
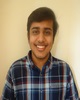
- Open Access
- Authors : Harsh Trivedi , Manav Shah
- Paper ID : IJERTV10IS090198
- Volume & Issue : Volume 10, Issue 09 (September 2021)
- Published (First Online): 01-10-2021
- ISSN (Online) : 2278-0181
- Publisher Name : IJERT
- License:
This work is licensed under a Creative Commons Attribution 4.0 International License
An Exploratory Analysis on Indian Netflix’s Genres
1st Harsh Trivedi
Department of Information Technology SVIT,VASAD, Gujarat, India
2nd Manav Shah
Department of Information Technology SVIT, VASAD, Gujarat, India
AbstractThis paper aims to construct a brief analysis of the content acquired by Netflix India till the year 2019-20. It works on integrating and evaluating the factors that hold weight forthe determinability of the content to be added. It examines the factors like which specifics genre is preferred by Indian market and how all other genres stand out in the watch time. Another aspect that was considered for the report was to verify the role of success rations of content with acquiring it in different timescales.
Index TermsContent Analysis in Acquisition time scale, Coreanalysis, Top 3 Genre According to year.
-
INTRODUCTION
To study and deduce the relation, we must initiate with our data. The section of the data we use for the analysis includes such parameters : 1. Genre 2. Content-type (Movie or TV show) 3. Rating 4. Release year 5. Year of Acquisition Another parameter included in Data is the names of content which statistically possess not much importance. The Data in inspection consists of 768 distinct entries noted over the years. The data has been collected from reputed open source Database of Kaggle and over it, other utility parameters were added without losing its credibility and reliability. The data that had been provided included greater noises and little acute relational scopes if analyzed directly. Thus, the data set was tidied on the factor of exclusive release in India and was takeninto the examination. The Genre and Rating are taken into account, sources from the platform like IMDb and in cases Netflix itself. However, for the consideration of parameters like Names, Acquisition dates and Release details it comes from the data provided on the platform. The sources and accuracy of details of it are presented by them. To demonstrate the data in the tier of Content type, here is in Fig 1 and Fig
-
To go through figures, there are 716 movies and 52 TV shows in the considered dataset. Which on calculating results in 93.229% of movies and 6.77% of the TV shows in the data considered. As witnessed, the Movie category dominates the content type in data and insights that more movies have been preferred to be added on the Netflix platform.
-
-
CORE ANALYSIS
Now to explore the horizons of various parameters in the data, we visualize the data and analyze it for a deeper interpretation.
Fig. 1. The graph is generated using matplotlib in Python
Fig. 2. The graph is generated using R environment
-
Genre vs Count
Firstly, to understand the basic and most important variable of the data, we process the quantification of Genre in graphicalterms. On plotting the graph its clearly seen, we can clearly list out of the top 3 Genre as: 1. Drama (25.91%) 2. Comedy (10.54%) 3. Drama- Romance (07.55%) Out of 47 distinct genres. To differentiate the Genre in the category of Content type and take a deeper perspective, we added a third variable in the plotto measure the variation and visualized it.
As shown in the previous figure, movies dominate content type which can be verified here too. On other interpretations, we can say even in TV shows as an independent variable, the Drama genre holds a lead in it. The major TV shows are not produced in many diversified categories of Genre. Going via figures to see the percent of Genre a Content-type withholds:
-
Drama Tv Shows (06.53%) Movies (93.46%)
-
Comedy – Tv Shows (09.87%) Movies (90.12%)
-
Drama-Romance TV shows (00.00%)
Movies (100%)
On seeing figures we can deduce that the Drama Romance category has no TV shows. Over Quantitative statistics on numbers we can tell that Comedy holds major TV shows as overall its production is less than Drama, even if Drama holds more no. of shows Drama – [ 13 out of 199] (i.e. There are199 total drama shows)
Comedy – [8 out of 81]
-
-
Genre vs Rating
Now to get major deductions and interrelations we compare Genre and IMDb rating. Here, we plot a line plot of Genrev/s Rating considering the third variable as content type to see better distribution and weightage of variables.
From earlier deductions we can tell that major rating values must be present in the Drama genre, which can be verified here too. On the parochial view of the graph we can say that TV shows possess better ratings than Movies. Even the highestrating in the content type is held by TV shows (9.2 IMDb). The average rating of the whole dataset is 6.35. IMDb. Range of the dataset is 7.5 Now to see the average rating of top 3 genre we plot graphs:
Drama: Movies [6.63 IMDb] and TV show [7.08 IMDb]Net average of Drama genre in General – 6.65 IMDb
Comedy: Movies [5.8 IMDb] and TV show [6.7 IMDb]Net average of Drama genre in General – 5.88 IMDb
Drama- Romance – Movies [6.56 IMDb]
TV shows [NULL]
Net average of Drama-Romance genre in General – 6.56IMDb
As there are no TV shows in the Drama-Romance genrethe net average and Movies average is collided. To parochiallyanalyze standalone production of TV shows in specific Genres we can see some genre like History (8.9 IMDb) and Biog- raphy(7.2 IMDb) have performed above the average and for other genre in similar classification such as Reality(5.7) and Action- Documentary (5.7) have underperformed, leaving the analysis for it obscure.
-
Quantifying genre vs Rating
Now to see the Genre and rating in Quantitative perspectiveand to analyze how the production of specific Genre is quantized for consumption of the public and how its success affects its next production, we plot the density graph for visualization.
On visualizing, we can see the lighter shades define max- imum density of content generated. Also, in the graph we defined the average line in orange. We consider the Drama genre in this graph, and we can see that it holds the lightest shade in the graph as it consists of maximum release. Herethe 1st lightest part is just above the average line and the majority of the second lightest part just below the average line. Suggesting that production is not diversified across the ends of axis(i.e. Super hit movies or Flop movies) rather is lying in the middle part of the graph generating the factor for Average line being in center. Similar trends can be seen in the majority. On
dividing the graph on parameters of content type, we can see the general trend repeating back in the Movie subdivision as it holds the privilege of quantity over TV shows. In subdivision of TV shows it holds darker shades representing low numbers as known.
-
Content-type released per decade
Another crucial factor in the analysis of data is the timeline of release decades of the content which has been acquired by the platform. It helps us to create an understanding of the content that has been acquired considering the predilection of people for the latest release or retro one assuming that the Netflix acquiring rights according to demand.
As seen in the graph, the major addition on the platform has been selected from the releases of 2010-20 decade. Also, TV shows additions are from the same decade.
The evident range of the TV show we consider in above figure which has been sorted year wise. As seen the range of TV shows added spans from 2009 to 2020. Now as considering timeline we add the parameter of Genre to the set to exlore the part and effect of it on the category. We append the term of release on top 3 genres to analyze the quantification.
-
-
TOP 3 GENRE TREND VISUALIZATION ACCORDING TO YEAR
-
Drama
As discussed earlier, the trend of the general genre extends to drama as major additions to it come from the latest decades than prior ones which can be seen below. To analyze further we consider the Drama genre average line and compare the rating.
Here we can see the distinction and variance of the content across the average line of Drama genre. We can see the ratingsare diversified.
Also, in year 2019 content rating has sort of equal diversification across the average line which in actual case plays an important role for whole data as it holds weight in quantity.
-
Comedy
The similar analysis can be extended for the Genre as done previously. The addition in category is steep and lacking from the earlier years and focused upon the latest decades.
Comedy genre in the decades of 2000s and reaching till 2010-20. But still the Drama-Romance of 60s are preferred for addition than like in comedy getting its source from 2000. For which can we say either there is a scarcity of Comedy content in the 60s or the viewer demands and acquisition is limited to the latest decades of 2000-2020.
-
Drama-Romance
The similar analysis can be extended for the Genre as done previously.
-
-
SUMMARY FOR TOP 3 GENRE
To give an overview of all the three genres presented above and resume their details as a single entity, the summary graph has been plotted. It represents a perfect interrelation of top3 genres with itself and other two parameters of release year and quantity.
-
TIME SERIES ANALYSIS
-
Content analysis on factor of acquisition time-scale
On statistical note:
-
2016 Movie: 0.018% of total acquisition
TV Show: NULL
-
2017 Movie: 21.09% of total acquisition TV Show: 1.82% of total acquisition
-
2018 Movie: 42.44% of total acquisition TV Show: 2.47% of total acquisition
-
2019 Movie: 27.34% of total acquisition
TV Show: 2.34% of total acquisition
-
2020 Movie: 0.78% of total acquisition TV Show: 0.13% of total
On direct observation of the graph we can notice the up- down hierarchy in the color pattern in terms of Quantity. As Drama in green has the maximum number of entries in the dataset, it leads to the top while blue at the bottom represents the minimum entry (#3) in the dataset of observation. We can notice the rise of the
acquisition
-
-
Relation between time of acquisition release year
Earlier, we saw the relation of the content quantity with the timeline of data acquisition, and we derived the statistical figures regarding it. In this section we try to examine therelation of the two major timelines of content i.e. Releaseyear and year of addition on the platform. We attempt to see how both factors affect each other. From the graph we can easily deduce that during the acquisition year of 2016 mainly the new content was featured. Which changes for early 2017 where older released content is acquired. That rapidly shifts back again and latest content is acquired from then till late December 2019 where maximum content is acquired in a balanced manner.
-
Rate of acquisition of content in terms of rating
On the vivid scale of presentation we can see the plot with quantity scale top to bottom. Here, we consider the third variable of rating to elucidate the influence of rating (success ratio) in the quantity scale of acquisition i.e. we can see if bulk acquisition considers the factor of rating of content. As shown, the Greener point indicates a major success rating and the redder one reflects the lower one. The gray- scale point represents the content which hasnt been rated. On examination of scale we can clearly evidence that in the bulk acquisition the higher rating factor can be seen, also in the single or minor acquisition, above average content is preferredunless the content has been just released and acquired by platform(e.g: For Aug-2019 only 2 content was acquired the older content has a greener rating but the one just released in 2019 has red as rating was not considered)
major addition of content has been added from the last two decades rather than to be distributed from the 70s. In the case of the major content acquisition of all time, we can say a balanced selection was done from the timeline. For the aim to analyze the trend of data acquisition depending on rating we saw major acquisition depends on the factor of quantity as well. In bulk acquisition and handful acquisitions mostly successful moviesare considered. On visualizing the part with release year we deduced the possibility that latest releases are not consideredin parameters of rating. They are acquired on the preferenceof popularity rather than success expectation.
VII. REFERENCES
-
R graph Gallery : https://www.r-graph-gallery.com/
-
R Documentation : https://www.rdocumentation.org/
-
Pandas : www.learndatasci.com/tutorials/python-pandas – tutorial-complete-introduction-for-beginners/
-
Seaborn: https://seaborn.pydata.org/tutorial.html
-
Matplotlibh: ttps://matplotlib.org/tutorials/index.html
-
Seaborn gallery: https://seaborn.pydata.org/examples/index.html
-
Matplotlib gallery:
https://matplotlib.org/3.1.1/gallery/index.html
VI. CONCLUSION
Upon examining and visualizing data, the principle question of which data leads the Indian market, can be answered as Drama with a great credibility of data and graphs. Majority content in Indian market is affiliated with Drama as a major in it such as Drama-Romance,
Drama, Sport etc. Other
major affiliation comes in the genre Action which in our considered dataset ties on number of affiliation as 10 with Drama. We can conclude the top 3 genres available in Indian market are Drama, Comedy and Drama- Romance respectively.Also, as graphically deduced, the