
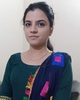
- Open Access
- Authors : Suman , Vinod Kumar
- Paper ID : IJERTV10IS090067
- Volume & Issue : Volume 10, Issue 09 (September 2021)
- Published (First Online): 14-09-2021
- ISSN (Online) : 2278-0181
- Publisher Name : IJERT
- License:
This work is licensed under a Creative Commons Attribution 4.0 International License
Analytical Investigation of a Deterministic Inventory Model using the Cubic Demand Rate and the Exponential Distribution as Deterioration Rate
,
,
Suman1 Vinod Kumar2
1Research Scholar, Department of Mathematics, School of Applied Sciences, Om Sterling Global University, Hisar-125001,
2Professor& Head, Department of Mathematics, School of Applied Sciences, Om Sterling Global University, Hisar-125001,
Abstract:- This research looks into an inventory model for decomposing items or products with a cubic demand over time, with an exponential deterioration rate. In the model, shortages are allowed. It also shows that the cubic demand function is convex and yields the best result. A three-dimensional graphical representation of this model's convexity is shown. An illustration is created to double- check the model. The ideal solution was put through a sensitivity analysis with respect to the main parameters, and the results were presented.
Keywords: Deterioration, cubic demand, Shortages, Total inventory cost.
INTRODUCTION:
Inventory is defined as the stock of items kept on hand to ensure a trade or business's efficient and smooth operation. It also contributes to the company's growth. Manufacturers, schools, farms, hospitals, and higher education institutions all rely on it. Both merchants and wholesalers must keep their inventory of things or products at a minimum. It is made up of several diverging conditions that can be turned into models. These circumstances could include deterministic demand, demand changes over time, degradation, and so forth.
The mathematical model is distributed into two kinds, i.e., Deterministic and Stochastic models. Deterministic and stochastic mathematical models are the two types of models available. The demand rate is constant in the Deterministic model. In inventory, demand is quite crucial. The demand rate was expected to remain constant in the basic inventory model. However, this isn't always possible.
For many inventory products, like fashionable items, dairy goods, electric items, fruits and vegetables, and so on, the hypothesis of not changing demand rate is not appropriate; the demand rate may be dependent on time, stock, and on price. Fruits, vegetables, medications, dairy products, and other items have a finite shelf life. They deteriorate over time. Items or things that deteriorate are referred to as degrading items. The inventory system is plagued by issues caused by the deterioration of items or products.
The change in demand rate could also be linear, i.e., demand might increase or decrease linearly with time. We employ a linear polynomial as a demand function for linear demand. It should occasionally be a significant change in demand, i.e., demand is rapidly increasing with relevance time. A biquadratic polynomial demand function can be utilized to achieve this increment. Biquadratic demand can help you reduce inventory costs and grow your business.
In 1963, Ghare and Schrader noted that some products deteriorate by a negative exponential function concerning time. This observation gives the differential equation of inventory products with deterioration. An inventory mathematical model for degrading products with exponentially deteriorating demand was proposed by Liang- Yuh Ouyang, Kun-Shan WU, and Mei- Chuan CHENG (2005). Alfares (2007) looked at the inventory policy for an item with a demand rate dependent on the stock level and a storage time dependent on the holding cost. In 2008, Ajanta Roy created an mathematical model for decaying items in which demand was depends on price. He'd also added a time-based holding cost. The case in which degrading rate follows Weibull distributions, C. K. Tripathy* and U. Mishra (2010) devised a listing model. A list model for decaying products was presented by R. Amutha and Dr. E. Chandrasekaran (2012). Demand was supposed to be a straight line in this model. The cost of holding is calculated as a linear function of time. Dr. Ravish Kumar Yadav and Ms. PratibhaYadav(2013) demonstrate the production model with assumption of cubic demand rate. R. Venkateswarlu and R. Mohan (2014) created a list model for deteriorating products constructed on the hypothesis that demand is a quadratic function of time. This concept was created to lower total inventory costs (TIC). Garima Sharma and Bhawna Vyas (2018) suggested a inventory model for decaying products with a Weibull distribution. Demand is measured to be a one degree function of time, with shortages permitted and partially backlogged. Ganesh Kumar, Sunita, and Ramesh Inaniyan (2020) proposed a listing model with a time-dependent demand rate employing various factors. It is supposed that the demand rate is a three degree polynomial of your time.
The demand rate is treated as a cubic polynomial of time in this paper, and the deterioration rate fluctuates with time. The cost of the order is believed to be constant and does not fluctuate over time. This model's convexity is checked using a three- dimensional graphical representation. To validate the model, an illustration is also created. The ideal solution has been subjected to a sensitivity analysis concerning essential factors, and the results are displayed.
NORMS AND REPRESENTATIONS:
The mathematical model is explained using the norms and representations listed below. Norms
-
a, b, c, and d are constant.
-
The demand rate () at time t is supposed as () = a + bt + ct2 + dt3;a, b, c, d is constant.
-
Replenishment takes place.
-
Shortages are permitted.
-
() = Denotes the deterioration rate.
Representations
CSC
Shortage cost per unit per unit time.
COC
Ordering cost per order.
CDC
Deterioration cost.
CHC
Holding Cost.
The maximum inventory level for each ordering cycle.
Shortage level for each cycle.
The order quantity ( = + ).
()
Inventory level at time t.
1
Time at which shortages start.
The total length of each ordering cycle.
Total inventory cost over the period (0, T).
MATHEMATICAL FORMULATION:
The graph below (Figure 1) shows how inventory changes over time. The ideal order quantity, Q, and the total optimal inventory cost, TC, are shown in this diagram.
Figure 1: Inventory level (Q) vs time
At t=0, the inventory level is extreme. Following that, the inventory level lowers over the time range [0, t] until falling to zero at t=1. Further, at = 1,shortages occur during the time interval [1, ].
Now till the shortages are allowed at interval [0, 1], the differential equation is given by:
1() + () = ( + + 2 + 3); 0
1 1
(1)
And during the interval [1, ], the shortage occurs, so the differential equation is given by:-
2()
+ () = ( + + 2 + 3); t
t T
(2)
2 1
With the boundary conditions: t=0, Q(0)=W T=t1; Q(t1)=0
T=T; Q(T)=S
Now, by solving the above equations (1), we get:
(t) = [( ) + (2 2) + (3 3) + (4 4) + (2 2) + (3 3) + (4
1 1 2 1 3 1 4 1 2 1 3 1 4
2 2 2 2
2 2 2 2
4) + (5 5) (3 3) (4 4) (5 5) (6 6) (( 2) +
5 1 6 1 8 1
10 1
12 1 1
(2 3) +
(3 4) +
(4 5) +
2
(2 3) +
2
(3 4) +
2
(4 5
2
1
3
1
4
1
2
1
3
1
4
1
(2 3) +
(3 4) +
(4 5) +
2
(2 3) +
2
(3 4) +
2
(4 5
2
1
3
1
4
1
2
1
3
1
4
1
) +
2 (5 6) 3 (3 4) 3 (4 5) 3 (5 6) 3 (6 7)) + (2 ( 2 3) +
5 1 6 1 8 1
10 1
12 1 2 1
2
(22
4) +
2
(32
5) +
2
(42
6) +
3
(22
4) +
3
(32 5) +
3
(42
4
1
6
1
8
1
4
1
6
1
8
1
2
(22
4) +
2
(32
5) +
2
(42
6) +
3
(22
4) +
3
(32 5) +
3
(42
4
1
6
1
8
1
4
1
6
1
8
1
3 4 4 4 4
3 4 4 4 4
6) + (52 7) (32 5) (42 6) (52 7) (62 8))]
10 1
12 1
16 1
20 1
24 1
(4)
(4)
By solving equation (2), we get:
Q2(t) = (1 ) + (2 2) + (3 3) + (4 4)
2 1 3 1 4 1
(3)
1
1
Now, at t=0, the maximum inventory level for each cycle is given by: Q(0)=W, t=0
W = Q (0) =
2
+ 1 +
3
1 +
4
1 +
2
1 +
3
1 +
4
1 +
5
1
23
1
24
1
25
1
26
1 1 2 3 4 2 3 4 5
6 8 10 12
And at t=T, the maximum amount of cubic demand per cycle is given by: t=T, Q2(t)= -S
S = ((
) + (2 2) + (3 3) + (4 4))
1 2 1 3 1 4 1
Now, the order quantity per cycle is:
2 3 4 2 3 4 5 23 24 25 26
= + = 1 + 1 + 1 + 1 + 1 + 1 + 1 + 1 1 1 1 1 ((1 ) +
(2 2) +
2 3 4 2
(3 3) + (4 4))
3 4 5 6
8 10 12 2 1
3 1 4 1
Holding cost per unit per unit time is given by:
t1
Holding Cost per cycle = CHC Q1(t)dt
0
(5)
1
1
HC
HC
1
1
Holding Cost per cycle = C [ 2 + (
2 3
+ 6
) 3 + (
1
1
4
+ 8
52
24
) 4 + (
1
1
5
+
10
52 + 30
53
60
) 5 + (
12
52 36
+ 53 72
4) 6 + ( 52 + 53 4) 7 + (53 4) 8 4 9]
72 1
42 84
84 1
96 96 1
96 1
Shortages cost per unit per unit time is given by:
T
Shortage Cost per cycle = () CSC Q2(t)
t1
(6)
2
2
2
3
3
3
4
4
Shortage Cost per cycle = CSC [ (1
1 ) + ( 1
1 ) + ( 1
1 ) +
4
5
2 2
5
2 6 3
3 12 4
( 1
4
1 )]
20 5
Ordering cost per order is given by:
Now, the deteriorating cost is given by:
Ordering cost per order = COC
t1
(7)
(8)
Cost due to Deterioration = CDC [W Q(t)dt ]
0
Cost due to Deterioration =
2
[ 1 +2
3
1 +
3
4
1 +
4
5
1
5
23
1
6
24
1
8
25
1
10
26
1 ]
12
Therefore, the total cost per unit time per unit cycle is given by:
(9)
TC =
1
(Holding Cost per cycle + Shortage Cost per cycle + Ordering cost per cycle + Cost due to Deterioration)
T
1 2
52
52
53
52
53
4
TC =
{ [
1 + ( +
2 3
) 3 + ( +
1
1
6 4 8
) 4 + (
1
1
24 5
+ +
10 30
) 5 + (
1
1
60 12
+
36 72
) 6 +
1
1
72
52
53
4
53
4
4
2
2
2
3
3
3
4
4
( +
) 7 + (
) 8
9]
[ (11 ) + ( 1
1 ) + ( 1
1 ) +
42 84
84 1
96 96 1
96 1
2 2 2 6 3
3 12 4
4
5
5
2
3
4
5
23
24
25
26
( 1 1 )] + COC + [ 1 + 1 + 1 + 1 1 1 1 1 ]}
4 20 5
2 3 4 5 6
8 10 12
This is the essential condition to minimize the total cost of inventory.
d(TC) 1
2
52
52
53
52
53
4
dT = 2
{ [
1 + ( +
2 3
) 3 + ( +
1
1
6 4 8
) 4 + ( + +
1
1
24 5 10 30
) 5 + ( +
1
1
60 12 36 72
) 6 +
1
1
72
2 3 4
3 4 4
2 2 2 3 3
3 4 4
( 5
+ 5
) 7 + (5
) 8
9] +
[ (1 ) + (1
1 ) + (1
1 ) +
42 84
84 1
96 96 1
96 1
1 2 2
2 6 3
3 12 4
4
5
5
2
3
4
5
23
24
25
26 1
( 1
1 )] + COC + [
1 + 1 + 1 + 1 1 1 1 1 ]}
[CSC (((10)
4 20 5
2 3 4 5 6
8 10 12
) +
) +
2 2 3 3 4 4
2 2 3 3 4 4
( ) + ( ) + ( ))]
2 2 3 3 4 4
2 2 3 2 3 4
2 2 3 2 3 4
d(TC) = 1 {
[1 + 3 ( + ) 2 + 4 ( + 5 ) 3 + 5 ( + 5 + 5 ) 4 + 6 ( 5 + 5 ) 5 +2 3 4 3 4 4
2 3 4 3 4 4
dt1
T 2
3 6 1
4 8 24 1
5 10
30 60 1
12 36
72 72 1
1 1
1 1
7 (5 + 5 ) 6 8 (5 ) 7 9 8]
[( 1) + ( 2) + (2 3) + (3 4)] +42 84
84 1
96 96
22
1 96
23
1
24
25
1 1 1 1
(11)
[1 + 2 + 3 + 4 1 1 1 1 ]}
1 1 1 2
2 2 2
We get the optimal values of 1 and by solving equations (11) & (12) by using MAPLE 15.
NUMERICAL ILLUSTRATION:
(12)
Now check the optimality of the model with the help of numerical illustration and solve the illustration with the help of Maple 15.
To explain the model numerically, assume the following parameters of the inventory system are:
= 15, = 5, = 3, = 1, = 4, = 15, = 100, = 10, = 0.005
Under the above-given parameters, by using Maple 15 get the optimal shortage value t1 =1.965520277 per unit time and the optimal length of the ordering cycle is T=2.169637827 unit time. The total inventory cost is TC=171.7030613
Figure 2: 3-Dimensional Graphical representation of TC of Inventory Model
SENSITIVITY ANALYSIS:
Here we study the effect of changes in the model parameters such that , , , , , , , , .The outcome is given in the below table:
Parameter
% change
Change in
1
a
+20%
1.0160680850
1.0889027267
18.18963695
252.5956185
+10%
1.7077281320
1.159175496
20.45041909
241.0584430
-10%
1.9920037314
1.185750586
27.07175271
218.2594875
-20%
2.044515532
1.816860712
48.10762535
158.6757269
b
+20%
2.126896788
1.915704782
60.49513233
176.6141174
+10%
2.1547467362
1.957865123
60.37356030
172.6973260
-10%
2.191291732
1.990975590
60.25658514
169.1540634
-20%
2.213117867
2.016789921
59.04959300
166.5729344
c
+20%
2.113447263
1.902173202
59.46007555
176.0471621
+10%
2.140927371
1.933085200
59.90343378
173.9257361
-10%
2.199662771
1.999598585
60.82422853
201.3702879
-20%
2.210247264
2.006920108
61.55764241
228.8856832
+20%
2.112338659
1.906072384
58.51067445
175.0532139
+10%
2.199954826
1.926720236
60.76614908
172.7088979
-10%
2.200425661
1.993271308
61.71324677
169.5107908
-20%
2.236482331
2.034550137
62.55851032
167.9186105
+20%
2.011399454
1.738349860
52.72142939
190.6949737
+10%
2.166399602
1.9346398922
58.90606113
172.7767587
-10%
2.260306526
2.091810959
65.10123636
160.0418475
-20%
2.361669905
2.229717027
70.74288999
146.5633663
+20%
2.2915508098
2.1824406245
68.55092132
255.0259308
+10%
2.235118114
2.066042302
63.75989053
230.6794186
-10%
2.092877649
2.03703989
59.82044284
216.7695858
-20%
2.030681482
1.933548455
53.59939368
195.9338203
+20%
2.208712799
1.999529473
62.35879326
180.8380976
+10%
2.189558638
1.982895452
61.37187552
176.2909535
-10%
2.148869472
1.947321081
59.31515212
167.0719555
-20%
2.128345692
1.928054384
58.95488632
159.6773670
+20%
2.117510096
1.912520935
58.25023377
171.9721423
+10%
.9995693954
.2251108959
18.74167160
229.6118473
-10%
.9994046753
.2248812972
18.73771531
229.6078397
-20%
2.171770536
1.968525070
60.46662157
171.4330541
+20%
.9996592125
.2252363333
18.74423028
229.6139733
+10%
.9996493226
.2251252345
18.74482032
229.6147822
-10%
.9994010197
.2248760819
18.73742754
229.6077803
-20%
.9993151458
.2247562686
18.73516556
229.6057199
-
With the increase in a,d TC increase, and Q decrease.
-
With the increase in b, TC and Q increases.
-
With the increase in c, TC, and Q decreases.
-
If CHC (Holding Cost) increases, TC increases, and Q will decrease.
-
If CSC (Shortage Cost), COC (Ordering Cost) increases, Q and TC will increases.
-
If increases, Q, and TC increase.
On the basis of supposition of cubic demand and exponential distribution as deterioration function different cases are arise:
CASE1: Study of Model in case of cubic demand and exponential distribution with variable Holding Cost
In this case we find out the Total Inventory Cost and verify with the help of numerical illustration
1
= ( + ) 1()
0
With the help of equation (3), we get
1
1
= [ 2 + (
2 3
+ 6
) 3 + (
1
1
4
+ 8
52
1
1
24
) 4 + (
1
1
5
+ 10
52 +
30
5360
) 5 + (
12
52 + 36
53
72
4 72
) 6 + (
52 +
42
53
84
1
1
4
) 7 + (
53
4
) 8
4
9] + [ 3 + (
+
2
) 4 + (
+
2
2
) 5 + (
+
1
1
2
2 +
84 1
96 96 1
96 1
6 8 24 8 1
10 30 10
40 1
12 36 12 48
73) 6 + ( 2 2 + 73 4) 7 + ( 2 + 73 4) 8 + (73 4) 9 4 10]
144 1
42 14
56 168
112 1
64 192
128 1
216
144 1
160 1
=
1
( + + + )
1 2
52
52
53
52
53
4
52
=
{ [
1 + ( +
2 3
) 3 + ( +
1
1
6 4 8
) 4 + (
1
1
24 5
+ +
10 30
) 5 + (
1
1
60 12
+
36 72
) 6 + ( +
1
1
72 42
53
4
53
4
4
3
2
2
2
2
2
84 84
) 7 + (
1
1
96
) 8
1
1
96
9] + [
1
1
96
1 + ( +
6 8 24
) 4 + (
1
1
8 10
+
30 10
) 5 + (
1
1
40 12
+ +
2
2
36 12 48
73) 6 + ( 2 2 + 73 4) 7 + ( 2 + 73 4) 8 + (73 4) 9 4 10]
[ (144 1
42 14
56 168
112 1
64 192
128 1
216
144 1
160 1
1 2
2
2
3
3
3
4
4
4
5
5
2
3
4
5
23
24
25
1 ) + ( 1
1 ) + ( 1
1 ) + ( 1
1 )] + [ 1 + 1 + 1 + 1 1 1 1
2
26
2 6 3
3 12 4
4 20 5
2 3 4 5 6
8 10
12
12
1 ] + }
This is the essential condition to minimize the total cost of inventory.
d(TC)
1 2
52
52
53
52
53
4
dT = 2
{ [
1 + ( +
2 3
) 3 + ( +
1
1
6 4 8
) 4 + (
1
1
24 5
+ +
10 30
) 5 + (
1
1
60 12
+
36 72
) 6 +
1
1
72
52
53
4
53
4
4
3
2
2
2
( +
2 2 3 2 2 3 4 2 3 4 3 4
2 2 3 2 2 3 4 2 3 4 3 4
42 84
) 7 + (
1
1
84 96
) 8
1
1
96
9] + [
1
1
96
1 + ( +
6 8 24
) 4 + (
1
1
8 10
+
30 10
) 5 +
1
1
40
( + + 7 ) 6 + ( + 7 ) 7 + ( + 7 ) 8 + (7 ) 9
12 36
12 48
144 1
42 14
56 168
112 1
64 192
128 1
216
144 1
4
2
2
2
3
3
3
4
4
4
5
5
2
3
4
10] [ (1
1 ) + ( 1
1 ) + ( 1
1 ) + ( 1
1 )] + [
1 + 1 + 1 +
160 1
5
23
24
2
25
2 2 6 3
26 1
3 12
4
2
4
2
20
3
5
3
4
2 3 4
4
1 1 1 1 1 ] + }
{CSC [( ) + (
) + (
) + (
)]}
5 6 8
10 12
2 2 3 3 4 4
d(TC) = 1 { 1 + 3 ( + ) 2 + 4 ( + 5 ) 3 + 5 ( + 5 + 5 ) 4 + 6 ( 5 + 5 ) 5 +
2 2 3 2 3 4
2 2 3 2 3 4
dt1
T
52
[2
53
3
4
6 1
53
4 8
4
24 1
4
5 10
30
2
60 1
12
2
36 72
72
2
1
2
7 ( +
) 6 + 8 (
) 7 + 9 (
) 8] + [ 1 + 4 (
+ ) 3 + 5 ( +
) 4 +
42 84
84 1
96 96 1
96 1
2 8 24 8 1
10 30 10
40 1
2 2 3 2 2 3 4 2 3 4 3 4
2 2 3 2 2 3 4 2 3 4 3 4
6 ( + + 7 ) 5 + 7 ( + 7 ) 8 + 8 ( + 7 ) 7 + 9 (7 ) 8 +
12 36
4
12 48
144 1
42 14
56 168
112 1
64 192
128 1
216
144
1
22
10 (
) 9] [( 1) + (1 2) + (2 3) + (3 4)] + [1 + 2 + 3 + 4 1
160 1
1 1 1 1 1
1 1 1 2
23 24 25
1 1 1 ]}
2 2 2
NUMERICAL ILLUSTRATION:
Now check the optimality of the model with the help of numerical illustration and solve the illustration with the help of Maple 15.
To explain the model numerically, assume the following parameters of the inventory system are:
= 15, = 5, = 3, = 1, = 15, = 100, = 10, = 0.005, = 3, = 1
Under the above-given parameters, by using Maple 15 get the optimal shortage value1 = 1.946300858 per unit time and the optimal length of the ordering cycle is = 2.144001938 unit time. The total inventory cost is = 163.8867454
CASE 2: Study of Model in case of cubic demand and exponential distribution with Variable Ordering Cost
COC
=
1
2
52
52
53
52
53
4
=
{ [ 1 + ( +
) 3 + ( +
1
1
6 4 8
) 4 + (
1
1
24 5
+ +
10 30
) 5 + (
1
1
60 12
+
36 72
) 6 +
1
1
72
2
2
3
3
52
53
4
53
4
4
2
2
2
3
3
3
4
4
( +
) 7 + (
) 8 + (
) 9] [ (1
1 ) + ( 1
1 ) + ( 1
1 ) +
42
4
5
84
5
84 1
COC
96 96
2
1
3
96
4
1
5
23
2
24
2
25
2 6 3
26
3 12 4
( 1
1 )] +
+ [
1 + 1 + 1 + 1 1 1 1 1 ]}
4 20 5
2 3 4 5 6
8 10 12
This is the essential condition to minimize the total cost of inventory.
d(TC) 1
2
52
52
53
52
53
4
dT = 2
{ [
1 + ( +
2 3
) 3 + ( +
1
1
6 4 8
) 4 + (
1
1
24 5
+ +
10 30
) 5 + ( +
1
1
60 12 36 72
) 6 +
1
1
72
52
53
4
53
4
4
2
2
2
3
3
3
4
4
( +
) 7 + (
) 8 + (
) 9] + [ (
1 ) + ( 1
1 ) + ( 1
1 ) +
42
4
5
84 84 1
5
96 96
2
1
3
96
4
1
5
23
1 2
24
2
<>25
2 6 3
26 1
3 12 4
( 1
1 )] + COC + [
1 + 1 + 1 + 1 1 1 1 1 ]}
{CSC [( ) +
2 2 3 3 4 4
2 2 3 3 4 4
4 20 5
2 3 4 5 6
8 10 12
( ) + ( ) + ( ) COC ]}
2 2 3 3
4 4 2
2 2 3 2 3 4
2 2 3 2 3 4
d(TC) = 1 {
[1 + 3 ( + ) 2 + 4 ( + 5 ) 3 + 5 ( + 5 + 5 ) 4 + 6 ( 5 + 5 ) 5 +2 3 4 3 4 4
2 3 4 3 4 4
dt1
T 2
3 6 1
4 8 24 1
5 10
30 60 1
12 36
72 72 1
7 ( 5 + 5 ) 6 + 8 (5 ) 7 + 9 ( ) 8]
[( 1) + ( 2) + (2 3) + (342 84
84 1
96 96
1
22
96
23
1
24
25
1 1 1 1 1
4)] + [1 + 2 + 3 + 4 1 1 1 1 ]}
1 1 1
1 2 2 2 2
NUMERICAL ILLUSTRATION:
Now check the optimality of the model with the help of numerical illustration and solve the illustration with the help of Maple 15.
To explain the model numerically, assume the following parameters of the inventory system are:
= 30, = 5, = 3, = 1, = 15, = 100, = 10, = 0.005
Under the above-given parameters, by using Maple 15 get the optimal shortage value t1 = 2.575399869 per unit time and the optimal length of the ordering cycle is = 2.683196227 unit time. The total inventory cost is = 183.6104201
CONCLUSION:
The sensitivity analysis found that in the case of cubic demand (a function of time), the model predicts that the rate of deterioration will alter over time. It can be shown that parameters a and b are directly proportional to TC, whereas the parameters c, is inversely proportional to TC. It also demonstrates that this technique can be used to determine the total inventory cost. This also shows that Total Inventory Cost minimizes in case of variable holding cost. Finally, a graphical depiction is used to verify the model. The obtained findings determine the model's stability. This model can be expanded or replaced with a different demandrate.
REFERENCES:
-
Ajanta Roy, An Inventory model for deteriorating items with price dependent demand and time-varying holding cost, AMO- Advanced modeling and optimization, volume 10, number 1, 2008.
-
Dr. Ravish Kumar Yadav, Ms. Pratibha Yadav, "Volume Flexibility in Production Model with Cubic Demand Rate and Weibull Deterioration with partial backlogging," IOSR Journal of Mathematics (IOSR-JM) e-ISSN: 2278-5728,p-ISSN: 2319-765X, Volume 6,Issue 4 (May. – Jun. 2013), PP 29-34.
-
Ganesh Kumar, Sunita, and Ramesh Inaniyan, "Cubical Polynomial Time Function Demand Rate and Pareto type Perishable rate based Inventory Model with Permissible delay in Payments under Partial Backlogging," Jnanabha, Vol. 50(1) (2020), 115-133.
-
Garima Sharma and Bhawna Vyas, "An Approach To Positive Reflectance On Inventory Cost By Developing EOQ Model With Linear Demand By Weibull Distribution Deteriorating Items And Partial Backlogging," ISSN 2231-5780 Vol.8(5), May (2018),pp. 125-133.
-
Ghosh, S.K. and Chaudhuri, K.S. (2004), An order-level inventory model for a deteriorating item with Weibull distribution deterioration, time- dependent quadratic demand and shortages,' International Journal of Advanced Modeling and Optimization, 6(1), 31-45.
-
G.P. Samanta and Ajanta Roy (2004), A Production Inventory model with deteriorating items and shortages, Yugoslav Journal of Operations research 14, No 2, 219-230.
-
Roy A., "An inventory model for deteriorating items with price dependent demand and time-varying holding cost," Advanced Modeling and Optimization,10, pp.2537, (2008).
-
Vinod Kumar et al., An inventory model for deteriorating items with time-dependent demand and time-varying holding cost under partial backlogging, Jour of Indus Engg Int., 9(4), (2013).
-
Yadav, R.K and Trivedi, R. A multi-echelon inventory models for deteriorating with partial backlogging, International journal of applied science and technology, 24-31, (2013).
-