
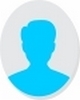
- Open Access
- Authors : Amandeep Sharma, Ashwini Kalyanasundaram, Prince Kumar, Shashank Dhamija
- Paper ID : IJERTV12IS080029
- Volume & Issue : Volume 12, Issue 08 (August 2023)
- Published (First Online): 31-08-2023
- ISSN (Online) : 2278-0181
- Publisher Name : IJERT
- License:
This work is licensed under a Creative Commons Attribution 4.0 International License
A Comprehensive Review on Prognostics Methodologies for State of Charge Estimation of Lithium-Ion Batteries in Electric and Hybrid Electric Vehicles
Amandeep Sharma
Team Lead, AGBG Industry, Accenture, India Ashwini Kalyanasundaram
Senior Manager, AGBG Industry, Accenture, India Prince Kumar
Application Development Associate Manager, AGBG Industry, Accenture, India Shashank Dhamija
Application Development Associate Manager, AGBG Industry, Accenture, India
Abstract
Sustainable development regards the improvement in future generation life by transitioning towards a fossil fuel free future. The extensive usage of fossil fuels has large impact on catastrophic climate variations and many other aspects of life. Also, the extensive usage of petrol, diesel and other fossil fuels cause a rapid decrease of global reserves. Due to promis- ing solutions in terms of sustainability, reduced Co2 emission and other environmental global issues, electric vehicles are gaining massive popularity in the automotive industries. Further, lithium-ion batteries are widely accepted in EVs because of their high energy density, fast charging/ slow discharging, low weight and satisfactory life cycle. In this paper, a com- prehensive review on state of charge (SoC) estimation for lithium-ion batteries is proposed which can lead towards reliable and safe operation of electric vehicles. The classification of different SoC estimation techniques, methodology used, estimation accuracy and drawbacks are discussed in detail. During review, it has been observed that SoC estimation analyze the charging and discharging cycles of battery and avoid the overcharging/discharging conditions of the battery. All the findings and insights of the presented review will lead to the advanced SoC estimation techniques for lithium-ion batteries for future electric vehicle applications.
Keywords: Lithium-Ion Batteries, State of Charge (SOC) estimation, Battery Management System, Battery Modeling
-
INTRODUCTION
Extensive usage of petrol, diesel in the transportation sector have led the world to some serious consequences including greenhouse gas emission and global warming. Also, increas- ing cost of crude oil set a bottleneck for the automotive industry. World emission regulatory agencies are more concerned about fossil fuel dependency and carbon impressions. As per International Energy Agency (IEA)-2022 transportation sector contributed 37% Co2 emis- sions. These issues have placed an urge to develop future generation vehicles with alternative fuel resources. In this context, Electric vehicles have proved to become promising alternative vehicles which are powered by rechargeable battery cells. Various battery technologies are used in EVs including nickel metal hybrid (NiMH) batteries, nickel cadmium (Nicd), lead acid and lithium-ion batteries. Among them, lithium-ion batteries are widely accepted and fastest growing storage technology due to its promising features.
However, despite of all positive features, lithium-ion batteries are highly dynamic and nonlinear in nature and its performance get affected by aging cycles, material degrada- tion, charging/discharging current and operating temperature variations. Thus, the state of charge (SoC) of lithium-ion batteries is one of the important evaluation parameters that confirms safe operation of electric vehicles. An accurate SoC estimation leads to extended battery life cycle, prevent battery failure by providing the information about driving range or remaining useful power in the battery. The main contribution of this review article is to classify different State of Charge (SoC) estimation methods for different materialistic com- positions of Lithium-ion batteries. This paper proposed a systematic review of published articles in literature to extract information of different SoC estimation methods in order to find out most accurate method with respect to battery material composition. Benefits/ drawbacks and challenges of implementing various SoC estimation methods are addressed that will be important for vehicle manufacturers.
The rest of the paper is structures as follows: Section 2 presents the basic concept of SoC in EVs. Section 3 explains the basic battery modeling methods for SoC estimation in EVs. Section 4 provides a detailed classified review of SoC estimation methods for different Lithium-Ion compositions. Section delivers some observations and suggestions that will be helpful in upgrading existing SoC methods and for future innovations of implementing new SoC techniques. Finally, section 5 summarizes conclusion and selective suggestions which have been coming out from the proposed literature review.
-
Concept of SoC for EVs
State of Charge (SoC) is not a physical quantity and can be estimated by measuring correlated battery parameters including voltage, current and temperature as shown in figure
-
The figure 1 shows the basic functions of a battery management system to ensure optimal and safe usage of battery charge and acquiring information about battery state for vehicle control.
a.kalyanasundaram@accenture.com (Ashwini Kalyanasundaram), prince.a.kumar@accenture.com (Prince Kumar), shashank.dhamija@accenture.com (Shashank Dhamija)
Fig. 1. Basic functions of Battery Management System in EVs [1]
Mathematically, SoC of a battery is defined as the ratio of remaining charge QRemaining to the actual amount of charge (as per battery specifications) QActual of the battery; generally expressed in percentage and given as:
SoC(t) = (QRemaining/QActual) 100%
(1)
The QActual parameter is the actual battery charge that is available at the initial charge/ discharge cycle and depends upon discharge current rate and State of Health (SoH). QRemaining is the maximum charge that can be used from the battery after a specific period of time. To consider the coulombic efficiency, the above equation can be modified as
where Soc(0) is the initial State of Charge value, I(t) is the charging and discharging battery current, i is the coulombic efficiency and defined as ratio of discharged electrons to charged electrons in one cycle. Its range lies between 0.9 to 1.
Table 1: Abbreviations
Definition
Notation
Definition
Notation
Open Circuit Voltage
VOC
Extended Kalman Filter
EKF
Back Propagation Neural Networks
BPNN
Dynamic Stress Test
DST
Nickel-Manganese-Cobalt
NMC
First and Second Derivative of Volt-
age
Vd1, Vd2
Transient Voltage Loss and Ohmic
Voltage Loss
LV T , LV O
Nickel Cobalt Aluminum
NCA
Long Short-Term Memory
LSTM
LevenbergMarquardt Algorithm
LM algo-
rithm
Highway Fuel Economy Test
HFET
Urban Dynamometer Driving
Schedule
UDDS
Unified Cycle Driving Schedule
LA92
Supplemental Federal Test Proce-
dure
US06
Beijing Dynamic Stress Test
BJDST
Federal Urban Drive Schedule
FUDS
Constant Discharge Test
CDT
Hybrid Pulse Power Characeriza-
tion
HPPC
-
Battery Modeling Methods
Various battery models have been developed over the past years with different accuracy levels and computational cost. The estimation accuracy of battery life cycle and associ- ated simulation results are dependent upon the effectiveness of the battery model. In the literature, these models have been classified in three broad categories including equiva- lent electrical circuit model [2] [3], physics based electrochemical models [4] and artificial intelligence approach-based data driven approach [5][6].
-
Electrical Equivalent Circuit Model
Equivalent circuit model of the battery has been comprised of basic circuit elements including resistor, capacitor and voltage source and used to trace the dynamic behavior of the battery. State space equations of the model have been used to analyze battery management systems and EV based modeling simulations. Different types of equivalent circuit models have been discussed in literature including Rint model [7], Thevenins model [8], RC model and PNGV (partnership for a new generation of vehicle) model [9]. Their circuit structures have been depicted in figure 2.
The Rint model [10] is simplest practical implementation of Li-ion batteries where output voltage UL is equal to the sum of open circuit voltage Uocv and internal circuit resistance
R. IL is the output load current. Battery SoC and SoH are dependent upon on these mentioned parameters but the model is not accurate for practical implementation as it leads to uncertainties in the state estimation.
UL = Uocv ILR (3)
The Thevenins model is an extension to Rint model with the introduction of parallel RC network in the circuit. The internal circuit resistance is the sum of ohmic resistance R and polarization Thevenin resistance RP. The transient response of the circuit is depicted by CP . The output voltage UL is given as:
Fig. 2. Different types of battery equivalent circuit models
The RC model is well suited for the dynamic voltage behavior of the battery. The output voltage of the circuit can be calculated by the given equation:
The PNGV model is an extension of Thevenins model by introducing a capacitor in series with the voltage source. The problem of accumulation and saturation of load current is solved by PNGV model. The output voltage is calculated as:
UL = Upp Upc Upb Upo
(8) Based on the available literature survey, PNGV model offers acceptable dynamic perfor- mance and less operational losses among all the discussed models.
-
Physics Based Electrochemical Model for SoC Estimation
Since lithium-ion batteries are electrochemical in nature, their electrochemical states can be examined to determine their real time state. Solid electrochemical particles and amount of lithium concentration in them determine the capacity and output voltage of the battery. The same parameter can be used to determine electrode SoC which can be calculated by the following mathematical equation [11]:
where L is the electrode thickness and R is the radius of the particle. Cs,max signifies the maximum concentration in the solid phase and Cs(x, r, t) is the solid phase concentration. Electrochemical model is useful to estimate battery degradation level which is mainly due to over-potential in the battery that cause unwanted side reactions, for e.g., lithium plating. If over-potential can be estimated, side reactions in the battery can be controlled.
-
Artificial Intelligence Based Data Driven Approach
Artificial Intelligence based data driven approach is a model free and flexible methodology for SoC prediction. Available correlated features or parameters during battery operational cycles can be used to estimate SoC of battery. For instance, artificial neural networks [12], fuzzy logic [13], support vector machine [14], Radial basis function [15], Gaussian process regression [16] and many more data driven algorithms can be utilized for battery health monitoring. These techniques first build battery degradation state space model followed by particle filter or Kalman filter to estimate SoC or remaining useful life of battery.
-
-
Review of Existing SoC Estimation Techniques
-
Lithium Iron Phosphate Battery (LiFePO4)
In Lithium Iron Phosphate Batteries [17], lithium iron phosphate act as the cathode material and graphitic carbon electrode work as anode with a metallic backing. Some distinctive features about this battery are stable and constant output voltage with high charge cycle, non-explosive and less heating and better power density. Some shortcomings of Lithium Iron Phosphate Battery are low operational performance at low temperature, high self- discharging rate, low nominal voltage and high manufacturing cost.
Table 2: Specifications of Lithium Iron Phosphate Battery
Cell level specifications
Lithium Iron Phosphate Battery
Nominal voltages(v/cell)
3.20V – 3.30V
Working voltage(v/cell)
3.0-3.20V
Maximum Charge Voltage
3.65V
Energy density
90-160Wh/kg
Cycle life(1C)
2000
Working temperature range
-20 – 75 C
-
Techniques for SOC Prediction
-
Artificial Neural Networks (ANN) and Machine Learning (ML) Based Approach
Anton et al. [18] proposed Support Vector Regression (SVR) based approach for SOC calculations of lithium iron manganese Phosphate battery cell. Model parameters have been extracted from charging and discharging cycles of 60 AH battery cell under dynamic stress test cycle. Results offer RMSE value of 0.71% and maximum error value less than 6%. Wang et al. [19] proposed Extreme Learning Machine (ELM) based SOC estimation framework for 180Ah/3.2v battery at 250c. The estimation accuracy is comparable with back propagation based neural networks and SVM with less number of training parameters and less computational complexity. Results shows that training time is 4% less in ELM as compared to BPNN with high accuracy. Dang et al. [20] presented the concept of dual neural networks that adopt open circuit voltage concept to predict state of charge information with fusion battery model. The proposed fusion battery model is comprised of serially connected two neural networks. First order or second order electrochemical battery parameters are identified by the first linear neural networks and relationship among these parameters are analyzed by applying dynamic stress test data on back propagation based second part of neural networks. Results shows that for Thevenins theory-based battery model, the maximum error in state of charge prediction is 4.82% and is reduced to 0.75% with second order battery model. It has been observed from the results that accuracy in state of charge estimation can be further improved by using higher order battery model.
Sheng et al. [21] presented Gaussian Process Regression (GPR) based SOC estima- tion. Revolutionary expectation maximum method has been used for the selection of optimum number of Gaussian processes. Feature selection has been performed with nonlinear correlation method. Chaoui et al. [22] proposed a input time delayed neural network based approach for State of Health (SOH) and State of Charge (SOC) prediction of lithium Iron Phosphate Batteries (LiFePO4). The proposed technique considers the output from three sensors including voltage, current and ambient temperature of the battery irrespective of other battery parameters. Time delayed neural networks are based on back propagation learning algorithm for analyzing the battery dynamics. For the training and testing of NN, four variations in data sets has been proposed. The first data set has been extracted from a new attery and depicted as 0h. The second, third and fourth data set has been taken with respect to the usage of the battery and it includes 352h, 544h and 650h respectively. It has been depicted in the results that the proposed method is capable to handle nonlinearity between battery voltage, cur- rent, surrounding temperature and its SOC more accurately as compared to traditional Multilayer Perceptron (MLP).
Chen et al. [23] worked to estimate the non-linearities in the Li-ion battery. The author designed an equivalent battery model and apply Radial Basis Function (RBF) based neural network to predict the SOC. The performance of the proposed model has been compared with Kalman filter. It has been observed from the results that the proposed approach offers high convergence speed and more precise SOC estimation. Guo et al. [24] proposed three-layer back propagation neural network for SOC estimation. Simulations has been performed on MATLAB platform with voltage, current, temperature and internal resistance as the inputs.
Wei et al. [25] introduced Long Short-Term Memory (LSTM) based exogenous in- put neural network for SOC predictions. The proposed hybrid model resolves the issue of gradient disappearance and gradient explosion by establishing the jump ahead connection which leads to shorter propagation path for gradient information. The performance analysis of proposed model has been carried out against PSO based back propagation neural networks, standard LSTM and least square support vector ma- chine under dynamic stress test and urban dynamometer driving schedule. The out- put results validate the satisfactory estimation results from the proposed hybrid model. Tian et al. [26] combined the positive attributes of Adaptive Cubature Kalman Filter (ACKF) and LSTM. To capture the nonlinear relationship among the measured bat- tery parameters and SOC, LSTM approach has been utilized. ACKF as a subsequent stage, smooth out the LSTM network output by adaptively updating the noise co- variance matrices. To validate the generalization ability of the proposed model, DST data set has been used for model training, US06 and FUDS cycles have been used for testing purpose. Results shows that model offer RMSE value less than 2.2% and maximum value up to 4.4%. Wang et al. [27] proposed back propagation based neural network for SOC estimation which has been optimized using artificial fish swarm optimization algorithm. Performance of the proposed model has been compared with EKF algorithm and concluded that the proposed approach is more cost effective and realistic then EKF approach.
-
Fuzzy Logic
Esfandyari [28] introduced fuzzy logic and predictive theory-based hybrid model to precisely estimate the state of power for series connected Li-ion battery cells. Initially, the power level of a single cell has been calculated by predictive control procedure. In the next step, difference between state of charge and concurrent aging state has been calculated by fuzzy logic-based model free control system. The proposed framework eliminates the need of online charging/discharging curves of individual cells and need only current value of cell voltage and current and can be
calculated in offline mode.
-
Filter-Based Approach/Hybrid Approach
The main issue with data driven approaches is the dependency on the available data. The biased data or incomplete data may lead to incorrect SOC estimations. To com- pensate this issue, hybrid model approach can be implemented to design an optimized Battery Management System (BMS). Several stochastic filtering approaches that can be classified in hybrid modelling have been reviewed in the following section. Gener- ally, filter-basedmethods have been classified as Gaussian process-based filtering and probability-based filtering.
Di et al. [29] presented EKF based SOC estimation approach. The proposed framework is based on electrochemical model of lithium-ion batteries. The performance of the proposed technique has been validated using HPPC profile and results in restricting maximum SOC estimation error below 3%. Chen et al. [30] proposed an extended Kalman filter based SOC estimation using nonlinear battery model. The nonlinear battery model has been designed using second order RC circuit model and open circuit voltage. Experiments have been conducted under four different conditions including known initial SOC values, unknown initial SOC values, in the presence of current noise and with limited battery parameters. Results validate the effectiveness of the proposed model. Mastali et al. [31] proposed Kalman filtering approach for SOC estimation of LiFePO4 batteries. The proposed approach is applicable to both prismatic and cylindrical cells. The extended Kalman filter has been used in zero state hysteresis model and dual extended Kalman filter has been used in varying parameter hysteresis model for SOC estimation. In dual approach, battery SOC and model parameters can be estimated simultaneously. Results shows that a maximum error of 4% has been observed in estimating the state of charge of the battery. Yu et al. [32] proposed Kalman filter based SOC estimation of Li-ion batteries using open circuit voltage as the only parameter to depict the dynamic behavior of the battery. The concept of zero axial straight line has been used to trace the relationship between SOC and open circuit voltage. The slope of the line changes with respect to the change in the SOC. Second order equivalent battery model has been used to simulate the partially and fully charged battery behavior. Results shows an error of less than .5% during the experiments.
Dong et al. [33] developed an equivalent linearized circuit model to depict the dynamic behaviour of the battery while considering open circuit voltage as linearized function for SOC. The estimation of SOC and State of Function (SOF) has been carried out by Kalman filter estimation method and analyzed under different temperatures and currents. The results indicate RMSE under four different experiments as 1.05% (open circuit voltage test), 4.31% (constant power test), 3.51% (maximum discharge capability test) and 1.22% (dynamic current test). Deng et al. [34] utilized dual adaptive extended Kalman filter together with first order RC model for SOC estimation. The first filter used to identify the battery parameters and second one is used to estimate the SOC. To improve the estimation accuracy, ampere-hour counting method has been employed for estimation where the relationship between OCV and SOC is highly nonlinear. For online SOC prediction, least square support vector machine has been implemented that will consider the effect of degradation and temperature while inputting relevant feature vectors. The proposed model has been analyzed using Hybrid pulse test and UDDS cycle test. The tests have been conducted at 120c, 0c, 250c and 520c and observed error is 6.37%, 4.44%, 4.40% and 4.04% for hybrid pulse test and 5.88%, 4.31%, 4.06% and 4.64% for UDDS cycle.
Zhao et al.[35] implemented SOC estimation framework using EKF and central dif- ference Kalman filter. Accuracy of the proposed models is evaluated using charge- discharge current and fast charging current. Two cases have been analyzed for per- formance measures including difference in the actual terminal voltage and estimated value of terminal voltage and second is the truncation error. In both cases central difference Kalman filter perform better than EKF.
Li ei al. [36] presented a methodology to calculate open circuit voltage, resistance and capacitance of Thevenins eqivalent circuit model of the battery. A capacity estimation algorithm has been introduced to estimate the capacity loss during the working cycle of the battery. Furthermore, extended Kalman filter has been used for SOC estimation while circumventing the effect of noise. MATLAB platform has been used to simulate the battery model. Wu et al. [37] consider the effect of temperature on model parameters. He proposed a battery model which is suitable to work at low temerature conditions and have slow discharging rate. Extended Kalman filter approach has been used for SOC estimation at different temperatures. The results shows that the average relative error is less than 1% for estimating battery terminal voltage and SOC prediction error is 2%. Kim et al. [38] proposed an integrated framework of reinforcement learning and extended Kalman filter for SOC estimation of lithium-ion batteries. EKF with RC equivalent circuit work as an iterative algorithm where we can define actions and reinforcement learning algorithm is used to optimize the EKF parameters. Optimization of rules for adjusting EKF parameters is
with respect to the battery characteristics that includes the usage characteristics of the battery, behavioral difference in real time battery and battery model and error in initial SOC estimation. Zheng et al. [39] proposed a dual Kalman filter algorithm for SOC estimation and to compensate the gaps of EKF algorithm and ampere hour integration algorithm. For this, the proposed approach implements linear Kalman fusion with SOC estimations from these two traditional methods. The results shows that maximum error has been restricted to less than 2% with the proposed technique with high convergence speed.
Dong et al. [40] presented a Sequential Monte Carlo Filter technique in association with Auto Regressive Exogenous Modelling (ARXM) approach for SOC estimation. An ARXM approach is used to trace the transient behaviour of the battery with the consideration of temperature variations and model order. Monte Karlo filtering approach using nonlinear comprehensive has been used for SOC estimation. Author also proposed Numerical Subspace State Space System Identification method for real time monitoring of battery parameters including voltages and currents.
Liu et al. [41] particle filter algorithm-based SOC estimation approach in which re- cursive estimation formula has been deduced using sequential importance resampling approach. To further improve the model accuracy, particle filter algorithm is revised
by optimizing the density function. MATLAB based simulation results shows that the proposed method reduced the RMSE value to 0.0163. Singh et al. [42] designed an equivalent battery model with three RC pairs connected in series with their internal resistance. The parameter values of the model have been calculated by considering the charging and discharging rates of the battery and optimization of these parameters has been done with Isqnonlin function. An integrated method of open circuit voltage method and coulomb counting method has been for soc estimation. The accuracy of the proposed framework has been further improved by using an Adaptive Neuro Fuzzy Inference System (ANFIS) algorithm. Simulation results shows that the estimations from the proposed model are very close to practical battery data and hence suitable for real time systems. Li et al. [43] focuses on the double layer electrochemical modelling of the li-ion batteries by considering electrolyte liquid phase and electrode solid phase. Based on the structural characteristics of the double layer model, parameter identification has been done with genetic algorithm. SOC estimation has been carried out by EKF and verified by NEDC and 1C pulse discharge cycle. Maximum error has been reported as 2.62% with 1C pulse discharge and 2.56% with NEDC.
Yang et al. [44] proposed LSTM based RNN model for describing the behaviour of the battery under different temperature ranges and to estimate the battery SOC with voltage, current and temperature as input variables. To further improve the estimation accuracy, unscented Kalman filter has been implemented to filter out the noise. Evaluation of the proposed model has been performed under DST, FUDS and US06 drive cycles under the temperature range from 00c to 500c. Results shows that RMSE has been restricted to less than 1.1% and mean average error is less than 1%. Nguyen et al. [45] presented an integrated framework for SOC estimation using particle filter and unscented Kalman filter. Equivalent circuit of the battery is modelled by second order ARXM approach and parameter identification has been performed with recursive least square identification approach. Observed RMSE is 0.76% in proposed framework as compared to unscented Kalman filter (1.85%) and adaptive unscented Kalman filter (1.01%). Sun et al. [46] presented an adaptive intelligent extended Kalman filter to detect the change in fixed length error innovation sequenceusing themaximum likelihood function. Based on the observed changes, innovation covariance matrix has been updated which further improves the SOC estimation accuracy. Results shows that in comparison with adaptive extended Kalman filter, the RMSE and mean absolute error have been decreased by 43.34% and 55.80% respectively. In addition to this, the computation time has been increased by a factor of 4.59%.
12
Table 3: Summary of SOC estimation techniques for Lithium Iron Phosphate Battery(LiFePO4) (Cont.)
Author
Battery
Ca- pac- ity
Temperature
Range
Methodology
Models Training and valida-
tion
Error Rate
Dang
[20]et
al.
20Ah
Serially c o n n e c t e d d u a l neural networks together with Thevenins theory based battery model
Dynamic stress data for training
BP neural networks
Observed error is 0.75% with sec-
ond order battery model
Chaoui et al. [22]
20Ah
100c, 250c, 400c
Multilayer perceptron
Back propagation-based time- delayed neural network w.r.t. us- age of the battery
Observed RMSE is 1.9 103,
3.2 103,3.3 103, 2.7 103
for 0h, 352h, 544h and 650h us-
age respectively
Chen [23]
et
al.
36Ah
200c
Neural networks linear observer
based
non-
RBF based neural network on FUDS drive cycle
Observed RMSE is 2.23% for 20 consecutive FUDS cycles
Wei et al. [25]
20Ah
200c
LSTM based exogenous i/p neu- ral network with nonlinear auto regressive approach
Training over UDDS and DST drive cycles
Observed RMSE is 0.76% for UDDS and 0.78% for DST cycles
Burgos et al.
[47]185Ah
–
EKF with fuzzy logic for deriving
state transition equation
Trained and validated by 4 sets
of designed experimental sys- tem for fuzzy model, Thevenins model, plett and Copetti models
Observed RMSE is minimum for
fuzzy rule- based system among all the systems
Mastali et al. [31]
20
Ah
250c
Kalman filtering approach
Validated on experimental test bench
Observed maximum error is 4%
Yu et al. [32]
280
mAh
250c
Standard Kalman filter
Validated on experimental test bench
Observed maximum error is 0.5%
Dong [33]
et
al.
9.5/10/
and 12.5/13 Ah
10 450c(Chg)
and 20 600c(Dis)
Kalman filter method
validated using open circuit volt- age test, constant power test, dy- namic current test and maximum
discharge capability test
Observed RMSE under four ex- perimetral tests are 1.5%, 4.31%,
3.51% and 1.22% respectively
Deng et al.[34]
10
Ah
120c, 0c, 250c and 520c
Adaptive extended Kalman fil- ter with ampere-hour counting method Least square support vector machine
Validated under Hybrid pulse test and UDDS cycle test
observed RMSE is 6.37%, 4.44%,
4.40% and 4.04% for hybrid pulse
test and 5.88%, 4.31%, 4.06%
and 4.64% for UDDS cycle
Li ei al. [36]
40
AH
–
Extended Kalman filter
Matlab simulations
Observed maximum error is 3.8%
after the consideration of capac- ity loss
to be contd on next page
Table 3: Summary of SOC estimation techniques for Lithium Iron Phosphate Battery(LiFePO4)
Dong
[40]et al.
9.5Ah
and 12.5Ah
100c, 250c,
450c,5 600c
Monte Karlo Filtering
approch with ARXM
Tested and verified against dif-
ferent current discharging con- diti and operating tempera- tures
Yang
[44]et al.
1.1Ah
00c,100c,
200c, 300c,
400c,500c
LSTM with RNN and
Unscented Kalman filter
trained and validated
DST, FUDS and US06
Nguyen et al.
[45]10
Ah
0 450c
EKF, Particle filter, ARXM and
Recursive least square identifica- tion approach
Trained a n d
validated UDDS drive cycle
Liu et al. [41]
2.3
Ah
–
Auxiliary Particle Filter
MATLAB based simulation
3
2.1002Ah
250c
Adaptive intelligent
extended Kalman filter, Genetic algorithm
MATLAB based simulation
Li et al. [43]
2.5
Ah
250C
Double layer electrochemical
model, Genetic algorithm, EKF
Verified by NEDC and 1C
pulse discharge cycle
o
Sun et al. [46]
-
-
-
Lithium-ion Polymer Battery (LiPB)
Lithium-ion Polymer Batteries are composed of rectangular and cylindrical shaped struc- ture and fabricated using solid form of polymer electrolyte. Polyethylene oxide or polyacry- lonitrile are the examples of polymer electrolyte which are used as a plastic like sheet which leads to ion exchange but no electricity conduction. Due to this feature, these batteries are flexible and can be designed in different economical shapes. Other positive attributes are light weight, low risk of overcharging and electrolyte leakage. High manufacturing cost, low energy density, shorter life cycle and sensitive to explosions.
Table 4: Specifications of Lithium-ion Polymer Battery
Cell level specifications
Lithium-ion Polymer Battery
Capacity
Typ. 1000 mAh
Nominal voltage(v/cell)
3.7V
Maximum Charge Voltage
4.2V
Energy density
100 to 158 Wh/Kg
Cycle life(1C)
500
Working temperature range
charging 0 45C discharging -10-60C
-
Techniques for SOC Prediction
-
Artificial Neural Networks (ANN) and Machine Learning (ML)
Sun et al. [48] addresses model complexity with respect to the prediction accuracy and presented a method to estimate the required model order and associated param- eter identification. An equivalent circuit model of twelve series connected LiPB cells has been used to evaluate the proposed framework. The author implemented Radial Basis Function (RBF) based neural network to model the bias function and to estimate terminal voltage of each battery cell. Finally, adaptive extended Kalman filter based framework for state of charge estimation of serially connected multi cell battery pack with bias correction techniques has been proposed. The results validate that the bias correction techniques can lead to extended battery model with less computation cost. Results shows that maximum absolute error of SOC estimation is less than 2% and mean absolute error and standard variations are less than 0.5% by using bias correction techniques.
-
Filter Based Approach/ Hybrid Approach
Xiong et al. [49] designed an equivalent battery model by considering open circuit voltage at different aging levels and parameters have been updated using recursive least square algorithm. Real time parameter updation has been carried out with adaptive extended Kalman filter while considering changing operating conditions and battery degradation. Results indicate that the proposed model limit the maximum SOC estimation error less than 1.5%. Hu et al. [50] proposed double step search based SVR approach for SOC prediction that will result in fast training process by avoiding
the parameter search in a large range. Simulation results have been extracted from advanced vehicle simulator that provide universal cycle conditions. Thus the proposed approach is applicable to all types of battery cells. Kim et al. [51] proposed equivalent circuit model for LI-ion battery while considering the hysteresis effect. Parameter identification has been performed with diagonal upper triangular least square method. SOC estimation has been performed with sliding mode control-based structure filter. Results shows that the proposed approach perform better as compared to Extended Kalman filter.
Meng et al. [52] proposed an integrated framework of Least Square Support Vector Machine (LSSVM) and Adaptive Unscented Kalman Filters (AUKF) for SOC esti- mation of Lithium Polymer Battery Cell. Moving window method is applied in the initial phase to limit the training samples. LSSVM method has been used to calcu- late measurement equation of AUKF technique and updated continuously with new training samples in online mode. This step helps to overcome the impact of changes in internal battery characteristics and ensure prediction accuracy. The simulation results shows that AUKF offer adaptive noise covariance adjustment as compare to Unsected Kalman Filter (UKF). Hao et al. [53] applied particle filter-based approach for SOC estimation using a second order equivalent battery model. The parameters of the model have been identified using least square method. Chen et al. [54] presented a radial basis function based neural network for SOC estimation. The concept used sliding mode observer that helps to adapt uncertain behaviour of the system. Parameter estimation of battery equivalent circuit model has been done by forgetting factor recursive least square algorithm. Simulation results shows that the proposed model is robust against the nonlinear and time varying behaviour of the batteries.
Lee et al. [55] introduced a temperature compensated equivalent model for Lithium-ion Polymer Batteries. The author also investigated EKF approach to trace the dynamic behaviour of the nonlinear devices. For this a least square error algorithm has been implemented between a temperature range of 370c to 400c. Simulation results shows
that maximum estimation error has been reduced to ±3%. Wang et al. [56] simulated Thevenins theory-based battery model to measure battery polarization resistance and
polarization capacitance. Parameters have been identified using bias compensation recursive least square method
to reduce the effect of colored noise. SOC estimation has been carried ot by EKF to further improve the estimation accuracy. Performance evaluation of the proposed model has been done under HPPC and DST drive cycles. Results shows that mean absolute error has been confined to less than 1%. Wu et al.
[57] introduced the concept of temperature compensation with Thevenins equivalent circuit of battery model and verified it with DST drive cycle. For SOC estimation, unscented Kalman filter has been implemented and verified using new European drive cycle. Simulation results shows that the maximum SOC estimation error observed is 3%. Cui et al. [58] introduced square root cubature Kalman filter approach for SOC es- timation that calculates the mean and variance of the state variables. The proposed approach propagates the square root of state variables as cholesky decomposition that eliminates the divergence of the filter. The performance has been compared with EKF, unscented Kalman filter and cubature Kalman filter. The results shows that the proposed model offer good convergence rate with high robustness. Feng et al. [59] integrated the concept of sliding mode observer with weighted Kalman filter to address the chattering problem. Second order RC equivalent circuit model has been used with two-or multi-order sliding window observer for improved SOC estimation. Performance of the proposed approach has been compared with EKF and weighted EKF. Results shows that maximum RMSE value has been confined to 0.096 with proposed model.17
Author
Battery
Ca- pac- ity
Temperature
Range
Methodology
Models Training and valida-
tion
Sun et al. [48]
2016
VOC
RBF with extended Kalman filter
Hybrid pulse test and DST cycles
Hu et al. [50]
–
–
Double search optimization ap-
proach on SVR
Training and validation over AD-
VISOR(advanced vehicle simula- tor the Matlab/Simulink)
Meng
al.[52]
et
70.0
Ah
200c to 450c
Least square support vector ma-
chine with adaptive unscented Kalman filter
Trained and validated on exper-
imental test bench
Lee et al. [55]
630
mAh
370c 400c
EKF with least square
curve-fitting method
error
MATLAB and LabView based
test bench
Xiong
[49]et
al.
24
to 34.5 Ah
250c
Recursive least square algorithm
with adaptive EKF
Validated using FUDS and DST
drive cycles
Wang
[56]et
al.
2.4Ah
100c
Bias compensation recursive
least squares method, EKF
Verified using HPPC and DST
Wu et al. [57]
2600
mAh
00c 450c
Temperature compensated un-
scented Kalman filter
Verfied using DST and HPPC
Chen
[54]et
al.
5.0Ah
250c
RBF based NN, Sliding mode ob-
server and Recursive least square algorithm
Verified by UDDS and HFET
Feng
[59]et
al.
36
Ah
250c Weighted EKF with discrete slid- ing mode observer
MATLAB based simulation
Observed RMSE value has been
confined to 0.096
Table 5: Summary of SOC estimation techniques for Lithium-ion Polymer Battery (LiPB) (Cont.)
-
-
-
Lithium Nickel Manganese Cobalt Oxide (LiNiMnCoO2) NMC
The combination of Lithium Nickel Manganese Cobalt Oxide (NMC) act as an electrode for lithium-ion batteries with high thermal stability with low heating rate. High energy density, lower cost and longer life span are the other advantages of NMC batteries. Low nominal voltage and poor mechanical stability are the main drawbacks of NMC batteries.
Table 6: Specifications of Lithium Nickel Manganese Cobalt Oxide Battery
Cell level specifications
Lithium Nickel Manganese Cobalt Oxide Battery
Capacity
2,800mAh
Nominal voltage(v/cell)
3.70V
Maximum Charge Voltage
4.2V
Energy density
150220Wh/kg
Cycle life(1C)
2000
Working temperature range
charging 0-50C
-
Techniques for SOC Prediction
-
Artificial Neural Networks (ANN) and Machine Learning (ML) Based Approach
Tong et al. [60] proposed neural network-based SOC estimation methodology with the implementation of load classification battery process model. The proposed ap- proach firstly pre-process the battery inputs to sort out the operational modes of the battery including idle state, charging and discharging states. Three separate neural networks have been trained parallelly with respect to each operational mode. The load profile of vehicle operational cycle has been used for model training and duty cycle pulse duration test has been used for validating the results. An average error of the order of 3.8% has been observed in estimating the State of Charge (SOCs) and can be further reduced by employing suitable filtering techniques at the output. Hossain et al. [61] utilized the Principal Component Analysis (PCA) and Particle Swarm Optimization (PSO) techniques in back propagation based neural networks to increase the SOC estimation accuracy. PCA technique is used to select most significant input feature space and simulation results depicts the selection of seven input parameters that have strong mapping with battery SOC. Optimization of proposed model in terms of number of hidden layers and learning rate has been done by the PSO algorithm. The proposed model has been evaluated under three electric vehicle drive cycles and comparison has been performed with conventional back propagation neural networks and radial basis function neural networks. The model offered Root Mean Square Error (RMSE) of 0.47% for USO6 drive cycle, 0.58% for Beijing dynamic stress test and 0.72% for Federal urban drive schedule.
Liu et al. [62] presented a Gaussian Process Regression (GPR) based data driven ap- proach for the capacity estimation of Nickel Cobalt Manganese Cobalt Oxide (NMC)lithium
ion (Li-ion) batteries(21Ah). Depth of discharge rate, cyclic temperature and tendency of capacity aging have been considered as the input features for predicting the battery capacity. The author proposed two different structures for GPR models by integrating empirical and electrochemical knowledge of battery aging with covariance function. The structure 1 is capable in removing the irrelevant inputs and extracting the most useful parameters for GPR by changing the squared exponential kernel with the relevance determination methodology. The structure 2 combines the empirical and electrochemical battery aging parameters with the proposed GPR model using the Arrhenius law. The results have been validated by doing comparison with single sequential exponential GPR. It was observed that structure 2 offers Root Mean Square Error (RMSE) and Mean Absolute Error (MAE)values less than 0.4% (0.09Ah) and 0.3%(0.07Ah). Lipu et al. [63] proposed Gravitational Search Algorithm (GSA) based Extreme Learning Machine (ELM) model as SOC estimator. The GSA will enhane the generalization performance, estimation accuracy and computational speed by optimizing the number of neurons in the hidden layer. The performance of the proposed model has been compared with Back Propagation Neural Network (BPNN) with GSA, Radial Basis Function Neural Network (RBFNN) with GSA and PSO under different temperatures, vehicle drive cycles and noise. The results shows that the model offer RMSE value less than 1% for BJDST cycle and 1.6% for US06 drive cycle.
Yang et al. [64] proposed a Gated Recurrent Unit (RLU) based recurrent neural net- work for battery health monitoring. The model makes use of past SOC measurements to estimate the current SOC. Training and testing data has been collected from dynamic load profiles of lithium nickel manganese cobalt oxide (NMC) batteries and lithium iron phosphate (LFP) batteries. It has been observed that LFP batteries more number of hidden neurons as compare to NMC to trace the battery behaviour. Furthermore, NMC offer 2.5% RMSE value and it is 3.5% for LFP batteries.
-
Support Vector Machine (SVM)
Feng et al. [65] proposed online SOC estimation framework for Li-ion batteries. SVM based predictive model analyze the charging data of battery cells to depicts the char- acteristic behaviour of Li-ion battery. With constant current, partial charging time segment of fifteen minutes length has been used as the input for the model. Once the support vectors are finalized, the model is trained to calculate the SVMs coefficients of cells for different state of health conditions. Similarity factor has been calculated by analyzing stored SVM charging curves with the data under consideration. Ex- periments have been conducted with graphite anode and Li(NiCoMn)O2 and results indicate an error rate of less than 2% in more than 80% cases and less than 3% error in more than 90% cases.
-
Fuzzy Logic
Hu et al. [66] presented a fuzzy logic-based SOC estimation technique for series connected battery cells. The fuzzy adaptive federated filtering technique overcome the effect of inconsistencies on SOC estimation accuracy. Battery cell inconsistencies have been characterized by mean plus difference model. To derive the fusion weights of the fuzzy system, cell mean model combine the initial SOC estimation with the standard deviation. The performance evaluation has been carried out for individual battery cell. Results shows that over the complete range of SOC estimation, root mean square error of less than 0.4% (online parameters) and 1% (offline parameters) has been observed.
-
Filter Based Approach/ Hybrid Approach
Wei et al. [67] analyzed three different approaches for online SOC estimation of Li-ion batteries. The methods include Extended Kalman filter with two parallel co-estimation filters, hybrid model that uses recursive least square algorithm for parameter identi- fication and EKF for SOC estimation and third one is Rayleigh quotient and noise compensation based recursive least square method (RNRLS) for parameter identification and EKF for SOC estimation. A comparative analysis shows that recursive least square approach with extended Kalman filter offers low computational cost but losses the accuracy with the increase in noise and bias factor. On the other hand, RNRLS- EKF is more robust to noise interference but suitable for higher order models. Zhang et al. [68] analyzed OCV-SOC characteristics, capacity characteristics, internal resistance characteristics, temperature and power characteristics of battery while conducting capacity and pulse test at different temperatures. Parameters of Second order equivalent circuit model has been identified using offline parameter estimation approach and used as reference data.
Liu et al. [69] designed a deep belief network and Kalman filter based hybrid model for SOC estimation. The relationship among battery parameters and battery SOC has been traced by the deep belief network by using its nonlinear fitting capabilities. The role of the Kalman filter is to enhance the estimation accuracy by eliminating the effect of measurement noise. The experiment results show maximum mean estimation error is less than 2.2%. Li et al. [70] considered the effect of white noise on model identifica- tion and SOC accuracy. To address this issue, three bias compensation techniques has been proposed for recursive least square approach and EKF to improve the estimation accuracy.
21
Table 7: Summary of SOC estimation techniques for Lithium Nickel Manganese Cobalt Oxide (LiNiMnCoO2) NMC (Cont.)
Author
Battery
Ca- pac- ity
Temperature
Range
Methodology
Models Training and valida-
tion
Error Rate
Tong
[60]et
al.
2016
VOC , Vd1, Vd2,
LV T , LV O, Current
Parallel training of three neural
networks based on charge, dis- charge and idle mode followed by filtering approach
LM Back Propagation algorithm
on US06 vehicle drive cycle for training and pulse test duty cycle for testing
Average estimation error is 3.8%
with simple filtering techniques
Hossain et al.
[61]2017
Voltage, Current
and Temperature
BPNN
PCA and PSO on BJDST, FUDS
and USO6 drive cycles
RMSE observed 0.58%, 0.72%,
0.47% for BJDST, FUDS and
US06 respectively.
Liu et al. [62]
2019
Battery aging ten-
dency, operational temperature and depth of discharge
GPR based model structure
GPR based model A to elim-
inate irrelevant inputs GPR based model B for integrating
empirical and electrochemical el- ements in to GPR model
Observed RMSE is 0.4%
Lipu
[63]et
al.
2019
Voltage, Current
and Temperature
BPNN and RBFNN
ELM and GSA algorithm on
BJDST and US06 drive cycles
Observed RMSE is below 1% in
BJDST and below 1.6% in US06.
Yang
[64]et
al.
2019
Voltage, Current
and Temperature
RNN with gated recurrent unit
Training and testing on
FUDS(8300 data points) and DST(8500 data points) drive cycles respectively and FUDS
Observed RMSE is 3.5% with
varying temperature range
Feng
[65]et
al.
24Ah
and 20Ah
250c
SVM
Trained and validated on Bat-
tery Cycler BT-3008
Observed error is less than 2% er-
ror for 80% of all the cases, and less than 3% error for 95% of all the cases
Hu et al. [66]
2.2
Ah
250c
fuzzy adaptive federated filtering
Simulation based test bench
Observed RMSE is 0.6% and
1.5% with inline and offline pa- rameters respectively
Liu et al. [69]
2200
mAh
–
Deep belief network with Kalman
filter approach
Validated using DST drive cycle
Observed maximum mean esti-
mation error is less than 2.2%
Wei et al. [67]
2200
mAh
–
Dual EKF, Recursive
square-EKF, RNRLS-EKF
least
Verified by hybrid pulse experi-
ment
Observed RMSE is 0.78%, 1.10%
and 0.69% for Dual EKF, Recur- sive least square-EKF, RNRLS- EKF rspectively
-
-
-
Lithium Nickel Cobalt Aluminum Oxide (LiNiCoAlO2) or NCA
The combination of Lithium Nickel Cobalt Aluminum Oxide is used as cathode in NCA batteries. The technology is similar to NMC batteries but Nickel amount is high in NCA batteries. Because of this feature, the battery capacity gets extended that contributes to cover longer distances with single charge. Also, integration of aluminum leads to higher operational stability. Thermal breakdown, early aging and safety issue are the main concerns for NCA technology.
Table 8: Specifications of Lithium Nickel Cobalt Aluminum Oxide Battery
Cell level specifications
Lithium Nickel Cobalt Aluminum Oxide Battery
Capacity
180 to 200 mAh/g
Nominal voltage(v/cell)
3.60V
Maximum Charge Voltage
4.2V
Energy density
256Wh/kg
Cycle life(1C)
500
Working temperature range
charging -30-60C
-
Techniques for SOC Prediction
-
Artificial Neural Networks (ANN) and Machine Learning (ML) Based Approach
Chemali et al. [71] offered a framework to self-learn the network parameters using long LSTM cell based Recurrent Neural Networks (RNN). The proposed approach precisely estimates the battery SOC directly from the voltage, current and temperature measurements without computationally complex inference algorithms and filters. Stochastic gradient descent algorithm based self-learning approach results in lesser number of drive cycles for model training. Furthermore, the proposed framework can accurately estimate the SOC at different ambient temperatures and with different scarce data sets. Zhang et al. [72] proposed deep learning-based estimation of remaining useful life of Li- ion battery. To consider the time dependent capacity degradations, Recur- rent Neural Network (RNN) with long short-term memory approach is used. Mini batch training of designed neural network has been implemented using mean square back propagation approach. To resolve the issue of overfitting, a dropout technique is employed which improves the prediction accuracy of proposed network. Monte Carlo approach has been used to simulate the performance analysis between the proposed model, Support Vector Machine (SVM)and simple RNN model. Predictions can be carried out independent to the offline training data and if some offline data is available, remaining useful life predictions can be carried out earlier in comparison to traditional methods. The experimentation has been carried out at two different temperatures and at different current rating. Results validate that the proposed RNN model leads to more precise and accurate results as compare to SVM and simple RNN model.
Xia et al. [73] presented a Levenberg-Marquardt (LM) algorithm optimized multi layer wavelet neural network for accurate SOC estimations. For optimum results, the proposed model is combined either with particle swarm optimization technique (PSO), piece-wise network model and and linear smoothing method, based on the specific characteristics of SOC estimation. The performance comparison has been carried out against Kalman filter and back propagation neural network. The piece wise network model offer minimum value of Mean Absolute Error (MAE) as 0.6% and maximum value up to 5% for New European Driving Cycle.
Chemali et al. [74] introduced deep learning-based feed forward neural networks model
for SOC estimations. Deep learning-based ability make the model to self-learn the weights and associated parameters. The ambient temperature ranges from 200c to 250c has been used to train the model so that the battery behaviour at different temperature ranges can be directly mapped to the weights of the proposed model. The model is also able to overcome the imperfections in vehicles measurement devices in terms of measurement offsets, noise and gains. The results validate that the proposed model offers Mean Absolute Error (MAE) in the range of 1.10%to2.17%. Zhang [75] further improves the performance of the model by introducing a fusion technique to reduce the amount of data that is needed for accurate estimation of battery health conditions. For the implementation of fusion technique, relevance vector machine is used for feature extraction and particle filter is employed to update the relevant parameters for accurate ageing model. Zhang et al. [76] introduced a hybrid approach of ampere hour counting method and back propagation neural networks for SOC estimation. The performance of the proposed method has been validated under different aging cycles and results shows that maximum SOC estimation error has been confined to ±2.0%.
Hannan et al. [77] proposed exogenous inputs based recurrent autoregressive non- linear neural network for SOC estimation. The proposed model integrates Lightning Search Algorithm (LSA) to make the system more robust and accurate under different working conditions. The experimental data has been obtained from hybrid pulse power characterization test and constant discharge test. The performance analysis has been carried out between the proposed model and backtracking search algorithm, gravitational search algorithm and particle swarm optimization technique. Results validate that the proposed model achieve lowest SOC estimation error and lowest objective function under different operating temperature ranges. Fasahat et al. [78] implemented a combined model of LSTM and autoencoder neural network. Relevant feature selection has been performed by autoencoder neural network and precise capturing of data trend has been performed by LSTM. The proposed model is evaluated and offer satisfactory estimation results with Federal Urban Driving Schedule (FUDS) and Dynamic Stress Test (DST). Zhang et al. [79] proposed a Fast Recursive Algorithm (FRA) based Radial Basis Function (RBF) neural network. FRA has been used to select relevant and compact input set which is highly correlated with SOC parameters. It is further utilized to prune redundant hidden layer neurons. For kernel parameter optimization, PSO algorithm has been used. Chandran et al. [80] evaluated six machine learning models for SOC estimation including Gaussian process regression, artificial neural networks, linear regression, support vector machine, ensemble boosting and ensemble bagging. From the simulation results, it has been concluded that Gaussian process regression and artificial neural networks with support vector machine offer high prediction accuracy as compared to rest of the models.
-
Genetic Algorithm (GA)
Chen et al. [81] designed a grey system theory-based battery model with sliding window concept for the adjustment of model variables with respect to different oper- ating conditions. Parameter optimization has been performed by Genetic algorithm. Performance validation has been carried out with device under test cycle for different discharge rates and temperature.
-
Fuzzy Logic
-
-
-
-
Zheng [82] introduced a fuzzy logic based sliding mode observer for state of health monitoring in EVs. Dynamic behaviour of the battery cells has been traced by resistor- capacitor equivalent circuit model with exponential fitting method for parameter up- dation. The relationship between state of charge and open circuit voltage has been demonstrated by piece-wise linear fitting approach. Results have been verified under New European Drive Cycle, West Virginia suburban driving schedule and Federal Ur- ban Driving Schedule. Comparison of the proposed model has been carried out with conventional sliding mode observer and extended Kalman filter. Results shows that an average estimation error with fuzzy logic based sliding mode observer is less than 1% and quickly converge to 3% with in 240s.
25
Table 9: Summary of SOC estimation techniques for Lithium Nickel Cobalt Aluminum Oxide (LiNiCoAlO2) or NCA (Cont.)
Author |
Battery Ca- pac- ity |
Temperature Range |
Methodology |
Models Training and valida- tion |
Error Rate |
|||
Chemali et al. [71] |
2017 |
Voltage, Current, Temperature |
RNN with LSTM |
Stochastic gradient descent al- gorithm over HWFET,UDDS, LA92 and US06 drive cycles |
MAE is 0.573% with fixed tem- perature MAE is 1.606% with temperature variations from 100c to 250c |
|||
Zhang [72] |
et |
al. |
2018 |
Voltage, Current, Temperature |
RNN with LSTM |
Mini batch gradient descent al- gorithm with dropout technique to address overfitting Monte carlo simulation to estimate un- certainties |
LSTM offer minimum error in comparison with simple RNN and SVM techniques |
|
Xia et al. [73] |
2150 mAh |
25% |
LM algorithm based wavelet neural networks optimized by integrating piecewise network method |
– |
– |
|||
Chemali et al. [74] |
2018 |
Voltage, Current, Temperature |
Deep feed forward neural net- works |
Stochastic gradient descent al- gorithm over HWFET,UDDS, LA92 and US06 drive cycles |
|
|||
Hannan et al. [77] |
3200 mAh |
00c 450c |
RNN with nonlinear au- toregressive exogenous inputs Lightning search algorithm |
Training and validation on CDT, HPPC, DST and FUDS drive cy- cles |
Observed RMSE is 0.68% for CDT, 0.43% for HPPC, 0.56% for DST and 0.86% for FUDS. |
|||
Fasahat et al. [78] |
2000 mAh |
00c,250c, 450c |
LSTM based autoencoder neural network |
Training and validation on DST and FUDS |
Observed RMSE is 0.99 at 00c, 1.1 at 250c, 0.6 at 450c for DST drive cycle Observed RMSE is 1.69 at 00c, 1.81 at 250c, 0.5 at 450c for FUDS drive cycle |
|||
Chen [81] |
et |
al. |
2.0 and 2.6 Ah |
40c, 240,440c |
Grey model and genetic algo- rithm |
Experimental test bed |
Observed relative error is 0.13 |
|
Zheng [82] |
2150 mAh |
00c, 250c, 450c |
Fuzzy logic based sliding mode observer, Piecewise linear fitting model |
Training and validation FUDS, WUBSUB, NEDC |
on |
Observed RMSE is 5,0.97,2.12 at 00c, 250c, 450c respectively |
5. CONCLUSION
The presented review classified different material compositions or technologies under lithium-ion batteries and compare them on the basis of their merits, demerits and specifica- tions. This review critically investigates different SoC estimation algorithms/methodologies with a focus on their use in electric vehicles. An accurate estimation of SoC is a major research issue because of the sensitivity of Li-ion batteries towards internal electro-chemical reactions, temperature, cell imbalance, variable hysteresis features, self-discharge and bat- tery aging.
The review concluded that direct measurements of physical quantities or conventional methods are easy in implementation but their performance is highly affected by temperature variations, battery aging and drifts. The battery model based SoC estimation is more precise in comparison to Conventional methods. Adaptive filtering-based estimation approach can precisely trace nonlinear dynamic behaviour of the battery state but offers poor robustness and high computational complexity. The machine learning based SoC estimation techniques performs precisely for a nonlinear dynamic behaviour system. These models maintain their accuracy level under temperature instability and battery aging effect but require a high-speed controller and high storage time for complex calculation. During survey, it has been observed that integration of various SoC estimation techniques for eg. Machine learning models followed by filtering approach can lead to higher degree of accuracy in battery health monitoring and thus safe EV operations.
5.1. Research Areas for Future Innovations
Based on the proposed comprehensive review, there are some selective suggestions for future development of SoC estimation methods, such as:
-
Detailed analysis is needed while finalizing battery modeling parameters and hyper parameters while implementing model driven or data driven SoC estimation methods.
-
Computation complexity of data driven models need to be addressed by considering various optimization techniques.
-
SoC estimations need to be further analyzed in real world environment including noise effect, aging and temperature variations.
-
In achieving full-fledged market acceptance, durability, safety and mobility of lithium-ion batteries need to be addressed.
-
A highly efficient real time battery management system is must for EVs that can achieve thermal stability, charge equalization, fault diagnostics and risk prevention for accurate SoC estimations.
-
A benchmark and validated, generalized SoC estimation method is needed.
The author assume that these suggestions would contribute in the direction of accurate SoC estimations. Also, future SoC estimation technologies for lithium-ion batteries will dominate the future EV market.
References
[1] D. N. How, M. Hannan, M. H. Lipu, P. J. Ker, State of charge estimation for lithium-ion batteries using model- based and data-driven methods: A review, Ieee Access 7 (2019) 136116136136. [2] S. Nejad, D. Gladwin, D. Stone, A systematic review of lumped-parameter equivalent circuit models for real-time estimation of lithium-ion battery states, Journal of Power Sources 316 (2016) 183196. [3] A. Seaman, T.-S. Dao, J. McPhee, A survey of mathematics-based equivalent-circuit and electrochem- ical battery models for hybrid and electric vehicle simulation, Journal of Power Sources 256 (2014) 410423. [4] Q. Zhang, D. Wang, B. Yang, X. Cui, X. Li, Electrochemical model of lithium-ion battery for wide frequency range applications, Electrochimica Acta 343 (2020) 136094. [5] Y. Wang, D. Yang, X. Zhang, Z. Chen, Probability based remaining capacity estimation using data- driven and neural network model, Journal of Power Sources 315 (2016) 199208. [6] J. Wei, G. Dong, Z. Chen, Remaining useful life prediction and state of health diagnosis for lithium-ion batteries using particle filter and support vector regression, IEEE Transactions on Industrial Electronics 65 (7) (2017) 5634 5643. [7] V. Johnson, Battery performance models in advisor, Journal of power sources 110 (2) (2002) 321329. [8] J. P. Christophersen, C. G. Motloch, C. D. Ho, D. F. Glenn, R. B. Wright, J. R. Belt, T. C. Murphy,T. Q. Duong, Doe advanced technology development program for lithium-ion batteries: Ineel interim report for gen 2 cycle-life testing, 9: INEEL/EXT-02-01055 (2002).
[9] B. A. Manual, Gates energy products, Inc., Gainesville, FL (1989). [10] S. Singirikonda, Y. Obulesu, Battery modelling and state of charge estimation methods for energy management in electric vehicle-a review, in: OP Conference Series: Materials Science and Engineering, Vol. 937, IOP Publishing, 2020, p. 012046. [11] W. He, M. Pecht, D. Flynn, F. Dinmohammadi, A physics-based electrochemical model for lithium-ion battery state- of-charge estimation solved by an optimised projection-based method and moving-window filtering, Energies 11 (8) (2018) 2120. [12] J. Wu, C. Zhang, Z. Chen, An online method for lithium-ion battery remaining useful life estimation using importance sampling and neural networks, Applied energy 173 (2016) 134140. [13] A. J. Salkind, C. Fennie, P. Singh, T. Atwater, D. E. Reisner, Determination of state-of-charge and state-of-health of batteries by fuzzy logic methodology, Journal of Power sources 80 (1-2) (1999) 293 300. [14] M. A. Patil, P. Tagade, K. S. Hariharan, S. M. Kolake, T. Song, T. Yeo, S. Doo, A novel multistage support vector machine based approach for li ion battery remaining useful life estimation, Applied energy 159 (2015) 285297. [15] M. Charkhgard, M. Farrokhi, State-of-charge estimation for lithium-ion batteries using neural networks and ekf, IEEE transactions on industrial electronics 57 (12) (2010) 41784187. [16] F. Li, J. Xu, A new prognostics method for state of health estimation of lithium-ion batteries based on a mixture of gaussian process models and particle filter, Microelectronics Reliability 55 (7) (2015) 10351045. [17] D. Anse´an Gonz´alez, M. Gonz´alez Vega, J. C. Viera P´erez, J. C. A´ lvarez A´ lvarez, C. J. Blanco Viejo,V. Garc´a, et al., Evaluation of lifepo4 batteries for electric vehicle applications, in: Conference and Exhibition- 2013 International Conference on New Concepts in Smart Cities: Fostering Public and Private Alliances, SmartMILE 2013, 2013.
[18] J. C. A. Anton, P. J. G. Nieto, C. B. Viejo, J. A. V. Vilan, Support vector machines used to estimate the battery state of charge, IEEE Transactions on power electronics 28 (12) (2013) 59195926. [19] Z. Wang, D. Yang, State-of-charge estimation of lithium iron phosphate battery using extreme learning machine, in: 2015 6th International Conference on Power Electronics Systems and Applications (PESA), IEEE, 2015, pp. 15. [20] X. Dang, L. Yan, K. Xu, X. Wu, H. Jiang, H. Sun, Open-circuit voltage-based state of charge estimation of lithium- ion battery using dual neural network fusion battery model, Electrochimica Acta 188 (2016) 356366. [21] H. Sheng, J. Xiao, P. Wang, Lithium iron phosphate battery electric vehicle state-of-charge estimation based on evolutionary gaussian mixture regression, IEEE Transactions on Industrial Electronics 64 (1) (2016) 544551. [22] H. Chaoui, C. C. Ibe-Ekeocha, H. Gualous, Aging prediction and state of charge estimation of a lifepo4 battery using input time-delayed neural networks, Electric Power Systems Research 146 (2017) 189197. [23] J. Chen, Q. Ouyang, C. Xu, H. Su, Neural network-based state of charge observer design for lithium-ion batteries, IEEE Transactions on Control Systems Technology 26 (1) (2017) 313320. [24] Y. Guo, Z. Zhao, L. Huang, Soc estimation of lithium battery based on improved bp neural network, Energy Procedia 105 (2017) 41534158. [25] M. Wei, M. Ye, J. B. Li, Q. Wang, X. Xu, State of charge estimation of lithium-ion batteries using lstm and narxneural networks, IEEE Access 8 (2020) 189236189245.
[26] Y. Tian, R. Lai, X. Li, L. Xiang, J. Tian, A combined method for state-of-charge estimation for lithium- ion batteries using a long short-term memory network and an adaptive cubature Kalman filter, Applied Energy 265 (2020) 114789. [27] Q. Wang, P. Wu, J. Lian, Soc estimation algorithm of power lithium battery based on afsa-bp neural network, The Journal of Engineering 2020 (13) (2020) 535539. [28] M. Esfandyari, M. H. Yazdi, V. Esfahanian, M. Masih-Tehrani, H. Nehzati, O. Shekoofa, A hybrid model predictive and fuzzy logic based control method for state of power estimation of series-connected lithium-ion batteries in hevs, Journal of Energy Storage 24 (2019) 100758. [29] D. Di Domenico, E. Prada, Y. Creff, An adaptive strategy for li-ion battery soc estimation, IFAC Proceedings Volumes 44 (1) (2011) 97219726. [30] Z. Chen, Y. Fu, C. C. Mi, State of charge estimation of lithium-ion batteries in electric drive vehicles using extended Kalman filtering, IEEE Transactions on Vehicular Technology 62 (3) (2012) 10201030. [31] M. Mastali, J. Vazquez-Arenas, R. Fraser, M. Fowler, S. Afshar, M. Stevens, Battery state of the charge estimation using Kalman filtering, Journal of Power Sources 239 (2013) 294307. [32] Z. Yu, R. Huai, L. Xiao, State-of-charge estimation for lithium-ion batteries using a Kalman filter based on local linearization, Energies 8 (8) (2015) 78547873. [33] G. Dong, J. Wei, Z. Chen, Kalman filter for onboard state of charge estimation and peak power capability analysis of lithium-ion batteries, Journal of Power Sources 328 (2016) 615626. [34] Z. Deng, L. Yang, Y. Cai, H. Deng, L. Sun, Online available capacity prediction and state of charge estimation based on advanced data-driven algorithms for lithium iron phosphate battery, Energy 112 (2016) 469480. [35] B. Zhao, J. Liu, H. Li, Soc estimation of lithium iron phosphate battery based on Kalman filtering algorithm, Chemical Engineering Transactions 62 (2017) 145150. [36] J. Li, F. Gao, G. Yan, T. Zhang, J. Li, Modeling and soc estimation of lithium iron phosphate battery considering capacity loss, Protection and Control of Modern Power Systems 3 (1) (2018) 19. [37] J. Wu, T. Li, H. Zhang, Y. Lei, G. Zhou, Research on modeling and soc estimation of lithium iron phosphate battery at low temperature, Energy Procedia 152 (2018) 556561. [38] M. Kim, K. Kim, J. Kim, J. Yu, S. Han, State of charge estimation for lithium-ion battery based on reinforcement learning, IFAC-PapersOnLine 51 (28) (2018) 404408. [39] T. Zheng, Application of Kalman filter algorithm in battery state-of-charge detection, Chemical Engi- neering Transactions 71 (2018) 12731278. [40] G. Dong, Z. Chen, J. Wei, Sequential monte carlo filter for state-of-charge estimation of lithium-ion batteries based on auto regressive exogenous model, IEEE Transactions on Industrial Electronics 66 (11) (2019) 85338544. [41] Q. Liu, S. Liu, H. Liu, H. Qi, C. Ma, L. Zhao, Evaluation of lfp battery soc estimation using auxiliary particle filter, Energies 12 (11) (2019) 2041. [42] K. V. Singh, H. O. Bansal, D. Singh, Hardware-in-the-loop implementation of anfis based adaptive soc estimation of lithium-ion battery for hybrid vehicle applications, Journal of Energy Storage 27 (2020) 101124. [43] H. Li, W. Zhang, X. Yang, H. Jiang, Y. Wang, T. Yang, L. Chen, H. Shen, State of charge estimation for lithium-ion battery using an electrochemical model based on electrical double layer effect, Electrochimica Acta 326 (2019) 134966. [44] F. Yang, S. Zhang, W. Li, Q. Miao, State-of-charge estimation of lithium-ion batteries using lstm and ukf, Energy 201 (2020) 117664. [45] T.-T. Nguyen, A. B. Khan, Y. Ko, W. Choi, An accurate state of charge estimation method for lithium iron phosphate battery using a combination of an unscented Kalman filter and a particle filter, Energies 13 (17) (2020) 4536. [46] D. Sun, X. Yu, C. Wang, C. Zhang, R. Huang, Q. Zhou, T. Amietszajew, R. Bhagat, State of charge estimation for lithium-ion battery based on an intelligent adaptive extended Kalman filter with improved noise estimator, Energy 214 (2021) 119025. [47] C. Burgos, D. Saez, M. E. Orchard, R. C´ardenas, Fuzzy modelling for the state-of-charge estimation of lead-acid batteries, Journal of Power Sources 274 (2015) 355366. [48] F. Sun, R. Xiong, H. He, A systematic state-of-charge estimation framework for multi-cell battery pack in electric vehicles using bias correction technique, Applied Energy 162 (2016) 13991409. [49] R. Xiong, F. Sun, X. Gong, C. Gao, A data-driven based adaptive state of charge estmator of lithium- ion polymer battery used in electric vehicles, Applied Energy 113 (2014) 14211433. [50] J. Hu, J. Hu, H. Lin, X. Li, C. Jiang, X. Qiu, W. Li, State-of-charge estimation for battery management system using optimized support vector machine for regression, Journal of Power Sources 269 (2014) 682693. [51] T. Kim, Y. Wang, Z. Sahinoglu, T. Wada, S. Hara, W. Qiao, State of charge estimation based on a realtime battery model and iterative smooth variable structure filter, in: 2014 IEEE Innovative Smart Grid Technologies-Asia (ISGT ASIA), IEEE, 2014, pp. 132137. [52] J. Meng, G. Luo, F. Gao, Lithium polymer battery state-of-charge estimation based on adaptive un- scented Kalman filter and support vector machine, IEEE Transactions on Power Electronics 31 (3) (2015) 22262238. [53] X. Hao, J. Wu, Online state estimation using particles filters of lithium-ion polymer battery packs for electric vehicle, in: 2015 IEEE International Conference on Systems, Man, and Cybernetics, IEEE, 2015, pp. 783788. [54] X. Chen, W. Shen, M. Dai, Z. Cao, J. Jin, A. Kapoor, Robust adaptive sliding-mode observer using rbf neural network for lithium-ion battery state of charge estimation in electric vehicles, IEEE Transactions on Vehicular Technology 65 (4) (2015) 19361947. [55] K.-T. Lee, M.-J. Dai, C.-C. Chuang, Temperature-compensated model for lithium-ion polymer batteries with extended Kalman filter state-of-charge estimation for an implantable charger, IEEE Transactions on Industrial Electronics 65 (1) (2017) 589596. [56] Z. Wang, Z. Liu, Z. Li, Bcrls-ekf-based parameter identification and state-of-charge estimation approach of lithium- ion polymer battery in electric vehicles, in: Society of Automotive Engineers (SAE)-China Congress, Springer, 2017, pp. 629640. [57] X. Wu, X. Li, J. Du, State of charge estimation of lithium-ion batteries over wide temperature range using unscented Kalman filter, Ieee Access 6 (2018) 4199342003. [58] X. Cui, Z. Jing, M. Luo, Y. Guo, H. Qiao, A new method for state of charge estimation of lithium-ion batteries using square root cubature Kalman filter, Energies 11 (1) (2018) 209. [59] L. Feng, J. Ding, Y. Han, Improved sliding mode based ekf for the soc estimation of lithium-ion batteries, Ionics (2020) 18. [60] S. Tong, J. H. Lacap, J. W. Park, Battery state of charge estimation using a load-classifying neural network, Journal of Energy Storage 7 (2016) 236243. [61] M. Hossain Lipu, M. Hannan, A. Hussain, M. Saad, Optimal bp neural network algorithm for state of charge estimation of lithium-ion battery using pso with pca feature selection, Journal of Renewable and Sustainable Energy 9 (6) (2017) 064102. [62] K. Liu, X. Hu, Z. Wei, Y. Li, Y. Jiang, Modified gaussian process regression models for cyclic capacity prediction of lithium-ion batteries, IEEE Transactions on Transportation Electrification 5 (4) (2019) 12251236. [63] M. S. H. Lipu, M. A. Hannan, A. Hussain, M. H. Saad, A. Ayob, M. N. Uddin, Extreme learning machine model for state-of-charge estimation of lithium-ion battery using gravitational search algorithm, IEEE Transactions on Industry Applications 55 (4) (2019) 42254234. [64] F. Yang, W. Li, C. Li, Q. Miao, State-of-charge estimation of lithium-ion batteries based on gated recurrent neural network, Energy 175 (2019) 6675. [65] X. Feng, C. Weng, X. He, X. Han, L. Lu, D. Ren, M. Ouyang, Online state-of-health estimation for li-ion battery using partial charging segment based on support vector machine, IEEE Transactions on Vehicular Technology 68 (9) (2019) 85838592. [66] L. Hu, X. Hu, Y. Che, F. Feng, X. Lin, Z. Zhang, Reliable state of charge estimation of battery packs using fuzzy adaptive federated filtering, Applied Energy 262 (2020) 114569. [67] Z. Wei, J. Zhao, C. Zou, T. M. Lim, K. J. Tseng, Comparative study of methods for integrated model identification and state of charge estimation of lithium-ion battery, Journal of Power Sources 402 (2018) 189197. [68] R. Zhang, B. Xia, B. Li, Y. Lai, W. Zheng, H. Wang, W. Wang, M. Wang, Study on the character- istics of a high capacity nickel manganese cobalt oxide (nmc) lithium-ion batteryan experimental investigation, energies 11 (9) (2018) 2275. [69] D. Liu, L. Li, Y. Song, L. Wu, Y. Peng, Hybrid state of charge estimation for lithium-ion battery under dynamic operating conditions, International Journal of Electrical Power & Energy Systems 110 (2019) 4861. [70] Y. Li, J. Chen, F. Lan, Enhanced online model identification and state of charge estimation for lithium- ion battery under noise corrupted measurements by bias compensation recursive least squares, Journal of Power Sources 456 (2020) 227984. [71] E. Chemali, P. J. Kollmeyer, M. Preindl, R. Ahmed, A. Emadi, Long short-term memory networks for accurate state-of-charge estimation of li-ion batteries, IEEE Transactions on Industrial Electronics 65 (8) (2017) 67306739. [72] Y. Zhang, R. Xiong, H. He, M. G. Pecht, Long short-term memory recurrent neural network for remaining usefullife prediction of lithium-ion batteries, IEEE Transactions on Vehicular Technology 67 (7) (2018) 56955705.
[73] B. Xia, D. Cui, Z. Sun, Z. Lao, R. Zhang, W. Wang, W. Sun, Y. Lai, M. Wang, State of charge estimation of lithium- ion batteries using optimized levenberg-marquardt wavelet neural network, Energy 153 (2018) 694705. [74] E. Chemali, P. J. Kollmeyer, M. Preindl, A. Emadi, State-of-charge estimation of li-ion batteries using deep neural networks: A machine learning approach, Journal of Power Sources 400 (2018) 242255. [75] Y. Zhang, R. Xiong, H. He, M. Pecht, Validation and verification of a hybrid method for remaining useful life prediction of lithium-ion batteries, Journal of Cleaner Production 212 (2019) 240249. [76] S. Zhang, X. Guo, X. Zhang, Modeling of back-propagation neural network based state-of-charge esti- mation for lithium-ion batteries with consideration of capacity attenuation, Advances in Electrical and Computer Engineering 19 (3) (2019) 310. [77] M. A. Hannan, M. H. Lipu, A. Hussain, P. J. Ker, T. Mahlia, M. Mansor, A. Ayob, M. H. Saad,Z. Dong, toward enhanced state of charge estimation of lithium-ion batteries using optimized machine learning techniques, Scientific reports 10 (1) (2020) 115.
[78] M. Fasahat, M. Manthouri, State of charge estimation of lithium-ion batteries using hybrid autoencoder and long short term memory neural networks, Journal of Power Sources 469 (2020) 228375. [79] L. Zhang, M. Zheng, D. Du, Y. Li, M. Fei, Y. Guo, K. Li, State-of-charge estimation of lithium-ion battery pack based on improved rbf neural networks, Complexity 2020 (2020). [80] V. Chandran, C. K Patil, A. Karthick, D. Ganeshaperumal, R. Rahim, A. Ghosh, State of charge estimation of lithium-ion battery for electric vehicles using machine learning algorithms, World Electric Vehicle Journal 12 (1) (2021) 38. [81] L. Chen, Z. Wang, Z. L u¨ , J. Li, B. Ji, H. Wei, H. Pan, A novel state-of-charge estimation method of lithium-ion batteries combining the grey model and genetic algorithms, IEEE Transactions on Power Electronics 33 (10) (2017) 87978807. [82] W. Zheng, B. Xia, W. Wang, Y. Lai, M. Wang, H. Wang, State of charge estimation for power lithium- ion battery using a fuzzy logic sliding mode observer, Energies 12 (13) (2019) 2491.