
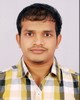
- Open Access
- Authors : Mafiz Uddin Ahmed, Anil Kumar Godea
- Paper ID : IJERTV13IS080071
- Volume & Issue : Volume 13, Issue 08 (August 2024)
- Published (First Online): 31-08-2024
- ISSN (Online) : 2278-0181
- Publisher Name : IJERT
- License:
This work is licensed under a Creative Commons Attribution 4.0 International License
Assessment of Optimum MPGE of An Electric Vehicle & Design of A Safer Battery Pack
Mafiz Uddin Ahmed
Cyient Ltd. (Hyderabad)
Abstract Conventional automobiles have an adverse effect on the climate due to their fuel use and subsequent emissions of CO2, which lead to air pollution. It is well accepted that these emissions are the cause of a global temperature shift and warming. Choosing electric vehicles is environmentally friendly. When compared to internal combustion engines, electric vehicles don’t contaminate the air. There are no emissions, and the surrounding air remains clean.
In this project, Ricardo Ignite has been used to design and simulate the models. The conventional Internal combustion engine model is designed and simulated according to the drive cycle and calculated the various results. The electric vehicle model is then modelled and simulated and thus calculated the MPGe (Miles per gallon of gasoline equivalent). Thus, compare the amount of energy consumed by the electric vehicle to that of the traditional Internal combustion engine. According to the drive cycles and various parameters, the miles per gallon equivalent of the electric vehicle of 4 different model will be computed. Thus, an optimized set of parameters is obtained for maximum MPGe.
In the second part of the project, it focusses with the development of the battery management system for the safe operation of the battery pack. A BMS is basically the brain and heart of a battery pack. It controls the battery pack’s temperature, charge level, voltage protection, and cell health monitoring. A battery controller is designed. It gives the SOC, current, battery voltage and load voltage for both charging and discharging.
Keywords Ricardo Ignite; Miles Per Gallon of Gasoline Equivalent; battery management system; Battery pack
- INTRODUCTION
- Background on Ricardo Ignite
Ricardo Ignite is a proper tool to model complex physical system and their sub-systems. It is a physics-based package originated for complete vehicle system modelling and simulation. It features comprehensive built-in automotive Modelica libraries. It allows users to speedily and accurately model conventional and highly complex vehicle system models which includes hybrid-electric, full electric and novel vehicles.
How it works:
Ricardo Ignite interfaces with design and optimisation tools and supply models for all phases of the development process with faster than real-time simulation. Users can simulate a wide range of applications which includes but are not limited to vehicle performance, system specification, fuel economy, handling, driveability and thermal management.
In this project, Ricardo Ignite has been used to design and simulate the models. The conventional Internal combustion engine model is designed and simulated according to the drive cycle and calculated the various results. The electric vehicle
Anil Kumar Godea
Cyient Ltd. (Hyderabad)
model is then modelled and simulated and thus calculated the MPGe (Miles per gallon of gasoline equivalent). Thus, compare the amount of energy consumed by the electric vehicle to that of the traditional Internal combustion engine. [14]
- Model of IC Engine
Figure 1: IC Engine Model using Ricardo Ignite
Using Ricardo Ignite tool, the Engine model for an Internal Combustion is modelled and shown in the figure above.
Figure 2: Fuel Consumption Output representation using Ricardo Ignite
Mathematical Representation of the model to calculate fuel consumption is prepared in Ricardo Ignite.
R-Post of IC Engine model:
Figure 3: Vehicle Speed using Ricardo Ignite
R-Post results for the vehicle speed of IC Engine model using Ricardo Ignite tool is shown in the figure above.
Figure 4: Fuel consumption using Ricardo Ignite
R-Post results for the Fuel consumption of IC Engine model using Ricardo Ignite tool is shown in the figure above.
- Model of Electric Vehicle
Figure 5: Electric Vehicle Model using Ricardo Ignite
Using Ricardo Ignite tool, the Engine model for an Electric vehicle is modelled and shown in the figure above.
R-Post of Electric vehicle model:
Figure 6: Vehicle speed using Ricardo Ignite
R-Post results for the vehicle speed of Electric Vehicle Engine model using Ricardo Ignite tool is shown in the figure above.
Figure 7: MPGe and SOC using Ricardo Ignite
R-Post results for the Miles Per Gallon Equivalent of Gasolene and SOC of an electric vehicle model using Ricardo Ignite tool is shown in the figure above.
We have used FTP72 (Federal Test Procedure) Drive Cycle for Electric vehicle. The cycle simulates an urban route of 12.07 km with frequent stops. the utmost speed is 91.25 km/h and the average speed is 31.5 km/h. Whereas, for combustion Engine NEDC (New European Drive Cycle) has been used. This drive cycle is intended to assess the emission levels of car engines and fuel economy in passenger vehicles. MPGe i.e., Miles per gallon of Gasoline equivalent is an energy efficiency matrix. It was introduced by the environmental protection agency to compare the amount of energy consumed by other fuel vehicles thereto of traditional gas-powered vehicle. [12][13]
- Background on Ricardo Ignite
- OPTIMIZATION OF EV PARAMETERS
In Ricardo Ignite, we are trying to compare simulations of four different electric vehicle model which are presently available in the Indian market. We have noted down the parameters of each of the model and then calculated the MPGe.
The four models taken are: Model 1: Tata Tigor EV Model 2: Mahindra E-Verito Model 3: Tata Nexon EV
Model 4: MG ZS EV
The basic parameters which affect the value of MPGe of an electric vehicle are:
-
- Initial State of charge (SOC_initial) in %
- Area(A), 2
- Nominal Voltage (V_nom), V
- Coefficient of Rolling radius (Coeff_RR)
- Tyre Radius (T_radius), m
- Motor Power (Pm), W
- Wheel Inertia (J_wheel), 2
- Mass of vehicle (Mass), kg
- Capacity of battery (C_bat), Ah
- Coefficient of drag of air (Cd)
- Final Drive ratio (FDR)
- Motor Torque (Tm), Nm
- Tractive efficiency (Trac_eff) [1]
(Cas e1) Defa ult Case2( tata tigor ev) Case3(Ma hindra e- verito) Case4( tata nexon ev) Case5( MG ZS EV) SOC_i nit 0.3 0.3 0.3 0.3 0.3 A 2.73 2.19 2.277 2.472 2.49 V_no m 375 72 72 320 394 Coeff_ 0.00 0.008 0.008 0.008 0.008 RR 8 T_radi 0.38 0.355 0.355 0.064 0.4318 us 14 Pm(W 2350 30000 31000 94879 10500 ) 00 0 J_whe el 2 1.0082 1.76 1.65 1.844 Mass 2154 1590 1704 1790 1966 C_bat( 136 225 288 94.375 112.94 Ah) 4 Cd 0.24 0.24 0.24 0.24 0.24 FDR 9.73 10.83 9.1 Tm 440 105 91 245 350 Trac_ eff 0.85 0.85 0.85 0.85 0.85 Table 1: Various Parameters of EV Models [1][4]
Some of the parameters like Initial SOC, Coefficient of Rolling radius, Coefficient of drag of air, Tractive efficiency are taken as same for all the cases.
Setup 1:
Setup 2:
Secondly, we considered on optimizing each of the parameters. For this, the parameter which is on focus is kept as it is for all the cases keeping all other parameters same as the default case. And then went on comparing the effect of the focused parameter.
The optimum value of the focused parameter is then noted down and in the next simulation, all parameters is kept constant except the next focused parameter. This is repeated till all the parameters are optimized. Thus, an optimized set of parameters is obtained for maximum MPGe.
Result for Setup 1:
Figure 8: MPGe for all the 5 cases using Ricardo Ignite
Figure 9: Mean MPGe value for all 5 cases using Ricardo Ignite
The mean MPGe values are:
Case 1: i.e., Default is 110.99 MPGe
Case 2: i.e., Model 1: Tata Tigor EV is 114.78 MPGe
Case 3: i.e., Model 2: Mahindra E-Verito is 104.888 MPGe Case 4: i.e., Model 3: Tata Nexon EV is 130 MPGe
Case 5: i.e., Model 4: MG ZS EV is 121.994 MPGe
Firstly, we have simulated all the cases together taking respective parameters into account and calculated the MPGe. The model having the maximum MPGe is noted down.
Thus, Tata Nexon EV is having the best value of 130 MPGe out of the remaining models.
Result for Setup 2:
Optimizing parameters:
- For Frontal Area (A):
Case order: case 2 > case 3 > case 4 > case 5 > case 1
Mean MPGe: 117.295, 116.24, 113.917, 113.708, 110.99
Area: 2.19, 2.277, 2.472, 2.49, 2.73
i.e., Lower the Frontal Area, Higher is the MPGe.
Figure 10: Mean MPGe value of all 5 cases for Frontal Area using Ricardo Ignite
- For Nominal Voltage (V_nom):
Case order: case 5 > case 1 > case 4 > case 2 = case 3
Mean MPGe: 111.144, 110.99, 110.388, 73.9964, 73.9964
V_nom: 394, 375, 320, 72, 72
i.e., Higher the Nominal Voltage, Higher is the MPGe.
Figure 11: Mean MPGe value of all 5 cases for Nominal Voltage using Ricardo Ignite
- For Tyre Radius (T_radius):
Case order: case 5 > case 4 > case 1 > case 2 = case 3
Mean MPGe: 111.181, 111.11, 110.99, 110.835, 110.835
T_radius: 0.4318, 0.4064, 0.3814, 0.355,
0.355
i.e., Higher the Tyre Radius, Higher is the MPGe.
Figure 12: Mean MPGe value of all 5 cases for Tyre Radius using Ricardo Ignite
- For Mass (gross weight of vehicle):
Case order: case 2 > case 3 > case 4 > case 5 > case 1
Mean MPGe: 136.94, 130.662, 126.313, 118.321, 110.99
Mass: 1590, 1704, 1790, 1966, 2154
i.e., Lower the weight of the vehicle, Higher the MPGe.
Figure 13: Mean MPGe value of all 5 cases for Mass using Ricardo Ignite
- For Capacity of battery (C_bat):
Case order: case 3 > case 2 > case 1 > case 5 > case 4
Mean MPGe: 111.047, 111.032, 110.99, 110.968, 110.942
C_bat: 288, 225, 136, 112.944, 94.375
i.e., Higher the Capacity of Battery, Higher the MPGe.
Figure 14: Mean MPGe value of all 5 cases for Battery Capacity using Ricardo Ignite
- For Motor Torque (Tm):
Case order: case 1 > case 5 > case 4 > case 2 > case 3
Mean MPGe: 110.99, 110.972, 110.95, 109.195, 106.798
Motor Torque: 440, 350, 245, 105, 91
i.e., Higher the Motor Torque, Higher the MPGe.
Figure 15: Mean MPGe value of all 5 cases for Motor Torque using Ricardo Ignite
- For Final Drive Ratio (FDR):
Case order: case 4 > case 2 = case 5 = case 1 > case 3
Mean MPGe: 111, 110.991, 110.991, 110.991, 110.967
FDR: 9.1, 9.73, 9.73, 9.73, 10.83
i.e., Lower the FDR, Higher the MPGe.
Figure 16: Mean MPGe value of all 5 cases for Final Drive Ratio using Ricardo Ignite
- For Wheel Inertia (J_wheel):
Case order: case 2 > case 4 > case 3 > case 5 > case 1
Mean MPGe: 111.422, 111.153, 111.101, 111.063, 110.99
J_wheel: 1.0082, 1.65, 1.76, 1.844, 2
i.e., Lower the wheel Inertia, Higher the MPGe.
Figure 17: Mean MPGe value of all 5 cases for Wheel Inertia using Ricardo Ignite
- For Motor Power (Pm):
Case order: case 2 > case 3 > case 4 > case 5 > case 1
Mean MPGe: 111.68, 111.561, 111.232, 111.187, 110.99
Pm: 30000, 31000, 94879, 105000, 235000
i.e., Lower the Motor Power, Higher is the MPGe.
Figure 18: Mean MPGe value of all 5 cases for Motor Power using Ricardo Ignite
Optimum parameters obtained are:
From the above simulation results, we can see the parameters which has a higher impact on the MPGe. The table shown below is written with the most significant parameter first followed by the rest in order.
Parameters Value Mass 1590 kg Frontal Area 2.19 m^2 Motor Power 30,000 W J_wheel 1.0082 kgm^2 Tyre Radius 0.4318 m Nominal Voltage 394 V Battery Capacity 288 Ah Final Drive Ratio 9.1 Motor Torque 440 Nm Table 2: Optimum Parameters obtained from Simulation performed
It can be seen that, the mean value of MPGe for Mass and Frontal Area is significantly larger than other parameters. Thus, we can say that, Mass (gross weight) and Frontal Area of the vehicle are the dominant parameters which has higher impact on MPGe.
OPTIMUM MODEL:
Figure 19: MPGe For Optimum Model using Ricardo Ignite
Comparing the Best model of SETUP 1 and Optimum model of SETUP 2:
Figure 20: MPGe for Best model of setup1 and Optimum model of setup2 using Ricardo Ignite
Figure 21: Mean MPGe For Best model of Setup1 and Optimum model of setup2 using Ricardo Ignite
-
- BATTERY MANAGEMENT SYSTEM
- INTRODUCTION
A battery management system (BMS) is basically the heart and brain of a battery pack. Its an electronic system that controls a rechargeable battery (cell or battery pack) by protectig the battery from working outside its safe operating area, monitoring its state, calculating secondary data, delineating that data, controlling its environment, authenticating it and balancing it. A BMS monitor the condition of the battery as represented by various terms, such as:
- Voltage: voltages of individual cells, or voltage of periodic taps and total voltage.
- Temperature: average temperature, coolant intake and output temperature and temperatures of individual cells.
- Coolant flow: for air or fluid cooled batteries.
- Current: current that comes in or out of the battery. [11]
Figure 22: Battery Management system (BMS) [11]
- BASIC TERMS IN BMS
State of Charge (SOC):
State of charge (SOC) is the level of an electric battery relative to its capacity. The units of state of charge are percentage points (0% = empty; 100% = full).
Depth of Discharge (DOD):
The Depth of Discharge (DOD) of a battery decides the fraction of power that can be withdrawn from the battery.
State of Health (SOH):
The SOH is a measurement that study the general condition of a battery and its ability to deliver the specified performance compared with a fresh battery. The units of SOH are percent points (100% = condition of the battery match the battery’s specifications). It is a measure of the long-term capability of the battery and gives an indication, not an absolute measurement, of how much of the available lifetime energy of the battery has been consumed and how much of it is remaining.
Nominal Cell Voltage:
It is the average voltage a cell outputs when charged. The nominal voltage of a battery relays on the chemical reaction behind it.
Nominal cell capacity:
It specifies the quantity of charge, in ampere hours (Ah) or milliampere hours (mAh), that the cell is rated to hold.
C-rate:
C-rates governs the charge and discharge rates of battery. Battery capacity is commonly rated at 1C, meaning that a totally charged battery rated at 1Ah should provide 1A for 1 hour. Discharging of the identical battery at 0.5C should provide 0.5A for 2 hours, and at 2C it delivers 2A for half an hour. [9]
- CELL BALANCING
While connecting the cells in series and parallel, it is ensured that they are of the same voltage and chemistry but once the battery pack is installed and subjected to charging and discharging the voltage values of the individual cells tends to vary which causes cell imbalance over a period. Cell imbalance can cause cell degradation, incomplete charging of the pack, incomplete use of pack energy. Hence, to maintain a proper battery pack health it is important that the cell undergo balancing during their charging cycle. [3]
Imbalance in cell can be caused by:
- Internal cell resistance variation
- Temperature of the cell
- SOC imbalance of any cell
- Repeated cycle of charge and discharge with voltage equalization/correction [3]
Figure 23: Cell Imbalance [5]
Cell balancing is a technique which ensures:
- Individual cell/module voltage level is maintained equal.
- Maintain maximum efficiency of the battery pack by voltage equalization/correction [3]
Figure 24: Cell Balancing [5]
There are Two types of Cell balancing:
- Active Cell Balancing
- Passive Cell Balancing
Active Cell Balancing:
In Active Cell Balancing:
- Charge is transferred actively from a cell with higher voltage to a cell with lower voltage using an internal circuit/logic
- Achieved by using charge storing components like Capacitors and Inductors
Passive Cell Balancing:
In Passive Cell Balancing:
- In this method a resistor is used to discharge the excess voltage of a cell which is at higher voltage, to equalize with other cells. This excess voltage/charge is dissipated as heat.
- Each cell connected in series has its own bypass resistor connected through a switch like MOSFET. [3]
- Charging and Discharging of Battery using MATLAB
Figure 25: Design of Battery Controller using MATLAB
When the voltage source is disabled, the battery will supply the load. When the voltage source is enabled, the battery will charge and the load will be supplied from the voltage source. Here in this model the battery specifications are taken as: Nominal Voltage (V) =24 V
Rated Capacity (Ah) = 50 A Initial SOC = 45 %
There are two cases:
- Charge
- Discharge
During Charge mode:
Charging Methods:
There are three common methods of charging a battery; constant voltage, constant current and a mix of constant voltage/constant current with or without a wise charging circuit. Constant voltage: It allows the full current of the charger to flow into the battery until the power supply reaches its pre-set voltage. The current will then taper down to a minimum value once that voltage level is reached. The battery can be left connected to the charger until ready for use and will remain at that float voltage, trickle charging to compensate for normal battery self-discharge.
Constant current: It is a simple form of charging batteries with the current level set at approximately 10% of the maximum battery rating. Charge times are relatively long with the disadvantage that the battery may overheat if it is over-charged, leading to premature battery replacement. This method is suitable for Ni-MH type of batteries. The battery must be disconnected, or a timer function used once charged.
Constant voltage / constant current (CVCC): It is a combination of the above two methods. The charger limits the amount of current to a pre-set level until the battery reaches a pre-set voltage level. The current then reduces as the battery becomes fully charged. The lead acid battery uses the constant current constant voltage (CC/CV) charge method. A regulated current raises the terminal voltage until the upper charge voltage limit is reached, at which point the current drops due to saturation. [6]
In this particular model, the allowed maximum current is 22A. Some battery companies separate the constant current and constant volage sides at 80% SOC level. The open circuit voltage at 80% SOC is 25.98V. A current based and voltage- based control effect are shown. We need PI controller for determining the reference battery current. When the ideal switch is set to 1, the battery is in charge mode and when it is set to 0, the battery is in Discharge mode.
Both the Charging and Discharging modes are combined together with the use of a switch. It will determine the mode. Charging or Discharging. It should work based on voltage source. Because we determine the modes based on the source
Output Graphs:
Figure 26: Simulation result for Charging using MATLAB
The battery is in Charge mode. SOC is increasing. The reference current is in maximum level i.e., 22A when the battery voltage is lower than 25.98V. Then it reduced by the voltage control PI and the battery is charging with 25.98V and 16A. While the battery is charging, the battery voltage will increase. Because of this, charge current should decrease. In any case the supply of the load is 48V.
Figure 27: Simulation result for Discharging using MATLAB
The reference voltage for load is 48V. We used different PI controller for Discharge mode. The battery is in Discharge mode. SOC is decreasing and the battery current is positive.
- INTRODUCTION
- CONCLUSION
According to the drive cycles and various parameters, the miles per gallon equivalent of the electric vehicle of 4 different model were computed. Thus, an optimized set of parameters is obtained for maximum MPGe.
We have seen from setup 1 that if we do the simulation of all the cases together taking respective parameters into account and then calculate the MPGe. The model having the maximum value of mean MPGe is case 4 i.e., It has a mean value of 130 MPGe.
From setup 2, it can be seen that the mean value of MPGe for Mass and Frontal Area is significantly larger than other parameters. Thus, we can say that, Mass (gross weight) and Frontal Area of the vehicle are the dominant parameters which has higher impact on MPGe. Lower the Mass or gross weight of the vehicle, Lower will be the effort required to overcome the resistive forces thus giving higher MPGe. And also, Lower the Frontal Area of the vehicle, lower will be the Aerodynamic drag acting on it. Thus, results in better MPGe value.
On comparing the best model of setup 1, i.e., Model 3: Tata Nexon EV and optimum model which we obtained after taking all the optimized parameters. We have seen that the the optimized model gives better mean MPGe value than Tata Nexon EV.
In order to safely manage the battery pack an electronic system called the Battery management system (BMS) needs to be built. A BMS is basically the brain and heart of a battery pack. It manages the temperature, state of charge, voltage protection and cell health monitoring of the battery pack.
To maintain a proper battery pack health, it is important that the cell undergo balancing during their charging cycle. It is done in two ways Active cell balancing and Passive cell balancing.
A battery controller is designed. It gives the SOC, current, battery voltage and load voltage for both charging and discharging. A current based and voltage-based control effect are shown. We need PI controller for determining the reference battery current. When the ideal switch is set to 1, the battery is in charge mode and when it is set to 0, the battery is in Discharge mode. Both the Charging and Discharging modes are
combined together with the use of a switch. It will determine the mode, Charging or discharging. It should work based on voltage source. Because we determine the modes based on the source. When the battery is in Charge mode, SOC is increasing. The reference current is in maximum level i.e., 22A when the battery voltage is lower than 25.98V. Then it reduced by the voltage control PI and the battery is charging with 25.98V and 16A. While the battery is charging, the battery voltage will increase. Because of this, charge current should decrease. In any case the supply of the load is 48V. The reference voltage for load is 48V. We used different PI controller for Discharge mode. The battery is in Discharge mode. SOC is decreasing and the battery current is positive.
REFERENCES
- https://sist.sathyabama.ac.in/sist_coursematerial/uploads/SEEA3028.pdf
- https://www.avnet.com/wps/portal/abacus/solutions/markets/automotive- and-transportation/automotive/power-train-and-ev-hc-phev-systems/ev- on-board-chargers/
- https://www.youtube.com/watch?v=ONmyxtp-p54
- https://www.cardekho.com/
- https://francebattery.com/wp-content/uploads/2021/06/equilibrage- batteries.png
- https://www.us.lambda.tdk.com/resources/blogs/20160526.html.
- Etkin Özen. Design of Smart Controllers for Hybrid Electric Vehicles.
Middle East Technical University. Ankara. Turkey. August 2005
- Leon. C. Rosario. Power and Energy Management of Multiple Energy Storage
- www.wikipedia.org [10]https://nptel.ac.in/