
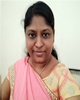
- Open Access
- Authors : Suchitra Reyya , Hemalatha Sugandapu , Abhiram Srungaram , Reshma Mutta, Sasi Kumar Thallapudi
- Paper ID : IJERTV11IS050167
- Volume & Issue : Volume 11, Issue 05 (May 2022)
- Published (First Online): 24-05-2022
- ISSN (Online) : 2278-0181
- Publisher Name : IJERT
- License:
This work is licensed under a Creative Commons Attribution 4.0 International License
An Efficient Approach for Detecting Building Defects using Class Activation Mapping (CAM)
Suchitra Reyya1, Hemalatha Sugandapu2, Reshma Mutta3, Abhiram Srungaram4, Sasi Kumar Thallapudi5.
1Associate Professor, Lendi Institute of Engineering and Technology
2,3,4,5 Lendi Institute of Engineering and Technology
Abstract:- Population is increasing every day but the Land percentage is constant. So, the number of Buildings is constantly increasing and Customer satisfaction is a key aspect of all building constructions. All Damaged or defective areas cannot be easily identified. Usually, Traditional methods require a lot of inspection to identify the affected areas. Its critical to have Classification models based on length, Width notation of Damaged area along with its type of Dampness. We are using Deep Learning models like Convolutional Neural Networks Techniques such as, Class Activation Mapping (CAM) With specific damage with high accuracy so that to predict damage rate previously so that to eradicate major incidents.
INTRODUCTION
Clients are having so many assets that it is an important task to have knowledge of the condition of each of their operational assets to enable them to effectively manage their portfolio and improve business performance. This is being driven by the increasing adverse effects of climatic changes, irregular damages so that its direct identification of where the problem arises will be difficult. Traditional methods for this type of work commonly involve engaging building surveyors to undertake a condition assessment which involves a lengthy site inspection resulting in a systematic recording of the physical conditions of the building elements with the use of photos, note taking, drawing sketch and information provided by the clients. That collected data can be analyzed to know the actual problem manually. This can also be used to produce estimates of immediate and projected long- term costs of renewal, repair and maintenance of the building. This enables facility managers to address current operational requirements, while also improving their real estate portfolio renewal forecasting and addressing funding for capital projects. Current asset condition assessment procedures are extensively time consuming, laborious and expensive, and pose health and safety threats to surveyors, particularly at height and roof levels which are difficult to access for inspection.
Image analysis techniques by using classification for detecting defects have been proposed as an alternative to the manual on-site inspection methods. Image analysis involves processing an image into fundamental components to extract meaningful information. Image analysis can include tasks such as finding shapes, detecting edges, removing noise, counting objects, and calculating statistics for texture analysis or image quality. In recent years, researchers have experimented with the application of a number of soft computing and machine learning-based detection techniques as an attempt to increase the level of automation of asset condition inspection. The notable efforts include; structural health monitoring with Bayesian method, support vector machines (SVM), and neural networks, Support Vector Machines for wall defects recognition, crack-detection on concrete surfaces using deep neural networks (DNN), deterioration assessment using fuzzy logic. Recently so many researchers have focused on the automated detection of defects in earthquake damaged structures. Considering all these studies, far too little attention has been paid to the application of advanced machine learning methods and deep learning methods in the advancements of smart sensors for the building defects detection.
The major objective of this research is therefore to investigate the novel application of deep learning methods of convolutional neural networks (CNN) in automating the condition assessment of buildings. The focus is mainly to automated detection and localization of key defects arising from dampness in buildings from images with exact accuracy, length and width so that identification becomes so easier. However, as the first attempt to tackle the problem, this paper applies a number of limitations. Firstly, there can be multiple types of defects that are not considered at once. This means that the images considered by the model belong to only one category. Secondly, only the images with visible defects are considered. Thirdly, consideration of the extreme lighting and orientation, e.g., low lighting and too bright images are not included in this study. In the future, however, these limitations will be considered to be able to get closer to the concept of a fully automated detection.
The rest of this paper is organized as follows. A discussion that includes selection of the most common defects that arise from the presence of moisture and dampness in buildings is presented. This is followed by a brief overview of CNN. We propose a deep learning-based detection and localization model using transfer learning utilizing the VGG-16 model for feature extraction and classification. Next, we briefly discuss the localization problem and the class activation mapping (CAM) technique which we incorporated with our model for defect localization with exact length and width. This is followed by a discussion of the dataset used, the model developed, the results obtained, conclusions and future work.
-
DAMPNESS IN BUILDINGS
Buildings are generally considered durable because of their ability to last hundreds of years. However, those that survive long have been subject to care through continuous repair and maintenance throughout their lives. Structural dampness is the presence of unwanted moisture in the structure of a building, either the result of intrusion from outside or condensation from within the structure. These have been classified in ISO 19208 into five major groups as follows: electro-magnetic agents (e.g.
solar radiation, radioactive radiation, lightening, magnetic fields); mechanical agents (e.g., loads, thermal and moisture expansion, wind, vibration and noises); thermal agents (e.g., heat, frost); chemical agents (water, solvents, oxidizing agents, sulfides, acids, salts); and biological agents (e.g., vegetable, microbial, animal). Carillion [35] presents a brief description of the salient features of these five groups followed by a detailed description of numerous defects in buildings including symptoms, investigations, diagnosis and cure.
Dampness is increasingly significant as the value of a building can be affected even where only low levels of dampness are found. Homes, and any kind of buildings, should be preserved at the structural level onwards so as to prevent wear and tear. The fabric of buildings under normal conditions contains a surprising amount of moisture which does not cause any harm. The term dampness is commonly reserved for conditions under which moisture is present in sufficient quantity to become either perceptive to sight or by touch, or to cause deterioration in the decorations and eventually in the fabric of the building. A building is considered to be damp only if the moisture becomes visible through discoloration and staining of finishes, or causes mould growth on surfaces, sulfate attack or frost damage, or even drips or puddles There are various forms of decay which commonly afflict the fabric of buildings which can be attributed to the presence of excessive dampness. Dampness is thus a catalyst for a whole variety of building defects. Moisture in buildings is a problem because it expands and contracts with temperature fluctuations, causing degradation ofmaterials. Subsoil moisture rising through foundation beds, Rainwater seeping in from external walls, Rainwater coming through parapet and compound walls, Moisture deposited on buildings due to condensation are the main reasons for the dampness.
Damp conditions encourage the growth of wood rotting fungi, the infestation of timber by wood boring insects and the corrosion of metals which leads to instability of the buildings.
-
Origin of Dampness
A high proportion of dampness problems are caused by Rain Penetration, Level of Site, Drain ability of soil. Climate Condition, Defective Orientation of the Building, Moisture Entrapped during Construction, Defective Construction e.g., Joints. Condensation occurs when warm air collides with cold surfaces, or when there's too much humidity in your home.
When this moisture-packed warm air comes into contact with a chilly surface, it cools down quickly and releases the water, which turns into liquid droplets on the cold surface. Through the daily routine of showers, baths, boiling kettles, cooking, using a tumble dryer, drying clothes and breathing a family of 4 will contribute approximately 4 pints of water per person a day, equal to over 100 pints of water vapor a week, which has to end up somewhere. Thus, condensation is dependent on the temperature of surfaces and the humidity of the surrounding air. Condensation is the most common damp that can be found in both commercial and residential property.
In many cases, rain penetration is caused by poorly designed or maintained building details (e.g., blocked downpipes or leaking gutters) causing large amounts of rainwater to flow over a small section of masonry. In these cases, the penetrating damp can usually be cured by rectifying the defect. It can be caused by the effects of incorrect design, bad worker construction, and the wrong choice of requirements, badly executed repairs or lack of regular maintenance. The most exposed parts of a building such as roofs, chimneys and parapets are the most susceptible to rain penetration. Rising damp is caused by the rising of groundwater through capillaries in masonry. As this has been a well-known problem for some years, buildings these days are built with a Damp Proof Course. However, rising damp can still occur, especially in older buildings. The cause of this is often a faulty or non-existent DPC.
Other causes of dampness include: construction moisture; pipe leakage; leakage at roof features; ground and surface water; and contaminating salts in solution.
-
The Effects of Damp
Moisture can damage the building structure, increase the heat transfer through the envelope and it may also cause poor indoor air quality and respiratory illness in occupants. Dampness gives rise to breeding of mosquitoes and creates unhealthy living conditions. Travel of moisture through walls and ceiling may cause unsightly patches. Moisture travel through walls may cause softening and crumbling of plaster, especially lime plaster. The wall decoration and Painting is damaged. Continuous presence of moisture in the walls may cause efflorescence resulting in disintegration of bricks stones, tiles, etc., and consequent reduction in strength. The flooring gets loosened because of reduction in the adhesion when moisture enters through the floor, timber fittings, such as doors, windows, almirahs, wardrobes etc., coming in contact with damp walls, damp floors may get deteriorated because of warping, buckling, dry-rotting of timber. Electrical fittings get deteriorated, giving rise to leakage of electricity and consequent danger of short circuiting. Floor coverings are damaged. On damp floors, one cannot use floor coverings. Dampness promotes and accelerates growth of termites Dampness along with warmth and darkness breeds germs of dangerous diseases such as tuberculosis, neuralgia, rheumatism etc. Occupants may even be asthmatic. Moisture causes rusting and corrosion of metal fittings attached to walls, floors and ceiling.
In the main, the types of deterioration driven by water in Building materials include: moulds and fungal growth; materials spalling; blistering; shrinkage; cracking; irreversible expansion; embrittlement; strength loss; staining or discoloration; steel and iron rusting; and decay from microorganisms. In extreme cases, mortar or plaster may fall away from the affected wall. The focus of this paper is on moulds, cracks, flakes damage and also damages present in roofs and paint deterioration which are the most common interrelated defects arising from dampness.
Moulds and fungi occur on interior and exterior surfaces of buildings following persistent condensation or other forms of frequent dampness. On internal surfaces, they are unsightly and can cause a variety of adverse health effects including respiratory problems in susceptible individuals. Moulds on external surfaces are also unsightly and cause failure of paint films. They are unsightly and can cause paint failure like moulds.
Paint deterioration occurs because of exposure to UV light (sunlight), moisture and freeze-thaw cycles. Free radicals are highly reactive and either form or break down chemical bonds in substances. In the case of paint durability on exposure, free radicals actually damage the film. The growth of mildew and fungi in the presence of moisture and humid conditions will cause paint damage. The exposure of exterior surfaces to sun and its ultraviolet radiation causes paint to fade and look dull. Exposure to airborne salts and pollution will also cause paint deterioration.
-
-
CONVOLUTIONAL NEURAL NETWORKS (CNN)
CNN, a class of deep learning techniques, are primarily used for solving fundamental problems in computer vision such as image classification, object detection,localization and segmentation. Although early deep neural networks (DNN) go back to the 1980s when Fukushima applied them for visual pattern recognition, they were not widely used, except in few applications, mainly due to limitation in the computational power of the hardware which is needed to train the network. It was in the mid-2000s when the developments in computing power and the emergence of large amounts of labeled datasets contributed to deep learning advancement and brought CNN back to light.
3.1 CNN Architecture
The simplest form of a neural network is called perceptron. This is a single-layer neural network with exactly one input layer and one output layer. Multiple perceptrons can be connected together to form a multi-layer neural network with one input, one output and multiple inner layers, which are also known as hidden layers. The more hidden layers, the deeper is the neural network (hence the name deep neural network). As a rule of thumb when designing a neural network, the number of nodes in the input layer is equal to the number of features in the input data, e.g224 pixels in each channel, therefore, the number of nodes in our input layer is 3x224x224. The number of nodes in the output layer, on the other hand, is determined by the configurati on of the neural network. For example, if the neural network is a classifier, then the output layer needs one node per class label, e.g., in our neural network, we have four nodes corresponding to the four class labels: mould, stain, deterioration and normal.
When designing a neural network, there are no particular rules that govern the number of hidden layers needed for a particular task. One consensus on this matter is how the performance changes when adding additional hidden layers,
i.e., the situations in which performance improves or becomes worse with a second (or third, etc.) hidden layer. One common way suggests that the optimal size of the hidden layer should be between the size of the input layer and the size of the output layer.
3.1.1 Working of CNN Layers
Although CNN has different architectures, almost all follow the same general design principles of successively applying convolutional layers and pooling layers to an inut image. Convolutional layer is the first layer, its purpose is to detect the presence of a set of features in the images received as input. This is done by convolution filtering: the principle is to drag a window representing the feature on the image, and to calculate the convolution product between the feature and each portion of the scanned image. A feature is then seen as a filter: the two terms are equivalent in this context
Figure 1. Basic ConvNet Architecture
The convolutional layer thus receives several images as input, calculating the convolution of each of them with each filter. The filters correspond exactly to the features we want to find in the images We get for each pair (image, filter) a feature map, which tells us where the features are in the image: the higher the value, the more the corresponding place in the image resembles the feature. The next layer Pooling layer is often placed between two layers of convolution: it receives several feature maps and applies the pooling operation to each of them. The pooling operation consists in reducing the size of the images while preserving their important characteristics. To do this, we cut the image into regular cells, then we keep the maximum value within each cell. In practice, small square cells are often used to avoid losing too much information. The most common choices are 2×2 adjacent cells that dont overlap, or 3×3 cells, separated from each other by a step of 2 pixels (thus overlapping). The pooling layer reduces the number of parameters and calculations in the network and this improves efficiency of the network and avoids over learning.
Thirdly, ReLU (Rectified Linear Units) refers to the real non-linear function defined ReLU(x)=max (0, x). Visually, it looks like the following:
The ReLU correction layer replaces all negative values received as inputs by zeros. It acts as an activation function.
The last fully-connected layer classifies the image as an input to the network: it returns a vector of size N, where N is the number of classes in our image classification problem. Each element of the vector indicates the probability for the input image to belong to a class. To calculate the probabilities, the fully-connected layer, therefore, multiplies each input element by weight, makes the sum, and then applies an activation function (logistic if N=2, SoftMax if N>2). This is equivalent to multiplying the input vector by the matrix containing the weights. The fact that each input value is connected with all output values explains the term fully-connected.
3.2 Transfer Learning
Data which depends on other factors is one of the most challenging problems in deep learning where sufficient training requires huge amounts of information in order for the network to understand the patterns of data. In deep learning, both training and testing data are assumed to have the same distribution and same feature space. In reality, however, adequate training data may exist in one domain, while a classification task is conducted on another. So, here comes the topic of Transfer Learning, which means the use of previously acquired knowledge and skills in new learning or problem-solving situations. Thereby similarities between previous and actual learning content and processes may play a crucial role. Suppose, if the data distribution changes on the target domain, a whole rebuild of the classification network is required with a newly collected training dataset. In most of the applications, constructing largely-enough, properly-labeled datasets can be quite challenging, particularly, when data acquisition is expensive or when data annotation is the timely consuming process. This could limit the development of many deep learning applications such as biomedical imaging where training data in most cases is not enough to train a network. It is not every case that the training data is sufficient for applying directly on the input network then at that time, transferring learning (knowledge) from one domain to another can be advantageous.
3.2.1 Mathematical Notations
Transfer Learning
The ImageNet dataset contains images of fixed size of 224*224 and have RGB channels. So, wehave a tensor of (224, 224, 3) as our input. This model processes the input image and outputs a vector of 1000 values.
This vector represents the classification probability for the corresponding class. Suppose we have a model that predicts that image belongs to class 0 with probability .1, class 1 with probability 0.05, class 2 with probability 0.05, class 3 with probability
0.03, class 780 with probability 0.72, class 999 with probability
0.05 and all other classes with 0. so, the classification vector for this will be:
To make sure these probabilities add to 1, we use the SoftMax function. This SoftMax function is defined as :
After this we take the 5 most probable candidates into the vector.
And ground truth vector can be defined as:
Error function can be refined as:
So, the loss function can be written as: Given a domain = {, ()}, (1)
Then is the feature map, and (X) is the probability distribution, = {1, ···, } and
{1, ···, } then the learning task is defined as
= {, = (|} (2)
Where is the set of all labels, and is the prediction function. The training data is represented by the pairs {, } where
,
. Suppose denotes the source domain
and denotes the target domain, the source domain data can be presented as: = {(1,
1), , (, )}, (3)
where is the given input data, and
is the corresponding label. The target domain DT can also be represented in the same way: = {(1, 1), , (,
)}, (4) where is the given input data, and
is the corresponding label. In almost all
real-life applications, the number of data instances in the target domain is significantly less than those in the source domain that
is 0 (5)
Definition For a given source domain and a learning task , with target domain and a learning task , then transfer learning is the use of knowledge in and to improve the learning of the prediction function in , given that and . Since any domain is defined by the pair = {, ()}, (6) where is the feature map, and () is the probability distribution, = {1, · ··, } , then according to the definition,
if and
() (). (7) Similarly, if a task is defined by = {, = (|} (8)
where is the set of all labels, and is the prediction function, then by definition, if
and, . (9)
Hence, the four possible scenarios of transfer learning are as follows: 1.
When both target and source domains are different, that is when and their feature spaces are also different, i.e.,
.
-
When the two domains are different,
and their probability distribution are also different, i.e. () (), where , and () ().
-
When the two domains are different,
and both their learning tasks and label spaces are different, that is S and,
, respectively.
-
When the target domain and a source domain are different, and their conditional probability distributions are also different, that is, when , where = (|), (10) and = ( |), (11) such that ,
. If both source and target domains
are the same, that is when = , the learning tasks of both domains are also the same (i.e. = ).
In this scenario, the learning problem of the target domain becomes a traditional machine learning approach and transfer learning
is not necessary. In ConvNet, transfer learning refers to using the weights of a well-trained network as an initializer for a new network. In our research, we utilized the knowlege gained by a trained VGGNET on ImageNet [61] dataset which is a set that contains 14 million annotated images and contains more than 20,000 categories to classify images containing mould, stain and paint deterioration.
-
Methods to enhance a classification accuracy:
Cross Validation: Separate your train dataset in groups, always separate a group for prediction and change the groups in each execution. Then you will know what data is better to train a more accurate model.
Cross Dataset: The same as cross validation, but using different datasets.
Tuning model: It's basically changing the parameters you're using to train your classification model. We are also using different things like adding more data, treating missing and Outlier values, Feature Engineering, Feature Selection, Algorithm Tuning, Ensemble methods, Cross Validation.
-
Things we are adding up for efficient classification:
-
Get More Data- One of the easiest ways to increase validation accuracy is to add more data. This is especially useful if you dont have many training instances and as we are using image recognition models, we have considered increasing the diversity of available dataset by employing data augmentation. These techniques include anything from flipping an image over an axis and adding noise to zooming in on the image.
-
Add More Layers- Adding more layers to the model increases its ability to learn your datasets features more deeply. This means that it will be able to recognize subtle differences that you, as a human, might not have picked up on. For complex tasks, such as differentiating between the category of cracks and flakes etc., adding more layers makes sense because the model will be able to learn the subtle features that differentiate a defected and unaffected area. For simple tasks, such as classifying defected and undetected, a simple model with few layers will do. But More layers -> More nuanced model.
-
Change Your Image Size- When you preprocess your images for training and evaluation, there is a lot of experimentation you can do with regards to the image size. If you choose an image size that is too small, your model will not be able to pick up on the distinctive features that help with image recognition. Conversely, if your images are too big, it increases the computational resources required by your computer and/or your model might not be sophisticated enough to process them.
-
Decrease Color Channels- Color channels reflect the dimensionality of image Most color images are composed of three-color channels while gray images have Just one channel. The more complex the color channels are, the more complex the dataset is and the longer it will take to train the model. Here color is not such a significant factor in our model, we are converting our color images to grayscale.
3.2.4 Fine-tuning
Another type of one that can actually outperform the feature extraction method. This method is called fine-tuning and requires us to perform network surgery. Fine-tuning is a way of applying transfer learning by taking a network which has already been trained for some given tasks and then applying a tune (or tweaking) the architecture of this network to make it perform a similar task. First, we take a scalpel and cut off the final set of fully connected layers (i.e., the head of the network where the class label predictions are returned) from a pre-trained CNN (typically VGG, ResNet, or Inception). By tweaking the architecture, we mean removing one or more layers of the original model and adding a new layer back to perform a new (similar) task. The number of nodes in the input and output layers in the original model also need to be adjusted in order to match the configuration of the new model. Once the architecture of the original model has been modified, we then want to freeze the layers in the new model that came from the original model. By freezing, we mean that we want the weights for these layers unchanged when the new (modified) model is being re-trained on the new dataset for the new task. In this arrangement, only the weights of the new (or modified) layers are updated during the re-training and the weights of the frozen layers are kept the same as they were after being trained on the original task.
The amount of change in the original model, i.e., the number of layers to be replaced, primarily, depends on the size of the new dataset and its similarity to the original dataset (e.g. ImageNet-like in terms of the content of images and the classes, or very different, such as microscope images). When the two datasets have high similarities, replacing the last layer with a new one for performing the new task is sufficient. In this case, we say, transfer learning is applied as a classifier. In some problems, one may want to remove more than just the last single layer, and add more than just one layer. In this case, we say, the modified model acts as a feature extractor.
Figure 2. VGG-16 model. Illustration of using the VGG-16 for transfer learning. The convolution layers can be used as features extractor, and the fully connected layers can be trained as a classifier.
3.4. Object Localization Using Class Activation Mapping (CAM)
Object detection is a complex problem that combines the concepts of image localization and classification. Given an image, an object detection algorithm would return bounding boxes around all objects of interest and assign a class to them.
Input: an image
Output: x, y, height, and width numbers around all object of interest along with class(es)
However, Image recognition is different from Object Localization reimage classification involves assigning a class label to an image, whereas object localization involves drawing a bounding box around one or more objects in an image. Object detection is more challenging and combines these two tasks and draws a bounding box around each object of interest in the image and assigns them a class label. Together, all of these problems are referred to as object recognition.
In the latter, when an algorithm looks at an image it is responsible for saying which class this image belongs to. For example, our model is responsible for saying this image is a Mould, or a Stain, or a Paint deterioration or Normal. In the localization problem however, the algorithm is not only responsible for determining the class of the image, it is also responsible for locating existing objects in any one image, and labeling them, usually by putting a rectangular bounding box to show the confidence of existence [64]. In the localization problem, in addition to predicting the label of the image, the output of the neural network also returns four numbers (x0, y0, width, and height) which parametrize the bounding box of the detected object. This task requires different ConvNet architecture with additional blocks of networks called Regional Proposal Networks and Boundary-Box regression classifiers. The success of these methods however, rely heavily on training datasets containing lots of accurately annotated images. A detailed image annotation,
e.g., manually tracing an object or generating bounding boxes, however, is both expensive and often timely consuming.
Class activation maps could be used to interpret the prediction decision made by the convolutional neural network (CNN). A CAM is a weighted activation map generated for each image. It helps to identify the region a CNN is looking at while
classifying an image. CAMs arent trained supervised, but in a weakly supervised fashion. This means that the objects do not have to be labeled manually and the localization is kind of learned for free. The only thing that has to be changed in the architecture is to get rid of the fully connected dense layers at the end in order to maintain the spatial information contained in the output of the last convolution layer. In addition, a global average pooling layer is aded afterwards In other words, CAM highlights the regions in an image which are relevant to a specific class by re-using classifier layers in the ConvNet for obtaining good localization results. The technique allows the visualization of the predicted class scores on an input image by highlighting the discriminative object parts which were detected by the neural network.
In order to use CAM, the network architecture must be slightly modified by adding a global average pooling (GAP) after the final convolution layer. This new GAP is then used as a new feature map for the fully-connected layer which generates the required (classification) output. The weights of the output layer are then projected back onto the convolutional feature maps allowing a network to identify the importance of the image regions. Although simple, the CAM technique uses this connectivity structure to interpret the prediction decision made by the network. The CAM technique was applied to our model and was able to accurately localized defects in images as depicted.
4. METHODOLOGY
The main aim of this research is to develop a model that classifies defects arising from dampness and classify them as mould, stain or deterioration, if they should appear in a given image, or as normal otherwise. In this work we also examine the extent of ConvNet role in addressing challenges arising from the nature of the defects under investigation and the surrounding environment. For example, according to one study, mould in houses can be black, brown, green, olive-green, gray, blue, white, yellow or even pink. Moreover, stains and paint deterioration do not have a defined shape, size or color and their physical characteristics are heavily influenced by the surrounding environment, i.e., the location (walls, ceilings, corners, etc.), the background (paint color, wallpaper patterns, fabric, etc.) and by the intensity of light under which images of these defects were taken. The irregular nature of the defects imposes a big challenge when obtaining an adequate large-enough dataset to train a model to classify all these cases.
So, we are collecting defected images from different sources to make our classification easier. The images were then appropriately, cropped and resized to generate the dataset which was used to train our model. To achieve higher accuracy, and exact location of detects instead of training a model from scratch, we adopted a transfer learning technique and used it in our model. Finally, the Class Activation Mapping technique was applied to address the localization problem.
-
Dataset
Images of different resolutions and sizes were obtained from many resources, including photos taken by mobile phone, a hand-held camera, and copyright-free images obtained from the internet. These images were sliced into 224×224 thumbnails to increase the size of our dataset producing a total number of 2622 images in our dataset. The data was labeled into four main categories: normal (image containing no defects), mould, stain,
Figure 3. Dataset used in this study. A sample of the dataset that was used to train our model using different types of damaged images.
and paint deterioration (which includes peeling, blistering, flacking, and crazing). The total number of images used as training data was 1890: mould (1000 images), flakes (700), paint deterioration (3000), and normal (500). For the validation set, 20% of the training data (400 images out of the 1890 images) was randomly selected. In order to avoid overfitting and for better generalization, a broad range of image augmentations were applied to the training set, including rescaling, rotation, height and width shift, horizontal and vertical flips. The remaining 732 images out of the 2622 were used as testing data with 183 images foreach class.
-
The model
For our model, we applied a fine-tuning transfer learning to a VGG-16 network pre-trained on ImageNet; a huge dataset of images containing more than 15 million annotated images and more than 30,000 categories. Our choice of using the VGG 16, is mainly because it is proven to be a powerful network although having a simple architecture. This simplicity makes it easier to modify for transfer learning and for the CAM technique without compromising the accuracy. Because accuracy is the main thing that we are focusing on in every aspect. Moreover, VGG-16 has fewer layers (shallower) than other models such as Inception. Generally Deep neural networks are harder to train. Since the VGG-16 has fewer layers than other networks, it makes it a better model to train on our relatively small dataset compared to deeper neural networks as figure 5 shows, accuracy is close, however VGG-16 training is smoother. VGGNet-16 consists of 16 convolutional layers and is very appealing because of its very uniform Architecture. Similar to Alex Net, it has only 3×3 convolutions, but lots of filters. It can be trained on 4 GPUs for 23 weeks. It is currently the most preferred choice in the community for extracting features from images. The weight configuration of the VGGNet is publicly available and has been used in many other applications and challenges as a baseline feature extractor.
However, VGGNet consists of 138 million parameters, which can be a bit challenging to handle. VGG can be achieved through transfer Learning. In which the model is pretrained on a dataset and the parameters are updated for better accuracy and you can use the parameters value.
The architecture of the VGG-16 model (illustrated in Figure 4) comprises five blocks of convolutional layers with max- pooling for feature extraction. The convolutional blocks are followed by three fully-connected layers and a final 1 X 1000 SoftMax layer (classifier). The input to the ConvNet is a 3-channel (RGB) image with a fixed size of 224 × 224. The first block consists of two convolutional layers with 32 filters, each of size 3 × 3. The second, third, and forth convolution blocks use filters of sizes 64 × 64 × 3, 128 × 128 × 3, and 256 × 256 × 3 respectively.
Figure 4. VGG-16 architecture. The VGG-16 model consists of 5 Convolution layers (in blue) each is followed by a pooling layer (in orange), and 3 fully- connected layers (in green), then a final SoftMax classifier (in red).
The input to the network is an image of dimensions (224, 224, 3). The first two layers have 64 channels of 3*3 filter size and same padding. Then after a max pool layer of stride (2, 2), two layers which have convolution layers of 256 filter size and filter size (3, 3). This is followed by a max pooling layer of stride (2, 2) which is the same as the previous layer. Then there are 2 convolution layers of filter size (3, 3) and 256 filters. After that there are 2 sets of 3 convolution layers and a max pool layer. Each has 512 filters of (3, 3) size with the same padding. This image is then passed to the stack of two convolution layers. In these convolution and max pooling layers, the filters we use is of the size 3*3 instead of 11*11 in Alex Net and 7*7 in ZF- Net. In some of the layers, it also uses 1*1 pixel which is used to manipulate the number of input channels. There is a padding of 1-pixel (Same padding) done after each convolution layer to prevent the spatial feature of the image.
After the stack of convolution and max-pooling layer, we got a (7, 7, 512) feature map. We flatten this output to make it a (1, 25088) feature vector. After this there are 3 fully connected layer, the first layer takes input from the last feature vector and outputs a (1, 4096) vector, second layer also outputs a vector of size (1, 4096) but the third layer output a 1000 channels for 1000 classes of ILSVRC challenge, then after the output of 3rd fully connected layer is passed to SoftMax layer in order to normalize the classification vector. After the output of classification vector top-5 categories for evaluation. All the hidden layers use ReLU as its activation function. ReLU is more computationally efficient because it results in faster learning and it alo decreases the likelihood of vanishing gradient problem.
-
Results Class prediction
The network was trained over 50 epochs using a batch size of 32 images and a step of 250 images per epoch. The final accuracy recorded at the end of the 50th epoch was 97.83% for training and 93.86% for the validation (Figure 5a). The final loss value was 0.0572 for training and 0.042 on the validation set (Figure 5b). The plot of accuracy in Figure 5.a, also shows that the model has trained well although the trend for accuracy on both validation, and training datasets is still rising for the last few epochs. It also shows that all models have not over-learned the training dataset, showing similar learning skills on both datasets
despite the spiky nature of the validation curve. Similar learning patterns can also be observed from Figure 5b as both datasets are still converging for the last few epochs with a comparable performance on both training and validation datasets. Figure 5 also shows that all models had no overfitting problem during the training as the validation curve is converging adjacently to training curve and has not diverted away from the training curve.
Inception model accuracy(96/ 95)
-
VGG-16 Model accuracy
-
VGG-16 Model loss
-
-
(a)Inception model loss (0.09 0.144)
Figure 5. Comparative models accuracy and loss diagram. In sub-figure a) the VGG-16 model final accuracy is 97.83% for training and 98.86% for the validation. In sub-figure b) the final loss is 0.0572 for training and 0.042 on the validation set. In sub-figure c) the ReseNet-50 model final accuracy is 96.23% for training and 95.61% for the validation. In sub figure d) the final loss is 0.103 for training and 0.102 on the validation set. In sub-figure e) the Inception model final accuracy is 96.77% for training and 95.42% for the validation. In sub-figure f) the final loss is 0.109 for training and 0.144 on the validation set.
To test the robustness of our model, we performed a prediction test on 732 non used images dedicated for evaluating our model. The accuracy after completing the test was high and reached 87.50%. A sample example of correct classification of different types of defects is shown in Figure 6. In this figure, representative images show the accurate classification of us model to the four classes: in the first row, mould prediction (n= 167 out of 183), in the second-row stain prediction, (n= 145 out of 183), in the third-row deterioration prediction (n=157 out of 183) and in the fourth row the prediction of normal class (n= 183 out of 183). An example of miss-classified defects is shown in Figure 7. The representative images in this figure show failure of the model to correctly predict the correct class. 38 images containing stains were miss-classified; 31 as paint deterioration and 6 as mould and 1 as normal. 26 images containing paint deterioration were miss-classified; 13 as mould and 13 as stain. 16 images containing mould were miss-classified; 4 as paint deterioration and 12 as stain.
The results in this figure illustrates an example where our model failed to identify the correct class of the damage caused by the damp. The false predictions for images by modern neural networks have been studied by many researcher [6872]. According to Nguyen et Al. [68], although modern deep neural network achieved state-of-the-art performance and are able to classify objects in images with near-human-level accuracy, a slight change in an image, invisible to human eye can easily fool the neural network and cause it to miss-label the image. Guo et al. argues that this is primarily due to the fact that neural networks are overconfident in their predictions and often outputs highly confident predictions even with meaningless inputs [70]. In this work Guo et al studied the relationship between the accuracy of neural network and the predictions scores (confidence). According to the authors, a network with a confidence rate equal to accuracy rate is a calibrated neural network. However, they concluded that, although the capacity of neural networks has increased significantly in the past years, the increasing depth of modern neural networks negatively affects model calibration [70].
Correct Classification Incorrect classification
Our model has performed very well on detecting images with mould with success rate of around 91%. The largest number of miss-classified defects occur in the stain and deterioration classes, with approximately 85% success rate in classifying stain and also 80% success rate in classifying paint deterioration (Figure 8). In Table 1, it can be seen that the overall precision of the model ranges between 82% for detecting deterioration, 84% for mould, and 89% for stain. The recall analysis shows similar results, with 82% for detecting deterioration, 90% for mould, 99% for normal, and 89% for stain. Note that the precision which is the ability of the classifier to label positive classes as positive is the ratio tp / (tp + fp) where tp is the number of true positives and fp the number of false positives. The recall quantifies the ability of the classifier to find all the positive samples, that is the ratio tp / (tp + fn) where fn is number of false negatives. F1 Score is the weighted average of precision and recall, that is
F1 Score = 2*(Recall * Precision) / (Recall + Precision).
Confusion matrix
-
DISCUSSION AND CONCLUSIONS –
The work is concerned with the development of a deep learning-based method for the automated detection and localization of key building defects from given images. This research is part of work on condition assessment of built assets. The developed approach involves classification of images into four categories: three categories of building defects caused by dampness namely: mould, stain and paint deterioration which includes peeling, blistering, flacking, and crazing of these defects and a fourth category Normal when no defects are present.
-
FUTURE SCOPE –
We are proposing a model which is very accurate to classify whether the buildings are defected or not so that if there is any situation of occurring earthquakes or cyclones in areas where the identification of building strength becomes difficult so at that time by using this application it is simple to predict building stability so that we can easily prevent so many lives before they get into end.
-
LIMITATIONS –
-
We are not considering multi classification in a single image i.e., it is not classified multiple defects at a time so that in the future works, these limitations will be be considered to be able to get closer to the concept of a fully automated detection.For our classification problem, we applied a fine-tuning transfer learning to a VGG-16 network pre-trained on ImageNet. A total of 2622 224x 224 images were used as our dataset. Out of the 2622 images, a total 1890 images were used for training data: mould (534 images), stain (449), paint deterioration (411) and normal (496). In order to obtain sufficient robustness, we applied different augmentation techniques to generate larger datasets. For the validation set, 20% of the training data (382 images) was randomly chosen. After 50 epochs, the network recorded an accuracy of 97.83% with 0.0572 loss on the training set and 98.86% with 0.042 loss on the validation. The robustness of our network was evaluated on a separate set of 800 images, 183 images for each class. The evaluation test showed a consistent overall accuracy of 87.50% and %90 of images containing mould correctly classified, 82% for images containing deterioration, 89% for images containing stain and 99% for normal images. To address the localization problem, we integrated the CAM technique which was able to locate defects with high precision. The overall performance of the proposed network has shown high reliability and robustness in classifying and localizing defects. The main challenge during this work was the availaility of large labeled datasets which can be used to train a network for this type of problem. To overcome this obstacle, we used image augmentation techniques to generate synthetic data for a largely enough dataset to train our model.
REFERENCES
[1] Noel, A. B.; Abdaoui, A.; Elfouly, T.; Ahmed, M. H.; Badawy, A.; Shehata, M. S., Structural health monitoring using wireless sensor networks: A comprehensive survey. IEEE Communications Surveys & Tutorials 2017, 19, (3), 1403-1423. [2] Kong, Q.; Allen, R. M.; Kohler, M. D.; Heaton, T. H.; Bunn, J., Structural health monitoring of buildings using smartphone sensors. Seismological Research Letters 2018, 89, (2A), 594-602. [3] Song, G.; Wang, C.; Wang, B., Structural health monitoring (SHM) of civil structures. In Multidisciplinary DigitalPublishing Institute: 2017. [4] Annamdas, V. G. M.; Bhalla, S.; Soh, C. K., Applications of structural health monitoring technology in Asia. Structural Health Monitoring 2017, 16, (3), 324-346. [5] Lorenzoni, F.; Casarin, F.; Caldon, M.; Islami, K.; Modena, C., Uncertainty quantification in structural health monitoring: Applications on cultural heritage buildings. Mechanical Systems and Signal Processing 2016, 66, 268-281. [6] Oh, B. K.; Kim, K. J.; Kim, Y.; Park, H. S.; Adeli, H., Evolutionary learning based sustainable strain sensing model for structural health monitoring of high-rise buildings. Applied Soft Computing 2017, 58, 576-585. [7] Mita, A. In Gap between technically accurate information and socially appropriate information for structural health monitoring systems installed into tall buildings, Health Monitoring of Structural and Biological Systems 2016, 2016; International Society for Optics and Photonics: 2016; p 98050E. Mimura, T.; Mita, A., Automatic estimation of natural frequencies and damping ratios of building structures. Engineering 2017, 188, 163-169. [8] Zhang, F. L.; Yang, Y. P.; Xiong, H. B.; Yang,J. H.; Yu, Z., Structural health monitoring of a 250 m supertall building and operational modal analysis using the fast Bayesian FFT method. Structural Control and Health Monitoring 2019, e2383.
[9] Davoudi, R.; Miller, G. R.; Kutz, J. N., Structural load estimation using machine vision and surface crack patterns for shear-critical RC beams and slabs. Journal of Computing in Civil Engineering 2018, 32, (4), 04018024. [10] Hoang, N. D., Image Processing-Based Recognition of Wall Defects Using Machine Learning Approaches and Steerable Filters. Comput. Intell.Neurosci. 2018, 2018.
AUTHORS BIOGRAPHY
Suchitra Reyya received her B. Tech degree from Gayatri Vidhya Parishad, JNTUH, Andhra Pradesh, India, in 2008 and MTech degree from Pydah college of Engineering, JNTUK, Andhra Pradesh, India, 2011. She was an Assistant Professor, in the department of computer science and
Engineering, VITAM Engineering College. She was an Associate Professor in the Department of Computer Science and Engineering, Lendi Institute of Engineering and Technology. She has 12 years of experience in teaching.
Sugandapu Hemalatha, currently pursuing B. Tech final year in the stream of computer science and engineering in Lendi Institute of Engineering and Technology.
Mutta Reshma, currently pursuing B. Tech final year in the stream of computer science and engineering in Lendi Institute of Engineering and Technology.
Srungaram Abhiram, currently pursuing B.Tech final year in the stream of computer science and engineering in Lendi Institute of Engineering and Technology.
Thallapudi Sasi Kumar, currently pursuing B. Tech final year in the stream of computer science and engineering in Lendi Institute of Engineering and Technology.