
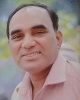
- Open Access
- Authors : Kailas Govinda Khadse , Aditya Kailas Khadse
- Paper ID : IJERTV9IS040267
- Volume & Issue : Volume 09, Issue 04 (April 2020)
- Published (First Online): 16-04-2020
- ISSN (Online) : 2278-0181
- Publisher Name : IJERT
- License:
This work is licensed under a Creative Commons Attribution 4.0 International License
Assessment of Wastewater Treatment Plant Performance using Capability Indices
Estimating Capability of Wastewater Treatment Processes
Kailas Govinda Khadse Department of Statistics, Moolji Jaitha College, Jalgaon, India
Aditya Kailas Khadse
Digitate,
Tata Consultancy Services Ltd, Pune, India
Abstract In usual practice, performance of wastewater treatment plant is assessed by percentage removal of Chemical Oxygen Demand (COD), Biochemical Oxygen Demand (BOD) and Total Suspended Solids (TSS) from wastewater after processing. Besides these, while measuring performance of wastewater plant environmental engineers also expect to consider the status of characteristics such as pH, NH4-N, N- Total, fecal coliform, etc at outlet. The problem with usual practice of performance assessment is that there is no direct comparison between these characteristics process distribution at outlet stage and effluent discharged standards or specification limits developed by Central Pollution Control Board (CPCB) for these characteristics. Considering this lacuna in mind, in this paper we propose and use probability based Process Capability Indices (PCIs) and Multivariate Process Capability Indices (MPCIs) which compares the output of wastewater treatment process with the process specifications and quantify how well the process performs. PCIs have been widely used in evaluating the performance of manufacturing processes and used as a basis to improve the process performance. From an environmental engineers point of view, the intention of this paper is to extend applications of PCIs and MPCIs in the field of environmental engineering. Measuring wastewater treatment process performance using capability indices effectively and then process improvement based on these indices is necessary to save the environment and enable reuse of processed water. The wastewater treatment process capability analysis using appropriate capability indices is presented in the paper based on secondary data which is collected from case studies through literature survey. It is found that using appropriate capability indices, wastewater treatment processes performance can be assessed effectively as compared to usual practice.
Keywords Process capability indices; process distribution; process variation; normal distribution; wastewater treatment plant capability; effluent discharged standards; wastewater treatment process; multivariate normal distribution.
-
INTRODUCTION
As compared to developed countries, there is sizeable gap in untreated wastewater and treated wastewater at India level [1]. Untreated wastewater and low quality of treated wastewater contaminate the environment. In view of this issue, there is an urgent need to increase the number of best performing wastewater treatment plants with huge capacity and to improve the performance of existing wastewater treatment plants. Enhancing the quality status of treated wastewater will save the environment and water crisis problem would be solved up to certain level by reusing this water. Process capability analysis using appropriate capability
indices is the base to improve the wastewater treatment process performance. Process capability analysis with capability indices uses statistical tools to know how well a process performs with reference to process characteristics standards or specification limits. Wastewater treatment process capability analysis with capability indices considers the definition of quality which is based on the viewpoint that wastewater treatment process must meet specified requirements regarding effluent discharged set by Central Pollution Control Board (CPCB) [2].
In usual practice performance of wastewater treatment plant is assessed by percentage removal of Chemical Oxygen Demand (COD), Biochemical Oxygen Demand (BOD) and Total Suspended Solids (TSS) from wastewater after processing. Besides these environmental engineer also expects to consider the status of characteristics such as pH, NH4-N, N- Total, fecal coliform etc at outlet while measuring process performance. There are number of research articles where wastewater treatment plant performance is measured through percentage removal of BOD, TSS etc. [3, 4, 5, 6, 7 and 8]. Evaluated performance of wastewater treatment plant with Activated Sludge Method was measured and noted as removal efficiency of BOD is 94.56% and removal efficiency of TSS is 93.72% [3]. Evaluated performance of wastewater treatment plant was measured as COD removal efficiency is 69.39% and BOD removal efficiency is 62.78% [5]. Indian standards with regard to effluent discharged for wastewater treatment plant, in case of characteristic BOD (mg/l) is not more than 10 and for COD (mg/l) is not more than 50 [2]. Formula of removal efficiency of BOD considers BOD at inlet stage and BOD at outlet stage. On similar lines formula of removal efficiency of COD considers COD at inlet stage and COD at outlet stage. These formulae do not consider specification limits for these characteristics while measuring performance of wastewater treatment plant. So the problem with usual practice of performance assessment is that there is no direct comparison between wastewater treatment process characteristics distribution at outlet stage and effluent discharged standards or specification limits developed by CPCB for these characteristics. Considering this lacuna in mind, in this paper we propose and use capability indices which compares the output of wastewater treatment process with the process specifications and quantify how well the process performance.
The study of univariate process capability analysis began as a comparison of the stable process distribution with product specification in the form of lower and upper specification limits. Initially the judgment of process capability was based on histogram which is one of the important statistical process
control tool. Considering the limitations of histogram tool to assess capability, there was quick demand of process capability measure from practitioners. Thus the PCI was born as a measure of process capability. PCIs have been extensively studied in the literature with their estimators, distributional and inferential properties [9, 10]. PCIs have been widely used in evaluating the quality performance of manufacturing processes; they can be effectively used to evaluate environmental performance [11, 12]. In real life situations, many processes are multivariate in nature. Considering the issue of multivariate process in mind, multivariate process capability indices (MPCIs) were developed as measures to measure multivariate process capability during the last three decades [13, 14, 15]. MPCIs are based on multivariate statistical methods and they consider the variables jointly with their correlation structure. PCIs as well as MPCIs were recently reviewed and strengths and weaknesses of each index were discussed[16].
The structure of this article is as follows. In section 2, we
If a tolerance region of quality characteristic is specified by LSL and USL then most frequently used capability index is Cpk , which is defined by min(CPU, CPL).
Capability requirement: A process is called not capable if capability index is less than one; it indicates process is not capable with respect to specifications; in such a situation process standard deviation ( ) needs to be reduced and/or process mean ( ) to be adjusted. A process is called capable
if capability index is greater than or equal to 1. Magnitude of capability index value gives us the answer of question how well the process performance with respect to standards or specificatins. Process performance status is satisfactory if capability index is lies between (1.33, 1.50) and is said to be excellent if it is greater than 1.5.
In practice parameters and are replaced by their
estimators and computed from sample X1, X2 ,…., Xn . That is
n
n
1 n and
discuss basic PCIs and their assumptions with their proposed probability based alternative forms. This section also extends definition of probability based PCIs in multivariate setup.
X
S
Xi i 1
n 1
n 1
1 n X X 2 .
From environmental engineers point of view, section 3 deals with two case studies taken from literature and then wastewater treatment plant capability analysis with quantification of process capability. The last section summarizes the investigation with some concluding remarks.
-
PCIS AND MPCIS TO MEASURE ACTUAL PROCESS PERFORMANCE
-
Univariate traditional PCIs
i
i 1
-
Probability based PCIs to measur process performance
In view of various problems of constructing capability indices for univariate as well as multivariate set up, probability based PCI are very useful as compared to traditional PCIs as well as MPCIs [14]. Assuming assumptions as mentioned in the beginning of section II, we define alternative form of CPU and CPL as given below. These alternative forms are smooth version of CPU and CPL.
PCIs CPU and CPL are defined under the assumptions
CPU
1 1 1 p ;
(3)
that:
-
the process is in statistical control
( p )
3 2
-
a tolerance region of a quality characteristic is
where p PX USL , 2
CPL
1 1 1 p ;
(4)
specified by lower or upper specification limits
( p )
3 2
(LSL or USL) and
-
the process measurement (X) are normally
Here
1
where p PX LSL , 2
denotes the inverse distribution function of the
distributed with mean and variance
2 . The
standard normal distribution. PCIs
CPU
( p)
and
CPL( p)
probability density function of normal distribution having mean and variance 2 is given below.
2
estimated using p by replacing and by their estimators
and .
Remark 1: If a tolerance region of quality characteristic is
1
1
1 x
f (x; , )
e 2
2
x,
,
0
specified by LSL and USL then alternative form of defined as given below.
Cpk is
C 1 1 1 p ;
The Upper (CPU) and Lower (CPL) Capability
pk( p )
3 2
Indices are defined as
For a given USL, the upper capability index is given by
where p PLSL X USL , 2 .
Remark 2: Range of random variable in normal distribution is
to ; and using the property of probability density
function P X 1. But some times for highly
CPU USL
3
(1)
capable processes, due to computational limitation it may happen that p defined in (3), (4) and remark 1 comes out to be 1; in that case take p to be just less than one, say
Similarly for given LSL, the lower capability
index is given by
p=0.99999999.
CPL LSL
3
(2)
7
8
24
32
8
6.5
18.8
10
9
7
15
16
7
8
24
32
8
6.5
18.8
10
9
7
15
16
-
-
Probability based MPCIs to measure process performance
We define multivariate version of
CPU( p) and
CPL( p) as
an alternative to multivariate analogue of CPU and CPL respectively denoting by MCPU( p) and MCPL( p). These MPCIs are defined under the assumption that
-
the process is in statistical control
-
only a single specification limit is given for each quality characteristics
-
denoting the ith (i=1,2,,m) quality characteristics by
-
Capability analysis: While measuring wastewater treatment process capability using PCIs and MPCI here we take following effluent standards for wastewater treatment plant developed by CPCB [2].
Characteristic
Characteristic Limit (Not more than)
BOD (mg/l)
10
COD (mg/l)
50
TSS (mg/l)
20
Characteristic
Characteristic Limit (Not more than)
BOD (mg/l)
10
COD (mg/l)
50
TSS (mg/l)
20
TABLE II. EFFLUENT DISCHARGED STANDARDS FOR WASTEWATER TREATMENT PLANT
X , the process distribution of X X , X ,…, X '
i 1 2 m
is m variate normal with mean vector and variance covariance matrix is . A m-dimensional normal density for the random vector X has the form is given below.
Step 1: Data is tested for outlier using Grubbs Test and following outliers are found at 5% significance level.
f (x)
1
2 m / 2 1/ 2
ex ' 1/ 2 x / 2
Variable
Sample no.
Outlier
BOD
5
11.2
COD
3
112.8
Variable
Sample no.
Outlier
BOD
5
11.2
COD
3
112.8
TABLE III. OUTLIERS USING GRUBBS TEST
xi , i ; (i 1,2,…,m)
and is positive definite matrix.
Alternative multivariate version for given below.
CPU
and
CPL are
So from further analysis sample number 3 and sample number 5 excluded from the data set.
MCPU
1 1 1 p ;
(5)
Step 2: Data is tested for multivariate normality. Based on
( p )
3 2
Roystons H test it is found that data come from a multivariate
MCPL
where p PX USL ,
1 1 1 p ;
(6)
normal distribution cannot be rejected at the 5% significance level because observed level of significance (P-Value) is
( p )
3 2
where p PX LSL ,
0.8822.
From this we conclude that
Here
1
denotes the inverse distribution function of the
multivariate normal distribution and variables BOD, COD and
standard normal distribution. These MPCIs estimated using p by replacing and by their estimators which are sample mean vector and sample variance covariance matrix.
-
-
CASE STUDIES WITH CAPABILITY ANALYSIS The wastewater treatment process capability analysis
presented which is based on secondary data taken from case study 1 [17] and case study 2 [7].
-
Case Study 1
Description with data: This study evaluates the performance efficiency of wastewater plant operating on biological treatment method with an average wastewater inflow of 6 MLD. This plant is located at Sector-8, Charkop, Mumbai, Maharashtra, India. This case study dataset contains nine biweekly (Oct 2018-May 2019) meaurements of BOD in mg/l, COD in mg/l and TSS in mg/l at four stages including inlet stage and outlet stage. For process capability analysis we have considered only outlet stage data. In statistical language, to analyze process capability, number of variables: 3 and number of observation per variable: n=9. Dataset is given in Table 1.
Sample
BOD in mg/l
COD in mg/l
TSS in mg/l
1
6.35
25
40
2
6.8
18.8
20
3
8.09
112.8
40
4
8.1
28.8
25
5
11.2
47
35
6
5
16.1
48
Sample
BOD in mg/l
COD in mg/l
TSS in mg/l
1
6.35
25
40
2
6.8
18.8
20
3
8.09
112.8
40
4
8.1
28.8
25
5
11.2
47
35
6
5
16.1
48
TABLE I. BOD, COD AND TSS AT OUTLET FROM CASE STUDY 1
TSS follows normal distribution.
To get rough idea about process capability with respect to BOD, a visual presentation of distribution of BOD having estimated mean=6.8214 and Standard deviation=1.0566 is shown below in Fig. 1. Here USL for BOD is not more than
10. Almost all observations are below 10.
TABLE IV. SAMPLE MEAN AND STANDARD DEVIATION
Variable
Mean
Standard deviation
BOD
6.8214
1.0566
COD
20.8429
4.9389
TSS
27.2857
13.5242
TABLE V. SAMPLE CORRELATION MATRIX
Variable
BOD
COD
TSS
BOD
1.0
0.6215
-0.4361
COD
0.6215
1.0
0.1717
TSS
-0.4361
0.1717
1.0
Step 3: Probability based PCIs are estimated using (3), estimated values are given below.
TABLE VI. ESTIMATED PROBABILITY BASED PCIS
Variable
p PX USL ,
CPU ( p )
BOD
0.9986866
1.0710
COD
0.99999999
1.9102
TSS
0.2950415
0.1262
Step 4: Probability based MPCI is estimated using (5), estimated value with estimation procedure is given below.
Sequence Batch Reactor (SBR) technology with an average wastewater inflow of 10 MLD (million liters per day). Wastewater treatment plant is located near Gadli village in Kaithal town, Haryana, India. This case study dataset contains four to five observations per month, data was collected during Jan 2014 to April 2014. Data collected on nine Physical and Chemical parameters of plant at inlet stage and outlet stage which includes BOD in mg/l, COD mg/l and TSS in mg/l. For process capability analysis and to compare process capability of this plant with respect to process capability of case study 1 plant, we have considered only outlet stage data of BOD, COD and TSS. So in statistical language, to analyze process capability, number of variables: 3 and number of observations per variable: n=17. Dataset is given in Table 7.
Sampling date
BOD (mg/l)
COD (mg/l)
TSS (mg/l)
03/01/14
06
29.12
07
11/01/14
06
32.12
10
17/01/14
08
29.2
12
24/01/14
07
22.12
08
30/01/14
08
27.12
08
05/02/14
09
36
12
12/02/14
12
37
12
18/02/14
09
38
12
23/02/14
11
40.12
13
28/02/14
10
25
12
06/03/14
08
49
12
10/03/14
06
37.12
13
14/03/14
10
26.0
14
19/03/14
07
32.57
14
24/03/14
09
29.13
15
30/03/14
12
31.12
16
06/04/14
10
48.28
20
Sampling date
BOD (mg/l)
COD (mg/l)
TSS (mg/l)
03/01/14
06
29.12
07
11/01/14
06
32.12
10
17/01/14
08
29.2
12
24/01/14
07
22.12
08
30/01/14
08
27.12
08
05/02/14
09
36
12
12/02/14
12
37
12
18/02/14
09
38
12
23/02/14
11
40.12
13
28/02/14
10
25
12
06/03/14
08
49
12
10/03/14
06
37.12
13
14/03/14
10
26.0
14
19/03/14
07
32.57
14
24/03/14
09
29.13
15
30/03/14
12
31.12
16
06/04/14
10
48.28
20
TABLE VII. BOD, COD AND TSS AT OUTLET FROM CASE STUDY 2
Here, X BOD, COD, TSS' follows multivariate
normal distribution with
6.8214
20.8429 and
27.2857
1.1165
3.2439
6.2321
3.2439
24.3928
11.4690
6.2321 11.4690
182.9047
p PX 10,50, 20' =0.293917. This probability is computed using R software with package mvtnorm.
MCPU
( p)
0.125.
Step 5: In step four we have noted point estimate of MCPU(p), we can estimate lower and upper limit of 95% confidence interval of MCPU(p) using simulation technique. To estimate interval estimate with 95% confidence coefficient, generate 5000 samples from predicted multivariate normal distribution with size 7. From each generated sample estimate p and then MCPU(p) following step 4 and in this way we get 5000 point estimates of MCPU(p) . Compute 2.5th and 97.5th percentile of estimated MCPU(p). The 2.5th and 97.5th percentile of estimated MCPU(p) is the lower and upper bound of confidence interval of MCPU(p).
Following step 5, 95% confidence interval of MCPU(p) is (0.0160, 0.2724).
Interpretation: Here wastewater treatment plant is not capable to meet the effluent discharged standards for wastewater treatment Plant developed by CPCB. Because
Capability analysis:
Step 1: Data is tested for outlier using Grubbs Test and it is found that no outlier at the 5% significance level.
Step 2: Data is tested for multivariate normality. Based on Roystons H test it is found that data come from a multivariate normal distribution cannot be rejected at the 5% significance level because observed level of significance (P-value) is 0.3212.
From this we conclude that X BOD, COD, TSS' follows
multivariate normal distribution and variables BOD, COD and TSS follows normal distribution.
Variable
Mean
Standard deviation
BOD
8.7058
1.9610
COD
33.4718
7.5757
TSS
12.3529
3.1412
Variable
Mean
Standard deviation
BOD
8.7058
1.9610
COD
33.4718
7.5757
TSS
12.3529
3.1412
TABLE VIII. SAMPLE MEAN AND STANDARD DEVIATION
estimated values of MPCI; MCPU ( p) is too less as compare to
desirable value 1. This is also observed through 95% confidence interval of MCPU(p). In view of univariate process capability, process is capable with respect to BOD and COD; but process is not capable with respect to TSS. This statement
Variable
BOD
COD
TSS
BOD
1.0
0.1744
0.5252
COD
0.1744
1.0
0.4854
TSS
0.5252
0.4854
1.0
Variable
BOD
COD
TSS
BOD
1.0
0.1744
0.5252
COD
0.1744
1.0
0.4854
TSS
0.5252
0.4854
1.0
is based on values of CPU
( p )
for these variables. To improve
TABLE IX. SAMPLE CORRELATION MATRIX
process capability we need to improve process capability with respect to variable TSS. Root cause analysis is needed to identify root causes of low capability with respect to variable TSS.
-
Case Study 2
Description with data: This study evaluates the performance efficiency of wastewater plant operating on
Step 3: Probability based PCIs are estimated using (3), estimated values are given below.
TABLE X. ESTIMATED PROBABILITY BASED PCIS
Variable
p PX USL ,
CPU ( p )
BOD
0.7453633
0.3797
COD
0.9854356
0.8143
TSS
0.9925428
0.8919
Step 4: Probability based MPCI is estimated using (5), estimated value with estimation procedure is given below.
Here, X BOD, COD, TSS' follows multivariate normal distribution with
8.7058
33.4717 and
-
-
CONCLUSION
The problem with usual practice of assessment of performance of wastewater treatment plant is that there is no direct comparison between wastewater treatment process distribution at outlet stage and effluent discharged standards or specification limits developed by CPCB. Considering this lacuna in mind, in this paper we have defined and used probability based PCIs and MPCIs which compares the output of wastewater treatment process with the process specifications and quantify how well the process performance. Capability indices have been widely used in evaluating the quality performance of manufacturing processes; it is found
that they can be effectively used to evaluate the performance
12.3529
3.8455
2.5911
3.2352
2.5911
57.3923
11.5537
3.2352
11.5537
9.8676
of wastewater treatment plant. We have studied two wastewater treatment plants through capability analysis and defined probability based PCIs and MPCIs. Both plants processes not capable to meet the standards developed by CPCB. Though both plants are not capable to meet the
p PX 10,50, 20' =0.7357307. This probability is
computed using R software with package mvtnorm.
MCPU ( p) 0.3721.
Step 5: To estimate interval estimate with 95% confidence coefficient, generate 5000 samples from predicted multivariate normal distribution with size 17. Remaining procedure is same as explained in step 5 of case study 1.
95% confidence interval of MCPU(p) is (0.2592, 0.5452)
A visual presentation of histogram of estimator of MCPU(p) is given below in Fig. 2. This will help us if needed to study properties of estimator.
Interpretation: Here wastewater treatment plant is not capable to meet the effluent discharged standards for wastewater treatment plant developed by CPCB because estimated values of MPCI; MCPU ( p) is too less as compare to
desirable value 1. This is also reflected through 95% confidence interval of MCPU(p). In view of univariate process capability, process is not capable with respect to all variables.
standards but wastewater treatment plant located near Gadli village in Kaithal town, Haryana, India is a little bit more capable as compared to other plant which is located at Sector- 8, Charkop, Mumbai, Maharashtra, India because former plant estimated MPCI is large as compared to latter. This is also observed through comparing confidence interval of respective plants MPCIs. To improve capability of these plants, root cause analysis is needed to identify root causes of low capability. Though we have assumed multivariate normal process we can extend definitions of probability based PCIs and MPCIs when distribution of process is not multivariate normal because our definitions base is conforming probability. To get good estimate of PCIs or MPCIs in accuracy and precision point of view, large sample is needed.
REFERENCES
-
Gautam S. K., Sharma D., Tripathi J. K., Ahirwar S. and Singh S. K. A study of the effectiveness of sewage treatment plants in Delhi region, Applied Water Science, vol. 3(1), pp. 5765, 2013
-
CPCB Environmental Information System Newsletter on Control of Pollution, Issue 1, 2015
-
K. S. Kumar, P. S. Kumar and M. J. Ratnakanth Babu Performance evaluation of wastewater treatment plant, International Journal of Engineering Science and Technology, Vol. 2(12), pp. 7785-7796, 2010
-
Coskuner, G. and Ozdemir, N. Perfomance assessment of a wastewater treatment plant treating weak campus wastewater, International Journal of Environment and Pollution, Vol. 28(1/2), pp. 185-197, 2006
-
G. Chandrakant, P. Jaswanth, S.Teja reddy, and G. Kiranmai Design & performance evaluation of wastewater treatment plant-D at Tirumala, International Journal of Scientific & Engineering Research,
Vol. 6(7), pp. 1672-1688, 2015
-
P. N. Wakode, and S. U. Sayyad Performance evaluation of 25MLD sewage treatment plant (STP) at Kalyan, American Journal of Engineering Research, Vol. 3(3), pp. 310-316, 2014
-
A. Pipraiya Performance evaluation of wastewater treatment plant based on SBR technology- A case study of Kaithal town, Haryana (India) International Journal of Innovative Research in Science,
Engineering and Technology, Vol. 6(6), pp. 10690-10695, 2017
-
Jamwal, P., Mittal, A. K. and Mouchel, J. Efficiency evaluation of sewage treatment plants with different technologies in Delhi (India),
This statement is based on values of CPU
( p )
for each variable.
Environmental Monitoring and Assessment, Vol. 153(1-4) , pp. 293-
305, 2009
To improve process capability we need to improve process capability with respect to all variables. Root cause analysis is needed to identify root causes of low capability with respect to all variables.
-
Kotz, S. and Johnson, N. Process Capability Indices, Ist ed., Chapman and Hall, London, 1993.
-
Kotz, S. and Lovelace, C. Process Capability Indices in Theory and Practice, Ist ed., Arnold, London, 1998
-
Corbett, Charles & Pan, Jeh-Nan. Evaluating environmental performance using statistical process control techniques European Journal of Operational Research. Vol. 139(1), pp. 68-83, 2002
-
Jeh-Nan Pan, Chung-I Li and Wei-Chen Shih New multivariate process capability indices for measuring the performance of multivariate processes subject to non-normal distributions, International Journal of Quality & Reliability Management, Vol. 33(1), pp. 42-61, 2016
-
Shinde, R.L. and Khadse, K.G. A review and comparison of some multivariate process capability indices based on fraction conforming interpretation, Statistical Methods, Vol. 7(2), pp. 95-115, 2005
-
Khadse, K. G. and Shinde, R. L. Probability based Process Capability Indices, Communications in Statistics – Simulation and Computation; Vol. 38(4), pp. 884-904, 2009
-
Shinde, R.L. and Khadse, K.G. Multivariate Process Capability using Principal Component Analysis, Quality Reliability Engineering International, Vol. 25(1), pp. 6977, 2009
-
de-Felipe, D. and Benedito, E. A Review of univariate and multivariate process capability indices, Int J Adv Manuf Technol,
Vol. 92(5-8), pp. 1687-1705, 2017
-
Bhave, P.P., Naik, S. & Salunkhe, S.D. Performance Evaluation of Wastewater Treatment Plant, Water Conservation Science and Engineering 2020, in press.