
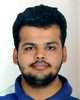
- Open Access
- Authors : Parth Kulkarni
- Paper ID : IJERTV9IS090106
- Volume & Issue : Volume 09, Issue 09 (September 2020)
- Published (First Online): 11-09-2020
- ISSN (Online) : 2278-0181
- Publisher Name : IJERT
- License:
This work is licensed under a Creative Commons Attribution 4.0 International License
Analysis of Throughput Parameters in A Flow Line Manufacturing System with Varying the Buffer Limits
Parth Kulkarni
School of Mechanical Engineering
Dr. Vishwanath Karad MIT World Peace University Pune, India
Abstract: For a Manufacturing System consisting of a Flow Line setup, the final throughput of the system depends on the efficiency of all the sub-components and processing stations efficiency. Considering the Flow Line Setup, it evident that the products manufactured using flow lines have low or in some cases zero variants. This makes it crucial for the system to produce high quantity of products, but for machines failure is inevitable. Moreover, the failure of one processing unit results in halting of the whole manufacturing system, which in turn lowers the overall throughput of the Manufacturing System. In order to avoid that from happening, many studies have been conducted that suggest incorporating a buffer zone or station in between the processing units. In this paper, I have explored this idea using Siemens Tecnomatix simulation software & analysed the throughput varying the buffer limits in a Flow line manufacturing systems setup in a simulated environment.
Keywords: Throughput, Siemens Tecnomatix, Buffer, Waiting time & Working time.
INTRODUCTION:
The Flow Line setup is a type of a Manufacturing system that incorporates a flow pattern in which processing units are grouped together in the series of operations required for manufacturing the product. The key skills required for a single operation are transferable from the machine to the worker, thus requiring a low skill workforce. But, the units manufactured by this process dont usually have many variants & moreover the production rate is high for this process when compared to Job shops. When machines are used in any process, there is always a probability of them failing and Flow Lines also experience the same effect. It has been observed that incorporating buffers between the processing units helps in increasing the overall throughput of the system taking into account the failure conditions of the system. A simulation of the Flow Line setup was created using Siemens Tecnomatix Plant Simulation Software, for a Manufacturing System consisting of 3 stations for processing between a Source and a Drain was used (Figure 1). The source was used to provide the raw material & the drain used to collect the end product. The Throughput per hour of the drain is of interest as it gives the overall throughput of the system and varies for different values of buffer numbers. Here, the buffer number is the maximum amount of buffer needed in between consecutive stations and the processed units from one station would be only passed on to another station after the buffer units in the buffer zone reaches a particular desired value (Figure 2).
Figure 1: Simulation of Flow Line Setup with 3 stations between a source and drain, without any buffers.
Figure 2: Simulation of Flow Line Setup with 3 stations and Buffer zones between consecutive stations with Source and Drain.
Necessity of Buffers and failures of Machines: The failures in machines are inevitable and when failures occur in the case of Flow Lines, it stops the whole system and the whole throughput is affected by it. If failure occurs in the processing stations which are at the start of the system, then the stations down the line remain idle and dont get operations done until the broken unit is repaired. And, if the failure occurs in the stations at the end of the line, the unfinished parts pile up until the station is repaired. It has been found that incorporating buffer zones has resulted in an overall increase in the throughput of the systems. As, it is seen that the buffer zones reduce the waiting time in case of a machine failure and provides a uniform flow to the setup.
Scope and characteristics of the Simulated Environment: For the need to understand the variations in the time parameters like throughput per hour, waiting and working time of the stations it was necessary to keep the processing time for each station to be the same, it was chosen that each station would have a processing time (Cycle Time) of 10 seconds (Figure 3).
Figure 3: The processing time for each station was set to 10 seconds.
And the availability of each station was taken to be 95% with a Mean Time to Repair (MTTR) of 1 minute (Figure 4).
Figure 4: The Availability for each station was setup to 95% with MTTR of 1 minute.
The unit component on which processing was conducted was chosen to be a default simulated part of dimensions: Length-0.5m; Width-0.4m & Height 0.1m (Figure 5)
Figure 5: Default unit component chosen for analysis
Initially when the simulation was tested with all the scope parameters input, a no buffer zone setup was used to check the initial conditions of the manufacturing system (Figure 1).
It was found that stations 2 and 3 experienced Waiting time while working
Figure 6: Graphical Representation of the resource statistics for each station plotted against percentage of time used.
It was observed that Station-2 & Station-3 both have working time in percentage to be 85.5% and, they have waiting time in percentage to be 4.56% and 9.05% respectively (Figure 7 &8).
Figure 7: Resource Statistics quantitative value for Station 2 when no buffer.
Figure 8: Resource Statistics quantitative value for Station 3 when no buffer.
Effects of Introduction of Buffer Zones: By incorporating Buffer Zones/Stations in between every consecutive it was observed that there was a general trend of increase in the overall throughput rate and a decrease in the waiting time for the stations with increase in the buffer number.
When a buffer of maximum capacity 2 parts per buffer station was implemented, there was a good increase in the throughput per hour, working time of Stations 2 & 3 for the manufacturing system:
Figure 9: Buffer Capacity of 2 was implemented in every buffer station.
Figure 10: Graphical Representation of the resource statistics for each station plotted against percentage of time used, for buffer capacity of 2.
When compared to Figure 6, it is quite evident that there has been an increase in working time percentage of station 2 and 3, and also a decrease in waiting time percentage in station 2 and 3 when compared to Figure 10.
Moreover, the working time percentages for station 2 & 3 were found to be 87.71% & 87.7% respectively (Figure 11). Furthermore, the waiting time percentages for station 2 & 3 were found to be 3.46% & 7.18% respectively (Figure 12).
Figure 11: Resource Statistics quantitative value for Station 2 when buffer capacity of 2 is implemented.
Figure 12: Resource Statistics quantitative value for Station 3 when buffer capacity of 2 is implemented.
Also, the overall Throughput per hour of the System increased to 315.73. (Figure 13).
Figure 13: The Statistics of Throughput per hour for the manufacturing system when a buffer capacity of 2 is implemented.
Similar observations were conducted for a range of buffer capacity from 2 to 20.
The figures below show the graphical representations of all the variations observed at different buffer capacities.
Figure 14: Graphical Representation of the resource statistics for each station plotted against percentage of time used, for buffer capacity of 4.
Figure 15: Graphical Representation of the resource statistics for each station plotted against percentage of time used, for buffer capacity of 6.
Figure 16:Graphical Representation of the resource statistics for each station plotted against percentage of time used, for buffer capacity of 8.
Figure 17: Graphical Representation of the resource statistics for each station plotted against percentage of time used, for buffer capacity of 10.
Figure 18: Graphical Representation of the resource statistics for each station plotted against percentage of time used, for buffer capacity of 12.
Figure 19: Graphical Representation of the resource statistics for each station plotted against percentage of time used, for buffer capacity of 14.
Figure 20: Graphical Representation of the resource statistics for each station plotted against percentage of time used, for buffer capacity of 16.
Figure 21: Graphical Representation of the resource statistics for each station plotted against percentage of time used, for buffer capacity of 18.
Figure 22: Graphical Representation of the resource statistics for each station plotted against percentage of time used, for buffer capacity of 20.
As it is seen from the graphical representations that working time percentage for stations 2 & 3 has increased by an extent (Figure 10 & 14 to 22), while the waiting time was found to have decreased for both the stations. The quantitative values for the waiting time percentage, working time percentages and throughput per hour was tabulated:
Moreover, the comparisons of Buffer Capacity with waiting time percentage, working time percentage and throughput time per hour from the table was graphically plotted with Buffer Capacity:
THROUGHPUT PER HOUR v/s Buffer Capacity
335
y = 0.004×3 – 0.1974×2 + 3.5401x + 308.83
330 R² = 0.9974
325
THROUGHPUT PER HOUR
320
Poly. (THROUGHPUT PER
315 HOUR)
310
305
0 5 10 15 20 25
THROUGHPUT PER HOUR v/s Buffer Capacity
335
y = 0.004×3 – 0.1974×2 + 3.5401x + 308.83
330 R² = 0.9974
325
THROUGHPUT PER HOUR
320
Poly. (THROUGHPUT PER
315 HOUR)
310
305
0 5 10 15 20 25
10
9
8
7
6
5
4
3
2
1
0
y = 4E-06×6 – 0.0002×5 + 0.004×4 – 0.0374×3 + 0.1995×2 –
1.2097x + 9.0503
R² = 0.9988
WAITING S3
Poly. (WAITING S3)
0
5
10
15
20
25
5
4.5
4
3.5
3
2.5
2
1.5
1
0.5
0
y = 1E-06×6 – 1E-04×5 + 0.0024×4 – 0.0305×3 + 0.2038×2 – 0.9028x + 4.5779
R² = 0.994
WAITING S2
Poly. (WAITING S2)
0
5
10
15
20
25
25
20
15
10
5
WAITING S2
Poly. (WAITING S2)
0
y = 1E-06×6 – 1E-04×5 + 0.0024×4 – 0.0305×3 + 0.2038×2 – 0.9028x + 4.5779
R² = 0.994
5
4.5
4
3.5
3
2.5
2
1.5
1
0.5
0
25
20
15
10
5
WAITING S3
Poly. (WAITING S3)
0
y = 4E-06×6 – 0.0002×5 + 0.004×4 – 0.0374×3 + 0.1995×2 –
1.2097x + 9.0503
R² = 0.9988
10
9
8
7
6
5
4
3
2
1
0
WAITING TIME FOR S3 V/S Buffer Capacity
WAITING TIME FOR S3 V/S Buffer Capacity
WAITING TIME FOR S2 V/S Buffer Capacity
WAITING TIME FOR S2 V/S Buffer Capacity
WORKING TIME FOR S2 V/S Buffer Capacity
WORKING TIME FOR S2 V/S Buffer Capacity
93
92
91
90
y = -2E-06×6 + 0.0001×5 – 0.0026×4 + 0.0333×3 – 0.2478×2 + 1.4706x + 85.506
R² = 0.9985
93
92
91
90
y = -2E-06×6 + 0.0001×5 – 0.0026×4 + 0.0333×3 – 0.2478×2 + 1.4706x + 85.506
R² = 0.9985
0
5
10
15
20
25
0
5
10
15
20
25
WORKING TIME FOR S3 V/S Buffer Capacity
WORKING TIME FOR S3 V/S Buffer Capacity
85
85
0
5
10
15
20
25
0
5
10
15
20
25
89
89
WORKING S2
Poly. (WORKING S2)
WORKING S2
Poly. (WORKING S2)
88
87
86
85
88
87
86
85
93
92
91
90
89
93
92
91
90
89
WORKING S3
Poly. (WORKING S3)
WORKING S3
Poly. (WORKING S3)
88
87
86
88
87
86
y = -2E-06×6 + 0.0001×5 – 0.0026×4 + 0.0324×3 – 0.2428×2 + 1.4603x + 85.505
R² = 0.9985
y = -2E-06×6 + 0.0001×5 – 0.0026×4 + 0.0324×3 – 0.2428×2 + 1.4603x + 85.505
R² = 0.9985
INFERENCE:
From the graphical representations, it can be inferred that the values of Throughput per hour & Working times increases by a polynomial equation while the working times decreases in the same manner following a polynomial pathway.
Here the R-squared (R2) is a statistical measure that represents the proportion of the variance for a dependent variable that's explained by an independent variable or variables in a regression model. Whereas correlation explains the strength of the relationship between an independent and dependent variable, R-squared explains to what extent the variance of one variable explains the variance of the second variable. Usually the R2 quantitatively gives the approximation of the amount of values that can be explained by the Graphical models input. For the observations made, the value is in the range of 0.99 or greater. That is, more than 99% of the observations are synchronise to the equations obtained.
Conclusion: There is a threshold up to which the amount of working times and throughput rate can be increased by increasing the buffer capacity and the same characteristic can be observed with the decrease in waiting time. When the threshold is reached, the Manufacturing System consisting of a Flow Line setup can be noted as fully optimized. The optimization which
leads to maximum throughput and reduces the waiting time for the stations is responsible for the maximum production potential for manufacturing systems.
REFERENCES:
-
Lutz, Christian & Davis, K. & Sun, Minghe. (1998). Determining buffer location and size in production lines using tabu search. European Journal of Operational Research. 106. 301-316. 10.1016/S0377-2217(97)00276-2.
-
Khalid A. Aldakhilallah. The Determination of the Average WIP Inventory and Buffer Size for a Two-stage Manufacturing System. J. King Saud Univ., Vol. 14, Admin. Sci. (1), pp. 33-47, Riyadh (1422/2002).
-
Conway, R., Maxwell, W., McClain, J. O., & Thomas, L. J. (1988). The Role of Work-in-Process Inventory in Serial Production Lines. Operations Research, 36(2), 229241. doi:10.1287/opre.36.2.229.
-
So, K.C. Allocating buffer storages in a flexible manufacturing system. Int J Flex Manuf Syst 1, 223237 (1989). https://doi.org/10.1007/BF00235266.