
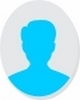
- Open Access
- Authors : Chetana C. Chaudhari , Rahul A. Patil
- Paper ID : IJERTV10IS050282
- Volume & Issue : Volume 10, Issue 05 (May 2021)
- Published (First Online): 29-05-2021
- ISSN (Online) : 2278-0181
- Publisher Name : IJERT
- License:
This work is licensed under a Creative Commons Attribution 4.0 International License
A Variations of HBass Model for Popularity Prediction on Social Media
Prof. Rahul A. Patil
Dept. of Comp Engg., PCCOE Pune-411044, India
Chetana C. Chaudhari
Dept. of Comp Engg., PCCOE Pune-411044, India
AbstractTwitter is the mostsizzling microblogging service these days and considering the characteristics of popular tweet. Twitter is significant in a wide range of fields, for example, viral advertising, customized messages proposal, breaking news recognition, etc. However, adopting existing topic or event prediction models cannot get acceptable outcomes. This paper investigates the problem of predicting the popularity of tweet. Spatial-Temporal Heterogeneous Bass (ST-HBass) and Feature- Driven Heterogeneous Bass (FDBass) are two versions of the Heterogeneous Bass model (HBass) that were first built in the process of marketing engineering. Predicting the popularity of a product, the Heterogeneous Bass Model (FT-HBass) was used. This model is approved by leading investigates certifiable Twitter information, and the outcomes show the proficiency and precision of our model, with less total percent blunder and the best Precision and Recall.
Keywords:- Heterogeneous Bass model, Single message popularity, Twitter network
-
INTRODUCTION
Twitter is a great social messaging service application that permits client to exchange information and discuss about everything, including news, anecdotes, and even their own life stories. As long as it is 2006, the Twitter has been experienced to become more and more rapid explosion in its tweet amount, to generate 200 million tweets per day as of 2011. Users on Twitter would almost certainly encounter information overload as a result of the high volume of messages, making it impossible for them to find valuable information. Due to the limitations of information diffusion trees, users can miss some important or interesting messages. To address these two problems, it is necessary to determine the popularity of tweet in Twitter.
The primary objective is to estimate the popularity of a single comment at every point in its life span through quantities. Then, need to create subjective forecast by grouping a comment as famous or disliked. This work is crucial for each everyday users and groups. For customers, it offers a device to help them filter via a massive quantity of new content a good way to identify thrilling gadgets in a well-timed way. Another critical software of this paintings is to help corporations capture the possibility to guide and generate a fashion or hot subject matter. Lastly, odd famous tweets can set alarm for disaster and crime. The facebook is assisting with getting hoodlums. In some cases, the suspect unavoidably boasts about his degenerate conduct on the informal communities, which catches the clients consideration.
Heterogeneous-bass-model(HBass) structure, which includes two varieties, to be specific Spatial-temporal-heterogeneous- bass-model(ST-HBass) and feature-driven-heterogeneous-
bass-model (FD-HBass), anticipating to the notoriety for solitary comment. The Bass version is certainly one of the maximum broadly used model in the executives science. It is initially using to display the deals of recently put-on-showcase item to gathering of individuals, they can be foresee the prevalence of a recently put up the single comment in a social network. It has made 12 highlights for every theme and determined subject prevalence utilizing typical bass dispersion models in Twitter. Be that as it may, it isnt quickly understandable the Bass-version is assigned to a single tweet expectation. It is difficult to include the highlights the informal organization in just 2-parameters. Also, the Bass -version accept spatial-and-temporal-homogeneity, prompting no differentiation of people.
The primary contributions of work presented here are listed as.
-
The HBass model incorporates twitter into the Bass model in social network single-tweet prediction. HBass often comes in two flavours: ST-HBass (where it relies on spatial-and-temporal-heterogeneity) [9] and FD-HBass (where it relies on the influence of different characteristics). To be more precise, help to guess a single tweets pattern and whether it will be popular in the future.
-
The Interaction Enhancement takes into the interaction enhancement account in the real-world situation in which various tweets about the same subject compete and cooperate with one another.
-
Instead of selecting a threshold based on experience, redefine the quantitative concept of popularity to include the relationship between favourite, retweet, and reply, as well as a threshold to identify common and controversial tweets based on clustering process.
-
Utilizing true twitter information to look at the productivity of the simulation results show that the effectiveness and exactness of the quantitative expectation with less outright percent mistake and the subjective forecast with a superior classification [3] discovery.
-
-
LITERATURE SURVEY
The most significant step in any kind of study is the literature survey. We want that to review the papers that are formerly used in our domain that we are working on before we start developing and we can predict or generate the disadvantage on the basis of study and using the references from previous papers as a guide. In this segment, we momentarily audit the connected work on popularity prediction and their different techniques and also compare methods or techniques advantages and limitations or future work.
Paper 1: XiaomingChen and et al had proposed [1] to used Multivariate Linear (ML) and ModelSzabo-Huberman (S-H) Model which gives better output. The advantage of this model are used for considering possible web content popularity based on recorded data provided by effective popularity measures. It is feasible to utilize diverse ubiquity expectation models for each example. That can prompt decreased forecast mistakes due to investigating the contrasts between designs in a more unequivocal structure.
Paper 2: Sirisup Laohakiat and et al had proposed [10] the twitter fame profile mining and expectation effectively. The proposed structure not exclusively can perform twitter ubiquity profile mining and expectation proficiently, yet additionally can be applied to mine the arrangements of any time arrangement. Later on, to improve the structure to consider both the substance of the posts just as the fleeting profile to make more precise expectation.
Paper 3: Przemys law Rokita and et al had proposed [11] to used Support Vector Regression to foresee the fame of online video content estimated as the quantity of perspectives. Later on work is to broaden the arrangement of highlights utilized for expectation by adding more semantic signs, like video point or the assumption of the social communications, to more readily comprehend.
Paper 4: Cheng-Te Li and et al had proposed [4] to used personalized self-exciting point process Model (PSEISMIC)for oneself energizing point cycles to build up a factual model, PSEISMIC, which prompts exact expectations of the personalized self-exciting point process Model doesnt deal with the various tweets for ongoing.
Paper 5: Almeida and Marcos A. Goncalves and et al [5] generally based on the Multivariate Linear (ML) Model Szabo- Huberman (S-H) Model and these model are generally used for foreseeing the future prevalence of Web content dependent on verifiable data given by early fame measures. It is feasible to utilize diverse notoriety expectation models for each example. That can prompt diminihed forecast mistakes due to investigating the contrasts between designs in a more unequivocal structure.
Paper 6: Yeyun Gong and Xuanjing Huang and and et al is based [2] on the Deep Neural Network and the attention based deep neural network is used to fuse context oriented and social data for the undertaking. They utilized inserting to mirror the client, the interests of the clients advantage, the creator and tweet, separately. In this strategy, the undertaking of retweet forecast is just in binary classification.
-
TECHNICAL BACKGROUND
Machine Learning methodologies usually involve a learning process with the intention of learning to perform a task from experience(training data). In ML data consists of a series of examples. Typically, a collection of attributes, also known as features or variables, defines an individual instance. A feature can be nominal, binary. ordinal, or numeric number, etc A
performance matrix that increases with experience is used to evaluate the ML models [6] performance in a given task. Various statistical and mathematical methods are used to measure the efficiency of ML models and algorithms. The trained model can be used to identify, predict, or cluster new examples (testing data) after the end of the learning process using the experience obtain during the training process. The system is used for predict the tweet popularity in for its entire life cycle with the client highlights and single-tweet highlights. From that point forward, we can get the pattern of a tweet. At long last, we can gauge the status whether the tweet is famous or disliked with the well popular threshold.
-
Techniques used
-
Feature-pivot method: Feature-pivot means strategies that revolve around functionality, such as how many users at any given moment are tweeting. This means the first and most important decision you have to make in feature-pivot methods
-
is what a feature means to you. Here are a few examples of general features:
-
The range of tweets published per minute.
-
The range of tweets that have been released since the last minute.
-
The rate at which a word is discussed in a minute.
-
-
-
Convolutional Neural Network: Convolutional neural network has been effectively applied to numerous fields, for example, natural language processing, image acknowledgment or Video processing, etc.That are used in a convolutional architecture (as shown in the Fig. 1) for the sentences showing and the assessment various approaches for extending the organization of a CNN in time space for the chronicles request. It has been join the bona fide power of colossal, multilayer neural associations together for scene text affirmation. Multiple layers of artificial neurons compose convolutionary neural networks. Artificial neurons are mathematical functions that measure the weighted sum of multiple inputs and output an activation value, similar to their biological counterparts.
Fig. 1. Convolutional neural networks basic component
-
Support Vector Regression: For two-group classification problems, a support-vector-machine(SVM) is a supervised machine learning model that uses classification algorithms. SVM models will categorise new text after being given sets of labelled training data for each group. In classification issues, however, it is mostly used. In the SVM algorithm [11], plotting every other informative item in n- dimensional space wherein is the number of characteristics you have with the value of each characteristic being the coordinate value. Then we perform finding the hyperplane that
corresponding to the classification by locating the hyper-plane that clearly distinguishes the two classes.
-
Personalized-self-exciting-point-process-Model (PSEISMIC): The envisaged PSEISMIC [4] model comprises of four stages. The flowchart is appeared in underneath Fig. 2 that including data-filter, cluster-analysis, memory-kernel- estimating, post-infectiousness-estimating, and predicting- final-popularity.
Fig. 2. Basic structure of PSEISMIC
-
Dynamic-time-warping(DTW): Dynamic-Time-Warping
-
-
is used to compare the similarity of two arrays or time series of different lengths or to measure the distance. In general, DTW is a method that calculates an with certain constraints and rules, the best match between two given sequences:
-
Each index in the first series must be paired with one or more indices in the second, and vice versa.
-
You must compare the first index of the first sequence with the first index of the second sequence.
-
It is important to compare the last index from the first sequence with the last index from the other set.
-
-
-
PROPOSED FRAMEWORK
To fathom the issue, we plan a system for our expectation framework. The generally system is appeared in Fig. 3. The information preprocessing module is utilized to channel the crude client highlights and single-tweet highlights, and select the ubiquity markers. The parameter setting module employments all the ubiquity pointers dataset that are shaped from the information preprocessing module to induce the indicators weight and the ultimate ubiquity limit. The expectation module is utilized to predict the slant of a tweet. After that, able to compare the well-known edge and the ubiquity to decide whether itll be prevalent or not at the conclusion.
-
Heterogeneous Bass model
The Bass show was proposed to anticipate the deals of a modern item when it is propelled on the advertise. Now, it is being broadly utilized in numerous sorts of inquires about. Since no require of expansive numbers of the preparing set, given the primary a few days or months of deals of a
unused item, able to effectively foresee the execution of the item afterward utilizing as it were two parameters. Although the Bass show is an great show in financial areas, it isnt straightforward [9] to relocate Bass model specifically for single tweet forecast due to the impediment of parameters number and the spatial and temporal homogeneity suspicion.
There are three sorts of terms to mirror the spatial heterogeneity: the inherent likelihood of selection, the helplessness to intra populace linkages and the infectiousness of adopters. For this restriction, able to enlist twitter highlights into the first show, and unwind it to individual level heterogeneity. Due to the character of Twitter highlights, we propose Heterogeneous Bass model(HBass) which has two variations, namely spatial-temporal-heterogeneous bass- model(STHBass) and feature-driven-heterogeneous-bass model(FDHBass).
-
ST-HBass Model : The standard Bass model suggests spatial and temporal homogeneity, which results under no small portion, making it unsuitable for tweet analysis. To transcend the shortcomings of the conventional Bass model, we suggest the STHBass model, which, while mixed with the actual Bass model, focuses more on spatial and temporal heterogeneity. The standard formulation of a diffusion method is as follows:
f(t) = (p+qF(t))(1F(t)) (1)
where, p = innovators coefficient q = the coefficient of imitators f(t)= hazard rate of adoption
-
FD-HBass Model : We centre more around the impact of various highlights dependent on heterogeneity to the standard Bass model, which is a helpful technique to loosen up the limit of the first Bass model. To recognize the distinctive impact on the two sorts of highlights, we propose the FD-HBass model. While considering the single-tweet highlights, they just effect the fame tally through the qualities of the actual tweet. All the while, client highlights can mirror the proliferation from a client to another client, somewhat, which like the imitators. At that point the equation is:
1+
1+
(1(+))
() =
(+) (2)
Fig. 3. Workflow of proposed work
Acordingly, we get the Feature-driven-heterogeneous-bass model.
-
Interaction Enhancement: Its not like every twitter message does indeed have a hashtag campaign, and then each message will have its own theme, and since we all recognize. Even though, seeing as its an overview with manual method [9], hash tag has been the safest gross generalisation of the subject. While many tweets of the very same subject emerge in a brief span of time, it might cause additional participants to become interested in the topic, increasing the growth of each tweet. We establish STIHBass after incorporating Interaction Enhancement and Hbass.
+ln ( )
=
(6)
1
1
() = 2
2 +1
+
+
(3)
+
+ln ( 1) 1 2 3
1+
FDI-HBass as:
2
= 2
+
(7)
() =
(1(+))
(+) 1 2 3 (4)
Table II appears the execution of quantitative forecast by totally multiple strategies. At whatever point well-liked time is
1+
-
-
DATASET
The Twitter API database became analyzed at variance from either the 21st of December 2018 to the 30th of February 2019. We mostly creep knowledge concerning new ways to tackle, besides retweets, although the actual tweets would have been prominent if any of their retweets were mostly prominent. Because of the obvious Twitter APIs IP rejection and advice game plans worker limitation, everyones scrambler advancement is limited to around two hours a day, limiting the datas dimensionality. After that, in order to maintain
TABLE I USERTWEET RELATIONSHIP
Table
Detail
Tweet
tweet id, user post time text catch time author id
User
user id
number of followers number of followings number of tweets number of favourites register time
User-tweet
user id, tweet id
TABLE II PERFORMANCE OF VARIOUS STRATEGIES
t = 24 hr
t = 240 hr
Model
precision
recall
Fscore
precision
recall
Fscore
ST-
HBass
0.8564
0.8233
0.8395
0.9316
0.8936
0.9122
FD-
HBass
0.9419
0.7864
0.8572
0.9621
0.8012
0.8743
STI-
HBass
0.9286
0.8756
0.9013
0.9554
0.8382
0.8930
FDI-
HBass
0.9683
0.9403
0.9541
0.9788
0.9244
0.9508
consistency, we deliver the remarks in English. As sensible a result, the breaking point data includes 2516440 tweets with specific between seasons of time and 2122135 clients. The TABLE: I is an overview of the usertweet relationship.
-
-
RESULT AND DISCUSSION
The temporal layers a lot of specifically so as to sight the disorders. The long tweets dont continually contain a lot of data than short tweets due to the unimportant words. We were using accompanying implementation guesstimates to evaluate the sustainability of a quantitative forecast:
to be assessed is t = 24 hr or t = 240 hr, our HBass has a very good keen execution in classification. Uncommonly, FDI- HBass receives the most effective exactness, higher than FD- HBass and best F-score.
-
CONCLUSION
We style the heterogeneous-bass-model (HBass) that represent two assortments, specifically spatial-temporal- heterogeneous-bass-model (ST-HBass) and feature-driven- heterogeneous-bass-model (FD-HBass), can forecast that future recognition of one comment. we tend to conjointly recommend associate degree Flavouring activity to keep the rivalry and partnership alive connection of various tweets along the same thing. More style associate degree clump methodology to certain the recognition threshold supported planet dataset. For qualitative research, we use real-world Twitter info. Our design has the highest precision and F-score, suggesting that it is the most accurate. This demonstrates that our models are more accurate in their classification the identification of the Bass model is introduced. We also display implementing measures in a social network that it produces outstanding results.
REFERENCES
-
X. Zhang, X. Chen, Y. Chen and J. Xia, Event detection and popularity prediction in microblogging, in Elsevier Neurocomputing, vol. 149, pp. 14691480, 2015.
-
Q. Zhang, Y. Gong and J. Wu, Retweet prediction with attentionbased deep neural network, in ACM International on Conference on Information and Knowledge Management (CIKM), 2016, pp. 7584.
-
W. Xie, F. Zhu, J. Jiang, E.-P. Lim, and K.Wang, Topicsketch: Realtime bursty topic detection from twitter, in IEEE Transactions on Knowledge and Data Engineering (TKDE), vol. 28, no. 8, pp. 2216 2229, 2016.
-
H.Y. Chen and C. Li, Pseismic: A personalized self-exciting point process model for predicting tweet popularity, in ACM International Conference on Big Data, 2017, pp. 27102713.
-
H. Wenjian, S. K. Kumar and X. Fanyi and H. Jinyoung Using early view patterns to predict the popularity of youtube videos, in ACM International Conference on Web Search and Data Mining (WSDM), 2018, pp. 19.
-
M. X. Hoang, X. H. Dang and A. K. Singh, Gpop: Scalable grouplevel popularity prediction for online content in social networks, in ACM International Conference on World Wide Web (WWW), 2017, pp. 725 733.
-
S. Hosseini, H. Yin, X. Zhou and N.M. Cheung, Leveraging multiaspect time-related influence in location recommendation, World Wide Web (WWW), pp. 128, 2017.
-
K. ubbian, B. A. Prakash, and L. Adamic. Detecting Large Reshare Cascades in Social Networks. Proc. of ACM International Conference on World Wide Web (WWW), 2017.
-
Xiaofeng Gao, Member, IEEE, Zuowu Zheng, Quanquan Chu, Shaojie Tang, Member, IEEE, Guihai Chen, Senior Member, IEEE and Qianni Deng Popularity Prediction for Single Tweet based on Heterogeneous Bass Model1041-4347 (c) 2019 IEEE.
-
Rattasit Sermsai and Sirisup Laohakiat, Analysis and Prediction of Temporal Twitter Popularity Using Dynamic Time Warping 978-
=
+
(5)
17281-0719-6/19/31.00 2019 IEEE
-
Tomasz Trzci´nski and Przemysaw Rokita, Predicting popularity of online videos using Support Vector Regression arXiv:1510.06223v4 [cs.SI] 12 May 2017