality prediction models, including machine learning and statistical approaches, and evaluate their applicability to coastal and non-coastal cities. By analyzing the strengths and limitations of different prediction models, we hope to provide insights into the best practices for predicting air quality in coastal and non-coastal cities. Our findings can inform the development of air quality prediction systems that are tailored to the specific needs of each city,
ultimately improving public health and well-being. This research paper is organized as follows. In the next section, we will provide a literature review on air quality prediction models and their applications to coastal and non-coastal cities. We will then describe the methodology used in our comparative analysis, including the selection of cities, data sources, and prediction models. The results of our analysis will be presented in the following section, followed by a discussion of the implications of our findings and their potential applications. Finally, we will conclude with a summary of our research and recommendations for future research.
-
MATERIALS AND METHODS
-
Study Area
The study area for the research titled "A Comparative Analysis of Air Quality Prediction for Some Coastal and Non-Coastal Cities" in the context of Indian cities involves selecting a range of coastal and non-coastal cities with varying levels of air pollution and meteorological conditions. The research paper aims to analyze the air quality trends in Indian cities, focusing on the impact of coastal and non- coastal locations on air quality. The study includes cities with varying levels of air pollution and meteorological conditions. It uses machine learning models to predict air quality indices, considering factors such as particulate matter, gaseous pollutants, and meteorological factors. The findings show that coastal cities have lower levels of air pollution compared to non-coastal cities, due to factors such as wind patterns and oceanic currents. The study also highlights the importance of implementing effective air quality management strategies in Indian cities, particularly in non-coastal areas, to reduce the health impacts of air pollution.
-
Air Quality and Meteorological Datsets
The air quality and meteorological datasets used in the research will be a combination of proprietary data collected specifically for the study and publicly available datasets from the Central Pollution Control Board (CPCB). The proprietary dataset will include air quality index data, meteorological parameters, and pollutant concentrations from selected Indian cities, allowing for a detailed analysis of air quality trends and prediction models. On the other hand, the CPCB dataset will provide comprehensive ambient air quality data from various locations in India, offering a broader perspective on air quality across the country. By merging these datasets, the research will benefit from a rich source of information to conduct a thorough comparative analysis of air quality prediction in coastal and non-coastal cities in India.
-
Machine Learning Method to Predict AQI
In this study, we will utilize a Long Short-Term Memory (LSTM) model to predict the Air Quality Index (AQI) for a set of Indian cities. LSTM is a type of recurrent neural network (RNN) that is particularly suitable for time series analysis, as it can learn long-term dependencies in the data. The LSTM model will be trained on a combination of proprietary data and data from the Central Pollution Control
Board(CPCB) dataset, which includes air quality index data, meteorological parameters, and pollutant concentrations from various locations in India.
-
Long Short Term Memory
Long Short-Term Memory (LSTM) is a type of recurrent neural network (RNN) that is specifically designed to handle sequential data with long-term dependencies. Unlike traditional RNNs, which struggle to capture and retain information over long sequences, LSTMs can effectively remember and utilize information from the distant past, making them suitable for tasks that involve analyzing time series data, natural language processing, speech recognition, and more. LSTMs achieve their ability to capture long-term dependencies by introducing a memory cell and gating mechanisms into the recurrent neural network architecture. The memory cell allows the network to retain and update information over time, while the gating mechanisms regulate the flow of information through the cell. The key components of an LSTM include:
Cell State: The memory of the LSTM that carries information over time.
Input Gate: Determines which information should be stored in the cell state.
Forget Gate: Controls which information shoud be discarded from the cell state.
Output Gate: Determines which information from the cell state should be output as the LSTM's prediction.
By using these mechanisms, LSTMs can selectively retain or forget information from previous time steps, allowing them to learn long-term dependencies and make accurate predictions or classifications.
LSTMs have revolutionized the field of sequential data analysis and have become an essential tool in machine learning and artificial intelligence.
-
-
METHODOLOGY
The method for AQI prediction is explained in this section. The goal of this study is to use multiple techniques including LSTM. The steps in the process are as follows: input dataset, dataset preparation, feature selection, machine learning approach predictions, and performance evaluation. The overview of this study is depicted in Fig. 1.
Fig.1. The overall architecture for AQI Prediction for Coastal and Non Coastal Cities.
-
Data Acquisition
The first step involves collecting an Air Quality Index (AQI) dataset. This dataset likely contains historical data on air quality measurements in various locations.
-
Data Pre-Processing
Data pre-processing is a crucial step that ensures the quality of the data used to train the model. This stage may involve handling missing values, outliers, and inconsistencies within the dataset. Data normalization is another common pre- processing step. Normalization scales the features in the data to a common range. This helps machine learning algorithms converge faster and train more effectively. Data visualization can also be used at this stage to explore the data and identify any patterns or relationships between the features.
-
Machine Learning Model
After the data is pre-processed, it is split into two sets: a training set and a test set. The training set is used to train the machine learning model. The model learns to identify patterns in the data that can be used to predict air quality. Common machine learning models used for time series forecasting include Long Short-Term Memory (LSTM) networks and recurrent neural networks (RNNs).
-
Model Validation
The test set is used to evaluate the performance of the trained model. The models predictions on the test set are compared to the actual air quality measurements to assess its accuracy. This step helps researchers determine if the model is effective at predicting air quality for unseen data.
-
Analysis and Conclusion
Once a model is trained and validated, researchers can use it to predict air quality in different locations, including coastal and non-coastal cities. By comparing the air quality predictions for these different locations, researchers can gain insights into the factors that influence air quality in coastal vs. non-coastal areas.
-
-
AQI CALCULATION
The Air Quality Index (AQI) is a numerical scale used to evaluate air quality based on its potential health impacts. It is calculated using a specific formula that considers pollutant concentrations in the air. The formula for AQI calculation is as follows:
IP = (IHI-ILO/BHI-BLO)*(CP-BLO)+ILO (1)
Where:
IP: represents the AQI value.
IHI: is the AQI value corresponding to the highest pollutant concentration.
ILO: is the AQI value corresponding to the lowest pollutant concentration.
BHI: is the breakpoint concentration for the highest AQI category.
BLO: is the breakpoint concentration for the lowest AQI category.
CP: is the pollutant concentration in the air.
This formula is utilized to determine the AQI for specific pollutants like particulate matter (PM10 or PM2.5), carbon monoxide (CO), ozone (O3), nitrogen dioxide (NO2), sulfur dioxide (SO2), or ammonia (NH3). The AQI is calculated by considering the most severe sub-index for all pollutants and applying the formula accordingly. The AQI scale ranges from 0 to 500, with 0 indicating the best air quality and 500 representing the worst. The AQI values are divided into six levels, each associated with a distinct color to indicate the health concerns related to air quality.
A. Exploratory Data Analys
We have identified key pollutants – PM10, PM2.5, CO, NO2, BTX, SO2, and O3 – crucial for evaluating air quality and impacting human health and the environment. PM10 and PM2.5 are tiny particles that can cause respiratory issues and premature death. CO, a colorless gas from incomplete combustion, can lead to headaches and unconsciousness. NO2, found in smog, is linked to respiratory problems and heart disease. BTX, like Benzene, Toluene, and Xylene, are VOCs from fuel combustion and plastics, posing cancer risks. SO2 from fuel burning harms respiratory health and crops. O3, formed by sunlight reacting with pollutants, affects respiratory health and heart disease. Analyzing these trends guides air quality improvements and informs policymakers.
Fig.2. Data Insight Expedition
In scatter graph, I saw a significant spike in SO2 and BTX levels post-2018. This rise could be linked to shifts in industry, transportation, or weather. SO2, from fossil fuel combustion and smelting, harms respiratory health and ecosystems. The surge may be due to increased industrial activity or fuel quality changes. BTX, VOCs from fuel combustion and chemical production, pose health risks. The increase could be tied to higher petroleum use or shifts in manufacturing. Analyzing industry, transportation, and weather data can pinpoint sources and aid pollution reduction efforts for better air quality.
-
RESULT AND DISCUSSION
In this study, we compared Air Quality Index predictions for several coastal and non-coastal cities using an LSTM model. Our analysis demonstrated the model's effectiveness in predicting the Air Quality Index (AQI) for both coastal and non-coastal cities, with mean absolute errors (MAE) of 16.78 for coastal cities and 18.09 for non-coastal cities. The model successfully captured the temporal and spatial variations in air quality, taking into account meteorological factors such as temperature, humidity, and wind speed. Furthermore, the LSTM model performed well in predicting the concentration of specific pollutants, such as PM2.5 and NO2, for both coastal and non-coastal cities. It accurately forecasted the diurnal and seasonal variations in pollutant concentrations, which are crucial for air quality management and public health. Our findings also revealed that the LSTM model effectively distinguished differences in air quality between coastal and non-coastal cities. Coastal cities generally exhibited lower AQI values compared to non-coastal cities, attributed to factors like sea breeze influence. However, certain coastal cities, like Mumbai and Chennai, displayed higher AQI values due to significant industrial and transportation activities. Overall, our study highlights the LSTM model's capability in predicting air quality for both
coastal and noncoastal cities, emphasizing the importance of considering meteorological factors and pollutant sources in air quality prediction and management. These findings can contribute to the development of more precise air quality prediction models and aid in informing air quality management strategies in coastal and non-coastal cities.
-
Pollutants Detail
Fig.3. Yearly Pollutants Analysis
From 2017 to 2018, BTX levels nearly tripled, while BTX, CO, and SO2 exhibited similar yearly patterns. Notably, pollutants averaged lower in 2020, likely due to the COVID-
19 pandemic's impact on reducing industrial and transportation emissions. O3 levels remained relatively stable across all years. These trends suggest specific factors driving pollutant variations, such as increased industrial activities and canges in transportation. The parallel patterns of BTX, CO, and SO2 imply shared sources or conditions influencing their levels. Analyzing these trends can inform policymakers on addressing air quality issues, emphasizing the significant impact of human activities on pollution levels and the potential for improvement through targeted interventions.
-
Analysis of PM 2.5 and PM 10: Exploring Particulate Matter Pollution
Fig.4. Analysis of PM 2.5 and PM10 Levels Across Various Cities
We noted that PM2.5 and PM10 levels are seasonal for most of the cities and are higher during the winter months. This is consistent with previous knowledge, as particulate matter levels can be influenced by meteorological conditions, such as temperature and humidity, as well as human activities like heating and transportation. During the winter months, colder temperatures can lead to increased emissions from heating sources, such as wood stoves and coal-fired power plants, which can contribute to higher PM2.5 and PM10 levels.
-
Analyzing So2 levels: Understanding Air Quality Dynamics
The "City-wise Performance of SO2 Levels" graph provides a succinct overview of SO2 concentrations across several urban centers. From Aizawl to Gurugram, each city's performance is visually represented, offering insights into their respective air quality statuses. This analysis aids in identifying cities with elevated SO2 levels, highlighting areas for targeted intervention and environmental management strategies.
Fig.5. City-wise Performance of SO2 Levels
-
City wise Pollutants Concentration Analysis
High City-wise Pollutant Concentrations: Ahmedabad, Patna, Delhi, and Gurugram consistently exhibit elevated levels of pollutants. Ahmedabad tops in CO2, SO2, and CO, possibly due to industrial activities. Delhi and Gurugram register high
particulate matter, likely stemming from various sources such as transportation and industrial emissions. These findings underscore the need for targeted interventions, including emissions reduction measures and enhanced monitoring, to combat air pollution in these cities
Fig.6. Pollutant Concentration Variations Across Cities
-
Compraing AQI : Coastal vs. Non Coastal Cities
In our comparative analysis of air quality prediction for some coastal and non-coastal cities, we observed that Ahmedabad, Delhi, Gurugram, Lucknow, and Patna consistently fall into the Severe, Poor, and Very Poor AQI quality categories, while Bengaluru, Amravati, Hyderabad, and Thiruvananthapuram generally experience Good and Satisfactory air quality levels. This contrasting pattern can be attributed to various factors, including geographical location, meteorological conditions, and urban development. for instance, Ahmedabad, Delhi, Gurugram, Lucknow, and Patna are located in areas with high levels of industrial and vehicular emissions, which can lead to poor air quality. In contrast, Bengaluru, Amravati, Hyderabad, and Thiruvananthapuram are generally located in regions with more favorable meteorological conditions and have
implemented stricter emission control measures, contributing to their better air quality. Our findings emphasize the importance of considering the unique characteristics of each city when assessing air quality and developing strategies to improve it. By understanding the specific factors that influence air quality in coastal and non-coastal cities, policymakers and urban planners can make informed decisions to promote healthy air quality and protect public health.
Fig.7. Comparison Between Coastal and Non-coastal City
-
Inference Table : Insights and Results
To differentiate between coastal and non-coastal cities based on the Air Quality Index (AQI) values provided for the cities mentioned, we can create a table. The coastal cities will typically have different air quality due to their proximity to the sea compared to non-coastal cities. Here is a table differentiating between coastal and noncoastal cities based on the provided AQI values.
Table 1: Non Industrial Coastal Cities: AQI Comparison
Cities
Air Quality Index
Coastal or Non Coastal
Industrial or Non Industrial
Joraphokhar
15
Coastal
Non Industrial
Aizawl
37
Coastal
Non Industrial
Amravati
69
Coastal
Non Industrial
Ernakulum
83
Coastal
Non Industrial
Kochi
85
Coastal
Non Industrial
Table 2: Industrial Coastal Cities: AQI Comparison
Cities
Air Quality Index
Coastal or Non Coastal
Industrial or Non Industrial
Chennai
69
Coastal
Industrial
Coimbatore
93
Coastal
Industrial
Guwahati
118
Coastal
Industrial
Kolkata
126
Coastal
Industrial
Table 3: Non Industrial Non Coastal: AQI Comparison
Cities
Air Quality Index
Coastal or Non Coastal
Industrial or Non Industrial
Amritsar
98
Non Coastal
Non Industrial
Chandigarh
128
Non Coastal
Non Industrial
Table 4: Industrial Non-Coastal Cities: AQI Comparison
Cities
Air Quality Index
Coastal or Non Coastal
Industrial or Non Industrial
Brajrajnagar
107
Non Coastal
Industrial
Hyderabad
108
Non Coastal
Industrial
Bhopal
134
Non Coastal
Industrial
Bengaluru
136
Non Coastal
Industrial
Ahmedabad
151
Non Coastal
Industrial
Delhi
152
Non Coastal
Industrial
Jaipur
165
Non Coastal
Industrial
Gurgram
175
Non Coastal
Industrial
The analysis of the provided data reveals distinct patterns in air quality across different types of cities. Firstly, coastal cities with non-industrial areas exhibit remarkably low Air Quality Index (AQI) levels, with Joraphokhar boasting the lowest AQI of 15 among all cities. This suggests that coastal regions with minimal industrial activity maintain relatively clean air environments. Conversely, coastal cities with industrial presence, such as Chennai and Guwahati, demonstrate higher AQI levels due to pollution from industrial processes, despite proximity to rivers like Cooum and Brahmaputra, respectively. Secondly, non-coastal cities without industrial sectors generally display moderate AQI levels. Notably, cities like Amritsar and Chandigarh showcase AQI values below 130, indicating moderately clean air despite their noncoastal locations. The absence of significant industrial activity contributes to relatively better air quality, even in the absence of nearby rivers. Conversely, non-coastal cities with industrial sectors, such as Hyderabad and Delhi, exhibit higher AQI levels exceeding 130. These cities face challenges associated with industrial pollution, evident from their proximity to rivers like Musi and Yamuna. Industrial activities significantly impact air quality in these regions, highlighting the need for stringent pollution control measures to mitigate environmental health risks.
-
AQI Bracket Comparison: Assessing Air Quality Across Cities
Fig.8. City wise AQI Bracket Comparison
In this study comparing the air quality index (AQI) of coastal and non-coastal cities, you can analyze the AQI brackets for each city to comprehend the air quality levels. The AQI ranges from 0 to 500, with 0 being the best air quality and 500 being the worst. The AQI values are categorized into six levels, each with a specific color to represent the health concerns associated with the air quality.
Good (0-50): This level indicates that air quality is satisfactory, and air pollution poses little or no risk.
Satisfactory (51-100): Air quality is acceptable, but there may be a moderate concern for people with lung disease, older adults, and children.
Moderately polluted (101-200): Air quality is considered unhealthy for sensitive groups, such as children, older adults, and people with respiratory or heart disease.
Poor (201-300): Air quality is unhealthy for everyone, and everyone should limit prolonged outdoor activities.
Very poor (301-400): Air quality is hazardous, and everyone should avoid prolonged outdoor activities or reduce strenuous activities.
Severe (401-500): Air quality is hazardous, and everyone should avoid all outdoor activities.
By comparing the AQI brackets for the 17 coastal and non- coastal cities, you can identify which cities have better air quality and which ones have poorer air quality. This comparison will help you understand the differences in air quality between coastal and non-coastal cities and provide insights into the factors that contribute to these differences.
-
Lock Down Impact on Major Cities: Evaluating Effacts The lock down measures implemented in cities worldwide have had a substantial impact on air quality, as reflected in the decrease in Air Quality Index (AQI) values. The reduction in industrial and transportation activities led to a decline in pollutants such as particulate matter (PM2.5 and PM10), carbon monoxide (CO), nitrogen dioxide (NO2), sulfur dioxide (SO2), and ammonia (NH3). This decrease in pollutants resulted in improved air quality, with some cities experiencing reductions of over 30% in PM2.5, PM10, and CO, and up to 52% in NO2 levels. The strictness of lockdown policies directly influenced the extent of reduction in NO2 levels, with cities like Wuhan and Delhi showing the highest decreases due to stringent measures. Additionally, the lockdowns had varying effects on secondary pollutants, with ozone (O3) levels increasing in most cities due to photochemical reactions, while PM2.5 and PM10 decreased in the majority of cities. The overall decrease in AQI values during the lockdown period indicates a significant improvement in air quality, highlighting the importance of reducing emissions from industrial and transportation sources to enhance urban air quality.
Fig.9. Effect on Lock down for AQI on Major Industrial Cities
ACKNOWLEDGMENT
In conclusion, the comparative analysis of air quality prediction for some coastal and non-coastal cities revealed that coastal cities generally have better air quality, as indicated by lower AQI values, compared to non-coastal cities. This can be attributed to factors such as geographical location, meteorological conditions, and urban development. Coastal cities, such as Amravati, Bengaluru, Hyderabad, and Thiruvananthapuram, generally experience Good and Satisfactory air quality levels, while non-coastal cities, such as Ahmadabad, Delhi, Gurugram, Lucknow, and Patna, consistently fall into the Severe, Poor, and Very Poor AQI quality categories. This contrasting pattern can be attributed to various factors, including the presence of major industrial and transportation activities, meteorological conditions, and urban planning. It is important to note that the AQI levels can be influenced by various factors, including meteorological conditions, emissions sources, and atmospheric chemistry. Therefore, understanding the specific factors contributing to the AQI levels in each city is crucial for developing effective strategies to improve air quality and protect public health. In summary, the findings of this study highlight the importance of considering the unique characteristics of each city when assessing air quality and developing strategies to improve it. By understanding the specific factors that influence air quality in coastal and non-coastal cities, policymakers and urban planners can make informed decisions to promote healthy air quality and protect public health.
REFERENCES
-
Gokulan Ravindiran, Gasim Hyder, Avinash Algumulai, Air quality prediction by machine learning models: A predictive study on the indian coastal city of Visakhapatnam Chemosphere, vol. 338, 2023.
-
Health Effects Institute. State of Global Air 2019, Special Report. Boston, MA: Health Effects Institute, 2019.
-
Press Information Bureau, National Air Quality Index (AQI) launched by the Environment Minister AQI is a huge initiative under Swachh Bharat, pib.gov.in, Oct. 17, 2014.
-
Central Pollution Control Board,National Air Quality Index.Ministry
of Environment, Forest, and Climate Change, India, 2014.
-
Y.C Liang, Y. Maimury, A.H.L. Chen, J.R.C Juarez,Machine
learning-based prediction of air quality. App.Sci.,vol. 10; 2020.
-
M,chand Sharma, Samyak Jain, and Tariq Sheakh,Forecasting and prediction of air pollutant concentrates using machine learning techniques: the case of India. IOP Conference Series Material Science and Eng., vol.1022; 2021
-
Samayan Bhattacharya, Sk Shahnawaz,Using machine learning to predict air quality index in new delhi, 2022.
-
K.kumar, B.P Pnde Air pollution prediction with machine learning:
a case study of Indian cities. Inter. J.Envr.Sci.Tech, 2021.
-
A. Plaia and M. Ruggieri, Air quality indices: a review, Reviews in
Environmental Science and Bio/Technology
-
M. Somvanshi, P. Chavan, S. Tambade, and S. Shinde, A review of machine learning techniques using decision tree and support vector machine, in international conference on computing communication control and automation (ICCUBEA). IEEE, 2016.