
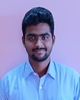
- Open Access
- Authors : Yash Bhandari , Mrunal Ingale , Mayur Jagtap , Avi Raghuvanshi, Pallavi Dhade
- Paper ID : IJERTV10IS120133
- Volume & Issue : Volume 10, Issue 12 (December 2021)
- Published (First Online): 22-12-2021
- ISSN (Online) : 2278-0181
- Publisher Name : IJERT
- License:
This work is licensed under a Creative Commons Attribution 4.0 International License
A Survey on Precision Agriculture: Crop Health Monitoring and Protection
Yash Bhandari, Mrunal Ingale, Mayur Jagtap, Avi Raghuvanshi, Prof. Pallavi Dhade
PCET's
Pimpri Chinchwad Engineering College of Engineering, Pune
Abstract : Precision Agriculture has been playing a significant role in the refinement of plants' health. It ensuresthat crops and soil receive exactly what they need for optimum health and productivity. The term also involvesminimizing pests, unwanted flooding, and disease.This paper gives a review on various plants protection andmonitoring techniques. Authors mentioned here have proposed different solutions like use of drone technologiesand deep learning methods. Deep learning has been playing a significant role in identifying the diseases in plants. Algorithms like VGG-net, Res-net,CNN and image processing techniques have proved to be significant inrecognizing many plant diseases.
Keywords: Precision agriculture, Quantized model, Deep learning, Drones technology, Agricultural Rover, ImageProcessing
-
INTRODUCTION
Due to increasing population,demand for production as well as consumption of food has also increased.In most extreme situations, plant distempers might engender no crop wholly. So, the automated identification of the plant diseases is considerably significant in the domain of farming. Smart husbandry will enhance IoT in agro-based products exporting countries. Crop Protection during growth of crops and after the culture of crops is a really important process for the growers.From multiple spaces it has been observed that there's huge bulk of loss in the yield of farming products due to different parasite attacks or unforeseen climate changes.With the hyping demands of the food product, these losses aren't affordable. There's an imperative need to reduce these losses.To eliminate this, authors have come up with few solutions on creating an automated system which will be efficient in identifying the crops attacked by various threats.
Plant disease detection can be achieved successfully using deep learning. Deep learning has played a very significant role in identifying plant defects or diseases. Deep learning techniques have proven to be a strong tool as it has the capacity to handle an immense amount of datasets which improves the chances for better detection. Deep Learning algorithms like CNN.With the help of image processing we can use CNN for recognition of various patterns in the dataset. CNN is quite adaptable and has a less complex structure than other algorithms. It also requires a lesser number of parameters for training.
Drone technology is one of the emerging technologies for achieving the precision agriculture objectives. The drones are used to optimize agricultural operations. Drones are self-operating machines which do not require any human on board, they can be controlled using a remote control or by training them with some programming instructions.The applications of drones in agriculture includes capturing images of the crops with the help of a camera attached to it.
The images obtained can be further processed to check whether crops are affected by any of the plant diseases.Also the drones help in identifying the locations of parasites on the crop and prevent it from getting affected by spraying pesticides on them. The drones can regularly monitor the crops and safeguard them from animals and thieves. Hence use of drones in agriculture increases the efficiency of the farmers and reduces their efforts.
-
CONVENTIONAL AGRICULTURAL SETBACKS
Farming has been one of the initial factors in establishing human civilization for more than 10,000 years. Back then only minimum handy tools were used for activities such as harvesting, sowing, etc. Today after thousands of years we have advanced immensely in terms of technology as well as lifestyle. With technical advancement, a growing population is also being witnessed
,hence, demand for food supply has increased drastically. In such a fast growing era it becomes even more important to monitor the agricultural sector than before, because even a single problem in a crops seasons due to any threat can affect the supply. Therefore it becomes very important to have a smart agricultural system for monitoring crop yield and prevent it from any mishap.
Thus, by implementing the following methods suggested by the authors conventional methods can be improved:-1.By developing smart applications which can capture images of crops and analyse their health conditions.
Fig 1:Android applications for prediction of crop diseases.
-
Using drones , which will be monitoring the whole field without human intervention and help in detecting the plants affected bypests and sprinkle pesticides over the affected area.
FIGURE 2: Agricultural Drone Technology
-
Using wireless rovers for examining the soil conditions, and also useful for identifying creepers which damage the crops.
-
Using various AI sensors for keeping track of the moisture content of the soil and notifying us using an email system whenthere's a deficiency.
In this paper,we have provided the analysis of techniques and how smart applications such as drones can be used for earlier detection of plant diseases and hence protect them from getting affected.Along with this, how regular crop monitoring techniquescan help in the effective growth are also reviewed.
-
WORK DONE BY RESEARCHERS
The authors in this paper [1], proposed a potent Deep Learning method for identification of various crop diseases.This is done by using a library built in PyTorch called fast.ai for implementation of Residual neural networks.A trained model on PyTorch, is inserted on a mobile application for detection of diseases in various crops.Finally, a mobile app named dCrop is developed for detecting diseases by capturing the images of leaves of crops.This app will be running on internet connectivity and enable the feature of taking live pictures of the leaves on the spot by the farmers and have user-friendly interface for ease of its use.
The authors of the paper [2], propose an application based on Deep Learning . A rigorous quantitative analysis was done by comparison of five different Deep Learning models to find the best fit. These models were compared by their characteristics based on correctness, space of disc used, total time for interpretation, and desolation of energy. The authors suggested an android app, named as Deep Leaf made using STM32 microcontroller, for diseases detection in plants, using Q- CNN model, which resulted to be the best fit model.The models were complex and hence consumed time while doing the comparative study based on different parameters. After much study, the Quantized CNN model outperformed every time with higher accuracy rate than others.For testing and validation of the Deef leaf, Smart Edge platform was used. The application was quite successful in correctly classifying the coffee plants health condition with accuracy of 96 percent.
The authors in this paper [3], put forward spectra of graphic images of barley plant leaves for identifying the degree of damage caused. This was done with the help of an AI system called Edios.The proposed system analysed the spectra of graphic imagesof the leaves by the spots on them to form generalized spectra of classes. The extraction of the information was done from the distribution of the colours represented by the images. Using this image analysis method we can avoid errors caused by humans and hence this can surely reduce the labour cost and a lot of time can be saved in doing the rapid assessment of the data providedfrom the fields.
The authors in this paper [4], come up with the concept of using a pre-trained dataset and inserting or fitting it in the particular task trained by our own model.In this method, pre-trained VGGNet module is tagged.By this method we can avoid training of
themodel from scrape.This model was able to successfully able to give an accuracy of about 92% even in complexities in the conditions of the background.
The authors in this paper[5], have proposed self operating tactics for fighting against the parasites and safeguarding the crops from them. In this method, authors have suggested the use of drones for detecting the location of parasites on the crops affected by parasite attacks and also spray the pesticides on them by the pesticide spray mounted on the drone. The aim of this ideology is to reduce the losses caused to the farmers due to crops affected due to parasite attacks. There is a team of drones which cover all the areas of the field. The drones are used for capturing the images of crops which are affected. To traverse over the field and detect the location of parasites the author suggested two parasite search algorithms.The team of drones coordinate with each otherby exchanging messages. They share the details of the areas covered to each other and update the map on the cloud to ensure whether all the areas of the farm are covered. Also they detect the plants where pesticides are to be sprayed. Hence, the real time monitoring of the crops can be achieved to fight against parasites.
The author in this paper[6], have proposed the methods to be used to establish a proper communication between the drones.The coordination between the drones is an important factor as it increases the efficiency of the tasks to be performed by drones.As the drones consumes lot of energy so it is necessary that no energy is wasted due to lack of communication between the drones.Also the drone when requires help from other drones they communicate by posting requests on the net and hence the nearest drone with required help can be replaced by the drone which have posted a request.The coordination strategies suggested by authors are flooding base recruitement and bio-inspired recruitment strategy. These strategies were compared on the factors like amount of energy consumed and number of parasites killed.It was concluded that bio-inspired strategy is superior then the flooding base method.
The author in this paper[7], have proposed an advanced method for real time monitoring of plants with help of drones.the design of drones suggested by authors consists of three types of imaging modes (i.e multispectral, thermal and optical).The images obtained from the drones are converted into various forms to extract diagnostic information from the crops.With the help of the data obtained, datasets for testing where created including the factors like chlorophyll, nitrogen content, soil carbon content,photosynthetic capacity.this dataset was further processed using some optimum algorithms and the factors which are in deficient amount and required for the crops where determined.Hence this method helps in achieving the smart farming practices.
The author in the paper[8], have proposed a ideology for monitoring and predicting the growth features of plants.In this method, the data is obtained in the form of images which are captured by drones, also the data is collected from various IOT sensors.This data is then further processed using some lightweight machine learning algorithms.The output from these algorithms is used to determine the growth dynamics of the plant depending on factors like leaf shape,leaf size etc.Also the optimal growing conditions required by the plants can be determined from the data collected from sensors like soil moisture, temperature, water content etc.This technique is nothing but an embedded sensing technique used to predict the growth dynamics of plants and monitor the growth.
The authors in this paper [9] have found a great way to detect weeds among crop plants using UAV (Unmanned Armed Vehicles) with high resolution and multihyper-spectral cameras and GIS technology. It is done by using image processing techniques. The problem of effective weed management is solved due to early detection of weed plants. The UAV is mainly used here to store, process and analyze imagery of weed plants at different stages of crop plant growth.
The authors in this paper [10] have developed a way to cure diseased crop plants using the latest technologies. It is basically a type of smart spraying system which releases agrochemicals on the affected area in the crop plants using image processing techniques. We found this method really useful because it reduced the amount of chemicals to be applied by almost 33%. The proposed model is a vehicle with machine-vision based sprayer which applies chemicals only to the affected area and also with the variable rate.
The authors in this paper [11] developed a low-cost weed management system using AI technology. The proposed model here hasthe ability to distinguish crop plants from weed plants and accordingly can treat them using a spraying system. The neural networks are used here for target detection. The system contains various units like real time kinematic GPS, video cameras, pump, nozzle, a smart controller,etc. For weed detection, they used video cameras, and based on the results received, the control unit manipulates the spraying system on affected areas.
The authors in this paper [12] developed an easy-to-deploy prototype using embedded systems and artificial intelligence. The main plant characteristics used here to analyze plant growth is the leaves area. They used RNN to analyse dynamic plant growth. The developed prototype is a testbed with self driven image acquisition system coupled with controlled LED illuminations. The raw images of leaves were taken as training data for NNs and other machine learning algorithms. This prototype can perform about 8600 continuous predictions in single battery charge lasting upto 4 hrs.
The authors in this paper [13] have used MRC technique for wireless power transfer to charge drones through a base station for the observation of the agriculture fields for yielding crops and to check climatic states and to expand the reach of communication for WSN in the areas.WPT is used in this as it gives high power transfer rate and increased efficiency with 1 meter of transfer range.WPT used a solar cell. At different distances, the multi turn coil (MTC) was planted in the receiver circuit (drone) and the coil was planted in the transmitter circuit. Efficiency of power transfer was improved to greater amounts for 0 cm. Proposed active/sleep strategy in the mentioned operation, the drones battery life increased to 14 hrs.
The authors in this paper [14] have used a smart irrigation method for protection and health monitoring of crops using self- operating rovers. Irrigation of crops is done by the water sprinklers taking in consideration the moisture level in soil with the help of sensors which detect the soil moisture, humidity, temperature and water level sensor. Agricultural self-operating rovers locomotes around the field to collect data from cameras fixed on them for health overseeing. The images collected by the rover are processed in MATLAB to identify if the differences in plants are because of nutrient deficiency or due to damage done by a distemper causing component. In case of fire accidents, UV flame sensors are used for detection of fire and even extinguishing it.Hence the field is protected from animals with the help of a buzzer.
The authors in this paper [15] have used a smart way for production of crops using IoT and machine learning methods. Smart agriculture management system that helps farmers to raise crop production. The system can help in minimizing the excessive waste produced by resources if precision agriculture methods are used . This system uses different sensors lie moisture sensor, DHT-11, hygrometer, and for the collection of data for analyzing many factors required for production crops. Data from the sensor is obtained and then visualized using graphs.Temperature is shown on the temperature gauge,and is plotted with the helpof different MATLAB tools. The accuracy of the model trained was around 97%.
-
ANALYSIS OF REVIEW:-
Table 1 provides us a short summary of the techniques used,their pros and cons given by different researchers for crop monitoringand protection.
Table 1:Analysis and review of existing schemes
Reference
Year
Technology used
Contributions
Advantages
Shortcomings
V. Pallagani,et al.[1]
2019
PyTorch library,fast.ai
Deep learning model used for prediction ofcrop disease .
Android app was ableto detect 38 different diseases
Cannot predict withoutinternet connectivity.
F. de Vita et al[2]
2020
(Q-CNN) model
Use of Deep leaf for detection of diseases incoffee plants
Accuracy upto 96%,reducing footprintsof NN,Maximized performance on
constrained devices
Limited to disease prediction in coffeeplants only
Eugeny V. Lutsenko et al.[3]
2020
Edios system for measuring spectraof graphic images
System allows to precisely defined thecolors present in the image
Reduction in errors
Incorrectness in data
Junde Chen etal.[4]
2020
Deep CNN
Transferred learning for detection of plant leaf disease
High accuracy
Uneven illumination caused errors in features extraction
Giuseppe Potrinoet al.[5]
2018
Random and Distributed Parasite search algorithms
Detection of the locationof parasites on the crops with help of Drones
Protection of crops from parasite attack.
Coordination between theteam of drones.
Mauro Tropea etal.[6]
2019
Flooding base andbio- inspired recruitment strategies
Selection of best drone recruitment strategy to establish proper
connection between thedrones
Increases the performance of dronesand also the efficiency to fight against pests
Energy consumed by thedrone is high.
Yoshio Inoue etal.[7]
2020
Plant stress indicator (spectralindices)
With help of diagnostic information the plant requirements are known
The factors which are deficient for growth ofcrops are determined and hence provided
with sufficient amount
As number of imaging modules are used higher battery life and high
amount of storage isrequired
Sergey Nesteruket al.[8]
2020
Embedded sensing
An embedded system for remote sensing and prediction of growth dynamics
The growth of plants is monitored.
Complex design and requires lot of hardwarecomponents
Eihab Fathelrahman etal.[9]
2019
UAV, GIS
Detecting weeds in cropplants using UAV and GIS system
It allows farmers to keep an eye on weeds atvarious geographical
areas under variousweather conditions.
The power system of theUAV vehicle offers limited flight time.
V.K.Tewari etal.[10]
2019
Image processing
Automatic spraying system using imageprocessing
The proposed system reduced chemical usageupto 33%
Due to plant spacingdeviations, it carriers some errors in image capturing
Victor Partel etal.[11]
2018
Artificial Intelligence
Smart spraying systemusing AI
It is a smart and precision weed mapping and spraying system
The sprayer missed sometargets on the right side
at time of operation
Shadrin et al.[12]
2019
Artificial Intelligence, Precision
Agriculture
Embedded system for continuous analysis of plant growth
It is autonomous toolfor continuous monitoring upto 6 months
Limited RAM and cache memory has negative
effect on powerconsumption
G. S. Nagaraja et al.[13]
2019
IOT and Machine Learning
Use of various sensorsfor data acquisition
which helps in analyzingall the environmental factorsnecessary for the
cultivation of crops.
Helps the user to monitor and analyze thecrops health based on the factors such as temperature, humidity, moisture, etc.
There could be some enhancements done
regarding plants health monitoring based on thefeatures of crop leaves,
Gobhinath S etal.[14]
2019
Autonomous Agricultural Rover
Develop a smart irrigation system using rover for monitoring andsensors for field protection
The rovers help in keeping an eye on the field for sending data todetect disease of leaves.Many sensors help protect the field from fire and from animals.
The rover needs to be charged from time to time.
A.M. Jawad, etal.[15]
2019
wireless power transmission
magnetic coupling forwireless power transmission a base station
Increases the battery life of the drone to 14 hours, the battery powersaving observed was 96.9%
Power consumption is increased by flight time.
-
CONCLUSION
This paper provides a review on various plant health monitoring and protection techniques.Plants' poor health conditions can lead to lower productivity and can fail to meet the increasing demands for food.This problem can be resolved using Deep learning applications.Variety of plants datasets have been used by the authors and they have obtained successful results with good accuracy rate.Hence, it can be concluded from the survey that,plant health monitoring and protection techniques help in early detection of plant diseases, thus increase the agricultural yield.
-
REFERENCES
-
-
V. Pallagani, V. Khandelwal, B. Chandra, V. Dhulapally, D. Das and S. P. Mohanty, "dCrop: A Deep-Learning Based Framework for Accurate Prediction of Diseases of Crops in Smart Agriculture," 2019 IEEE International Symposium on Smart Electronic Systems (iSES) (Formerly iNiS), Rourkela, India, 2019, pp. 29-33, doi: 10.1109/iSES47678.2019.00020.
-
F. de Vita, G. Nocera, D. Bruneo, V. Tomaselli, D. Giacalone and S. K. Das, "Quantitative Analysis of Deep Leaf: a Plant Disease Detector on the Smart Edge," 2020 IEEE International Conference on Smart Computing (SMARTCOMP), Bologna, Italy, 2020, pp. 49-56, doi: 10.1109/SMARTCOMP50058.2020.00027.
-
Eugeny V. Lutsenko, Valery E. Korzhakov and Valery I. Loko Kuban state agrarian University named after I. T. Tribilin, 350004 Krasnodar, Russia, Adyghe State University, 385000 Maykop, Russia.
-
Junde Chen,Jinxiu Chen,Defu Zhang,Yuandong Sun,Y.A.Nanehkaran,School of Informatics, Xiamen University, Xiamen 361005, China,Spira Inc, Los Angeles,
CA 90032, USA
-
Giuseppe Potrino, Nunzia Palmieri, Venturino Antonello, Abdon Serianni, DRONES Support in Precision Agriculture for Fighting agaist Parasites. 978-1- 5386-5541-2/18/$31.00 ©2018 IEEE
-
Mauro Tropea, Abdon Serianni DIMES department, University of Calabria 87036 Rende (CS), Italy mtropea, a.serianni@dimes.unical.it. Bio-Inspired Drones Recruiting Strategy for Precision Agriculture Domain.978-1-7281-7343-6/20/$31.00 ©2020 IEEE
-
Yoshio Inoue, Masaki Yokoyama University of Tokyo, Tokyo, Japan Institute for Agro-Environmental Sciences, NIAES-NARO, Tsukuba, Japan. DRONE- BASED OPTICAL, THERMAL, AND 3D SENSING FOR DIAGNOSTIC INFORMATION IN SMART FARMING SYSTEMS AND ALGORITHMS. 978-1- 5386-9154-0/19/$31.00 ©2019 IEEE
-
Sergey Nesteruk, Dmitrii Shadrin, Vladislav Kovalenko, Antonio Rodr´guez-Sanchez,Plant Growth Prediction through Intelligent Embedded Sensing. 978-1- 7281-5635-4/20/$31.00 ©2020 IEEE
-
Fathelrahman, E.Neumann, E.Hussein, M.Jalil, A.Hassan, F.Dirir, A.Muhammad, S. (2019). Unmanned Aerial Vehicle Imagery and Manual Sampling for Weed Recognition and Measurements.
-
V.K.Tewari, Gurdeep Lal, L.K.Dhruw, C.M.Pareek, Naseeb Singh, Agricultural and Food Engineering Department, Indian Institute of Technology Kharagpur, West Bengal 721302, India. Division of Agricultural Engineering, ICAR Research Complex for NEH Region, Umiam, Meghalaya 793103, India.
-
Victor Partel, Sri Charan Kakarla, Yiannis Ampatzidis, Agricultural and Biological Engineering Department, Southwest Florida Research and Education Center,
University of Florida, IFAS, 2685 SR 29 North, Immokalee, FL 34142, USA
-
Shadrin, D.Menschikov, A.Somov, A.Bornemann, G.Hauslage, Jens & M.Fedorov (2019). Enabling Precision Agriculture through Embedded Sensing with Artificial Intelligence. IEEE Transactions on Instrumentation and Measurement, 11. doi:10.1109/tim.2019.2947125
-
Gopinath S , Devi Darshini M, Durga K , Hari Priyanga R, Smart Irrigation with Field Protection and Crop HealthMonitoring system using Autonomous Rover , IEEE Region 10 Symposium, 2019.
https://ieeexplore.ieee.org/document/8728468
-
G. S. Nagarajan, Avinash B Soppimath, T. Soumya, AbhinithA,Department of Computer Science and Engineering,R VCollege of Engineering, Affiliated to VTU, Bengaluru 560059, India
-
A.M. Jawad, Haider M. jawad, R. N,S.K.Gharghan, N.F. Abdullah , M.J. Abu-Alshaeer. Centre of Advanced Electronic and Communication Engineering, Faculty of Engineering and Built Environment, Universiti Kebangsaan Malaysia (UKM),Bangi 43600, Malaysia,Department of Computer and Communication Engineering, Al-Radain University College, Baghdad 10064, Iraq,Department of Medical Instrumentation Techniques Engineering, Electrical Engineering Technical College, Middle Technical University, Baghdad, Iraq Department of Statistics, Al-Radain University College, Baghdad 10064, Iraq.