
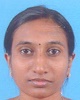
- Open Access
- Authors : I.Dhurga Bai, Dr.A.Selvapandian
- Paper ID : IJERTV13IS010058
- Volume & Issue : Volume 13, Issue 01 (January 2024)
- Published (First Online): 27-01-2024
- ISSN (Online) : 2278-0181
- Publisher Name : IJERT
- License:
This work is licensed under a Creative Commons Attribution 4.0 International License
A Survey Summary on Cervical Cancer Prodiagnosis and Diagnosis by Computer Aided Systems
I.Dhurga Bai
Assistant professor
Department of electronics and communication engineering Theni kammavar sangam college of technology
Dr.A.Selvapandian Assistant professor
Department of electronics and communication engineering Psna college of engineering and technology
Abstract
Medical care and services are expected by people now a days irrespective of expsnses. Medical image analysis are moving with challenges and interest as this analysis makes early detection of fatigue disease. Early detection helps preventing and curing the disease at right time. The medical related data are turning out as important data in prognosis of disease as image acquiring devices are packed with advanced technology . The storage and networking for human health related data becomes greater challenge. Medical image analysis creates impacts in field like ophthalmology,pathology,carcinomas etc. Deep learning is the derivative of machine learning and artificial intelligence.deep learning is preferred over machine learning and conventional pattern recognition or medical image interpretation to achieve good accuracy in diagnosis. Deep learning has different structural style in its architecture and some structural styles include convolutional neural network, deep auto encoder, deep neural network, deep belief network, .the deep learning needs more dataset for classification .the data sets used should be interoperable and so standardisation of image is needed.this deep learning method offers good accuracy ,sensitivity and specificity.deep learning finds application in medical health care sector and drug designing cervical cancer is promptly addressing high cancer-related deaths in women worldwide. Early detection of cervical intraepithelial neoplasia (cin) significantly improves patient survival.conventional screening for classification of cervical cancer types mainly relies on the experience of pathologists,colposcopists and they are all very limited in count and is an important factor in mortality.however, due to the increased human intervention, visual acuity testing leads to misdiagnosis and reduced diagnostic efficiency. Deep learning is getting revolutionized as data availability occupies large volume and also with deep learning models available. To get more worth
,organisation involving in maintaining health care records should coordinate ,collaborateand take steps to invest more on data. Privacy and legal limits medical image analysis accuracy and for no way have to abide hippaact. Deep learning based medical image analysis gives positive result as early disease detection is possible. The accuracy ,sensitivity and specificity of medical image analysis has to be improved by refining deep learning model and with accountable dataset and this paper highlights the machine aided diagnostic systems available for cervical cancer classification and prodiagnosis.
INTRODUCTION
Cervical cancer is the second deadliest threatening disease worldwide and pap smear is popular methods for of cervical cancer diagnosis. In growing nations like india, there are boundaries of econimally dipped areas where sufferers are increasing quitely. Computer visioned clinical evaluation offers many benefits in diagnosing human diseases. Clinical image evaluation is an area that has substantially benefited from machine learning to know to identify or classify. Cervical carcinoma occurs inside the cervix of women. The cervix is classed into types as type i, type ii, and type iii and diagnosing the proper sort of cervix determines the effectiveness of treatment. There are diffused variations among the three sorts. Figuring out the right type of cervix can consequently be a daunting task for health care experts.
About 528,000 new case emerges on each year and approximately 85% of recent patients emerges in developing nations. Cervical cancer screening includes pap smear test ,hpv test,colposcopic examination hpv(human papilloma virus )dna blended test and cytology are the primary steps for disease diagnosis.a colposcope is inserted to collect cervix cells and vaginal canal and cells areanalyzed for abnormality. Cytology outcomes are tough to interpretwithout an expert clinician. Hpv dna blended checking out gives result better than cytology results. Colposcopy is the next variety of visual inspection carried out with a colposcope (a medical magnifying microscopic equipment) andit sights the cervix closely to have better glance.only colposcopic clinicians can predict the abnormality in cervix accurately. Diagnostic rating is high however specialists are confined to small count.to conform the abnormality biopsy is prescribed and results are quite tough to interpret with limited cytologists. So computer aided device is needed to clear up the drawbacks. It represents the gold widespread for the fundamental evaluation of the presence of cervical most cancers.
Cervigrams may be histopathologically classified. Reaserches are prefering the path of applying deep learning to clinical diagnosis, in particularly cancers.convolutional neural networks (cnns) are an opportunity to traditional visual methods for
sickness diagnosis of lung, pores and skin, brain, prostate, breast cancers. Cnn has tactics for absolutely analyzing clinical image.early detection of the ailment is vital to reduce mortality rate. Detection and classification of cancer are important for effective disorder diagnosis . Deep learning is terrifically becoming an important in computer-aided diagnosis and becomes popular for pathological analysis of cervical intraepithelial lesions and invasive cervical cancer. A skewed oncologist-to-affected person ratio additionally exacerbates the problem as it of much surplus. Cervical cancer boom is connected to human papillomavirus infection. It has become a preventable ailment, accordingly minimizing the worldwide burden of cervical malignancy. Cervical cancer cells expand slowly inside the cervix. This slow progression of disease make as comfort to identify prior the disease and to get treatment in right time. Detection of precancerous abnormality (intraepithelial neoplasia) prevents risks of danger.as of 2018, who requires movement on cervical cancer.a aggregate of screening and diagnostic approaches dramatically decreased mortality rate from deadly cervical cancer . Therefore, there is a want for less expensive and extra automated screening methods that may be carried out, in particular in useful socio economic weaker regions.
Colposcopy is used to diagnose cervical cancer and this reduces the variety of fake positives. A constrainednumber of certified colposcopists reduce accuracy. To improve the accuracy of colposcopy examination, a computer diagnostic machine support is needed.for powerful screening and prognosis of cervical region cancers the usage of automatic diagnostic systems favours good result and categorizes cervical region cancers into normal, slight (early level ib), slight and intense (level iib) classes and subdivides most cancers location on cervigrams.deep learning is synthetic intelligence that has been applied to many regions in extraordinary fields, which includes healthcare and drug design. Accurate and well timed diagnostic and prognostic determinations are of fantastic advantage .
Computer aided diagnostic structures proves equivalent toexpert physicians with several achievements in making accurate diagnosis .evaluating cervical lymph node (cln) severity in benign and malignant categories performs an essential function in the treatment management of patients. Invasive pathological examinations such as biopsies asses the severity with the aid of mri is a painful and runs with significant processing time. Computerised tomography (ct) is the non-invasive radiation modality of diagnosis mode and widely used for the imaging assessment of all neoplastic sicknesses. Manual analysis is time consuming and complicated.this addresses the use of deep learning and machine learning in classifying human cancers.
DISEASE CLASSES OF CERVICAL CANCER
There are three neoplastic states have an effect on the cervix epithelium in the course of ailment development. Cervical intraepithelial neoplasia (cin) has three stages.the stage one is cin i and this is impose low abnormality and it could be cleared on its own way and will not cause much trouble to patients.the next stage of cin is cin ii and is moderate case which gets cleared on its own and it may be a precancerous changes.the high end stage is cin iii and this stage has highest abnormality cancerous stage and treatment is needed immediately to extend lifetime.cancer grows slowly and it may take more than ten years to fully collapse.
Fig 1: Normal and Abnormal Cervical Region CERVICAL CANCER DIAGNOSIS TESTS
Cervical cancer diagnosis is done by any one of the following clinical procedures.bimanual pelvic examination and sterile speculum examination in which speculum is inserted to vagina to have look into vaginal wall and cervix and samples are collected
.this also to view organs like uterus and ovaries.pap test is done by scratching vaginal regions to collect cells and this diagonise precancerous cells.human papillomavirustyping testis done with the same procedure undertaken for pap smear test.this test tells about thehigh risk viral infection in vagina and it bdoes not tell person have cancer and it inference the chance of cancer.colposcopy is the procedure to detect abnormal cells or cancerous cell present in vagina or cervix.biopsy is the procedure of removing cells for microscopic examinations.
Pelvic examination under anesthesiais done by making patient sedative and this will help for further surgical procedure.x ray by illumination of radiation shows the organs and pelvic bones for enlargement.ct scan test locate enlarged nodes and tells the size of abnormality and this helps in surgical process
LITERATURE SURVEY
Machine assisted execution in medial image analysis produces accurate result in prediction of final end results.machine assisted models includes two master domain.The outer enclosure is machine learning and inner enclosure is deep learning .Cervical cancer procasting severe risk on women and the disease contamination level builds slowly .The pretransfer phase could be marked early and immediately treated to fight against the disease at right time.Computerised diagnosis will avoid human intervention in diagnosis and provide atmost equivalent results predicted by expert clinicians.Many machine learning models and deep learning models are proposed to classify cervical cancer images.Researchers proposed and refined models to give better classification reports and validated their models outpetforms well.this review will portrait the machine trained models to classify the medical images.The review stratergy is adopted by filtering papers mainly between 2018 and 2020 and the paper sources electronically otaineed from standard journals and research articles. Two deep learning architectures for detecting cervical cancer from colposcopy images is proposed by Venkatesan
Chandranet.al.[1]. Thedeep learning architecture adopts VGG19 as transfer learning modelfor cervigram classification. Colposcopy Ensemble Network (CYENET) is another novel model developed to classify the cervical cancer from colposcopy cervigram images. Accuracy, specificity, and sensitivity are estimated for the developed models. VGG19 shows classification accuracy of seventy three percent.VGG19 relatively offers desired results.Kappa score polls moderate model for cervical cancer classification.CYENET model scores high interms of accuracy comparing with VGG19 and has remarkable accuracy of ninety two percent approximately.
Kelei Heaet.al[2]reviewed that within the discipline of clinical image analysis, transformers have additionally been successfully playing a vital role in full diversified process.The significant role of transformers lifts the medical image processing to another bright level.This explains the architecture of transformers and how it can be modified to suit for the meant applications and also evaluates the best and limitations.Analysed the key challenges in the area of using transformers in deep learning paradigms, enhancing version efficiency, and coupling with different techniques..
Eduardo Let.al[3] made cervical cancer classification with cytological images.women are affected widely with cervical cancer and this triggers the computer assisted diagnosis.The aitomatic screening models face drawbacks as clinical data are limited and it has to be ethically protected. Considering the drawbacks the new framework of cascading deep learning model RetinaNet for anomalous region detection and support vector machine for categorization. Complemented by using domain knowledge in their addressed classification and its design. Provides a set of nuclear-specific features that are segmented and whose impact on classification results is also examined. The model used are evaluated seperately for its efficiency and overall efficiency of the entire system is evaluated and the result shows improved results.
Deep learning paradigm and conventional machine learning paradigm are combined for brain tumor diagnosis by
Ebrahim Mohammed Senan et.al.[4].Alexnet followed by support vector machine (SVM) algorithms is used to feature detection and categorisation of brain tumors.similarly .Resnet followed by SVM is proposed as alternate to feature detection and classification of brain tumor. Magnetic resonance imaging of brain tumor is preprocessed with mean filter for enhancement.Alexnet and Resnet are the deep learning models to extract deep features and softmax layer is used to link support vector machine. The blended task improves efficiency .Comparitive analysis is made to forecast the performance analysis.The alexnet blended with SVM outperforms well in terms of training and testing time compared with resnet blended with SVM.The dataset used contains MRI images which is divided into three tumor sets and one category of normal.
Hayato Tomita et.al[5] insists the value of deep learning in cervical cancer classification.The process of extracting deep feature and classification is made on preprocessed computerized tomography image.The pre processing is done to enhance the images so that it works well for next phase of processing.The classifier modelled by modifying VGG Mesh with squeeze excitation methodology.This model is adopted to improve classsification rating and to reduce manipulation complexity .The classifier classifies efficiently the cervical inage into precancerous and cancerous .The meta state is also identified and so early treatment can be adopted and this reduces the risks to be faced.
Yuexiang Liet.al[6] proposed a dodge architecture for cervical cancer classification. Classification model is made to get applied on acetic acid images.Acetic acid is applied on cervix and then it is visualy inspected for diagnosis.The post acetic effects are made for colposcopic examination.some researchers applied deep classification algorithm to single post acetic images and others used combined examination of saline ,iodine and acetic images.They are feature extracted seperately and classified and finally fused .The final image fusion stratergy is quite difficult to build as images taken are of different types.To solve multimodal image fusion complexity ,temporal acetic acid images are taken after 60,90,120 and 150 seconds and deeply studied and image feature fusion is attained by graph convoluttion network.This form of dodge shows good accuracy in classification and the clinical clue of duration of whitening is also considered for classification.
Sanjeev Dhawanet.al [7]modelled an algorithm based on the standard transfer learning to classify cervical images. This develops a cervical cancer prediction model based on deep learning and transfer learning techniques that recognizes cervical images and classifies them into one of classes (type 1/type 2/type 3). Techniques used to perform experimental work include deep learning and transfer learning. Created a ConvNet to classify cervical images using three related models: InceptionV3, ResNet50, and VGG19. Experimental results show that the Inception v3 classification model performs well than Vgg19 and ResNet50 with 96.1% accuracy .
Zaid Alyafeaiet.al[8] develop a fully automated structure for cervix region detection and classify cervical lymph nodes.The pipelined structure of deep learning model holds two phases of cervix detection and then with another learning model is used to classify cancer. The region of cancer is detected and trimmed so that learning level can converge on the area under interest.This proposal shows improvement interms of taining and testing test.It is working faster.This system uses the learning model which efficiently detect faster than state of art driven models and accurate in relating with union intersection measurements.The fine cropped cervix area is deep feature extracted for classification.
Avijit Kumar et.al[9] framed triple phase processing for feature detection and cervical cancer classification.Initially genetic algorithm is used to select features and that selected features are further mapped to reduced class sets and finally a learning model is used to classify cancer.The proposed method uses genetic algorithm and Logistic Regression for feature selection and finally logistic regression to classify into cancer and non cancerous class.To analse the performance of proposed work comparitive analysis is made with changing the lastend classification stage with its alternatesNaive Bayes (NB), Support Vector Machines (SVM), Extra Trees (ET), Random Forest (RF), and Gradient Boosting (GDB) .Comparitive study shows the proposed work outperforms well.
Aditya Khampariaet.al proposed internet of health thing driven deep learning model for feature selection and classification of cervical cancer types.Transfer learning is used to extract features and classification is adopted by several architectures like InceptionV3, VGG19, SqueezeNet and ResNet50 to have comparitive analysis. The result of pap smear test is fused within the feature extraction.The cytology image is diagnosed using the deep learning models and comparitive results shows resnet50 outperforms in classification efficiency.The already trained resnet 50 model classifies well and it gets trainined with short time.
Shenghua Chenget.al[11]presented a recurrent neural neteork to classify the precancerous changes into categories to study the grade of precancerous lesion.The whole slide cervical image with low resolution and high resolution are taken for process of cervical cancer classification.The low resolution whole slice and high resolution whole slide images are mixed for process of classification of lesion grade.The test results shows good true positive rate.
Chunnv Yuanet.al [12]framed an architecture of seperate deep learning models running parallel over acetic ,saline,iodine colposcopic images ,pap smear test results to extract robust features and multimodal fusion is done for destination classification process.this algorithm works for normal colposcopic images rather high resolution rate images.The multimodal fusion for final feature detection increses design complexity but sounds well interms of accuracy.
Ye Rang Parket.al[13]applies machine learning dodge and deep learning dodge over cervical images for classificationThe image is preprocessed for detecting the region of interest.The Machine learning algorithms Support vector machine,xGB,random forest are choosen for making a comparitive analysis with deep learning model.Resnet50 deep learning model is proposed for cervical cancer classificationThe comparitive study projects deep learning Resnet50 model a promising model for classification compared with three machine learning paradigms.
Hua Chenet.al developed cytobrain a deep learning flow model for cervical cancer classification.This model gets applied over whole slice image for cell image extraction.A classifier model of compact visual geometric group model is used for cell classification of cancerous and noncancerous and it is visual accessibility diagnostic module.Comparitive study is made with VGG11this compact VGG model works well with improved sensitivity ,specificity and F1 score.
Rohan Gorantlaet.al [15] performed image enhancement of cervigrams, followed by segmentation of cervix area which is the desired area for process and subsequent classification of the RoIs, in order to identify appropriate treatment decisions. Hierarchical Convolutional Mixture of Experts (HCME) algorithm is used for the classification task.This methodology outperforms all existing methods and is trained with the public Intel and Mobile ODT Kaggle datasets.This achievies 96.77% accuracy and a 0 kappa score. The results thus obtained promotes this approach of providing primary screening at low cost.
MavraMehmoodet.al[16] uses CervDetect algorithm to valuate the degree of cervical abnormality. CervDetect uses the degree of correlation in images and uses differential mapping as preprocessing stage and the correlator used is pearson correlator CervDetect selects significant features using a random forest (RF) feature selection technique. The pipeline architecture of CervDetect blends RF and flat neural networks to detect cervical cancer. This blended hierarchial structure of deep learning model accurately classifies and predict the degree of abnormality.
Sohely Jahanet.al[17] applied many machine learning models with best feature extraction engineering process with chi square select module .After feature engineering the result is made to evaluated with multilayer perceptron, random forest, k means nearest neighbours, support vector classifier, adaboost and gradient boosting machine learning models to have a comparative study.The comparitive results show multilayer perceptron has better evaluation metrics in cervical cancer classification and suitably aids early detection of cancer.
Elima Hussaina et.al [18]says prognosis of cervical carcinoma is essential to reduce morbidity risk and diagnosis of cervical cancer reduces mortality risk.The deep learning models are used to classify pap smear test results.Six deep learning models are explored in commbination with ensemble classifier to improve multi class classification accuracy.The algorithm works with pap smear images.
Peng Guo et.al [19] proposed a deep learning approach of singular ensemble to identify cervix image .It is trained with smartphone obtained cervical image dataset. The ensemble method blended the assessment of deep mastering architectures,RetinaNet, Deep SVDD, and a custom designed CNN.Each architecture works on its choice producing better feature detection and classification This fusion of features from different deep learning models improves the efficiency of the desired specification to met.
Lidiya Wubshet Habtemariam et.al [20] took 4005 colposcopy clinical snaps and 915 histopathology for training and validation.The model pipeline starts with cervix area detection with lightweight MobileNetv2-YOLOv3 and as the follow up, the EffecientNetb0 model is used for classification .
Jialin Shp et.al [21] uses RACTVNet(Area aware reweight stratergy and recursive refinement stratergy) for cervical clinical volume segmentation and shows two percent high attainment in results compared with other similar expert stratergy.
COMPARISION OF MACHINE LEARNING AND DEEP LEARNING
Machine and deep learing plays complete rule over machine assisted implementation.ML and DL are widely used in medical image analysis and provides good workout in all diversified medical image types..They both are versatile and finds in many applications other than healthcare.Machine learning needs feature engineering to do its end computation.
Deep leearning has ability to engineer the feature by itself and reduces human intervention further.Now a days deep learning is the preferable choice. Computer aided diagnosis system sticks more to clinical analysis because it makes diagnosis early ,reduces interobserver error and solves the problem of limited physician.Many deep learning algorithms were developed for image classification,Segmentation,image Registration.The convolution neural network, recursive neural nertwork, long term short memory neural network are the deep learning primary models and is modified in set to convenience for the desired application and specification.Comparing machine and deep learning, google survey says deep learning has high search record and also considering number of research publication deep learning has good graphical hike .The number of projects with AI technology of deep learning increases drastically in all domain.
Table 1 Summarisation of reviews
Reference |
Medical Image type |
Model used for automation |
Remarks |
VenkatesanChandranet.al |
colposcopy images of cervical cancer |
CYENET and VGG19 |
Attained classification accuracy of 73. 3% for VGG19 and improved classification accuracy of 92.3% for CYENET |
Eduardo Let.al |
cytological images of cervical cancer |
RetinaNet |
Achieves precision, recall, and F1 scores of 0.04, 0.20, and 0.07. |
Magnetic resonance imaging (MRI) images of brain tumors |
AlexNet+SVM |
Scores accuracy of 95.10% and 95. 25% sensitivity and 98.50% specificity |
|
Hayato Tomita et.al |
computed tomography of cervical cancer |
Modified VGG mesh and Squeeze and Excitation concept |
Sensitivity= 96.8% and specificity =95. 5%, Precision = 96.56%, Area Under the Curve = 96.16%. |
Yuexiang Liet.al |
Temporal colposcopy imagesof cervical cancer |
Graph convolutional networks with edge features |
classification accuracy of 78.33% |
Sanjeev Dhawanet.al |
Cervical images |
ConvNet |
96.1% accuracy |
Zaid Alyafeaiet.al |
Cervical images |
Two pipelined convolutional neural networks |
Gives Speedy detection |
Avijit Kumar et.al |
Cervical images |
Genetic algorithm- logistic regression- logistic regression |
Achieves 97% accuracy |
Aditya Khampariaet.al |
Cervical images |
Internet of Health Things (IoHT)-driven deep learning framework for detection and classification |
higher classification rate of 97.89% and works well with less training and test time |
Shenghua Chenget.al |
cytological images of cervical cancer |
recurrent neural network- based WSI classification model |
5% specificity and 95.1% sensitivity |
Chunnv Yuanet.al |
Saline , vinegar and iodine images of cervical cancer |
Deep learning model |
average accuracy of 95.59% and best with acetic acid image |
Ye Rang Parket.al |
Cervical images |
ResNet-50 , XGB, SVM, and RF |
ResNet-50 performs best |
Hua Chenet.al |
Cervical images |
CytoBrain |
reduces the time required for model training or sample testing |
Rohan Gorantlaet.al |
cervigrams |
Hierarchical Convolutional Mixture of Experts |
Attains 96.77% accuracy |
MavraMehmood et.al |
cervigrams |
CervDetect combined Random Forest and flat neural networks |
accuracy of 93.61% |
Sohely Jahanet.al |
cervigrams |
Machine learning(Multi layer perceptron) to detect cervical cancers |
Compared different machine learning algorithms |
DATASET
Mobileodt dataset,kaggle dataset,nci guanacaste challenge dataset,cvt dataset,alts dataset,diyss dataset provides cervical images for training,validating and testing and coco dataset is collection of commercial smart photographic taken by smart phones for cervix detection.the datasets used will have diference in brightness, tweezers, object position ,capturing position .
MOBILEODT DATASET
Mobileodt available at https://www.mobileodt.com contains dataset of colposcopic images captured by mobile device. Hospital universitario de caraca labels 9000 dataset images and verified by experts at the national cancer institute (nci) and delegates at mobileodt. The dataset holds diversed cervical images shoeing variation in age ,medical history etc
KAGGLE DATASET
Kaggle dataset has cervical images used to classify cervix types and also used for training validating and testing the cervical cancer types.
COCO DATASET
COCO collective general image dataset for theclassifying eight classes like cnine bike cat,cervical cancer etc.This is used for cervical image and non cervical image classification and mainly used for cervix detection.
NCI GUANACASTE CHALLENGE DATASET
The Guanacaste challenge cervical cancer dataset is framed by the American national cancersInstitute .Information is collected from 7000 patients and collected about 44000 cervical cervigram images.The other statistical data included in dataset includes age
,grade of HPV test and duration of visits.
CVT DATASET
Costa Rica Vaccine Trial (CVT) was conducted by means of the NCI and dataset is made from this campaign.HPV16/18 vaccinatedwomen suggested forcolposcopy if the cervical lesion sustains longer or if they have abnormal immature cancer status. The cervical images of women referred for colposcopy arerecorded and digitized.This dataset isannotated labelled professional gynecologist.
ALTS DATASET
Squamous cells of undetermined significance low-grade squamous intraepithelial lesion images were collected for triage study.Triagearea dataset images concentrateson evaluation of observer discrimination in visual interpretation of cervical abnormalities.Images arelabelled by medical experts.
DIYSS DATASET.
Habitual colposcopy examinations have been received and 17 serial
images were received before and after acetic acid by colposcope .Variations are noted within the clinical imagesin the dataset interms of cervix position and orientation, image brightness,noise level and edge levels.
EVALUATION METRICS
Evaluation Metrics used for comparing the performance of classification models are accuracy,sensitivity and specificity.Accuracy is the measure of precise detection.it is the ratio of sum of tue positives and true negatives to sum of true positive,true negative,false negative and false positive.Sensitivity is the ratio of true positive to sum of true positive and false negative.Specificity is the measure of rato of true negative to the sum of true negative and false positive.
Fig3:comparative analysis of evaluation parameter accuracy for different models.
The above chart shows comparative analysis of classification accuracy obtained in different deep learning models to classify cervical cancer.
CONCLUSION
Deep learning algorithms is promising stratergy for the early detection of cervical cancer in clinical images withequal performance to human clinicians, in phrases of sensitivity and specificity. Early detection of deadly disease reduces mortality risks.Cancer is slowly developing disease and so pre cancerous detection reduces risk.Datasets available of training and validating the machine is limited Augmentation techniques getting applied on images can be used to improve accuracy. A diagnostic system based on knowledge based deep learning may be useful for colposcopy diagnosis and biopsy of HSIL.This is helful in bringing transition to clinical practise ,high level with further research in deep learning application.
REFERENCES
[1]Venkatesan Chandran, M. G. Sumithra, Alagar Karthick, Tony George, M. Deivakani, Balan Elakkiya, Umashankar Subramaniam, S. Ma noharan, "Diagnosis of Cervical Cancer based on Ensemble Deep Learning Network using Colposcopy Images", BioMed Research International, vol. 2021, Article ID 5584004, 15 pages, 2021. https://doi.org/10.1155/2021/5584004 [2]Kelei He, Chen Gan, Zhuoyuan Li, Islem Rekik, Zihao Yin, Wen Ji, Yang Gao, Qian Wang, Junfeng Zhang, Dinggang Shen,Transformers in medical image analysis,Intelligent Medicine,2022,ISSN 2667-1026,https://doi.org/10.1016/j.imed.2022.07.002. [3] Silva, E.L., Sampaio, A.F., Teixeira, L.F., Vasconcelos, M.J.M. (2021). Cervical Cancer Detection and Classification in Cytology Images Using a Hybrid Approach. In: , et al. Advances in Visual Computing. ISVC 2021. Lecture Notes in Computer Science(), vol 13018. Springer, Cham. https://doi.org/10.1007/978- 3-030-90436-4_24 [4]Senan, Ebrahim & Jadhav, Mukti & Rassem, Taha & Aljaloud, Abdulaziz & Al-Shaibani, Badiea & Al-Mekhlafi, Zeyad. (2022). Early Diagnosis of Brain Tumour MRI Images Using Hybrid Techniques between Deep and Machine Learning. Computational and Mathematical Methods in Medicine. 2022. 1-17. 10.1155/2022/8330833. [5] Tomita H, Yamashiro T, Heianna J, Nakasone T, Kobayashi T, Mishiro S, Hirahara D, Takaya E, Mimura H, Murayama S, Kobayashi Y. Deep Learning for the Preoperative Diagnosis of Metastatic Cervical Lymph Nodes on Contrast-Enhanced Computed ToMography in Patients with Oral Squamous Cell Carcinoma. Cancers (Basel). 2021 Feb 3;13(4):600. doi: 10.3390/cancers13040600. PMID: 33546279; PMCID: PMC7913286. [6]Y. Li et al., "Computer-Aided Cervical Cancer Diagnosis Using Time-Lapsed Colposcopic Images," in IEEE Transactions on Medical Imaging, vol. 39, no. 11, pp. 3403-3415, Nov. 2020, doi: 10.1109/TMI.2020.2994778. [7]Dhawan S, Singh K, Arora M. Cervix Image Classification for Prognosis of Cervical Cancer using Deep Neural Network with Transfer Learning. EAI Endorsed Trans Perv Health Tech [Internet]. 2021 Apr. 12 [cited 2023 Mar. 4];7(27):e5. Available from: https://publications.eai.eu/index.php/phat/article/view/1210 [8]A fully-automated deep learning pipeline for cervical cancer classification,Expert Systems with Applications,Volume 141,2020,112951,ISSN 0957- 4174,https://doi.org/10.1016/j.eswa.2019.112951. [9]Chaudhuri, Avijit. (2021). A Multi-Stage Approach Combining Feature Selection with Machine Learning Techniques for Higher Prediction Reliability and Accuracy in Cervical Cancer Diagnosis. International Journal of Intelligent Systems and Applications. 13. 10.5815/ijisa.2021.05.05. [10] Khamparia, A., Gupta, D., de Albuquerque, V.H.C. et al. Internet of health things-driven deep learning system for detection and classification of cervical cells using transfer learning. J Supercomput 76, 85908608 (2020). https://doi.org/10.1007/s11227-020-03159-4 [11] Cheng S, Liu S, Yu J, Rao G, Xiao Y, Han W, Zhu W, Lv X, Li N, Cai J, Wang Z, Feng X, Yang F, Geng X, Ma J, Li X, Wei Z, Zhang X, Quan T, Zeng S, Chen L, Hu J, Liu X. Robust whole slide image analysis for cervical cancer screening using deep learning. Nat Commun. 2021 Sep 24;12(1):5639. doi: 10.1038/s41467-021-25296-x. PMID: 34561435; PMCID: PMC8463673. [12] Yuan, C., Yao, Y., Cheng, B. et al. The application of deep learning based diagnostic system to cervical squamous intraepithelial lesions recognition in colposcopy images. Sci Rep 10, 11639 (2020). https://doi.org/10.1038/s41598-020-68252-3 [13] Park, Y.R., Kim, Y.J., Ju, W. et al. Comparison of machine and deep learning for the classification of cervical cancer based on cervicography images. Sci Rep 11, 16143 (2021). https://doi.org/10.1038/s41598-021-95748-3 [14] Chen, H., Liu, J., Wen, QM. et al. CytoBrain: Cervical Cancer Screening System Based on Deep Learning Technology. J. Comput. Sci. Technol. 36, 347360 (2021). https://doi.org/10.1007/s11390-021-0849-3 [15]R. Gorantla, R. K. Singh, R. Pandey and M. Jain, "Cervical Cancer Diagnosis using Ce rvixNet – A Deep Learning Approach," 2019 IEEE 19th International Conference on Bioinformatics and Bioengineering (BIBE), Athens, Greece, 2019, pp. 397-404, doi: 10.1109/BIBE.2019.00078. [16] Mehmood M, Rizwan M, Gregus Ml M, Abbas S. Machine Learning Assisted Cervical Cancer Detection. Front Public Health. 2021 Dec 23;9:788376. doi: 10.3389/fpubh.2021.788376. PMID: 35004588; PMCID: PMC8733205. [17] Jahan, S., Islam, M.D.S., Islam, L. et al. Automated invasive cervical cancer disease detection at early stage through suitable machine learning model. SN Appl. Sci. 3, 806 (2021). https://doi.org/10.1007/s42452-021-04786-z [19] Lin-peng Jin, Jun Dong, "Ensemble Deep Learning for Biomedical Time Series Classification", Computational Intelligence and Neuroscience, vol. 2016, Article ID 6212684, 13 pages, 2016. https://doi.org/10.1155/2016/6212684 [20]Lidiya Wubshet Habtemariam, Elbetel Taye Zewde & Gizeaddis Lamesgin Simegn (2022) Cervix Type and Cervical Cancer Classification System Using Deep Learning Techniques, Medical Devices: Evidence and Research, 15:, 163-176, DOI: 10.2147/MDER.S366303 [21] Shi J, Ding X, Liu X, Li Y, Liang W, Wu J. Automatic clinical target volume delineation for cervical cancer in CT images using deep learning. Med Phys. 2021 Jul;48(7):3968-3981. doi: 10.1002/mp.14898. Epub 2021 May 19. PMID: 33905545. [22]. T. Xu, H. Zhang, C. Xin, E. Kim, and X. Huang, Multi-feature based benchmark for cervical dysplasia classification evaluation, Pattern Recognition, vol. 63, pp. 468475, 2017. [23] J. L. Belinson, R. G. Pretorius, W. H. Zhang, L. Y. Wu, Y. L. Qiao, andP. Elson, Cervical cancer screening by simple visual inspection after acetic acid,Obstetrics & Gynecology, vol. 98, no. 3, pp. 441444, 2001.
[24] W. Li, S. Venkataraman, U. Gustafsson, J. C. Oyama, D. G. Ferris, andR. W. Lieberman, Using acetowhite opacity index for detecting cervical intraepithelial neoplasia, Journal of Biomedical Optics, vol. 14, no. 1, pp. 014 0201014 02010, 2009 [25] D. Song, E. Kim, X. Huang, J. Patruno, H. Munozavila, J. Heflin et al., Multimodal entity coreference for cervical dysplasia diagnosis, IEEE Transactions on Medical Imaging, vol. 34, no. 1, pp. 229245, 2015. [26] S. K. Saini, V. Bansal, R. Kaur, and M. Juneja, ColpoNet forautomated cervical cancer screening using colposcopyimages, Machine Vision andApplications, vol. 31, no. 3,pp. 115, 2020.
[27] P. Sanyal, P. Ganguli, and S. Barui, Performance characteristics of an artificial intelligence based on convolutional neuralnetwork for screening conventional Papanicolaou-stained cer-vical smears, Medical Journal, Armed Forces India, vol. 76,no. 4, pp. 418424, 2020. [28] A. M. Iliyasu and C. Fatichah, A quantum hybrid PSO com-bined with fuzzy k-NN approach to feature selection and cellclassification in cervical cancerdetection, Sensors, vol. 17,no. 12, p. 2935, 2017.
[29] T. Xu, H. Zhang, X. Huang, S. Zhang, and D. N. Metaxas,Multimodal deep learning for cervical dysplasia diagnosis,in Medical Image Computing andComputer-Assisted Interven-tion MICCAI 2016, Lecture Notes in Computer Science,pp. 115123, Springer, Cham, 2016.
[30] B. J. Cho, Y. J. Choi, M. J. Lee et al., Classification of cervicalneoplasms on colposcopic photography using deep learning,Scientific Reports, vol. 10, no 1, p. 13652, 2020.