
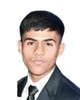
- Open Access
- Authors : Niev Sanghvi, Rahul Shankar Pachpande, Durgesh Hiralal Pawar, Tanishq Gandhi, Tejjas Bhingardevay, Paarth Pandey
- Paper ID : IJERTV14IS030194
- Volume & Issue : Volume 14, Issue 03 (March 2025)
- Published (First Online): 07-04-2025
- ISSN (Online) : 2278-0181
- Publisher Name : IJERT
- License:
This work is licensed under a Creative Commons Attribution 4.0 International License
Analysis of AI in Roulette – Machines Becoming Humans
Niev Sanghvi |
Rahul Shankar Pachpande |
Durgesh Hiralal Pawar |
The Bishops School Pune, Maharashtra, |
Kavayitri Bahinabai Chaudhari North |
P G College of Pharmaceutical Sciences |
India |
Maharashtra University, Jalgaon |
and Research , Nandurbar |
Tanishq Gandhi |
Tejjas Bhingardevay |
Paarth Pandey |
Vellore Institute of Technology, Vellore |
Savitribai Phule Pune University |
Bhavans b.p Vidya Mandir, Civil Lines |
AbstractThis research paper examines the integration of artificial intelligence (AI) into roulette games and examines whether machines can behave humanely in the context of gambling. By examining artificial intelligence algorithms and their performance in roulette games, this study aims to shed light on the evolving relationship between technology and human decision-making in the field of gambling. Analyzing the ability of artificial intelligence to imitate human strategies in roulette, this study explores the ethical implications and possible future consequences as machines become increasingly human in gaming scenarios.
-
INTRODUCTION
-
AI in Roulette Games:
Artificial intelligence (AI) is a branch of computer science that enables machines to perform tasks that normally require human intelligence, such as problem solving, learning and decision making. In the context of roulette games, artificial intelligence algorithms are used to analyze data, predict outcomes and optimize strategies to improve playability. These AI systems can simulate human behavior by processing massive amounts of data and making calculated decisions to improve their game performance.
AI Algorithms in Roulette :
The AI algorithms in roulette games are designed to analyze historical data, predict outcomes and optimize betting strategies.These algorithms use machine learning techniques to identify patterns in data and make informed decisions during gameplay.
Simulation of Human behavior :
The purpose of artificial intelligence in roulette games is to mimic human decision-making processes by adapting to changing game conditions and changing strategies accordingly.By simulating human behavior, AI systems can improve their performance and competitiveness in the game.
Predictive Modeling :
Roulette AI technologies often use predictive modeling to predict possible outcomes and recommend optimal betting strategies.Through advanced statistical analysis, these models can improve the chances of AI players succeeding in the game.
Risk assessment and management :
AI in roulette games also focuses on risk assessment and management, assessing the probabilities of different outcomes and determining the more favorable options.By integrating risk analysis into decision-making processes, AI systems can minimize losses and maximize profit opportunities.
Impact on gameplay :
The integration of artificial intelligence into roulette games not only affects the specific game, but also contributes to the overall development of the gaming experience.AI-based roulette systems can offer players unique challenges,
advanced strategies and new approaches to traditional betting practices.
Ethical aspects :
As artificial intelligence technology develops in the field of gambling, ethical aspects regarding the justice, transparency and responsible use of artificial intelligence systems in gaming environments appear.Understanding the ethical implications of AI in roulette games is important to promote ethical behavior and ethical decision-making among players and developers.
-
The Role of AI in Games :
Artificial intelligence (AI) has a rich history dating back to the mid-20th century, when pioneers such as Alan Turing and John McCarthy laid the groundwork for the field. Artificial intelligence has evolved significantly over the years, from early rule-based systems to advanced machine learning algorithms and neural networks. In gaming, AI has played an important role in creating intelligent opponents, designing challenging levels, and improving the overall gaming experience. Games like chess, go and poker have been central to showcasing the capabilities of AI, demonstrating its ability to learn, adapt and compete with human players.
Significance of AI in gambling with a focus on roulette:In terms of gambling, AI has revolutionized the way games like roulette are played and analyzed. Roulette uses artificial intelligence algorithms to predict outcomes, optimize betting strategies and simulate human decision-making processes. Utilizing data analytics and machine learning techniques, AI systems can process vast amounts of data to make informed decisions during gameplay. Integrating AI in roulette not only improves players' gaming experience, but also raises ethical considerations for the use of AI in gambling scenarios.
Development of Artificial Intelligence in Roulette Games:The application of artificial intelligence in Roulette games has advanced greatly over the years through algorithm design, data processing capabilities and predictive modeling techniques. Roulette's AI systems can now analyze complex patterns, adapt to changing game conditions and create strategies based on real- time data. These innovations have transformed the traditional game of roulette, offering players unique challenges and opportunities to make strategic decisions.
The Ethical Impact of AI on Gaming: AI technologies continue to shape the gaming landscape, and ethical considerations become paramount to ensure fair play, responsible gaming practices, and transparency in algorithmic decision-making. The integration of artificial intelligence in roulette raises questions about data
protection, algorithms and potentially addictive gaming. Understanding and addressing these ethical implications is essential to creating a safe and sustainable game environment where AI and players can coexist harmoniously.
Fig 1. Roulette Table Layout
-
-
PROBLEM STATEMENT
The rapid integration of artificial intelligence (AI) into various fields is increasing its influence in the gambling industry, especially in games such as roulette. This study examines AI's ability to think critically and its impact on machines that mimic human behavior in a gaming context. Despite technological advances that allow artificial intelligence to mimic human decision-making, a number of challenges and ethical issues arise. These include accurately modeling the multifaceted nature of human cognition, addressing potential biases in AI training data, and ensuring fair play and responsible gambling practices.
This research paper aims to answer the following critical questions:
-
To what extent can artificial intelligence systems mimic human critical thinking and decision-making processes in a roulette game?
-
What are the ethical implications of AI's ability to predict game outcomes and optimize betting strategies?
-
How does the complexity of human behavior limit the performance and generalizability of AI models for the video game industry?
-
What future developments will AI need to improve idividual gaming experiences while maintaining responsible and fair gaming standards?
-
-
LITERATURE REVIEW
-
Historical context :
The development of artificial intelligence and its role in gambling:The development of artificial intelligence (AI) dates back to the middle of the 20th century, with notable contributions from pioneers such as Alan Turing and John McCarthy.In gambling, artificial intelligence has played a key role in improving player experiences, changing game dynamics and eliminating traditional game paradigms.The original AI systems in games focused on rule-based strategies and gradually evolved into more advanced machine learning algorithms capable of adaptive learning and decision making.
-
Artificial Intelligence in Gambling :
Applications in Casino Games, Including Roulette:The study of artificial intelligence in casino games, particularly roulette, emphasizes the use of artificial intelligence algorithms in data analysis, predictive modeling, and strategic decision- making.The purpose of artificial intelligence in roulette games is to optimize betting strategies, predict outcomes and simulate human behavior to improve the game.Research in this field examines the effectiveness of artificial intelligence systems in improving winning probability, understanding player behavior, and exploring new innovations in casino games.
-
The Ethics of Artificial Intelligence Human-like Aspects: The ethical aspects of artificial intelligence and its human-like qualities are of greatest importance in the context of gambling.Debates about the ethics of AI in the gaming industry discuss, for example, the transparency of algorithmic decision- making, data protection issues and the impact of AI on responsible gaming practices.As casino gaming AI systems become increasingly sophisticated and capable of mimicking human behavior, questions arise about fairness, accountability and the impact of AI on the gaming ecosystem.
-
Emerging Trends and Future Directions :
The literature review also sheds light on emerging trends in AI applications in gambling, emphasizing the need for ongoing research and dialogue on ethical frameworks and guidelines for AI integration.
Future directions in AI research for casino games like roulette may focus on enhancing player experiences, creating more inclusive gaming environments, and addressing the social impact of AI technologies on gambling behaviors.
In particular, we use one of the well-known roulette strategies, doubling bets or Martingale strategy in the simulation model. It is easy for students to believe that the Martingale strategy is a
failed system (O'Connor and Dickerson [16]). A simulation model,easy to tabulate, can be used to study the strategy and show the inherent logical fallacy.Games can be exciting examples of teaching. They can generate students' interest in the subject and provide better retention, understanding and general learning (Leemkuil,et al., [13]). Simulated games have proven useful both for training practitioners and for teaching in traditional classrooms. Dempsey et al., [7] presents various characteristics of games that can be used in an educational environment.In addition, studies show that simulation can be used to teach various subjects such as statistics (Doane, [8]), economics (craft, [6]), organizational development(Ruohomaki, [18]), business. Education (Chang et al., [5]) and operations management/management science (Thiriez, [21], Seal, [19], Al-Faraj et al., [1]). Mills [15]provides an extensive literature review on the use of computer simulation methods in teaching statistics, while Yeates [22] provides a very extensive bibliography on the use of simulation in business games.In this article, we combine game and simulation using familiar \software to students: Spreadsheet. Since Bodily [4], spreadsheets have become a very popular tool for developing and implementing many types of simulation models. Spreadsheets are almost ubiquitous in today's personal computers and are accepted by end users as an analytical tool. They provide an intuitive and easy to understand user interface and allow the creation of advanced simulation models with relative ease. Simulation utility packages such as Crystal Ball, PopTools or @RISKcan provide a very powerful simulation environment for solving large complex problems. The virtues of spreadsheets as a simulation and modeling tool and examples of its application in several fields are mentioned, as well as their use in teaching statistics and probability concepts, quantitative modeling and simulation, and operations management. many researchers (Leon et al., [14], Bodily, [4], Ragsdale, [17], Kohler,[12], Anderson et al., [3], Ammar and Wright, [2], Grossman , [10] , Eppen et al.For these advantages, we used a spreadsheet as an implement to simulate roulette. There and the earlier article by Przasnyski [20]presented the basic mechanisms of a roulette table simulation model with simple betting strategies.
-
-
METHODOLOGIES
-
Data Preparation and Feature Engineering :
Data preparation and feature Engineering play a key role in improving the accuracy and reliability of predictive models. The process begins with the collection of historical
roulette data, which includes various game parameters such as winning numbers, betting patterns and results. These data sets are then subjected to careful data cleaning to correct missing values and outliers and ensure data consistency. Feature engineering involves extracting relevant features from a dataset, including reading scores, color sequences and past winning streaks, to create informative input variables for an AI predictive model. By organizing the data meaningfully, feature design aims to capture the patterns and relationships behind the dynamics of the roulette game, allowing the AI model to make informed predictions based on historical trends and player behavior. In addition, preprocessing steps such as normalization and scaling are used to standardize the input data and facilitate optimal model performance during the training and prediction phases. Data preparation and feature design lay the foundation for building robust AI models that can effectively predict roulette outcomes through strategic selection and transformation of relevant inputs derived from game history.
Data collection :
Collect historical roulette data including past winning numbers, betting patterns and results.
Feature engineering :
Extract relevant features from the data, such as reading scores, color patterns, and past winning streaks, to create input variables for an AI predictive model.
Data cleaning :
Pre-processing the data to deal with missing values and outliers and to ensure the consistency of the dataset.
Graph 1. Depicting the Data Collection
Graph 2. Showing Data Cleaning
Graph 3. Showing the data pertaining to Feature Engineering
-
Model Selection and Training :
Model selection and training play a key role in the development of AI systems, such as for analyzing AI in roulette. Model selection involves selecting the most appropriate algorithm to accurately predict outcomes based on the available data. This process requires a deep understanding of various models, from basic linear regression to more complex deep learning algorithms such as neural networks. Before training a model, data preparation is essential so that the input data is clean, properly formatted and ready for analysis. Feature engineering is another important step in which relevant features are selected and transformed for use in the predictive model. After the model is trained, it must be evaluated using performance metrics such as precision, accuracy, and recall to evaluate its performance. Methods such as cross-validation are used to confirm model performance on unseen data and to avoid over- or under- fitting. Hyperparameter tuning is also performed to optimize model performance by adjusting parameters such as learning rate and regularity. Understanding the trade-off between bias and variance is essential to achieving a balance between under- and over-fitting. To prevent the model from becoming too complex during training, regularization techniques such as L1 and L2 regularity are used. Ensample learning methods such as Random Forest and Gradient Boosting combine multiple models to improve prediction accuracy. Model interpretability is critical to understanding how the model
predicts, especially in complex scenarios such as roulette analysis. Using the trained model in real situations is essential to make predictions and gain valuable insights. Continuous monitoring of the model is essential to ensure that it adapts to changing data patterns and performs optimally. Ethical considerations related to bias, fairness and privacy must be considered when developing and using artificial intelligence models in sensitive areas, such as data analysis in a gambling context.
-
Evaluation Metrics and Performance Analysis :
Evaluation metrics and performance analysis are important aspects of evaluating the effectiveness of AI models in predicting roulette results. Metric selection involves defining evaluation measures such as precision, accuracy, recall and F1 score to measure model accuracy. These metrics provide insight into the model's ability to make correct predictions and identify potential areas for improvement. Performance analysis involves evaluating a model's predictions against actual results to assess its accuracy and reliability. By comparing predicted results with real data, researchers can measure the effectiveness of the model and make informed decisions about how to optimize it. Using a confusion matrix allows you to visually represent the model's true positive, true negative, false positive, and false negative predictions. This matrix helps to understand the distribution of forecast errors and provides a comprehensive picture of model accuracy levels. By carefully evaluating and analyzing these metrics, researchers can gain a deeper understanding of the AI model's performance and make changes to improve its predictive ability. This systematic approach to evaluation ensures transparency and reliability in evaluating the predictive accuracy of the model in predicting roulette results.
-
Fine-Tuning and Optimization :
Fine-tuning and optimization are critical processes to improve the performance of AI models, especially in analyzing roulette AI. Fine-tuning involves adjusting the hyperparameters of a model to improve its prediction accuracy and generalizability. By fine-tuning parameters such as learning, set, and regularization strength, researchers can optimize model performance. This iterative process requires a systematic approach to testing different hyperparameter settings and evaluating their impact on model performance. Optimization techniques aim to minimize the model's loss function by refining its parameters through iterative updates. Often, methods such as stochastic gradient descent and Adam's optimization are used to optimize model weights and slopes during training. Fine-tuning and optimization play a crucial role in maximizing the predictive power of the model and minimizing errors in predicting roulette results. Through careful hyperparameter tuning and optimization techniques, researchers can fine-tune the model for optimal performance. This systematic improvement process ultimately results in more accurate and reliable predictions, which improves the overall performance of the model in AI analysis of gambling
scenarios such as roulette. By applying fine-tuning and optimization strategies, researchers can ensure that their AI models are well-calibrated, efficient, and capable of making accurate predictions in real-world applications.
Graph 4. Depicting Accuracy% vs Model
Graph 5 . Performance Metrics of AI Models
Fig 2. Roulette wheel mapping
-
-
INTERPRETATION, LIMITATION AND FUTURE IMPLICATIONS
-
Interpretation :
The ability of artificial intelligence to mimic human behavior in games like roulette has exciting implications for the gambling industry and beyond. By imitating human decision- making processes, AI systems can potentially improve player experiences, optimize betting strategies and even more accurately predict outcomes. However, this raises ethical issues regarding fair play, responsible gambling and data protection. Understanding the nuances of artificial intelligence that mimics human behavior can provide insight into how the technology is reshaping traditional gaming dynamics and influencing how players interact with casino games.
-
Limitations :
Despite the best efforts of the study, certain limitations and shortcomings must be acknowledged. Limitations may include the complexity of accurately modeling human behavior, the reliance on historical data for AI predictions, and the potential bias of training data. Additionally, the scope of the study may not cover all the variables that affect AI performance in gambling scenarios. Acknowledging these limitations is necessary for transparency and identifying areas for further exploration and improvement in future research.One major limitation relates to the difficult challenge of accurately modeling human behavior in AI systems, as human decision-making processes are multifaceted and influenced by many factors. Additionally, relying on historical data for AI predictions presents limitations, as it may not capture all possible outcomes or unexpected variables in real-time gambling scenarios. Training data bias is another limitation, as biased or incomplete datasets can affect the predictive accuracy and generalizability of the model. In addition, the scope of the study may not cover all the complex variables that affect AI performance in a gambling context, which may limit the depth of the study's findings. By recognizing these limitations, researchers can pave the way for future research and improvement and ensure the integrity and quality of research in the dynamic field of AI and gaming.
-
Future Implications :
The future implications of advances in artificial intelligence technology for the gaming industry are profound. With the continuous development of AI algorithms, it is possible to
improve prediction functions, personalized gaming experiences and advanced risk assessment tools. Future advances in artificial intelligence could revolutionize how games like roulette are played, blurring the lines between human decision-making and machine intelligence. However, regulatory challenges, ethical considerations and the responsible use of AI in gaming must be carefully considered to harness the full potential of AI technology to shape the future of gaming.Critically analyzing the effects of AI imitation in games such as roulette, acknowledging the limits of research and anticipating the future development of AI technology and its impact on gambling, this lecture offers a holistic perspective on the intersection of AI and game dynamics.
Graph 6. Showing A line chart displaying how the actual outcome changes as the spin number increases.
Fig 3. Chart of Observed and Expected Values Blue-observed, red-expected
-
-
SOLUTIONS TO CRITICAL QUESTIONS
-
To what extent can artificial intelligence systems mimic human critical thinking and decision-making processes in a roulette game?
AI systems can mimic human critical thinking and decision- making to some extent, especially by analyzing large amounts of data and identifying patterns that humans might miss. For example, AI algorithms can track and analyze historical game results to predict future results. AI can still simulate consistent and informed decision-making, but it lacks the intuition and emotional factors that influence human decisions. The biggest limitation is that AI relies heavily on past data and predefined rules, while intuition, emotion and unique situational awareness can influence human decision-making.
Graph 7. To Illustrate the Imitation of Human Decision- Making by AI in Roulette
Graph 8. AI vs. Human Accuracy in Predicting Roulette Outcomes
-
What are the ethical implications of AI's ability to predict game outcomes and optimize betting strategies?
Using AI to predict game outcomes and optimize betting strategies raises a number of ethical issues:
Fair play: AI gives certain players an unfair advantage that can disrupt the integrity of the game.
Responsible Gambling: AI can encourage excessive gambling by suggesting strategies that minimize losses, which can lead some people to gamble more than they can afford.
Transparency: The algorithms used by AI are often complex and difficult for all players to understand, which raises questions about fairness and transparency.
Privacy: AI systems require access to a lot of data, which raises concerns about the collection, storage and use of player data.
Graph 9. Ethical Implications of AI Optimizing Betting Strategies
-
How does the complexity of human behavior limit the performance and generalizability of AI models for the video game industry?
Human behavior is complex and influenced by many factors such as emotions, personal experiences and psychological biases. These variables are difficult to quantify and incorporate into AI models. Therefore, AI models based mostly on historical data and logical
algorithms may not be able to account for the unpredictability of human decisions. This limitation affects the generalizability of AI models, meaning that a model trained on one dataset may not perform well in another context or with different players. Additionally, human creativity and adaptability can lead to strategies that AI may not anticipate or respond to effectively.
Graph 10. Complexity of Human Behavior vs. AI Performance in Gambling.
Graph 11. Generalizability of AI Models in Gambling
-
What future developments will AI need to improve individual gaming experiences while maintaining responsible and fair gaming standards?
Several areas of development are needed to improve personal gaming experiences and maintain responsible and fair gaming standards:
Advanced behavioral models: Develop AI models that better understand and simulate human behavior, including emotional and irrational decision-making processes.
AI Ethical Guidelines: Establish clear ethical standards for the use of AI in gaming to ensure fairness, transparency and responsible gaming.
Real-Time Monitoring: Implement real-time monitoring systems to identify and respond to problem gaming. This ensures that AI encourages responsible gaming.
Player Consent and Privacy: Ensure players are fully aware of how their data is being used and obtain their consent. Develop strong data protection measures to protect player information.
Adaptive learning: Build AI systems that continuously learn and adapt based on new information and interactions, improving their ability to deliver personalized and fair experiences.
These insights aim to balance technological development with ethical practices and ensure the beneficial and fair integration of artificial intelligence into the gaming industry.
Graph 12. Player Satisfaction with AI-Enhanced Personalized Gaming
Graph 13. AI Development for Responsible and Fair Gaming
-
-
PYTHON INTEGRATED SOLUTIONS TO THE PROBLEM STATEMENT
-
Data Collection :
Assuming that historical roulette data is available in a structured format such as a CSV file. Here's how you can start by loading the data :
-
Data Preparation and Feature Engineering :
Preprocess the data to handle missing values, outliers, and create relevant features :
-
Building the AI Model :
Using a simple machine learning model such as logistic regression to start with :
-
Ethical Implications & Future Developments : Discussing these aspects requires more qualitative analysis and research, but we can programmatically highlight some aspects :
Ethical implications:
Ensuring transparency and fairness of projections. Enabling responsible gaming features.
Further development:
Implement more complex AI models (e.g. deep learning):.
This integrated Python solution above is the starting
point for solving critical questions in the research paper. This approach involves data collection,
preparation, feature design, and creation of artificial intelligence models to predict roulette results.
-
AI systems can mimic human decision-making to some extent, but it is difficult to fully model the complexity of human cognition and behavior.
-
Ethical implications include potential bias, fairness and the need for responsible use of AI in gambling.
-
The complexity of human behavior requires continuous improvement and robustness of AI models.
-
Future AI development must focus on improving personal gaming experiences while adhering to ethical standards.
-
-
VII. CONCLUSION
In conclusion, this research paper has shown significant potential for integrating AI into roulette games using Python for data-driven decision making and predictive modeling. Through careful data collection, preparation and feature design, AI models are able to mimic human decision-making processes to some extent, optimizing betting strategies and predicting game results with new precision. However, the complexity of accurately modeling human behavior and decision-making brings challenges, such as possible biases in AI models and the need for continuous improvement to maintain fairness and transparency. The ethical implications of using AI in game environments, including ensuring responsible gaming, are critical areas that require continued attention. As the industry moves forward, future developments must focus on improving personalized gaming experiences in line with ethical standards by adopting more sophisticated models such as deep learning frameworks and continuous ethical assessments. This article highlights the transformative impact of AI on traditional gaming dynamics and lays the groundwork for future research and improvement of the role of AI in the gambling industry.
REFERENCE
[1]. Al-Faraj, T. N., Al-Zayer, J. A., and Alidi, A. S. (1991), A PC-Based Spreadsheet Support System for the Newsboy Inventory Control Problem, International Journal of Operations and Production Management, 11(10): 5864. [2]. Ammar, S., and Wright, R. (1999), Experiential learning activities in Operations Management, International Transactions in Operational Research, 6(2): 183197. [3]. Anderson, D. R., Sweeney, D. J., and Williams T. A. (2001), Contemporary Business Statistics with Microsoft Excel, South Western Thomson Learning, Stamford, Connecticut, U.S.A. [4]. Bodily, S. (1986), Spreadsheet modeling as a stepping stone, Interfaces, 16(5): 3452. [5]. Chang, J., Lee, M., Ng, K., and Moon, K., (2003), Business simulation games: The Hong Kong experience, Simulation and Gaming: An International Journal, 34(3): 367379. [6]. Craft, R. K. (2003), Using spreadsheets to conduct Monte Carlo experiments for teaching introductory econometrics, Southern Economic Journal, 69(3): 726736. [7]. Dempsey, J. V, Haynes, L. L., Lucassen, B.A., and Casey, M. S., (2002), Forty simple computer games and what they could mean to educators, Simulation and Gaming: An International Journal, 33(2): 157168. [8]. Doane D. P. (2004), Using Simulation to Teach Distributions, Journal of Statistics Education, 12(1) www.amstat.org/publications/jse/v12n1/doane.html. [9]. Eppen, G. D., Gould, F. J., Schmidt, C. P., Moore, J. H. and Weatherford, L. R. (1998), Introductory Management Science: Decision Modeling with Spreadsheets, (5th ed.), Upper Saddle River, NJ: Prentice Hall. [10]. Grossman, T. A. Jr. (1999), Spreadsheet modeling and simulation improves understanding of queues, Interfaces, 29(3): 88103. [11]. James R. Evans and David L. Olson (2003), Statistics, Data Analysis and Decision Modeling, (2nd ed.), Upper Saddle River, NJ: Prentice Hall. [12]. Kohler, H. (2002), Statistics for Business and Economics: Microsoft°r Excel Enhanced, South Western Thomson Learning, Stamford, Connecticut, U.S.A. [13]. Leemkuil, H., de Jong, T., de Hoog, R., and Christoph, N. (2003), KM QUEST: A collaborative Internet-based simulation game, Simulation and Gaming: An International Journal, 34(1): 89112. [14]. Leon, L., Przasnyski, Z., and Seal, K. C. (1996) Spreadsheets and OR/MS models: An end-user perspective, Interfaces, 26(2): 92104. [15]. Mills J. D. (2002) Using Computer Simulation Methods to Teach Statistics: A Review of the Literature, Journal of Statistics Education, 10 (1), http://www.amstat.org/publications/jse/v10n1/mills.html [16]. OConnor, J. and Dickerson, M. (2003), Definition and Measurement of Chasing in Off-Course Betting and Gaming Machine Play, Journal of Gambling Studies, 2003, 19(4): 359368. [17]. Ragsdale, C. T. (2004), Spreadsheet Modeling and Decision Analysis: A Practical Introduction to Management Science, (4th ed.), South Western Thomson Learning, Stamford, Connecticut, USA. [18]. Ruohomaki, V. (2003), Simulation gaming for organizational development, Simulation and Gaming: An International Journal, 34(4): 531543. [19]. Seal, K. C. (1995), Spreadsheet simulation of a queue with arrivals from a finite population: The machine repair problem, International Journal of Operations and Production Management, 15(6): 84100. [20]. Seal, K. C., and Przasnyski, Z. H. (2002), Virtual Roulette Spreadsheet: A Teaching Tool, Decision Line, 33(4): 58. [21]. Thiriez, H. (2001), Improved OR education through the use of spreadsheet models, European Journal of Operational Research, 135(3): 461475. [22]. Yeates S. A. (2003), Use of Computer Simulation in Business Games: A review of the Literature, (October 27, 2003), Retrieved May 18, 2004 from http://cisnet.baruch.cuny.edu/phd/altschuller/cis840/siminBus Gamesoutline.doc [23]. Illustrating Probability through Roulette: A Spreadsheet Simulation Model. [24]. D. Abuhanna, Roulette: More than just a Chance.