
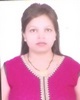
- Open Access
- Authors : Pooja Verma , Swastika Chakraborty
- Paper ID : IJERTV11IS030175
- Volume & Issue : Volume 11, Issue 03 (March 2022)
- Published (First Online): 05-04-2022
- ISSN (Online) : 2278-0181
- Publisher Name : IJERT
- License:
This work is licensed under a Creative Commons Attribution 4.0 International License
Analyses of Modeling Forecasting for the Monthly Rainfall In Shillong, Meghalaya, India
Pooja Verma
Research Scholar:
Electronics and Communication Engineering Dept.
Sikkim Manipal Institute of Technology, Sikkim Manipal University,
Majitar, Rangpo, India.
Swastika Chakraborty
Associate Professor:
Electronics and Communication Engineering Dept.
Sikkim Manipal Institute of Technology, Sikkim Manipal University,
Majitar, Rangpo, India.
Abstract- The purpose of this paper is analyses a regression- based modelling for rain prediction utilising historical rainfall data from India's hill stations, specifically Shillong, Meghalaya, India. Rainfall forecasting is required for social benefits such as preventing landslides, which are common in tropical areas. This research analysed historical data from 1980 to 2018 to construct an ARIMA model for predicting monthly rainfall in Shillong. Rainfall throughout this time was used to train the model, which was then tested and validated. The best fit model, ARIMA (1, 2,
-
and (1, 0, 1) was chosen using the Bayesian Information Criterion (BIC). The model forecasts matched the observed data perfectly. Model's exceptional precision is outstanding in predicting monthly rainfall over a 39-year period warrants its future implementation in the study region.
KeywordsARIMA; Shillong; Bayesian; rainfall
-
INTRODUCTION
Climate change has emerged as this generation's is the most important concerns. Climate change has been resulted in exceptionally significant cumulative losses when compared to other challenges [1]. Because climatic conditions will be change with respect to long-term changes, meteorological variables, the most important of which is rainfall, are vital to track. Rainfall variability assessment is a major challenge that necessitates the previous quantification. Not unexpectedly, rainfall time- series has been analysis and forecasting have become increasingly an important around the world [2, 3]. Rainfall forecasting is usually done at a relatively broad spatial scale. Rainfall is expected over India or in specific states [4, 5]. To evaluate the time-series models, some well- known time series forecasting models are utilized together with their functionalities [6]. The act of predicting the future by studying the past is known as time series forecasting. Some of the strategies employ frequency decomposition to differentiate between regression modelling and other techniques [7]. Some research has shown that hybrid architecture is more reliable and accurate than individual forecasts [8]. The hybrid version, which combines two techniques, single spectrum analyses (SSA) and ARIMA [9], and models based on the evaluation of the measured records prediction approach of metro wheels based on ARIMA (p, d,
q) models, is also utilized in the literature [10]. An ARIMA model combines three operators: an autoregressive (AR) function applied parameter (rainfall), a moving average (MA) applied completely and last integration (I). The ARIMA modelling approach was used at a finer scale in this study,
over Shillong. Finally, the model predicts rainfall for 39 years. In the following sections, we will go over the study area in detail, as well as the ARIMA modelling and results. As a result, we need to analyses the rainfall to investigate a suitable model in the state such as Shillong, Meghalaya, India. This work is to investigate the most appropriate model for Shillong to address orographic precipitation using regression-based models.
Fig.1. Picture of Land-slides in Shillong, Meghalaya, India [11]
-
Study area and data
Shillong has been chosen as the location for this study which lies in Meghalaya, India. The area of Shillong is 64.36 km2. This is the capital of the state Meghalaya which is very well known for its climate and beautiful nature. The district's coordinates are 25°.34°N and 91°.53°E, As Shillong is a hilly rainfall region so there are many possibilities of landslides. Number of landslides have been seen in the previous year. The landslides occurred in Shillong is captured shown in fig.1. Due to this, the roads were blocked, people were facing the problems to not to be travelled for so many hours and it is the serious problem of natural disaster which affect nature and human lives. So, we need to protect the environment and human lives from this by doing the prediction of the rainfall so that we have the early alarm signal to detect landslides and other natural disasters.
Fig.2. Study Area of Shillong, Meghalaya, India [12]
-
Methodology
This study forecasts rainfall over 39 years, which is a long time in meteorological terms. The ARIMA model forecasts future rainfall based on historical data. The seasonal effect is mathematically modified in the ARIMA model,
p(B)p (Bs) (1-B)dyt= q(B) Q(Bs)ut (1)
Where P is the number of seasonal autoregressive parameters, Q denotes the number of seasonal moving average orders, s denotes the period length (one month in this case), and D denotes the number of differencing the passes.
-
-
RESULTS
The model is trained for 19802018, the best model is estimated by minimum BIC values. The ARIMA (1, 2, 1) and (1, 0, 1) is the best fit models of the observed data. In this scenario, these indicates that seasonal ARIMA (SARIMA), which is frequently used to model having a large seasonal component. The model (1, 2, 1) and model (1, 0, 1) are identical. Rainfall in India is generally known to be extremely seasonal, increasing during the monsoon months and being scant during the rest of the year. As a result, the seasonal ARIMA is more accurate at forecasting rainfall of study area. Any empirical model's performance, however, should be evaluated before it is employed. The model's ability to forecast rainfall from 1980 to 2018 is tested here. Fig. 3 and Fig.4 show a comparison of the forecasting of rainfall.
Fig.3. Predicted rainfall for Shillong for model (1, 2, 1).
Fig.4. Predicted rainfall model ARIMA (1, 0, 1).
To gain a better understanding of the variation of the data, actual time series data from the last 39 years is seasonally changing. The seasonal consideration of the ARIMA model accounts for the seasonal variation in the data series. In the above figure, the graph of the ARIMA model (1, 2, 1) and model (1, 0, 1) for predicted and observed rainfall shows the seasonal effect.
-
MOTIVATION
The aim of this research is to representation of a model with a very specific rainfall known as orographic rainfall in Shillong which is surrounding by the Himalayan hill while considering of the historical time series.
-
SPECIALITY
The hilly region experiences the rain i.e. very intense at lasts for a longer period. Rain, which accounts for 75% of total duration, has a big impact on the society that causing landslides and the other problems that should be predicted in advance to take any necessary precautions.
-
CONCLUSION
Climate change will have serious consequences, needing special attention to establish proper readiness, which can be achieved by precise rainfall forecasting. A model of rainfall for Shillong, Meghalaya, India is developed in this study. Using rainfall data collected over a 39-year period, and the results show excellent consistency between forecasted and observed values. Outstanding performance in predicting rainfall over a 39-year period applicable in the future over the region, will aid in the development of better planning and management practces.
-
ACKNOWLEDGMENT
-
Authors thankfully acknowledge the ISRO- RESPOND program, and T. M. A. Pai Research Grant, Sikkim Manipal University, India. The Giovanni online data system used in this study also thankfully acknowledged.
REFERENCES
[1] A.K. Gosain., S. Rao, D. Basuray, Climate change impact assessment on hydrology of Indian River Basins,. Curr. Sci. 90(3), 346353 (2006) [2] S. Swain, M. Verma, Statistical trend analysis of monthly rainfall for Raipur District, Chhattisgarh, Int. J. Adv. Eng. Res. Stud./IV/II/Jan- March, 8789 (2015) 4. Swain, S.: Impact of climate variability over Mahanadi river basin. Int. J. Eng. Res. Technol. 3(7), 938943 (2014). [3] I.S. Jacobs and C.P. Bean, Fine particles, thin films and exchange anisotropy, in Magnetism, vol. III, G.T. Rado and H. Suhl, Eds. New York: Academic, 1963, pp. 271-350. [4] P. Narayanan, Basistha, A., Sarkar, S., Kamna, S, Trend analysis and ARIMA modelling of pre-monsoon rainfall data for western India, C. R. Geosci. 345(1), 2227 (2013). [5] S. Chattopadhyay, S., Chattopadhyay, G.: Univariate modelling of summer-monsoon rainfall time series: comparison between ARIMA and ARNN. C. R. Geosci. 342(2), 100107 (2010) Y. Yorozu, M.Hirano, K. Oka, and Y. Tagawa, Electron spectroscopy studies on magneto-optical media and plastic substrate interface, IEEE Transl. J. Magn. Japan, vol. 2, pp. 740-741, August 1987 [Digests 9th Annual Conf. Magnetics Japan, p. 301, 1982].
[6] Z. Farhath Asha, B. Arputhamary, and L. Arockiam. "A survey on ARIMA forecasting using time series model." Int. J. Comput. Sci. Mobile Comput 5 (2016): 104-109. [7] W. Jacobs, A. M. Souza and R. R. Zanini Combination of Box- Jenkins and MLP/RNA Models for Forecasting Combining Forecasting of Box-Jenkins, IEEE LATIN AMERICA TRANSACTIONS, 14( 4), 2016. [8] S. I. Vagropoulos, G. I. Chouliaras, E. G. Kardakos, C. K. Simoglou and A. G. Bakirtzis, "Comparison of SARIMAX, SARIMA, Modified SARIMA and ANN-based Models for short-term PV generation forecasting", IEEE International Energy Conference (ENERGYCON), Leuven 1-6, 2016. [9] Rodrigo N. Calheiros ; Dept. of Comput. & Inf. Syst., Univ. of Melbourne, Melbourne, VIC, Australia; Enayat Masoumi; Rajiv Ranjan; Rajkumar Buyya, Workload Prediction Using ARIMA Model and Its Impact on Cloud Applications, QoS IEEE Transactions on Cloud Computing, 3(4), 2015 [10] G. Liu, D. Zhang and T. Zhang, "Software Reliability Forecasting: Singular Spectrum Analysis and ARIMA Hybrid Model", Theoretical Aspects of Software Engineering (TASE), 2015 International Symposium on Nanjing, 111-118, 2015 [6] Yun-Jian, Z. 2017. Changes in extreme precipitation events over the Hindu Kush Himalayan region during 1961-2012. Advance in Climate Change. 8: 166-175. [11] https://www.google.com/search?q=shillong+india+map&sxsrf=APq- WBuW6tBnkYYmiOnraGN1wzqEQhT- oQ:1648708267398&source=lnms&tbm=isch&sa=X&ved=2ahUKEwi0 8fb03O_2AhVYwTgGHTktDzUQ_AUoAXoECAEQAw&biw=1366& bih=657&dpr=1#imgrc=S8a2c2Id65d1PM [12]https://www.google.com/search?q=shillong+land+slide+picture&sxsrf= APq-WBu_lrct6J3jsx_puQW9dGE8eCBQtQ:1646008650195&tbm=isch& source=iu&ictx=1&vet=1&fir=g7eUBqtESSb00M%252CGyJk64eVO 57CwM%252C_%253B4W3b0_jeydAKsM%252C-kCxjYRXbWGj- M%252C_%253BRcRlj8f8spBUJM%252Cv2W0mUT36qekrM%252 C_%253BMsKNX3mZ4_8k- M%252CTzTENLmOwYHyDM%252C_%253B56pFUwRMuiEdlM
%252CFby5lqB0- G_1MM%252C_%253B7AHUMZZX7r5B2M%252Cnk5czp7pd8Aq
qM%252C_%253Bjv_DRMFpftPzAM%252CSrq3gMpnGLgu5M%2 52C_%253BpvXfPK74oNZ25M%252CvFtXbzAIvKZmmM%252C_
%253BDk7- 42_W8JZu7M%252C9HHZqkLQNgZYDM%252C_%253BklxfpjIfs GZOgM%252CpvdcHF61KGGNPM%252C_&usg=AI4_-
kRd9LxWec_iHGBMHttAO GxwREAw&sa=X&ved=2ahUKEwih 8JCHlKH2AhUgSGwGHaTsCDwQ9QF6BAgDEAE#imgrc=BvjITG 3c7MJOqM&imgdii=6V9c9rK3QjxEIM