
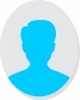
- Open Access
- Authors : Anak Agung Sri Nandini , Rino Andias Anugraha , Teddy Sjafrizal , Murni Dwi Astuti, Mario Adiprana Muki, Mohd. Rasidi Ibrahim
- Paper ID : IJERTV9IS070376
- Volume & Issue : Volume 09, Issue 07 (July 2020)
- Published (First Online): 22-07-2020
- ISSN (Online) : 2278-0181
- Publisher Name : IJERT
- License:
This work is licensed under a Creative Commons Attribution 4.0 International License
Applying Response Surface Methodology to Optimize the Performance of Longitudinal Vibration-Assisted Turning (L-VAT)
Anak Agung Sri Nandini, Rino Andias Anugraha,
Teddy Sjafrizal, Murni Dwi Astuti, Mario Adiprana Muki
Industrial Engineering Department
School of Industrial Engineering, Telkom University Bandung 40257, Indonesia
Mohd. Rasidi Ibrahim
Precision Machining Research Center (PREMACH) Faculty of Mechanical and Manufacturing Engineering, Universiti Tun Hussein Onn Malaysia Batu Pahat 86400, Johor Darul Takzim, Malaysia
AbstractLongitudinal vibration-assisted turning (L-VAT) is an advanced turning process, capable of producing high precision parts with high process efficiency. L-VAT exploits the intermittent contact between the cutting tool and the workpiece, produced by an ultrasonic vibration device attached on the cutting holder. This advanced process results in low cutting force, low cutting temperature and fine surface finish. Optimizing the performance of L-VAT requires a selective decision on the process configurations. This study aims to highlight the optimization process of L-VAT parameters in obtaining minimum surface roughness and cutting temperature. The Response Surface Methodology (RSM) with Box-Behnken Design (BBD) were utilized in selecting the preferred cutting parameters setup (i.e., spindle speed (s), feed rate (f), depth-of-cut (d) and vibrational frequency (F)). Among the investigated parameters, feed rate contributed the most to both surface roughness and cutting temperature. In the case of turning AA6061, the model suggests that the optimum surface roughness (Ra= 2.05m) and cutting temperature (T= 72.50C) could be obtained when turning with s= 635 rpm, f= 0.17mm/rev, d= 0.1 mm, F= 19.9kHz. Eventually, the proposed model may facilitate further industrial adoption of this advanced turning process to machine high hardness materials.
KeywordsMachining; vibration-assisted turning; cutting tool; optimization; response surface methodology.
-
INTRODUCTION
The decisions on process, material and design are crucial to the performance of a fabricated product. Fabricating a complex product design with high-performance material leans on the capability of the available manufacturing process. For instance, the jet engine components, which are designed with tight dimensional tolerance, are required to be fabricated from high temperature withstanding materials. Often these materials possess high hardness level. Thus, a manufacturing process with capability in machining hard material with a high finishing standard is very much expected.
The major challenges of machining the high hardness material (e.g., titanium alloys, ceramic) are to achieve high precision and fine surface finishing while maintaining the process efficiency. Typically, high hardness material is machined by controlling the parameters to achieve the ductile mode cutting [1]. Failure to achieve such condition results in brittle and sharp machining chips. These conditions may harm the new surface after machining. Moreover, high cutting force and cutting temperature are the other harmful factors, reducing cutting tool and machines lifetime [2]. Hence, slower and
shallow machining is favored. However, this conventional approach harms the efficiency of the process. As an alternative solution to this challenge, a vibrational assisted turning (VAT) is introduced.
Vibration-assisted turning (VAT) applies high-frequency vibration with low amplitude to the cutting tool via the ultrasonic vibration device attached on the cutting holder. This advanced process is capable of yielding high quality and precision components with better process efficiency. Applying ultrasonic vibration to the tooltip increase cutting speed, reduce cutting force, reduce cutting temperature, lengthen tool life and produce finer surface [3]. Empirically, the turning machine with VAT capability experiences up to 50% less cutting force than the conventional process [4]. This achievement is because of the less contact time between the cutting tool and the workpiece surface. Besides, the VAT process is proven to produce finer surface than that of conventional turning (CT)[5]. Fundamentally, the cutting tool of VAT can vibrate in 1- dimensional directions, either along the feeding direction, cutting direction or depth-of-cut direction. These directions could be combined to become the 2-dimensional VAT directions [3, 6]. Among those options, the 1-dimensional vibration is still the frequently used method by its simplicity
and its powerful impact on the turning outcomes [7].
Recent studies of 1-dimensional VAT present interesting findings. Examination on the surface integrity of among the turning assisted with the 1-dimensional vibration reveals the impact of the vibration mode to the surface roughness. Turning with vibration along the depth-of-cut direction has more influence on the surface roughness than the other two vibrational directions [8]. Extending this capability to higher capacity, a textured surface could be produced by optimizing the cutting parameters [9].
The optimization works on VAT process have been conducted by various VAT types, materials and optimization techniques. [10] showed a computer simulation and empirical validation to predict and find the optimum performance of VAT. They examined the effect of cutting speed, feed rate and depth-of-cut of VAT (vibrating along the cutting direction) of stainless steel 304 on surface roughness. Combination of Taguchi and TOPSIS was selected as the optimization technique. A similar study was performed by [11] to highlight the effect of VAT on residual stress of 4340 hardened steel. This study demonstrated the application of response surface methodology in constructing the prediction model. Recently,
[12] extended the optimization works of VAT to Ti-6Al- 4Vowing to its higher hardness. They integrated grey relational analysis with ANOVA in deciding the preferred cutting parameters to minimize surface roughness.The present study focuses on selecting the cutting parameters setup for maximizing the capability of VAT (i.e., optimum surface roughness and cutting temperature). The selected VAT mode is 1-dimensional vibration along the depth- of-cut direction, as it has more influence to the surface roughness. For the purpose of this study, this VAT mode is referred to as longitudinal VAT (L-VAT). Fig. I exhibits the typical setup of the chosen L-VAT system. To obtain optimum L-VAT performance, the response surface methodology was selected. This powerful method is capable of constructing a prediction model as well as suggesting the optimized value from the identified factors and responses [13].
FIGURE I. THE CHARACTERISTICS OF LONGITUDINAL-VAT. THE CUTTING TOOL VIBRATES LONGITUDINALLY TO THE CUTTING HOLDER OR ALONG THE DEPTH-OF-CUT DIRECTION.
-
PROCEDURES
The optimized L-VAT performance was obtained from the optimum point of a numerical model constructed by the response surface methodology (RSM). All the modelling processes including the optimization was processed by Design Expert 11 software. The model was established from analyzing a set of data gathered by a series of experiments. The experiments were designed by considering four factors of the L-VAT parameters with three levels for each factor (Table I). From each experiment, the response in the form of surface roughness (Ra) and cutting temperature (T) were measured and to be analyzed by RSM.
TABLE I. LIST OF FACTORS AND LEVELS.
factors, = 1.6818) to meet the rotatability as that of CCD. As many as 28 experiments were deigned with 4 replications at the center combination (Table II).
The experiments were conducted on aluminium alloy AA6061 workpiece. Every workpiece was prepared by performing the conventional turning process (s: 635 rpm, f: 0.05 mm/rev, and d: 0.1 mm) to form a specimen with a diameter of 110 mm and length of 340 mm. Fig. II presents the L-VAT experimental setup. All the L-VAT works were operated on the WINHO S530x1000 lathe machine. The cutting tool, TiN cemented carbide insert (VBMT 160404, manufactured by Mitsubishi), was mounted on the front end of an L-VAT device. The device vibrated at a frequency of 18 kHz22 kHz with an amplitude of 2 m, actuated the cutting tool to vibrate along the long axis of the holder. During the turning process, E6 FLIR infrared camera was set up to collect the in-situ cutting temperature. The surface roughness of the newly machined surface was measured by Mitutoyo Surftest SJ-410.
TABLE II. THE DATASET SETUP AS DESIGNED BY THE BOX- BEHNKEN DESIGN METHOD.
Exp
Coded levels
Exp
Coded levels
s
f
d
F
s
f
d
F
1
-1
-1
0
0
15
0
-1
0
+1
2
+1
-1
0
0
16
0
+1
0
+1
3
-1
+1
0
0
17
-1
0
0
-1
4
+1
+1
0
0
18
+1
0
0
-1
5
0
0
-1
-1
19
-1
0
0
+1
6
0
0
-1
+1
20
+1
0
0
+1
7
0
0
+1
-1
21
0
-1
-1
0
8
0
0
+1
+1
22
0
+1
-1
0
9
-1
0
-1
0
23
0
-1
+1
0
10
+1
0
-1
0
24
0
+1
+1
0
11
-1
0
+1
0
25
0
0
0
0
12
+1
0
+1
0
26
0
0
0
0
13
0
-1
0
-1
27
0
0
0
0
14
0
+1
0
-1
28
0
0
0
0
Note:
s= spindle speed, f= feed rate, d= depth of cut, F= vibrational frequency Experiment 25,26,27 and 28 are the four replications of the center points.
Parameters/Factors
Level (-1)
Level (0)
Level (+1)
Spindle speed (s), rpm
635
855
1350
Feed rate (f), mm/rev
0.17
0.21
0.24
Depth of Cut (d), mm
0.10
0.15
0.20
Frequecy (F), KHz
18
20
22
Two typical RSM design methods are available in Design Expert 11, namely the Central Composite Design (CCD) and the Box-Behnken Design (BBD) method. The latter was selected to design the current experiments. Box-Behnken design is comparatively more efficient than CCD, in particular for experiments with more than 3 factors [14]. In addition, BBD requires no experiment to be run at the extreme level (at 3
.
FIGURE II. THE L-VAT EXPERIMENT SETUP.
-
RESULTS AND DISCUSSION
Table III compiles the measured roughness and cutting temperature of each designed experiment. The measured
roughness of the samples was in the range of fine roughness with typical Ra value ranged between 1.6 – 4.7 m. The relatively low cutting temperature was observed at all samples with a range of 70-920C. These two observations provide additional data to support the advantage of L-VAT in manufacturing high-quality surface finishing.
Exp
Ra (µm)
T(°C)
Exp
Ra (µm)
T (°C)
1
1.886
78.61
15
1.640
79.26
2
2.266
78.97
16
4.357
82.60
3
3.650
74.73
17
3.328
70.41
4
3.850
85.88
18
3.979
83.58
5
3.450
78.67
19
2.642
77.18
6
3.630
78.01
20
3.296
92.41
7
3.100
85.09
21
1.982
78.45
8
3.579
84.81
22
4.393
76.29
9
3.751
71.54
23
1.795
86.26
10
3.005
85.32
24
4.684
88.09
11
3.402
84.47
25
3.414
77.92
12
3.661
83.47
26
3.394
78.06
13
1.758
82.86
27
3.375
76.50
14
3.948
85.10
28
3.103
74.92
Note: Ra= surface roughness ; T = cutting temperature
Exp
Ra (µm)
T(°C)
Exp
Ra (µm)
T (°C)
1
1.886
78.61
15
1.640
79.26
2
2.266
78.97
16
4.357
82.60
3
3.650
74.73
17
3.328
70.41
4
3.850
85.88
18
3.979
83.58
5
3.450
78.67
19
2.642
77.18
6
3.630
78.01
20
3.296
92.41
7
3.100
85.09
21
1.982
78.45
8
3.579
84.81
22
4.393
76.29
9
3.751
71.54
23
1.795
86.26
10
3.005
85.32
24
4.684
88.09
11
3.402
84.47
25
3.414
77.92
12
3.661
83.47
26
3.394
78.06
13
1.758
82.86
27
3.375
76.50
14
3.948
85.10
28
3.103
74.92
Note: Ra= surface roughness ; T = cutting temperature
TABLE III. THE MEASURED ROUGHNESS AND CUTTING TEMPERATURE FROM EACH EXPERIMENT
Sequential model sum of square
Stage A: Selecting Model Terms
Stage A: Selecting Model Terms
Stage B: ANOVA and Fit Statistic Analysis
Stage B: ANOVA and Fit Statistic Analysis
Stage C:
Optimization
Input Response
Model Summary statistic
Suggested Basic Model
ANOVA & Fit statistics analysis
Model qualified?
Yes
Optimization
Lack of fit test
Reduction or Modification
No
The designed experiments were suitable to replicate a wide range of cutting mode, indicated by the condition of the chips. Similar to the conventional turning process, the VAT chips range from continuous to broken chips. Fig. III exhibits the continuous and broken chips produced at experiment 6 and 8, respectively. These experiments were different only at the depth of cut (d) value. Experiment 6 was set at d= 0.1 mm, while the specimen of experiment 8 was cut deeper with d= 0.2 mm. These conditions suggest that higher d value was associated with the brittle mode cutting.
FIGURE III. CHIP FORMATION OF L-VAT. (A) EXPERIMENT 6, FEED RATE=
-
MM/REV (B) EXPERIMENT 8, FEED RATE= 0.2 MM/REV. CHANGE OF FEED RATE AFFECTS CHIP FORMATIONS.
Fig. IV portrays the procedural flow of RSM modelling and optimization. Each of the stages is described and discussed in the following sections.
Stage A: Selecting Model Terms
All data obtained from the designed experiment were analysed to find the matched model term. Table IV is the fit summary of the analysed data for each basic model terms (i.e. linear, 2FI, quadratic and cubic). The sequential model sum of square, model summary statistic and lack of fit test was utilized to generate p-value and R2 of each model terms, and later to be used as the judging criteria. The fittest model was selected from the model with p-value <0.05 and (adjusted R2 predicted R2)
< 0.2.
FIGURE IV. THE FLOW OF MODELLING AND OPTIMIZATION BY RSM.
The suggested model of surface roughness was identified as a linear model (Table IVA). The model had sequential p-value
: <0.0001, lack of fit p-value was 0.0814 and (adjusted R2 predicted R2) < 0.2. Though it was recommended, this model had a lack of fit p-value > 0.05 indicating that the model did not fit enough to use as a prediction model. Thus, alteration of the model was necessary.
Interestingly, the linear and quadratic model were originally suggested as the fit model for cutting temperature (Table IVB). As two models were recommended, the selected model was the highest polynomial model. Hence, the cutting temperature was modelled with the quadratic term. However, it was noticed that the (adjusted R2 predicted R2) value was less than < 0.2, indicating a large block effect condition. To mitigate such condition, an adjustment to the model was required.
TABLE IV. FIT SUMMARY TABLE OF SURFACE ROUGHNESS AND CUTTING TEMPERATURE
-
Response: Surface roughness
Source
Sequential
p-value
Lack of Fit p-value
Adjuste d R²
Predicted
R²
Decisions
Linear
< 0.0001
0.0814
0.8329
0.7806
Suggested
2FI
0.6269
0.0698
0.8206
0.6775
Quadratic
0.3524
0.0698
0.8292
0.5287
Cubic
0.0145
0.4939
0.9703
0.5631
Aliased
-
Response: Cutting temperature
Source
Sequential
p-value
Lack of Fit p-value
Adjuste d R²
Predicted
R²
Decisions
Linear
0.0012
0.0592
0.4485
0.2834
Suggested
2FI
0.2907
0.0632
0.4943
0.072
Quadratic
0.0593
0.1004
0.6555
0.0384
Suggested
Cubic
0.0501
0.3255
0.8971
-1.099
Aliased
Note :
2FI: Sequential sum of squares for the two-factor interaction Judgment criteria: sequential p-value <0.05, lack of fit p-value >0.1,
and (adjusted R2 predicted R2)< 0.2
Stage B: ANOVA and Fit Statistic Analysis
The Analysis of Variance (ANOVA) was performed to see how much variation in response is explained by the model. The analysis was conducted with a significant level of 95%. This analysis also tested individual term independently. Within this analysis, the p-value of lack-of-fit and pure error were also produced. Lack-of-fit describes how the prediction by the model fitted the response, and the pure error shows the difference among the replicated runs. Thus, the suggested models earlier were iteratively modified and analyzed to improve the fitting level. On every modification iteration, the p-value of the model (< 0.05) and the lack-of-fit (> 0.1) were the judging criteria.
TABLE V. ANOVA RESULT OF THE FINAL MODELS. (A) THE MODIFIED LINEAR MODEL OF SURFACE ROUGHNESS, (B) THE REDUCED QUADRATIC MODEL OF CUTTING TEMPERATURE.
-
Surface roughness
Source
SS
df
MS
F-value
p-value
Model
17.160
9
1.910
21.460
< 0.0001
S
s
0.172
1
0.172
1.930
0.1816
f
7.660
1
7.660
86.260
< 0.0001
F
0.015
1
0.01
0.165
0.6892
d
0.007
1
0.007
0.000
0.9929
sf
0.010
1
0.010
0.107
0.7479
s²
0.006
1
0.006
0.064
0.8028
f²
0.199
1
0.199
2.240
0.1518
d²
0.254
1
0.254
2.850
0.1084
s²f
0.413
1
0.413
4.650
0.0449
Residual
1.600
18
0.089
Lack of Fit
1.530
15
0.102
4.760
0.1123
NS
Pure Error
0.065
3
0.022
Cor Total
18.760
27
-
Cutting Temperature
-
-
Source
SS
df
MS
F-value
p-value
Model
545.930
8
68.240
7.720
0.0001
S
s
236.070
1
236.070
26.690
< 0.0001
f
5.700
1
5.700
0.645
0.4319
F
6.130
1
6.130
0.694
0.4153
d
75.020
1
75.020
8.480
0.0089
sd
59.350
1
59.350
6.710
0.018
f²
43.290
1
43.290
4.890
0.0394
F²
52.960
1
52.960
5.990
0.0243
d²
55.310
1
55.310
6.250
0.0217
Residual
168.050
19
8.840
Lack of Fit
161.610
16
10.100
4.700
0.1138
NS
Pure Error
6.440
3
2.150
Cor Total
713.980
27
s= spindle speed, f= feed rate, d= depth of cut, F= vibrational frequency SS= sum of square, MS= mean square; S= significant, NS= not significant
Table V describes the final ANOVA iteration results of each response. The ANOVA results of both responses meet the requirement of the acceptable fitting level. The p-value of the modified linear model suggested for surface roughness was
<0.0001, and the lack-of-fit was 0.1123. Likewise, the reduced model suggested (p-value of model= 0.0001, p-value of lack- of-fit= 0.1138) for the cutting temperature complies with the requirements. Hence, both improved models of the responses were adequate to use as the prediction and optimization model.
The residual data of the responses was also exploited to diagnose the fitting level of the selected models. Fig. V and VI
exhibits the normal plot of residual and the plot of residuals vs. predicted response for both responses. As shown in Fig. V.A1 and B1, the residual data of both responses followed the linear trend, inferring normal distribution. While in Fig. VI.A2 and B2, the plot of residuals vs. predicted response for both responses displayed no obvious patterns. These two conditions support the adequacy of the selected models.
Finally, the prediction model of the responses as a function of the factors are as follow:
Surface roughness (Ra):
= 11.66 0.04 3.67 0.02 25.88 + 0.21 + 0.000022 147.892 + 79.642
0.00012 (1)
Cutting temperature (T):
= 422.66 + 0.04 875.63 28.47 95.17 0.21 + 2183.722 0.722 +
11.78.502 (2)
where, s is the spindle speed (rpm), f is the feed rate (mm/rev), d is the depth of cut (mm) and F is the vibrational frequency of the L-VAT device.
Refer to eq. (1) and (2), the factor of feed rate was identified to have a major influence on the surface roughness and the cutting temperature of L-VAT. Fast feed rate results in a finer surface, while slower feed rate dissipates less heat. The prediction model for surface roughness is in a good agreement with the fundamental surface roughness equation where roughness is proportional to the f [15]. The similar condition where slower feed rate generates finer surface finishing was also reported by [16, 17].
Stage C: Optimization process
The optimization process was conducted by finding a point that maximizes the desirability function (Eq. 3). This function considers several responses and factors to be combined into one desirability function. However, importance or weighted factor could be suited to the ultimate goal of the optimization process. The quantification of the ultimate point is translated into a desirability value (maximum is 1). One to take note that the aim of this optimization value is not to find the bigger desirability value. The value is only a translation of the optimum point.
The present study set the constraints of the optimization process to be similar to the experiment setup. The importance level of all factors and responses were set to be equal to 3. The goals of the factors were defined to be within the setup ranges, while the goals for the responses were to find the minimum value.
Eventually, the optimized performance with the associated cutting parameters was suggested with a desirability value of 0.884 (Fig.VII). The desirability function found the optimum condition of turning AA6160 (Ra= 2.05m, T= 72.50C), when the L-VAT is set at s= 635rpm, f= 0.17mm/rev, d= 0.1mm, and F= 19.9kHz.
FIGURE V. NORMAL PROBABILITY PLOT OF RESIDUALS AND PLOT OF RESIDUALS VS. PREDICTED RESPONSE FOR (A) SURFACE ROUGHNESS, AND (B) CUTTING TEMPERATURE. BOTH RESPONSE MODELS EXHIBIT AN ACCEPTABLE FITTING LEVEL AS INDICATED BY LINEAR RESIDUAL PLOT (NORMALLY DISTRIBUTED)
FIGURE VI. 3D SURFACE GRAPH OF (A) SURFACE ROUGHNESS AND (B) CUTTING TEMPERATURE. PERFORMING L-VAT AT LOWER FEED RATE RESULTS IN A FINER SURFACE, WHILE SETTING UP AT LOWER SPINDLE SPEED AND SHALLOW DEPTH OF CUT DISSIPATES LOWER CUTTING TEMPERATURE.
FIGURE VII. THE RAMP PLOT OF THE OPTIMIZED SURFACE ROUGHNESS AND CUTTING TEMPERATURE WITH THE RESPECTIVE CUTTING PARAMETERS.
-
-
CONCLUSIONS
The application of RSM to optimize the performance of L- VAT has been conducted. Modelling and optimizing L-VAT performance by using RSM along with the Box-Behnken design was relatively fast and efficient. The surface roughness could be modelled by a modified linear model, while the suggested model for cutting temperature is the reduced quadratic model. From both models, feed rate reveals its influence on the surface roughness and cutting temperature. Optimization by desirbility function allows the user to have multiple optimized solutions, by adjusting the weighted factor of the responses. Thus, when turning AA6160, one of the optimized performance (Ra= 2.05m, T= 72.50C) could be achieved at spindle speed= 635rpm, feed rate= 0.17mm/rev, depth-of-cut= 0.1mm, Frequency= 19.9kHz. Finally, L-VAT is very promising to be adopted by industry, especially for turning hard material. The outcomes of this study may be used as the reference point for further industrial investigations of L- VAT.
ACKNOWLEDGMENT
This work was supported by Penelitian Dasar Terapan 2020, funded by Telkom University, Indonesia. Authors also express their best gratitude to the technical support and assistance provided by Rio Aurachman.
REFERENCES
-
E. K. Antwi, K. Liu, and H. Wang, "A review on ductile mode cutting of brittle materials," Frontiers of Mechanical Engineering, vol. 13, no. 2, pp. 251-263, 2018/06/01 2018, doi: 10.1007/s11465-018-0504-z.
-
M. A. Xavior and M. Adithan, "Determining the influence of cutting fluids on tool wear and surface roughness during turning of AISI 304 austenitic stainless steel," Journal of Materials Processing Technology, vol. 209, no. 2, pp. 900-909, 2009/01/19/ 2009, doi: https://doi.org/10.1016/j.jmatprotec.2008.02.068.
-
D. E. Brehl and T. A. Dow, "Review of vibration-assisted machining," Precision Engineering, vol. 32, no. 3, pp. 153-172, 2008/07/01/ 2008, doi: https://doi.org/10.1016/j.precisioneng.2007.08.003.
-
S. Patil, S. Joshi, A. Tewari, and S. S. Joshi, "Modelling and simulation of effect of ultrasonic vibrations on machining of Ti6Al4V," Ultrasonics, vol. 54, no. 2, pp. 694-705, 2014/02/01/ 2014, doi: https://doi.org/10.1016/j.ultras.2013.09.010.
-
H. Soleimanimehr, M. J. Nategh, and S. Amini, "Prediction of Machining Force and Surface Roughness in Ultrasonic Vibration- Assisted Turning Using Neural Networks," Advanced Materials Research, vol. 83-86, pp. 326-334, 2010, doi: 10.4028/www.scientific.net/AMR.83-86.326.
-
V. I. Babitsky, A. N. Kalashnikov, A. Meadows, and A. A. H. P. Wijesundara, "Ultrasonically assisted turning of aviation materials," Journal of Materials Processing Technology, vol. 132, no. 1, pp. 157- 167, 2003/01/10/ 2003, doi: https://doi.org/10.1016/S0924- 0136(02)00844-0.
-
W. Cong and Z. Pei, "Process of Ultrasonic Machining," in Handbook of Manufacturing Engineering and Technology, A. Y. C. Nee Ed. London: Springer London, 2015, pp. 1629-1650.
-
A. Schubert, A. Nestler, S. Pinternagel, and H. Zeidler "Influence of ultrasonic vibration assistance on the surface integrity in turning of the aluminium alloy AA2017," Materialwissenschaft und Werkstofftechnik, vol. 42, no. 7, pp. 658-665, 2011, doi: 10.1002/mawe.201100834.
-
X. Liu, J. Zhang, X. Hu, and D. Wu, "Influence of tool material and geometry on micro-textured surface in radial ultrasonic vibration- assisted turning," International Journal of Mechanical Sciences, vol. 152, pp. 545-557, 2019/03/01/ 2019, doi: https://doi.org/10.1016/j.ijmecsci.2019.01.027.
-
K. Vivekananda, G. N. Arka, and S. K. Sahoo, "Finite Element Analysis and Process Parameters Optimization of Ultrasonic Vibration Assisted Turning (UVT)," Procedia Materials Science, vol. 6, pp. 1906-1914, 2014/01/01/ 2014, doi: https://doi.org/10.1016/j.mspro.2014.07.223.
-
V. Sharma and P. M. Pandey, "Optimization of machining and vibration parameters for residual stresses minimization in ultrasonic assisted turning of 4340 hardened steel," Ultrasonics, vol. 70, pp. 172-182, 2016/08/01/ 2016, doi: https://doi.org/10.1016/j.ultras.2016.05.001.
-
D. V. Sivareddy, P. V. Krishna, A. V. Gopal, and C. P. Raz, "Parameter Optimisation in Vibration Assisted Turning of Ti6Al4V Alloy using ANOVA and Grey Relational Analysis," International Journal of Automotive and Mechanical Engineering, vol. 15, no. 3, pp. 5400-5420, 2018.
-
R. H. Myers, D. C. Montgomery, and C. M. Anderson-Cook, Response surface methodology: process and product optimization using designed experiments. John Wiley & Sons, 2016.
-
S. L. C. Ferreira et al., "Box-Behnken design: An alternative for the optimization of analytical methods," Analytica Chimica Acta, vol. 597, no. 2, pp. 179-186, 2007/08/10/ 2007, doi: https://doi.org/10.1016/j.aca.2007.07.011.
-
W. A. Knight and G. Boothroyd, Fundamentals of metal machining and machine tools. CRC Press, 2005.
-
M. J. Nategh, S. Amini, and H. Soleimanimehr, "Modeling the Force, Surface Roughness and Cutting Temperature in Ultrasonic Vibration- Assisted Turning of Al7075," Advanced Materials Research, vol. 83-86, pp. 315-325, 2010, doi: 10.4028/www.scientific.net/AMR.83-86.315.
-
S. Amini, M. R. Khosrojerdi, R. Nosouhi, and S. Behbahani, "An Experimental Investigation on the Machinability of Al2O3 in Vibration- Assisted Turning Using PCD Tool," Materials and Manufacturing Processes, vol. 29, no. 3, pp. 331-336, 2014/03/04 2014, doi: 10.1080/10426914.2013.864411.