
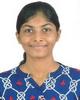
- Open Access
- Authors : Serena Santosh , Sharon Markose , Namitha Susan John , Nishal Koshy Philip, Jacob P Cherian
- Paper ID : IJERTV9IS050274
- Volume & Issue : Volume 09, Issue 05 (May 2020)
- Published (First Online): 19-05-2020
- ISSN (Online) : 2278-0181
- Publisher Name : IJERT
- License:
This work is licensed under a Creative Commons Attribution 4.0 International License
Assessment of Mental Health and Emotional Stability
Serena Santosh, Sharon Markose, Namitha Susan John, Nishal Koshy Philip, Jacob P Cherian
Department of Computer Science and Engineering Saintgits College of Engineering, Pathamuttom
APJ Abdul Kalam Technological University, Thiruvananthapuram, Kerala, India Pin: 695016
Abstract- Early detection and assessment of mental health problems such as depression will help patients receive early treatment, which can enhance the patients quality of life and empower them to live up to their full potential. The research forecasts people's mental health and emotional stability, and seeks to rehabilitate those vulnerable to depression. Depression is a major health issue in the world, especially in India, leading to significant diseases, disability and mortality as well as significant socioeconomic losses. Machine learning techniques can be used to diagnose the depression or anxiety disorders accurately. The project has a web application that people can avail to ensure whether they are mentally stable or not. Unlike existing apps, here the details as well as any family history of depression or anxiety disorders are entered besides answering questions so as to come up with accurate predictions. The machine learning model used here is trained on the responses obtained through survey using PHQ 9 questionnaires. It also includes a module for sentiment analysis using Chatbot that communicates with the user and finds out the reason for depression. The community as a whole will be benefited and every person regardless of age or gender can make use of the application to get an accurate diagnosis. The project can be extended to predicting other mental and behavioral disorders other than depression and thus help in building a mentally fit society.
KeywordsMental Health Diagnosis, Depression, Machine Learning, Prediction, Classification Problems, mental health, Sentiment analysis, mood prediction
-
INTRODUCTION
Based on the U.K. Surgeon Journal (1999), Mental Health is the positive achievement of mental function, resulting in productive activities, satisfying relationships with others, and having the ability to adapt to adversity and to cope. Mental health is thus a definition of our cognitive, behavioral and emotional well-being. It is a matter of how we feel, think and act. Occasionally, the word 'mental health' is used in a way that means absence of a mental illness. The term mental illness generally refers to all mental disorders that can be diagnosed health conditions that are marked by altered or damaged changes in thought, mood, or behaviour. Mental health and mental illness are two continuous terms. Those with good mental health may also suffer from mental illness, and those without mental illness may also have poor mental health.
Issues of mental health may emerge from stress, isolation, depression, anxiety, relationship issues, a loved one's death, suicidal thoughts, grief, addiction, ADHD, self-harm, various mood disorders or other mental disorders of varying degrees, as well as learning disabilities. Therapists, counselors, psychologists, social workers, nurse practitioners, or doctors may help manage mental illness with therapies such as counseling, counselling, or medication.
Mental disorders affect a large number of children, teenagers, elderly and middle-aged groups living in India's urban and rural areas. Depression triggers include biological, physical, economic, and cultural factors, which start with environmental factors. It is the cause and effect of non- communicable diseases, disorders of substance use and dietary disorders, and is also related to communicable diseases such as TB, HIV and others. Depression and Suicide are inextricably linked. Depression can lead to suicide, as well. Approximately one person in 5,000-15,000 dies each year as a consequence of suicide (1.4 per cent of all deaths), and the numbers will increase significantly in future. If we can ensure that everyone's mental wellbeing is fine, we will avoid this. For this, recognizing depression at an early stage and providing treatment is critical. A lot of data related to the mental health of people is available and by extracting the key features and analyzing the similarities between those prone to depression, we can predict those who are likely to be mentally unstable. These predictions can be done using machine learning algorithms. Once the prediction is done, those who are vulnerable can be rehabilitated by counselling and medication.
All emotions are the impulses to act, the instant plans to govern life, instilled into us by nature. The very root of the word emotion is the motere of the sense of the Latin word 'to move', plus the suffix 'e' to connote to move away, suggesting a tendency to act in each emotion. Therefore emotions are the fundamental source of human energy, desire, and motivation. This stimulates our innermost feelings and purpose in life, and turns them into the things we think and the values we live by. In the human mind, mental well-being has been studied and accepted as a personality representation. Under the self-organizational theory framework it is characterized as a property to mark whether or not a complex emotional system can sustain its equilibrium effectively. We find a raging sense of out of control emotions in society. Everyday news comes to us rife with stories of disintegration of civility and stability, an assault of mean spirited impulses
running amok. Such outbursts of emotional ineptitude, desperation and recklessness are a pervasive and growing ill will that has exposed a spike in depression and a rising tide of violence. Some questionnaires help doctors determine the extent of the depression. The rating scale for Hamilton depression is one such test with 21 questions, resulting in ratings reflecting the seriousness of the disorder. The Hamilton Scale for physicians assessing depression is one of the most widely used measurement instruments in the world. More specific device is the PHQ-9. Patient Health Questionnaire, is an exam that patients may take to test for symptoms of mental health. Specifically the PHQ-9 screens for symptoms and signs of depression. This consists of nine short and simple questions, based on the depression criteria defined in Diagnosis and Statistical Manual of Mental Disorders, 4th edition.
-
LITERATURE REVIEW
Many articles have been published over the years, relevant to this project. Critical evaluation of the use of research tools in evaluating quality of life for people with schizophrenia, 2007, by Jeanette Hewitt[1] provides information on schizophrenia and two commonly used methods for treating the same. But there is no concern about other mental disorders, the causes and the protocols for recovery. The psychological costs of unsustainable housing commitments[2], 2006, by Mark P Taylor, David J Pevalin and Jennifer Todd is concerned with how a person's psychological well-being is influenced by house loans, threats of eviction and so on. It was easier to provide some solutions to this problem, since that is not the emphasis here. One way to examine the question that is not stated in this paper is also those trends shown by people living in the same geographical location.
Consequences of antenatal mental health problems for child health and development [3] 2007, by Hollins and Kathryn deals with the connection between maternal health issues and their effects on foetal development. The studies focus on the mother but don't offer any sense to the family backgrounds and the community se lives in. These factors also influence her mental state, and hence her foetus' well-being. Investigating the Evidence for the Effectiveness of Risk Assessment in Mental Health Care, 2012, by Timothy Wand
[4] discusses the importance of assessing risk factors linked to mental health problems. This explains how the mindset of society that such people are dangerous must be modified, because those who are really aggressive are the people under the influence of alcohol and drugs. Some of the things the paper does not discuss is that sometimes other forms of mental illness contribute to intemperate and violent behavior which is the main reason people have a misconception about mental problems.'Demographic, physical and mental health assessments in the adolescent brain and cognitive development study: Rationale and description [5], 2017, by Deanna M Barch and her co-authors is concerned with adolescent mental health. The advantage of having separate tests for different age groups is clearly illustrated. Such concerns are more
likely to develop in adolescents. But that is not the subject of this article.
Beyond McNaghten: Perspectives On Criminal Law Relating to Insanity, 2019, by Dr. Amitabh S Saxena[6] talks about how insanity leads to criminal behavior. The emphasis here is on the defendant who is seen as a mentally ill person, which will ultimately lead to his acquittal. This strategy is problematic if the person knows what is right or wrong even though he is mentally unstable. Our proposed project "Assessment of Mental Health and Emotional Stability" takes all of these drawbacks into account and suggests an effective approach to evaluation.
Illiou Machine Learning Data Preprocessing Method for Suicide Prediction from Family History, 2019, by Theodoros Illiou, Georgia Konstantopoulo, Christina Lymperopoulo, Konstantinos Anastaspoulos, George Anastassopoulos, Dimitrios Margounakis, Dimitrios Lymberopoulos, describes the comparison of Illiou preprocessing method with Principal Component Analysis in prediction of suicide based on family history. The dataset consisted of 360 students aged between 18 and 24 years, and whose family history indicated problems. The output of preprocessing methods for Iliou and Principal Component Analysis data was evaluated and compared using the 10-fold cross validation method that assessed ten classification algorithms, J48, Random Forest, MLP, JRip, RBF, SMO, Naïve Bayes, IB1, HMMand AdaBoostM1. Experimental results showed that the preprocessing algorithm of Iliou data out-performs the preprocessing method of the primary component analysis data. In classification results it hit 100 percent against 71.34 percent. Iliou preprocessing approach is found to be the most suitable one for suicide prediction according to classification tests. Suicide is a major public health problem with increasing rates in many developed countries as well as developing ones. It is one of the leading causes of death for all groups Factors often associated with increased suicide risk include physical health issues, socio- demographic factors, psychological morbidity and biological backgrounds.
Identification of Suicidal Tendencies of Individuals Based on the Quantitative Analysis of their Internet Texts, 2017, by Tatiana A. Litvinova, Pavel V. Seredin, Olga A. Litvinova, Olga V. Romanchenko, talks on texts by suicidal individuals (mostly suicidal notes or literary texts by well- known people, e.g.
authors, essays, etc.) and texts by individuals in the control group using preprocessing software (mostly LIWC). Models designed to identify the texts as those written by or not suicidally tendentious individuals are quite popular. Research was conducted in English for texts, and due to the linguistic complexity it was found to have many restrictions. In this paper, mathematical models were developed using the numerical values of the linguistic parameters as features to identify the texts as those by suicidal or non-suicidal individuals. Texts especially blogs by people who committed suicides were processed with user dictionaries using the Russian version of LIWC. The use of features in the model is
not highly dependent on the content as not all individuals who committed suicides dealt with the topic in the texts. The resulting model was found to be accurate at 71.5 per cent. The method showed that the classification of texts was only fairly accurate. Listed features were as independent as possible of the material (comma proportions, function names, etc.). The suggested method uses only a relatively small number of features and predictions based solely on text material. No other important parameters such as family history and other medical parameters were taken into consideration.
Both Hair Cortisol Levels and Perceived Stress Predict Increased Symptoms of Depression: An Exploratory Study in Young Adults, 2013, by Gerber M, Kalak N, Elliot C, Holsboer-Trachsier E, Puhse U, Brand S defines depression as a common mental health issue in young adults but the physiological mechanisms that mediate between stress and depressive symptoms are not clear.
This study examined how concentrations of hair cortisol were linked to self-perceived stress and young adult symptoms, and testing was done to find out whether levels of hair cortisol could be used to explain the variation in depressive symptoms beyond perceived stress before and after rates of extreme physical activity were regulated. The study consisted of 42 university students in exercise and health science, which consisted of 20 males and 22 females with 21.2 years of mean age. Concentrations of cortisol were drawn from the hair strands nearest to the scalp. Participants were also asked to complete self-rated questionnaires on the symptoms of depression and perceived stress. The results showed lower depressive symptoms as well as lower perceived stress reported by the students with higher levels of hair cortisol. Increased perceived stress was reported as associated with elevated depression symptoms. Measurement of hair cortisol may serve as a biomarker of chronic stress and mental disorders but further research is required. The prediction was based solely on a single biomarker. No account has been taken of other environmental or genetic factors.
As of today, apps such as Moodpath that ask you in-the- moment questions over a series of 14 days to measure your emotional well-being and CBT Depression, which through information posts, diary-like mood monitoring, and built-in motivational features, help you change your thinking patterns for a better mood along with depression severity tests that allow you to assess your progress.
-
EXPERIMENTAL WORK
Machine learning approaches can be used to determine emotional stability and mental health. The data to be trained is based on clinically proven methods such as PHQ-9 which entail a collection of nine questions assessing depression of a person. Along with this, to evaluate the anxiety among young people, another clinically tested method GAD-7 which comprises of 7 questions is used. Some additional information such as the family history of depression that helps in arriving at a better result is also included. Fig.1. indicates the design diagram of the work and shows the steps followed. The data was collected through questionnaires
circulated among the required age group 13 to 25. Since the data is already labeled using the scoring of the forms, supervised learning method is employed and the prediction will be either of the two classes – depressed or not depressed, based on which it can be identified whether the person requires help or not. The focus is mainly on the youth, since they are most prone to mental health disorders like depression.
The collected dataset is trained to find the one with the highest accuracy and efficiency for different Machine learning models. Until finalizing the model, models such as KNN, Logistic Regression, Random Forests, Linear Regression, Boosting algorithm and Decision rees were compared. To analyze the relationship between different attributes graphical plots were drawn. The plots between the attributes and labels are shown in Fig. 2,3,4 and 5. The attributes on which the label most dependent on were found to be age, gender ,family history and the responses to the three questions which are Little interest or pleasure in doing things; Feeling down, depressed, or hopeless; Trouble falling or staying asleep, or sleeping too much. The Adaboost model has the greatest accuracy for this particular problem.
AdaBoost is a method of training a boosted classifier. A boost classifier is a classifier that can be represented in the form
(1)
Here each is a weak learner that takes input and a value is returned that indicates the class of the object. Each weak learner produces a hypothesis as output, for each sample in the training set. At each , a weak learner is selected and a coefficient is assigned such that the sum training error of the resulting -stage boost classifier is minimized. The sum training error is given by
(2)
Here is the boosted classifier that is built up to the previous stage of training, is some error function and is the weak learner that is being considered for addition to the final
Classifier. At each iteration, a weight is assigned to each sample in the training set equal to the current error. The coefficient is given by
(3)
The Sentiment Analyzer module using Chatbot analyzes the user's text input and predicts the cause of depression.
-
RESULTS
In this section, after the performance analysis of some classification algorithms, Boosting algorithm outperformed the others with an accuracy score of 0.9. The accuracy of the classifier depends on how well a classifier classifies the dataset being tested. It is measured by the area under the ROC Curve. So using the boosting classifier, when an input is given by the user, the model correctly classifies whether the person suffers from depression or not. The final diagnosis is also dependent on the input from the sentiments analyzer module as well.
This plotting is between the important features Family history v/s Depressed. The information acquired from this plot is: – 0 indicates there is no family history of depression and 1 indicates there is family history of depression, the first bar shows that almost 70% are not depressed and the rest are
depressed. The second bar shows that among the people who has had a family history half are not depressed and another half are found to be depressed.
This plot is between little interests in doing things v/s depressed. There are 4 options for this question and points are given as 0,1,2,3. The first bar shows those who have point 0 and among those 90% are not depressed and the rest are depressed. The second bar shows those who have point 1 and among those almost 70% are not depressed and the rest are depressed. The third bar gives the people who got points
2 and in that about 50% are not depressed and 50% are depressed. The last bar shows the people who got 4 points and 60 % are found to be not depressed and the rest are depressed.
The above plot is between Trouble falling asleep v/s Depressed. The options for this question are given points as 0,1,2,3. The first bar shows those who got point 0 and around 95% are not depressed while the rest 5% are found to be depressed. The second bar shows people with point 1 and 70% of them are not depressed while the remaining 30% are depressed. The third bar shows people who got point 2 and among them 45% are not depressed and the rest are found to be depressed. The last bar indicates point 3 and almost 35% are not depressed and the rest are depressed.
The above plot is between Feeling depressed v/s Depressed and for this question also the points are given as 0,1,2,3. The first bar indicates the people with point 0 and almost 96% are not depressed the rest 4% are found to be depressed. The second bar shows those with point 1 and among those 60% are not depressed the remaining are depressed. The third bar shows the ones that got point 2 and only 10% are not depressed the rest are depressed. The last bar shows the people with point 4 and among those 5% are not depressed while the rest are depressed.
-
CONCLUSION
Expert programs in the field of mental health are also developed to forecast the issue of mental health at an earlier stage. Today, a variety of expert systems are used in the medical domain to predict diseases correctly at an early stage, so care can be done effectively and efficiently. The patient should be able to get proper treatment right away, based on the predictions. Automation in this field is a giant leap, since it eliminates all human limitations. This method is faster, easily accessible and guarantees privacy to the individuals. The application can also be linked with psychologists/psychiatrists, who can follow up who are mentally unstable or those who are vulnerable to the same. The data set is very small, and the work may be extended in the future to achieve greater precision through a wide set of data, to predict any mental illness. The classifiers must be trained and validated before applying any techniques in real prediction. Chatbot can also be further extended to predict the cause of any mental health problem, not just depression.
-
REFERENCES
-
J. Hewitt, Critical evaluation of the use of research tools in evaluating quality of life for people with schizophrenia, 2007.
-
M. P. Taylor, D. J. Pevalin and J.ToddThe psychological costs of unsustainable housing commitments, 2006.
-
Hollins and Kathryn, Consequences of antenatal mental health problems for child health and development, 2007.
-
T.Wand, Investigating the Evidence for the Effectiveness of Risk Assessment in Mental Health Care, 2012.
-
D. M. Barch, Demographic, physical and mental health assessments in the adolescent brain and cognitive development study: Rationale and description, 2017.
-
Dr. A. S. Saxena, Beyond McNaghten: Perspectives On Criminal Law Relating To Insanity, 2019.
-
https://www.indiatoday.in/education-today/gk-current-affairs/stor y/india-is-the-most-depressed-country-in-the-world-mental-health-da y-2018-1360096-2018-10-10
-
https://yourstory.com/herstory/2019/10/world-mental-health-day- women-suicides
-
https://www.livemint.com/Politics/YCw8vC0qZUzAYkWSEVXS 9N/Suicides-in-India-What-data-shows.html