
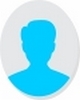
- Open Access
- Authors : Ahmad Bandiya Jega , Ran Vijay Kumar Singh
- Paper ID : IJERTV10IS080078
- Volume & Issue : Volume 10, Issue 08 (August 2021)
- Published (First Online): 21-08-2021
- ISSN (Online) : 2278-0181
- Publisher Name : IJERT
- License:
This work is licensed under a Creative Commons Attribution 4.0 International License
A Separate Exponential Ratio-Type Estimator of finite Population Mean under Power Transformation
1Ahmad Bandiya Jega and 2Ran Vijay Kumar Singh 1,2Kebbi State University of Science and Technology, Aliero, Nigeria
Abstract: In this paper, a separate ratio-type exponential estimator for estimating the finite population mean has been proposed. Mathematical expressions for the bias and mean square error (MSE) of the proposed estimator have been derived to the first order of approximation. Theoretical conditions have been obtained under which the proposed estimator is more efficient than the estimators under study. Numerical illustration has also been carried out to compare the efficiency of proposed estimator and found that the proposed estimator was more efficient.
Keywords:- Bias, Exponential estimator, Mean square error, Separate estimator, Stratified sampling.
-
INTRODUCTION
The problem of estimation of population parameters has been an important issue in sample survey and many methods have been used in order to improve the efficiency of the estimators. In survey sampling, it is well established that the use of auxiliary information results in substantial gain in efficiency over the estimators which do not use such information. In some cases, in addition to mean of auxiliary variable, various other parameters related to auxiliary variable such as coefficient variation, correlation coefficient etc are used to estimate the population parameter. Sisodia and Dwivedi (1981), Rao (1991), Upadhayaya and Singh (1999), developed various estimators to improve the ratio estimators in simple random sampling. Kadilar and Cingi (2003) modified the various estimators under stratified random sampling. Bahl and Tuteja (1991) introduced ratio and product- type exponential estimators which perform better than the classical ratio and product estimators respectively. Singh et al. (2008) proposed a ratio and product-type exponential estimators which were more efficient than the Bahl and Tuteja (1991) estimators. Upadhyaya et al. (2011), Singh and Ahmed (2014), Singh and Ahmed (2015a, 2015b), Singh (2016) did remarkable work in this direction. Singh et al. (2018) suggested combined ratio-type exponential estimator of population mean which was equally efficient as combine linear regression estimator.
Let U U1,…..,UN
-
NOTATIONS
be a finite population of size N which are partitioned into K distinct strata with ith
K
stratum
containing
Ni units i 1, 2….., k such that Ni N . Let a sample of size ni units i 1, 2,…., k
i1
be drawn from
k
k
the population using simple random sampling preferably without replacement (SRSWOR). Such that ni n .
i1
Let y x
be the observed values of Y , X
on the
jth
unit of the
ith
stratum j 1, 2,…, N
Moreover, the
ij , ij
1 Ni
i
1 Ni
population means of the variables Y and X in the ith stratum are Yi
Yij , Xi
N
N
i j1
Xij and the corresponding
N
N
i j1
1 ni
1 ni
sample means of the variable Y and X in the ith stratum are
yi
yij
n
n
i i1
, xi
xij
n
n
i i1
respectively.
Estimators of the variable Y and X , in stratified random sampling are given by;
k k k
yst Wi yi i1
k
and
xst Wi xi
i1
are the unbiased estimators of the population means
N
Y WiYi
i1
and
X Wi Xi
respectively. Where Wi
i
N
denotes the stratum weight.
i1
Let be the correlation coefficient between the study variable and the auxiliary variable.
-
EXISTING ESTIMATORS
The separate ratio estimator for the population mean Y is defined as;
k yi
ysr Wi x X i
(1)
i1 i
and its bias and MSE are given as;
k 1 2
B ysr Wii
i1
Ri Sxi SYXi
Xi
MSE y
k
W 2 S2 R2S2 2R S
(2)
sr i i yi i xi i YXi i1
The separate product estimator for the population mean Y is define as;
k yi . xi
ysp Wi X
(3)
i1 i
and its Bias and MSE are given as;
k 1
B ysp Wii
i1
SYXi
X
X
i
MSE y
k
W 2 S2 R2S2 2R S
(4)
sp i i yi i xi i YXi i1
The separate linear regression estimator for the population mean Y is defined as;
k
k
y W y
-
b X xi
(5)
sl i
i1
i i i
And its MSE is given as;
MSE( y
) W 2 S 2
1 2
(6)
k
k
sl
i1
i i Yi YXi
-
-
ADAPTED ESTIMATORS
Bahl and Tuteja (1991) ratio-type exponential estimator for the population mean Y under stratified random sampling can be defined as:
k X i xi
ystBTR Wi yi exp X
-
x
(7)
i1
i i
its bias and MSE are given as;
Bais y
k
W 1
3 R S 2
1 S
stBTR i i X i 8
i Xi
2 XYi
MSE y
i1
k
W 2 S2 1 R2S2 R S
(8)
stBTR i i yi 4 i xi i YXi
i1
Bahl and Tuteja (1991) product-type exponential estimator for the population mean Y under stratified random sampling can be defined as:
k xi X i
(9)
ystBTP Wi yi exp x X
and bias and MSE are given as;
i1
i i
k 1 1 1
Bais y
W S R S 2
i1
i1
stBTP i i X i 2 XYi 8 i Xi
MSE y
k
W 2 S2 1 R2S2 R S
(10)
stBTP i i yi 4 i xi i YXi
i1
Singh et al (2018) estimator
y
y
A combined ratio-type exponential estimator for population mean under stratified random sampling using information on single auxiliary variable has been suggested by Singh et al ( 2018) given as:
y
x
st
st
S st
S st
exp X x
st
where is a constant. (11)
X X xst
Bias and MSE of tS are obtained as
2 1 1
4 2 8 3 1
B yS Y
COV yst xst
2 V xst
2 XY
k
2 12
8 X
4
4
MSE y W 2 S 2
R2 S 2
2 1 RS
S
i1
i i Yi
Xi YXi
For the optimum value of , the Minimum MSE of tS is obtained as
k 1 1
2 2 *2
MSE yS
i1 ni
-
Wi S Yi 1
Ni
YX
(12)
That is the MSE of combined linear regression estimator.
-
-
THE SUGGESTED ESTIMATOR
In stratified random sampling, we suggest a separate ratio-type exponential estimator as
K x
i
X x
i
i
i
i
t W y
i i
i i
exp i
P
i1
X i X i xi
(13)
Where i is a constant.
-
BIAS AND MEAN SQUARE ERROR OF THE SUGGESTED ESTIMATOR In order to obtain the Bias and MSE to the first order of approximatin, let us define
yi Yi (1 e0i ) and
Therefore
E(e0i ) E(e1i ) 0
xi Xi (1 e1i )
(14)
1 1
S 2
E(e2 )
Yi
0 n N Y 2
(15)
i i i
1 1 S 2
E(e2 )
-
Xi
1i n N X 2
Now, by substituting the values of xi and y from (14) into (13) and on solving, the
i i i
1 1 S
i
bias of proposed estimator is obtained as;
E(e0ie1i )
-
YXi
K 1 1 1
4 2 8
3
2
1
ni
Ni Yi Xi
Bais t
W
i
i
i i R S 2
i S
P
(16)
i1
ni
Ni Xi
8 i Xi
2 YXi
Now, MSE of the proposed estimator tP can be obtained by squaring, simplify up to the first order of approximation and taking the expectation we will have;
K 1 1 2 12
MSE t
W 2 S 2 i R2 S 2
Yi
Yi
2
1 R S
(17)
P i
i1 ni Ni
4 i Xi i i YXi
To obtain the optimum mean squared error,
MSEtP 0 gives 1 iCYi
i
iopt 2
CXi
and minimum MSE of tP is obtained as
k 1 1
2 2 2
min
min
MSE tP
i1 ni
-
Wi SYi 1 i
Ni
(18)
That is the MSE of the separate linear regression estimator.
-
-
THEORETICAL EFFICIENCY COMPARISON
In this section, conditions have been found under which the proposed estimator is more efficient than existing estimators under study.
-
Comparison of the separate ratio estimator with the proposed estimator
MSE tP MSE ysr if and only if
3
C 1 3
C 1
min
2i Yi
i max
2i Yi
(19)
2
CXi ,
2
2
CXi ,
2
Therefore the proposed estimator is more efficient than
ysr
if condition (19) is satisfied.
-
Comparison of the separate product estimator with the proposed estimator
MSE tP MSE ysp if
3 1
min 2
CYi
max 3 1 2
CYi
(20)
2, 2
i C i
2, 2
i C
Xi
Xi
Therefore the proposed estimator is more efficient than
y sp
if condition (20) is satisfied.
-
Comparison of the Bahl and Tuteja (1991) ratio-type exponential estimator under stratified random sampling with the proposed estimator
MSE tP MSE ystBTR
min 0,1 2
CYi
max 0,1 2
CYi
(21)
i C i i C
Xi Xi
Therefore the proposed estimator is more efficient than
ystBTR if condition (21) is satisfied.
-
Comparison of the Bahl and Tuteja (1991) product-type exponential estimator under stratified random sampling with the proposed estimator
MSE tP MSE ystBTP
min1, 2
CYi
max1, 2
CYi
(22)
i C i i C
Xi Xi
Therefore the proposed estimator is more efficient than
ystBTP if condition (22) is satisfied.
-
-
NUMERICAL EFFICIENCY COMPARISON
For numerical illustration the data of Kadilar and Cingi (2003) is used which is given in table 1. Y represents apple production amount (as variable of interest) and X represents number of apple trees (as auxiliary variable) in 854 villages of Turkey in 1999 (Source: Institute of Statistics, Republic of Turkey). The data is stratified by regions of Turkey and from each stratum (region) the samples (villages) are selected by using Neyman allocation.
Table 1: Data Statistics
Ni
ni
X i
Y i
SXi
SYi
i
i
W 2
i
Stratum1
106
9
24375
1536
49189
6425
0.82
0.102
0.015
Stratum 2
106
17
27421
2212
57461
11552
0.86
0.049
0.015
Stratum 3
94
38
72409
9384
160757
29907
0.90
0.016
0.012
Stratum 4
171
67
74365
5588
285603
28643
0.99
0.009
0.04
Stratum 5
204
7
26441
967
45403
2390
0.71
0,138
0.057
Stratum 6
173
2
9844
404
18794
946
0.89
0.006
0.041
Table 2: Theoretical comparison supported numerically using data set provided in Table 1
MSE tP MSE ysr
1 = -1.1997
-1.8994 < -1.1997 < -0.5
Satisfied
2 = -1.6433
-2.7866 < -1.6433 < -0.5
Satisfied
3 = -0.7920
-1.0839 < -0.7920 < -0.5
Satisfied
4 = -0.8213
-1.1426 < -0.8213 < -0.5
Satisfied
5 = -0.5220
-0.5439 < -0.5220 < -0.5
Satisfied
6 = -0.5916
-0.6831 < -0.5916< -0.5
Satisfied
MSE tP MSE ysp
1 = -1.1997
-3.8994 < -1.1997 < -1.5
Satisfied
2 = -1.6433
-4.7866 < -1.6433 < -1.5
Satisfied
3 = -0.7920
-3.0839 < -0.7920 < -1.5
Satisfied
4 = -0.8213
-3.1426 < -0.8213 < -1.5
Satisfied
5 = -0.5220
-2.5439 < -0.5220 < -1.5
Satisfied
6 = -0.5916
-2.6831 < -0.5916 < -1.5
Satisfied
MSE tP MSE ystBTR
1 = -1.1997
-2.3994 < -1.1997 < 0
Satisfied
2 = -1.6433
-3.2866 < -1.6433 < 0
Satisfied
3 = -0.7920
-1.5839 < -0.7920 < 0
Satisfied
4 = -0.8213
-1.6426 < -0.8213 < 0
Satisfied
5 = -0.5220
-1.0439 < -0.5220 < 0
Satisfied
6 = -0.5916
-1.1831 < -0.5916 < 0
Satisfied
MSE tP MSE ystBTP
1 = -1.1997
-3.3994 < -1.1997 < 1
Satisfied
2 = -1.6433
-4.2866 < -1.6433 < 1
Satisfied
3 = -0.7920
-2.5839 < -0.7920 < 1
Satsfied
4 = -0.8213
-2.6426 < -0.8213 < 1
Satisfied
5 = -0.5220
-2.0439 < -0.5220 < 1
Satisfied
6 = -0.5916
-2.1831 < -0.5916 < 1
Satisfied
Table 3: MSE and PRE of the Proposed Estimator with other estimators
ESTIMATORS
MSE
PRE
yst
673477.7
100
ysr
159137.4
423.2
y sp
1790757
37.6
ystBTR
340940
197.5
ystBTP
1156750
58.2
yS
202122.1
333.2
tp
107065.8
629
-
CONCLUSION
In the present study, Singh et al (2018) estimator has been improved by modifying it as separate ratio-type exponential estimator and its properties have been studied. Theoretical conditions have been obtained under which the proposed estimator is more efficient than the estimators under study and supported numerically as mentioned in table 2. Table 3 reveals that the proposed
estimator tp
has smallest mean square than the conventional ratio, product and Bahl and tuteja (1991) estimators under stratified
random sampling as well as Singh et al (2018) estimator. Therefore, tp
estimating the finite population mean.
REFERENCES
is more efficient than the other existing estimators for
-
Bahl, S. and Tuteja, R.K. (1991). Ratio and product type exponential estimator. Journal of information and Optimization Sciences, 12(1), 159- 163.doi:10.1080/02522667.1991.10699058
-
Kadilar C. and Cingi H. (2003). Ratio estimators in stratied random sampling, Biometrica Journal. 45, pp . 218-225.
-
Rao, T. (1991). On certain methods of improving ratio and regression estimators Communications in Statistics-Theory and Methods 20(10), 33253340.
-
Singh, R., Chauhan, P. And Sawan, N. (2008).On linear combination of ratio-product type exponential estimator for estimating finite population mean. Statistic in transition, 9 ,105-115.
-
Singh, R. V. K. and Ahmed, A. (2015). Improved Exponential Ratio and Product Type Estimators for Finite Population Mean International Journal of Engineering Science and innovative Technology, 4(3) , 317-322.
-
Singh, R. V. K. and Ahmed, A. (2015). Improved Exponential Ratio and Product Type Estimators for Finite Population Mean Under Double sampling Scheme. International Journal of Scientific & Engineering Research, 6(4), 509-514.
-
Singh, R. V. K. and Ahmed, A. (2014). Ratio Type Estimators in Stratified Random Sampling Using Auxiliary Attributes IAENG Conference IMECS, 389-393.
-
Singh, R. V. K. (2016). Study of predictive approach product type estimator under polynomial regression model. International Journal of Scientific & Engineering Research, 7(1), 667-671.
-
Singh, R.V. K., Mariam, M. and Sifawa, A. (2018). Combined exponential ratio-type estimator for estimating population mean. 37th Annual conference, Nigerian Mathematical Society, 8th -11th May 2018, Bayero University, Kano.
-
Sisodia, B. V. S., and Dwivedi, V. K. (1981). A modified ratio estimator using coefficient of Variation of auxiliary variable. Journal of the indian of Agricultural Statistics,33(2),13-18
-
Upadhyaya, L.N. and Singh, H. P. (1999).Use of transformed auxiliary variable estimating the finite population mean. Biometrical Journal.41 (5), 627-636.
-
Upadhyaya, L.N., Singh, H.P., Chatterjee S., and Yadav, R. (2011). Improved ratio and product exponential type estimators. Journal of Statistical Theory and Practice,5(2): 285-302.