
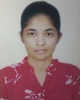
- Open Access
- Authors : Shrutika Satish Kherde , Dr. Ajay Anil Gurjar
- Paper ID : IJERTV10IS050357
- Volume & Issue : Volume 10, Issue 05 (May 2021)
- Published (First Online): 01-06-2021
- ISSN (Online) : 2278-0181
- Publisher Name : IJERT
- License:
This work is licensed under a Creative Commons Attribution 4.0 International License
A Review: Detection of Abnormalities in Liver using Image Processing Techniques
Shrutika S. Kherde Dr. Ajay A. Gurjar
Department of Electronic and Department of Electronic and
Telecommunication Engineering Telecommunication Engineering Sipna C.O.E.T. Amravati. Sipna C.O.E.T. Amravati, Maharashtra, India Maharashtra, India
Abstract: The abnormal growth of cell in the liver causes liver cancer which is also known as hepatic cancer where, Hepatocellular Carcinoma (HCC). Maximum number of the peoples who have liver tumour were died due to the fact of inaccurate detection. The detection of this tumours is difficult and mostly found at advanced stage which causes life-threatening issues. Hence it is far essential to discover the tumour at an early stage. The liver is the second organ most normally involved by metastatic disease, being liver cancer one of the prominent causes of death worldwide. The early detection and diagnosis of liver tumor is consequential for the detection of liver tumor. Medical image processing is used as a non-invasive method to detect tumors. Many techniques have been developed for the detection of liver tumor utilizing the aberrant lesion size and shape. This paper reviews many different liver tumor detection algorithms and methodologies utilized for liver tumor diagnosis.
Keywords -: Liver tumour detection, CT image, Liver segmentation.
INTRODUCTION
Billions of cells in our body divides each day to produce new cells. The newly formed cells occupy the space of dead cells. Basically, cells get together to form tissues together to form organs. Hence, in some abnormal cases, cells divide more than the body needed and form as lumps or growths normally called as tumors. Liver cancer is one of the major death factors in the world. The detection of tumours is difficult and mostly found at advance stage which causes life threating issues. There are two types of cancer namely Primary Liver Cancer and Secondary Liver Cancer. Primary Liver Cancer starts in the liver, and not from another organ which ultimately travels to the liver. Cancers that originate in the liver are known as primary liver cancers. Secondary Liver Cancer is a type growth of cancer cell where the cancer cell originates from different organ and spread to liver. In other words, there may be cancers which start from somewhere else and end up in the liver those are secondary liver cancer. The most common type of liver cancer is hepatocellular carcinoma (or hepatoma or HCC), and it tends to affect males more than females. The early detection and accurate presentation of liver cancer is a significant issue impractical radiology. Liver lesions refer to those abnormal tissue cell that are found in the liver. Liver lesions are a wound or injury in the tissue areas of the body due to harm caused by a wound or disease. These lesions can be identified in a CT scan by a difference in pixel intensity from other regions of the liver. For proper clinical treatment, manual segmentation of this CT scan is difficult and excessively time-consuming task. Digital image processing is the technique of using computers to process the image with the necessary algorithms. In the
recent year medical image processing has become an essential part of diagnosing and accumulating disease in formation for adding in clinical decision support. It is also helpful for monitoring and examining the impact of treatment of infected Detection of liver cancer involves three main steps. It includes pre-processing of image, feature extraction, pattern recognition and classification
LITERATURE REVIEW
-
Diagnosis of liver tumours Using Image Processing In this work the research aims to develop an automated way to extract suspicious regions of liver area in the scanner image to the abdominal area use a new method for extracting the liver area of the scanned image for abdominal area automatically, depending on the use of connected compounds numbering algorithms CCL, in addition to the use of data collection algorithms FCM to extract suspicious regions of the extracted area of the liver.[1]
-
Detection of Tumor in Liver Using Image Segmentation and Registration Technique author propose an algorithm for liver cancer detection which is based on concepts of fuzzy logic and neural network. Neuro-fuzzy (NF) systems are suitable tools to deal with uncertainty found in the process of extracting useful information from image.[2]
-
Computer Aided Detection of Liver Tumor using SVM Classifier In this paper, researcher have a new and accurate method for liver tumour segmentation from computed tomography (CT) scans. Initially, the liver CT image is pre-processed, i.e., noise removal and contrast of the image is enhanced. Than they employ a support vector machine (SVM) classifier, which is trained using the user fed image sets, to classify the tumour region from liver image.[3]
-
Intelligent Image Processing Techniques for Cancer Progression Detection, Recognition and Prediction in the Human Liver The proposed intelligent CDS framework will automate real-time image enhancement, segmentation, disease classification and progression to enable efficient diagnosis of cancer patients at early stages.[4]
-
Detection and classification of liver cancer using CT image In this work presents the enhancement of Computed tomography (CT) images using two different algorithms. CLAHE enhanced the tumour region in a new look. CVHE enhanced with preserving the globalization of an image. The normal liver detection is done by the ox plot
comparison in CLAHE. Primary liver cancer detection is done by CVHE. State vector machines (SVM) classifier works for the classification of CT liver images.[5]
-
Liver tumour detection for CT images using image processing techniques This paper consists of various image processing techniques like image pre-processing and image enhancement which is used to improve the quality of the liver image. This helps to detect and segment the tumour in liver effectively. The automated segmentation of liver is addressed first and then filtering is used to remove unwanted noise finally. The clustering algorithm helps to detect the tumour in liver CT image.[6]
-
Liver Tumor Segmentation in Noisy CT Image Using Distance Regularized Level Set Evolution Based on Fuzzy C-Mean Clustering. In this research work, the liver tumors are detected by the medical images in three stages, pre- processing stage, processing stage and detection stage. First in pre-processing stage, median filter is used to remove the noise from CT image, and then the denoised image is segmented by fuzzy c-means clustering (FCM) algorithm. Finally, in the detection stage distance regularized level set evolution (DRLSE) is used to extract tumor boundaries.[7]
-
Deep learning base liver cancer detection using watershed transform and gaussian mixture model techniques In this work they proposed a CAD model called watershed gaussian based deep learning technique which consist of intensity base segmentation to efficiently delineate the cancer lesion in CT image of the liver.[8]
-
Detection of liver cancer using image processing techniques In this paper, it has been used to detect cancer cell of the liver. Here ostus method is used for enhancing the MRI image and watershed method is used to segment the cancer cell from the image.[9]
-
Liver cancer detection using image processing technique. In this proposed work the image undergoes enhancement using anisotropic diffusion filters and segmented by morphological operations which is simple ad easy to work.[10]
PRIOR WORK
Wassem Abdulrahman Diagnosis of liver tumours Using Image Processing In this work, a computer-aided diagnostic (CAD) system for the diagnosis of benign and malignant liver tumors from computed tomography (CT) images is presented. The research aims to develop an automated way to extract suspicious regions of liver area in the scanner image to the abdominal area, this research uses a new method for extracting the liver area of the scanned image for abdominal area automatically, depending on the use of connected compounds numbering algorithm CCL, in addition to the use of data collection algorithm FCM to extract suspicious regions of the extracted area of the liver.[1]
Priyanka Kumar, Shailesh Bhalerao Detection of Tumor in Liver Using Image Segmentation and Registration Technique author propose an algorithm for liver cancer detection which is based on concepts of fuzzy logic and neural network. Neuro-
fuzzy (NF) systems are suitable tools to deal with uncertainty found in the process of extracting useful information from images. In this work, the liver tumor is detected through the medical images in three phases, pre-processing phase, processing phase and detection phase. Initially in the pre- processing phase, a set of medical images is filtered for removing noise. Then the filtered image is segmented automatically using fuzzy logic, neural network and windowing technique. In the detection phase neuro-fuzzified segmented images of CT and MRI is registered to obtain the tumor. The result is obtained for few different sets of data base. In this proposed work the Image segmentation done by using K-means clustering and neural network, fuzzy logic for processing of image. [2]
R. Rajagopal, P. Subbiah Computer Aided Detection of Liver Tumor using SVM Classifier In this paper, researcher have a new and accurate method for liver tumor segmentation from computed tomography (CT) scans. Initially, the liver CT image is pre-processed, i.e., noise removal and contrast of the image is enhanced. Than they employ a support vector machine (SVM) classifier, which is trained using the user fed image sets, to classify the tumor region from liver image. Sequentially, morphological operations and feature extractions are performed over the segmented binary image to further refine the rough segmentation result of SVM classification. The experiment results prove that the accuracy and efficiency of the proposed algorithm to be higher than conventional methods. [3]
L. Ali, A. Hussain, J. Li, U. Zakir, X. Yan, A. Shah, U. Sudhakr, B. Luo, Mufti Mahmud, M. Rajak Intelligent Image Processing Techniques for Cancer Progression Detection, Recognition and Prediction in the Human Liver The proposed intelligent CDS framework will automate real-time image enhancement, segmentation, disease classification and progression in order to enable efficient diagnosis of cancer patients at early stages. The CDS framework is inspired by the human interpretation of US images from the image acquisition stage to cancer progression prediction. Specifically, the proposed framework is composed of a number of stages where images are first acquired from an imaging source and pre- processed before running through an image enhancement algorithm. The detection of cancer and its segmentation is considered as the second stage in which different image segmentation techniques are utilized to partition and extract objects from the enhanced image. The third stage involves disease classification of segmented objects, in which the meanings of an investigated object are matched with the disease dictionary defined by physicians and radiologists. In the final stage; cancer progression, an array of US images is used to evaluate and predict the future stages of the disease. [4]
Abhay Krishan, Deptti Mittal Detection and classification of liver cancer using CT image In this work presents the enhancement of Computed tomography (CT) images using two different algorithms: Contrast Limited Adaptive Histogram Equalization (CLAHE) and Constrained Variable Histogram Equalization (CVHE). CLAHE enhanced the tumour region in a new look. CVHE enhanced with preserving the globalization
of an image. The normal liver detection is done by the ox plot comparison in CLAHE. Primary liver cancer detection is done by CVHE. State vector machines (SVM) classifier works for the classification of CT liver images. [5]
Saranya, S. and Dr. M. Pushpa Rani Liver tumour detection for CT images using image processing. In proposed work involves three stages that is image pre-processing, image enhancement and image segmentation. The input is taken here is CT image. After collecting the image from scanner, pre- processing should take place for reducing unwanted noise in an image. Tumours sometimes present in a very small region which is difficult to identify. To overcome this the proposed work, take CLAHE method which is used to enhance the contrast of an image. FCM clustering algorithm is used to detect the tumour in liver CT image. [6]
Pavushetty Yugandar G. Raghotham Reddy Liver Tumor Segmentation in Noisy CT Image Using Distance Regularized Level Set Evolution Based on Fuzzy C-Mean Clustering In this paper, author propose an approach to detection and segmentation of liver tumors in noisy computed topography (CT) images. The essential goal of this framework is to identify liver cancer by segmenting liver tumors from noisy CT scan images. Liver segmentation is difficult task in medical applications because inter-patient variability in size, shape and disease. In this research work, the liver tumors are detected by the medical images in three stages, pre-processing stage, processing stage and detection stage. First in pre-processing stage, median filter is used to remove the noise from CT image, and then the denoised image is segmented by fuzzy c-means clustering (FCM) algorithm. Finally, in the detection stage distance regularized level set evolution (DRLSE) is used to extract tumor boundaries. [7]
Sukanta Sabut, Amita Das, U Rajendra Acharya Deep learning base liver cancer detection using watershed transform and gaussian mixture model techniques In this work they proposed a new system called as watershed Gaussian based deep learning (WGDL) technique for effective delineate the cancer lesion in computed tomography (CT) images of the liver. A total of 225 images were used in this work to develop the proposed model. Initially, the liver was separated using marker-controlled watershed segmentation process and finally the cancer affected lesion was segmented using the Gaussian mixture model (GMM) algorithm. After tumor segmentation, various texture features were extracted from the segmented region. These segmented features were fed to deep neural network (DNN) classifier for automated classification of three types of liver cancer i.e. hemangioma (HEM), hepatocellular carcinoma (HCC) and metastatic carcinoma (MET). [8]
Atrayee Dutta and Aditya Dubey Detection of liver cancer using image processing techniques. In this paper, it has been used to detect cancer cell of the liver. Here ostus method is used for enhancing the MRI image and watershed method is used to segment the cancer cell from the image. [9]
R Aarthi, S Nivetha, P Vikashini, Dr. V. T. Balamurgan Liver cancer detection using image processing technique. The principle intention of this research work is to detect liver cancer at earlier stage using image processing technique. Here the malignant liver tumours are detected from Computed Tomography (CT) images. The image undergoes enhancement using anisotropic diffusion filters and segmented by morphological operations which is simple and easy to work. This operation uses combination of two processes, dilation and erosion. The scope of this propounded technique is to highlight the tumour region present in the Computed Tomography. [10]
Sr. No |
Name of Author |
Year |
Techniques Used | ||||
1. |
Wassem Abdulrahman |
2014 |
CAD system, CCL Algorithm, FCM Algorithm. |
||||
2. |
Priyanka Kumar, Shailesh Bhalerao |
2014 |
fuzzy logic, Network. |
K |
means |
clustering, |
Neural |
3. |
R .Rajagopal, P. Subbiah |
2014 |
Otsus algorithm, LBP Feature Extraction, SVM Classifier |
||||
4. |
L. Ali, A. Hussain, J. Li, U. Zakir, X. Yan, A. Shah, U. Sudhakr, B. Luo, Mufti Mahmud, M. Rajak |
2014 |
Intelligent CDS framework, Classifier |
||||
5. |
Abhay Krishan, Deptti Mittal |
2015 |
Contrast Limited Adaptive Histogram Equalization (CLAHE) and Constrained Variable Histogram Equalization (CVHE) algorithm, SVM classifier. |
||||
6. |
Saranya, S. and Dr. M. Pushpa Rani |
2016 |
CLAHE, FCM clustering algorithm |
||||
7. |
Pavushetty Yugandar G. Raghotham Reddy |
2017 |
Fuzzy c-means clustering (FCM) algorithm, distance regularized level set evolution (DRLSE) |
||||
8. |
Sukanta Sabut, Amita Das, U Rajendra Acharya |
2018 |
Marker-controlled watershed segmentation, Watershed Gaussian based deep learning (WGDL) technique, Gaussian mixture model (GMM) algorithm, deep neural network (DNN) classifier |
||||
9. |
Atrayee Dutta and Aditya Dubey |
2019 |
Ostus method, Marker-Controlled Watershed Segmentation |
||||
10. |
R Aarthi, S Nivetha, P Vikashini, Dr. V. T. Balamurgan |
2020 |
Anisotropic diffusion filters, Morphological operations |
CONCLUSION
[9] |
Atrayee Dutta, Aditya Dubey Detection of liver cancer using image |
||
REFERENCES |
processing techniques. International Conference on Communication |
||
[1] [2] |
Wassem Abdulrahman, Diagnosis of liver tumours Using Image Processing Vol.3 Issue 4, April -2014 Priyanka Kumar, Shailesh Bhalerao Detection of Tumor In Liver |
[10] |
R Aarthi, S Nivetha, P Vikashini, Dr. V.T. Balamurugan Liver cancer detection using image processing techniques Volume:7 Issue :03| Mar 2020. |
[3] |
Using Image Segmentation and Registration Technique volume9, Issue 2, Ver. VIII (Mar-Apr. 2014). PP 110-115. R. Rajagopal, P. Subbiah Computer Aided Detection of Liver Tumor |
[11] |
Amitha Raj, Jayasree M Automated Liver Tumor Detection Using Markov Field Segmentation Procedia Technology 24 (2016) 1305 – 1310. |
[4] |
using SVM Classifier An ISO 3297: 2007 Certified Organization) Vol. 3, Issue 6, June 2014. L. Ali, A. Hussain, J. Li, U. Zakir, X. Yan, A. Shah, U. Sudhakr, B. |
[12] |
Dr. K.A.M. Sajad Hyder Least Distance Based Liver Segmentation Techniques (LDBLST) For Liver Segmentation Form CT Scan Image Volume 119 No. 15 2018, 845-855. |
Luo, Mufti Mahmud, M. Rajak Intelligent Image Processing Techniques for Cancer Progression Detection, Recognition and Prediction in the Human Liver December 2014. |
[13] |
A. Bathsheba Parimala, R.S. shanmugasundaram Assessment on liver Disease Classification using Medical Image Processing Volume-8 Issue-5 January2020. |
[9] |
Atrayee Dutta, Aditya Dubey Detection of liver cancer using image |
||
REFERENCES |
processing techniques. International Conference on Communication |
||
[1] [2] |
Wassem Abdulrahman, Diagnosis of liver tumours Using Image Processing Vol.3 Issue 4, April -2014 Priyanka Kumar, Shailesh Bhalerao Detection of Tumor In Liver |
[10] |
R Aarthi, S Nivetha, P Vikashini, Dr. V.T. Balamurugan Liver cancer detection using image processing techniques Volume:7 Issue :03| Mar 2020. |
[3] |
Using Image Segmentation and Registration Technique volume9, Issue 2, Ver. VIII (Mar-Apr. 2014). PP 110-115. R. Rajagopal, P. Subbiah Computer Aided Detection of Liver Tumor |
[11] |
Amitha Raj, Jayasree M Automated Liver Tumor Detection Using Markov Field Segmentation Procedia Technology 24 (2016) 1305 – 1310. |
[4] |
using SVM Classifier An ISO 3297: 2007 Certified Organization) Vol. 3, Issue 6, June 2014. L. Ali, A. Hussain, J. Li, U. Zakir, X. Yan, A. Shah, U. Sudhakr, B. |
[12] |
Dr. K.A.M. Sajad Hyder Least Distance Based Liver Segmentation Techniques (LDBLST) For Liver Segmentation Form CT Scan Image Volume 119 No. 15 2018, 845-855. |
Luo, Mufti Mahmud, M. Rajak Intelligent Image Processing Techniques for Cancer Progression Detection, Recognition and Prediction in the Human Liver December 2014. |
[13] |
A. Bathsheba Parimala, R.S. shanmugasundaram Assessment on liver Disease Classification using Medical Image Processing Volume-8 Issue-5 January2020. |
In this paper discussed about various image processing techniques. These techniques are used to detect the liver tumor from the CT/MRI image. After data collection, pre-processing starts which can be used to reduce the noise and to increase the contrast of the image using different method. Filters are used for noise removal. The image enhancement, segmentation and classification is done by using different algorithms. Automatic identification of liver tumour helps doctors for better treatment.
-
Abhay Krishan, Deptti Mittal Detection and classification of liver cancer using CT image ISSN: 2349-794,093 098 Volume: 2 Issue: 5 IJRMEE | May 2015, May 2015.
-
Saranya, S, Dr. M. Pushpa Rani Liver tumour detection for CT images using image processing techniques Research Vol. 8, Issue, 06, pp.32426-32429, June 2016.
-
Pavushetty Yugandar, G. Raghotham Reddy Liver Tumor Segmentation in Noisy CT Image Using Distance Regularized Level Set Evolution Based on Fuzzy C-Mean Clustering May 2017.
-
Sukanta Sabut, Amita Das, U Rajendra Acharya Deep learning base liver cancer detection using watershed transform and gaussian mixture model techniques, Dec 2018
and Signal Processing, April 4-6, 2019, India.