
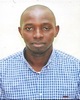
- Open Access
- Authors : Osayoghoghome Peter Aigbogun, Jacob Olaitan Akindapo
- Paper ID : IJERTV11IS010099
- Volume & Issue : Volume 11, Issue 01 (January 2022)
- Published (First Online): 17-02-2022
- ISSN (Online) : 2278-0181
- Publisher Name : IJERT
- License:
This work is licensed under a Creative Commons Attribution 4.0 International License
Comparative Study of Plastic Injection Mould Filling Analysis using ANSYS General-Purpose CFD Software and Specialised Injection Moulding Simulation Software
1Osayoghoghome Peter Aigbogun 1Defence Industries Corporation of Nigeria 45 Ahmadu Bello Way, Kaduna
Nigeria
2Jacob Olaitan Akindapo 2Department of Mechanical Engineering Nigeria Defence Academy, Kaduna
-
2109, Kaduna Nigeria
Abstract: This study involves a comparative analysis of polymer flow in an injection moulded part using a dedicated injection moulding simulation software; Autodesk Moldflow and a general-purpose simulation software; ANSYS, in a bid to establish if a general-purpose software can match results obtained from a niche injection moulding software, thereby alleviating the need for investments in multiple software for different physics. The top cover of an electric socket adaptor was modelled with Creo Parametric and four gate locations were studies to obtain the optimal gate location for the study. Simulation was carried out with Autodesk Moldflow and a comparative study with ANSYS. The polymer, Acrylonitrile Butadiene Styrene (ABS), was modelled in the ANSYS Fluent solver using the Cross Model for a shear-thinning non-Newtonian flow. Flow analysis into the cavity resulted in a fill pressure and velocity of 17 MPa and 0.05576 m/s for Moldflow, 17.17 MPa and 0.0520 m/s for ANSYS. The similarity in results obtained from both software demonstrated that ANSYS, a general-purpose simulation software is a viable alternative to Autodesk Moldflow in the numerical study of plastic injection moulding.
Keywords: ANSYS, Fluent, Computational Fluid Dynamics (CFD), Injection moulding, Creo Parametric.
-
INTRODUCTION
Plastics are versatile engineering materials consisting of wide range of synthetic and semi-synthetic organic material and can be moulded into solid objects. They find applications in consumer and industrial products such as food and beverage containers, packaging, housewares, housing for computers and monitors, medical devices, foams, paints, safety shields, toys, appliances, lenses, gears, electronic and electrical products, and automobile bodies and components [1].
Plastics finds its widest application in the packaging industry, especially in products like containers, bottles, drums, trays, boxes, cups, baby products, and protective packaging [2].
Plastic processing requires special tooling such that the design and production of plastic injection moulding tools is a complex undertaking involving many critical parameters such as; flow rate, wall thickness, force, warpage and sink marks, structural integrity, temperature distribution, cooling effect, gate locations, and runner system, that must be accurately factored. The cost of raw materials for plastic mould making deters young engineers from undertaking design and production of required moulds as errors can become very expensive to fix. Plastic processing tool are made from high grade tool steel known as P20 and the cost of the tools required is typically high. It is expensive to try to fix problems or optimise the design once a trial is underway [3].
Computer Aided Engineering (CAE) involves the use of computer methods to support basic error checking, analysis, optimisation, and manufacturability of a product design [4]. With CAE, performance can be simulated in order to improve product designs or assist in the resolution of engineering problems for a wide range of industries. Applied to plastic processing; optimal parameters and mould configuration can be determined in a cost-effective manner as the benefits of CAE include reduced product development cost and time, with improved product quality and durability. Applying Computer Aided Engineering, designs can be evaluated and refined using computer simulations rather than physical prototype testing, thereby saving money and time [5]. Plastic processing is facilitated by the application of heat and pressure [6], therefore the relationship between temperature and pressure has to be controlled for part quality. The temperature and pressure are kept within the injection moulding process window for an optimised production. Figure 1 depicts the process window where insufficient temperature and pressure results in short-shot, therefore the temperature should be sufficiently high to melt the polymer but not too high to result in thermal degradation. On the other hand, too high a pressure would result in flash formation. The process window is bounded by these four defects as shown.
Figure 1: Plastic Injection Moulding Process Window [7]
This study attempts to provide a cost-effective means of validating plastic processing tool design by applying Computer Aided Engineering (CAE) in simulating the mould filling process, identify quality issues before building the plastic moulding tool. The primary goal of the study is to determine if ANSYS, a general-purpose simulation software can match results obtained from Autodesk Moldflow which is a specialised injection moulding simulation software. The injection pressure would be the specific parameter to compare as the required injection pressure is a major indicator of the ability to successfully mould a part in a given injection moulding machine.
An optimal injection location would initially be selected based on criteria that contribute to the ease of manufacture, part quality, and minimised visual defects. The comparative study would be carried out based on the selected injection location.
-
REVIEW OF RELATED WORKS
Stanek et al. (2011) worked on the optimisation of injection moulding process to determine ideal processing conditions with keen interest in the dimensions, shapes, and properties of the parts produced. Moldflow Plastics Xpert (MPX) was utilised for the analysis and optimisation. The MPX system was able to achieve effective optimisation of the injection process with optimum process parameters resulting in elimination of possible product defects. Besides correct process conditions setting and elimination of defects during production, the optimisation process also accomplished reduction of the total cycle time [8].
Tie et al. (2012) worked on stresses and strain of the cavity during the injection moulding to ensure the cavity have sufficient stiffness and intensity to withstand the pressure of high-temperature plastic melt. Autodesk Moldflow was used to simulate the flow process parameters to obtain the maximum pressure in the cavity which serves as input parameter for the stiffness analysis. ANSYS Workbench was used to simulate the forces and determine the extent of deformation of the cavity. The study ensured that the elastic deformation of the cavity was not sufficient enough to distort the size or shape of the moulded part outside its tolerances as external forces were controlled not to exceed allowable stress [9].
Hussin et al. (2012) worked on the optimisation of plastic injection moulding parameters using Taguchi optimisation method. The focus of the study was to minimise warpage on Polycarbonate/Acrylonitrile Butadiene Styrene (PC/ABS) shine guard injection moulding. Simulation of the process was carried out using Moldflow Insight software. The study was based on Taguchis Method and Analysis of Variance (ANOVA) to analyse and optimise processing parameters such as mould and melt temperature, packing time and pressure, cooling time and temperature, ambient temperature, and runner size. Twenty-seven experiments were carried out according to the Design of Experiment (DOE) and results obtained indicated that melt temperature was the most significant parameter ffecting warpage. The study resulted in recommended settings for all parameters of interest [10].
Meiabadi et al. (2013) worked on optimisation of plastic injection process by combining Artificial Neural Network (ANN) and Genetic Algorithm (GA) [11-13]. The goal of the study was to obtain process parameters to ensure accurate part weight, minimise cycle time, and injection pressure. The most critical control variables to achieved specified injection goals were determined by statistical design of experiments (DOE) feature of Moldflow software. Findings from the study indicated that the approach can effectively help engineers determine optimal process parameters setting and achieve competitive advantages of product quality and cost. Result was verified by confirming with experimental fabrication [13].
Babu et al (2016) worked on design and simulation of plastic injection moulding process using ANSYS. Two parts were modelled in Siemens NX 8.0 and a single mould base with interchangeable cavity inserts were designed. The flow analysis and simulation
were carried out in ANSYS 15.0. The analysis resulted in the most suitable injection location, flow temperature, and injection pressure being established [14].
Sreedharan et al (2018) worked on the perdition of moulding defects and achieving optimised process settings by applying Response Surface Methodology (RSM). Moldflow was used for flow simulation to ascertain possible moulding defects and initial process parameters. RSM helped in identifying relationships between various process parameters and performance. Four process parameters; injection time, holding time, filling time, and mould closing time, were considered for the study with the output being the cycle time. Regression analysis was carried out to construct a quadratic model for prediction of cycle time within an accuracy of 96.88%. Results obtained from the RSM analysis was evaluated through Analysis of Variance (ANOVA) [15] table to determine an optimal cycle time that reduces or eliminates the shrinkage and warpage, based on varying the four selected process parameters [16].
From reviewed literature thus far, multiple tools were leveraged to study and optimise plastic injection flow. A consolidated simulation tool would be beneficial to a developing economy like Nigeria with reduced software licensing, support, and training cost.
-
MATERIALS, EQUIPMENT AND METHODS
-
Materials
The following materials were used for this study;
Software: Creo Parametric 7.0, Autodesk Moldflow 2019, and ANSYS 2020 R2
Measuring Instruments: Digital Calliper and Radius Template
Raw Material: Acrylonitrile Butadiene Styrene (ABS)
-
Equipment
Mobile Workstation: Lenovo ThinkPad P1 Gen 2 (Intel Core i7, 32GB Memory, Nvidia Quadro T1000).
-
Methods
-
Creating Three-Dimensional Model of Part
Simulation study begins with a CAD model of the part. PTC Creo Parametric 7.0 was used to develop the model of an electric socket adaptor top cover. The 3D model captured the details of the part such as openings for plugs and indication LED, screw bosses, and ribs as shown in Figure 2. From the figure, the part has an overall length of 66 mm, width of 48 mm, height of 15 mm, and the wall thickness being 2 mm.
Figure 2: Three-Dimensional Model of Electric Socket Adaptor
-
Flow Analysis using Autodesk Moldflow
An Autodesk Moldflow Adviser 2019 study was carried out to determine the optimal gate location for the production of the part. Then subsequent studies were carried out using the determined gate location.
-
Determination of Optimal Injection Location
Moldflow 2019 reads Creo Parametric files up to version 5.0, hence the 3D model created in Creo 7.0 had to be converted to a STEP file [17] prior to importation into Moldflow. STEP file was chosen over IGES as it offers extensive 3D data and part geometry [18]. Moldflow carries out an Automatic clean up to fix any defects in the imported model. Upon importing into Moldflow Adviser, the orientation was adjusted such that the parting plane is on the XY plane with the Z axis representing the height as shown in Figure 3.
Four studies were created to represent the different injection locations; a) Inner Edge, b) Top, c) Outer Centre, and d) Inner Centre as shown in Figure 4. Injection location is typically chosen to allow the melt transvers from the gate to the edges of the cavity simultaneously to achieve an even fill [19]. In theory, the top gate, centralised at the middle of the moulding should result in an even fill and requiring minimal injection pressure as the flow length is about half the other locations. The down side of this position is an aesthetic defect, an obvious witness mark at the top of the moulding upon ejection of the part. Having the gate centralised along the parting line represents the other alternative to achieving even filling of the cavity, albeit with a longer flow length resulting in higher injection pressure. The Outer Centre position moves the witness mark to a less obvious position. The Inner Centre position further enhances the visual appeal by effectively hiding the witness mark. The fourth position, Inner Edge, acts as a counter-intuitive control position that is obviously expected to result in an uneven fill but may reveal other benefits from the simulation study.
Generic ABS was chosen as the material with a melt temperature of 230 oC being the mid-point of the recommended temperature, 190 oC 270 oC, for ABS [20], injection velocity and cooling time were set to automatic, mould temperature set to 60 oC [20]. Machine pressure was set to 140 MPa which is a conservative value for most injection moulding machines [19]. As a thin-wall moulding, Dual-domain mesh was chosen. A fill analysis was then carried out for each injection location. Results were compared to ascertain the effect of injection location on fill time, injection pressure, weld lines, quality prediction, temperature at flow front, and confidence of fill to determine the optimal injection location.
Figure 3: Imported Model in Moldflow Adviser Showing Desired Orientation
-
In-depth Fill Analysis
Figure 4: Injection locations under study
Having obtained an optimal injection location, an in-depth fill study was carried out in Moldflow Insight 2019. The CAD model was imported and the orientation set to the desired mould opening direction. The model was then meshed as a dual-domain mesh with a minimum mesh length of 0.5 mm chosen, this translated to 51,600 triangular elements. Moldflow Insight suggest a
minimum mesh length of 0.79 mm, this was reduced to 0.5 mm for more finer mesh which is expected to translate to more accurate result [21]. A second study was carried out with a more refined mesh of length 0.25 mm, resulting in 144,058 elements, to ascertain the effect of mesh refinement on the study. Analysis was carried out on both studies after specifying process parameters similar to that of the injection location study. As a dedicated injection moulding software, Moldflow Insight provides sensible defaults for most of the meshing parameters.
-
-
Flow Analysis using ANSYS
-
ANSYS 2020 R2 was utilised for the study. Unlike in Moldflow where the CAD file was converted to a STEP file, ANSYS 2020 R2 read the Creo 7.0 file directly. However, the gate at the chosen location had to be modelled in Creo prior to importation, whereas for Moldflow study, modelling the gate involved a single click on the model within Moldflow interface.
An ANSYS Workbench project was created with Fluid Flow (Fluent) as the study, the CAD geometry was imported into ANSYS SpaceClaim as shown in Figure 5. ANSYS Mechanical was launched to carryout meshing. Within ANSYS Mechanical, Named Selections were created to serve as labels for the boundary conditions in the Fluent Solver, these were; inlet, wall, flow domain, and outlet. For better reslts with curved surface, the sizing section of the mesh detail was set to capture curvature and proximity. Further sizing settings for the mesh resulted in 192,335 elements
ANSYS Fluent was launched to setup the mathematical model for the study. The flow type was set to laminar and a custom material defined by specifying the density of ABS melt and its rheological properties according to the Cross Model. The specified properties were zero-shear viscosity, power law index, and time constant.
Figure 5: CAD Geometry in ANSYS SpaceClaim
The ANSYS 2020 documentation [22] provides the Cross Model as shown in equation 1:
= 0
1+( )1
(1)
Where:
= Flow viscosity
0 = Zero-shear-rate viscosity
= natural time (inverse of the shear rate at which the fluid changes from Newton to power- law behaviour)
n = power-law index
= Shear rate
)
)
The zero-shear viscosity, 0, is given by equation 2 [19]:
= D exp[ 1( ] (2)
0 1
where:
2+( )
T is the melt temperature (k)
T* is the glass transition temperature determined by curve fitting
A2 = A3 + D3P (3)
P is the pressure (Pa)
The model parameters D1, A1, A3, and D3 are data-fitted coefficients obtained by curve fitting experimental shear- viscosity data taken by a capillary rheometer at shear rates from 1 to 10,000 s-1 [19].
The glass transition temperature is given by equation 4 [19]:
T* = D2 + D3P (4)
Where D2 is a data-fitted coefficient.
The value of the data-fitted coefficients was obtained from the rheological properties of ABS as provided by Autodesk Moldflow Plastics Labs as shown in Figure 6.
Comparing ANSYS Cross Model to the CROSS-WLF Model [19, 23, 24], the time natural time, , is obtained from equation 5:
= 0
(5)
Boundary Conditions specified were inlet mass flow of 0.0144 Kg/s to match the flow rate for the Moldflow study, outlet gauge pressure 0.0, and No slip shear condition for wall. Convergence criteria was set to 1e-6, standard initialization for inlet boundary condition was selected and number of iterations set to 1,500. On running the calculation, the setup converged after 55 iterations. The result from the study was plotted with ANSYS post-processor; CFD-Post.
Figure 6: Rheological Properties of ABS (Autodesk Moldflow Plastics Labs)
The mesh was further refined and the study repeated to determine the effect of discretisation error on the parameters of interest. The refinement resulted in 446,592 elements and all 1,500 iterations ran without convergence.
-
-
RESULTS, ANALYSIS, AND DISCUSSIONS
-
Result and Analysis of Injection Location Study
Four gate locations were chosen for this study, they are; Inner edge, Top, Outer centre, and Inner centre. The results of the different gate locations on selected parameters are presented in Table 1.
Table 1: Effect of Injection Location on Process Parameters
A
Inner edge
B
Top
C
Outer centre
D
Inner centre
Fill time
0.7544 s
0.5263 s
0.6419 s
0.6363 s
Injection Pressure
26.75 MPa
14.33 MPa
24.01 MPa
19.12 MPa
Confidence of fill
100%
100%
100%
100%
Quality prediction
High: 86.2%
Mid: 13.8%
Low: 0%
High: 84.4%
Mid: 14.6%
Low: 0%
High: 86.2%
Mid: 13.9%
Low: 0.04%
High: 90.4%
Mid: 9.59%
Low: 0%
Weld lines
135o 5.268o
135o 8.013o
135o 2.494o
135o 26.68o
Difference in temperature at flow front
-7.1 oC
-12.4 oC
-5.2 oC
-5.8 oC
Table 2 is a ranking matrix to determine the optimal gate location. In the ranking matrix, numbers 1 to 4 represent the rank of a particular gate location in a specific result, with 4 being the best and 1 the least.
Table 2: Injection Location Ranking Matrix
A
Inner edge
B
Top
C
Outer centre
D
Inner centre
Fill time
1
4
2
3
Injection Pressure
1
4
2
3
Confidence of fill
4
4
4
4
Quality prediction
3
1
2
4
Weld lines
3
2
4
1
Difference in temperature at flow front
2
1
4
3
TOTAL SCORE
14
16
18
18
The selected results for comparison were those related to productivity, and defects of concern such as short shot and structural integrity, hence they can be considered to be of equal importance, therefore not requiring some form of weighting.
As observed from the ranking matrix, the inner centre gate and the outer centre gate are the highest-ranking positions with a sum of 18 each.
The inner centre gate has the advantage of leaving no visible mark in the moulding as the injection point is internal to the product, however, it would be difficult to design and machine as it may require a tunnelled runner system. The outer centre gate would be much easier to design and manufacture and this is the location of choice for this study.
-
Result of In-depth flow Study with Moldflow Insight
Moldflow insight study was carried out with a coarse and more refined mesh to obtain more granular information about the flow within the cavity and also ascertain the effect of mesh refinement on the accuracy of the solution. Figure 7 and Figure 8 show the results for the initial and refined mesh respectively for fill time, injection pressure, average velocity at sampled point, and pressure at end of fill. For the initial mesh; A) fill time, B) injection pressure, C) average velocity at sampled point and D) pressure at the end of fill were 0.6370s, 21.74MPa, 4.119cm/s and 17.39MPa respectively. While for the refined mesh; 0.6375s, 21.25MPa, 5.576cm/s, and 17.00MPa. Demonstrating that with Autodesk Moldflow, mesh refinement has very minimal effect on the result while considerable increasing the computational time.
Figure 7: Fill time, Pressure, Pressure at end of fill, and Average velocity with initial mesh (Moldflow Insight)
Figure 8: Fill time, Pressure, Pressure at end of fill, and Average velocity with refined mesh (Moldflow Insight)
-
Result of Flow Study with ANSYS Fluent
The ANSYS Cross viscosity model was used for this study, required parameters; zero-shear-rate viscosity, 0, and natural time, , had to be computed and input into ANSYS so the viscosity of the polymer flow can be obtained.
These values were obtained from the computation as presented in
Table 3. The values were used to define the properties of the fluid in the ANSYS Fluent solver and the results obtained are presented in Figure 9 to Figure 14 for the initial and refined mesh.
Table 3: Determination of ANSYS Cross Model Parameters
(i) Determination of ABS Cross Model Coefficient A2
Data
Calculations
Resuts
A3 = 51.6 K
D3 = 1.2 x 10-7 K/Pa P = 101325 Pa
Where, A3 and D3 are data fitting coefficients for ABS (Figure 6), P is atmospheric pressure
From equation (3):
A2 = A3 + D3P
A2 = 51.6 + (1.2 x10-7 x 101325)
= 51.6 K
A2 = 51.6 K
(ii) Determination of glass transition temperature, T*, for ABS
D2 =373.15 K
D3 = 1.2 x 10-7 K/Pa P = 101325 Pa
Where, D2 and D3 are data fitting coefficients for ABS
From equation (4)
T* = D2 + D3P
T* = 373.15 + (1.2×10-7 x 101325)
= 373 K
T* = 373 K
(iii) Determination of Zero-shear-viscosity, 0, of ABS at process condition
D1= 2.81579 x 1012 Pa-s
A1 = 27.763 A2 = 51.6 K T = 503.15 K T* = 373 K
Where, T is the melt temperature.
From equation (2)
)
= D exp[ 1( ]
0 1 2+( )
= 2.81579 × 1012 [ 27.763(503.15373.16) ] = 6592.768
0 51.61+ (503.15373.16)
Pa-s
0 = 6592.768 Pa-s
(iv) Determination of natural time, .
0 = 6592.768 Pa-s * = 15701.5 Pa
From equation (5)
= 0
= 6593.768 = 0.42 S
15701.5
= 0.42 S
Figure 9: Pressure Contour from ANSYS Showing Pressure at the End of Filling for Coarse Mesh
Figure 10: Pressure Contour from ANSYS Showing Pressure at the End of Filling for Refined Mesh
Figure 11: Velocity Streamline from ANSYS for Predicting Weld lines for Coarse Mesh
Figure 12: Velocity Streamline from ANSYS for Predicting Weld lines for Refined Mesh
Figure 13: Velocity probe indication the velocity value (0.0438561 m/s) at the sampled point for Coarse Mesh
Figure 14: Velocity probe indicating the velocity value (0.519971 m/s) at the sampled point for Refined Mesh
-
Comparison of Results Obtained from Moldflow, ANSYS, and Analytical Study
The results for the flow analysis with Moldflow for the initial mesh (Figure 7) and refined mesh (Figure 8) as well as for ANSYS Fluent (Figure 9 to Figure 14) are presented in
Table 4.
Table 4: Comparison of results and effect of mesh refinement on process parameters with numerical studies
Moldflow Insight 2
019
ANSYS Fluent
2020 R2
Initial Mesh
Finer Mesh
Coarse Mesh
Refined Mesh
Fill time
0.6537 s
0.6370 s
N/A
N/A
Pressure
21.74 MPa
21.25 MPa
16.66 MPa
17.17 MPa
Pressure at end of fill
17.39 MPa
17 MPa
16.66 MPa
17.17 MPa
Average Velocity at specific point
0.04119 m/s
0.05576 m/s
0.0439 m/s
0.0520 m/s
ANSYS Fluent did not readily provide results for fill time. The pressure value of interest was the injection pressure or pressure at end of fill where mesh refinement brought the values of the Moldflow and ANSYS study closer at 17 MPa for Moldflow and
17.17 MPa for ANSYS. The average velocity sampled at approximately the same point on the face of the moulding also revealed close velocities for Moldflow and ANSYS, with the mesh refinement resulting in a velocity of 0.05575 m/s for Moldflow and 0.0520 m/s for ANSYS.
4.5 Discussion of Flow Analysis Results
This study looked at flow analysis of plastic injection moulding process with an interest in investigating if ANSYS as a widely used general purpose computer aided engineering software can match results obtained from specialised injection moulding software such as Autodesk Moldflow. This was in a bid to make plastic injection moulding simulation more accessible rather than relying on niche software.
From results obtained, it was evident that ANSYS Fluent did provide near numerical solutions for pressure and average velocity to those obtained from Moldflow as presented in
Table 4.
However, Autodesk Moldflow and ANSYS Fluent provided varying degree of ease of use as well as fidelity and range of possible results and studies.
Autodesk Moldflow being a specialised plastic processing software has the advantage of ease of use and a high degree of accuracy as it has been optimised from the study of plastic processing only. Moldflow 2019 has over 10,000 different plastics predefined with complete rheological properties so that the engineer can focus on the study even with little knowledge of the physics of the material as it relates to plastic injection moulding.
ANSYS being a general-purpose software has the advantage of having a vast range of engineering applications. However, its application in plastic injection moulding, as with its application in any other field, requires a proper understanding of the physics relating to injection moulding. Hence, the success of the study is strongly tied to the accurate modelling of the material. For instance, in this study, the relationship between the viscosity and shear rate of the material had to be accurately defined according to the Cross Model. As a result, ANSYS proved to be accurate in determining the pressure and velocity; two key parameters in injection moulding, when compared with Moldflow study. In terms of result presentation, while Moldflow provides injection moulding specified results, such as weld lines prediction, one would have to rely or on the velocity stream and velocity vector plot to predict weld lines in ANSYS. The meshing process in ANSYS was also more involved and the quality and method of meshing has significant influence on the fidelity of the results obtained.
-
-
CONCLUSIONS
This study successfully carried out flow analysis of a plastic injection moulding process by applying Computer Aided Engineering. The research work demonstrated how ANSYS; a CAE software already familiar in the engineering community, can successfully validate injection moulding process parameters.
The following conclusions were drawn from the study:
-
Comparison of numerical results obtained from Autodesk Moldflow and ANSYS revealed near identical results. Flow analysis into the cavity obtained a 1% and 6.7% difference in results for injection pressure and velocity respectively for Moldflow and ANSYS. In light of these results, ANSYS; a general-purpose numerical software, is a viable alternative to Autodesk Moldflow for injection moulding simulation.
-
In choosing a CAE system, if injection moulding is the focus of the production endeavour, Autodesk Moldflow would be the preferred choice with the benefits of all the plastic processing optimisation built into the software. However, if the endeavour involves multiple engineering or manufacturing processes, ANSYS would be a better choice with the advantage of being a consolidated tool that can be used for various engineering simulations.
-
-
RECOMMENDATIONS The following recommendations are made for further studies:
-
-
A heat transfer analysis can be undertaken in ANSYS to study the cooling system in plastic injection moulding process.
-
A multiphase volume of fluid (VOF) study could be undertaken in ANSYS Fluent to capture the time taken for the displacement of air by the flowing polymer melt in the cavity.
REFERENCES
-
Kalpakjian, S. and Schmid, S. R (2009), "Manufacturing Engineering and Technology", 6th ed. Prentice Hall, Singapore.
-
Yang, Y., Chen, X., Ningyun, L., and Furong , G (201), "Injection Molding Process Control, Monitoring, and Optimization". Hanser Publications, Munich.
-
Marquez, C., Cano, S., and Rodriguez, O (2019), "CFD for Injection Molding," Ansys Advantage, no. 2, p. 27.
-
Rao, P. N (2013), "CAD/CAM Principles and Applications". McGraw Hills Education (India), New Delhi.
-
Siemens "Computer-Aided Engineering." [Online]. Available: https://www.plm.automation.siemens.com/global/en/our-story/glossary/computer- aided-engineering-cae/13112 (accessed 25 January 2020).
-
Riegel, E. R (1974), "Riegel's Handbook of Industrial Chemistry", 7th ed. Van Nostrand Reinhold, New York.
-
Hart, J, (2021) "Design and Manufacturing II," Massachusetts Institute of Technology, Lecture Note.
-
Stanek, M., Manas, D., Manas, M., and Suba, O (2011), "Optimization of Injection Molding Process," International Journal of Mathematics and Computers in Simulation, vol. 5, no. 5, pp. 413-421.
-
Tie, G., Wei-qing, T. U., and Dan-dan, L (2012), "Injection Mold Cavity Stiffness and Intensity Analysis Based on Ansys Workbench and Moldflow," in 2nd International Conference on Electronic & Mechanical Engineering and Information Technology, Shenyang.
-
Hussin, R., Saad, R. M., Hussin, R., and Dawi, M. S. I. M (2012), "An Optimization of Plastic Injection Molding Parameters Using Taguchi Optimization Method," Asian Transactions on Engineering, vol. 02, no. 05, pp. 75-80.
-
Chauhan, N. S (2019), "Introduction to Artificial Neural Networks (ANN)." [Online]. Available: https://towardsdatascience.com/introduction-to- artificial-neural-networks-ann-1aea15775ef9 (accessed 4 March 2020).
-
Mallawaarachchi, V (2017), "Introduction to Genetic Algorithms Including Example Code." Towards Data Science. [Online]. Available: https://towardsdatascience.com/introduction-to-genetic-algorithms-including-example-code-e396e98d8bf3 (accessed 4 March 2020).
-
Meiabadi, M. S., Vafaeesefat, A., and Sharifi, F (2013), "Optimization of Plastic Injection Molding Process by Combination of Artificial Neural Network and Genetic Algorithm," Journal of Optimiation in Industrial Engineering, vol. 13, pp. 49-54.
-
Babu, K. S., Basha, M., and Sreedhar, C (2016), "Design and Simulation of Plastic Injection Molding Process by Using ANSYS," Anveshana's International Journal of Research in Engineering and Applied Sciences, vol. 1, no. 11, pp. 100-110.
-
Sullivan, L, "Hypothesis Testing – Analysis of Variance (ANOVA)." [Online]. Available: http://sphweb.bumc.bu.edu/otlt/MPH- Modules/BS/BS704_HypothesisTesting-ANOVA/BS704_HypothesisTesting-Anova_print.html (accessed 16 March 2020).
-
Sreedharan, J. and Jeevanantham, A. K (2018), "Optimization of Plastics Injection Molding Process Using Response Surface Methodology,"
International Journal of Engineering and Technology, vol. 10, no. 1.
-
Sivakumar, S. and Dhanalakshmi, V (2013), "An approach towards the integration of CAD/CAM/CAI through STEP file using feature extraction for cylindrical parts," International Journal of Computer Integrated Manufacturing, vol. 26, no. 6, pp. 561-570.
-
Rosenberger, B (2019), "IGES vs STEP." [Online]. Available: https://randrmanufacturing.com/blog/iges-vs-step/ (accessed 29 August 2021).
-
Kazmer, D. O (2016), "Injection Mold Design Engineering 2nd Edition". Hanser Publications, Cincinnati.
-
PlastikCity "Material Melt and Mould Temperature Chart." [Online]. Available: https://www.plastikcity.co.uk/useful-stuff/material-melt-mould- temperatures (accessed 16 October 2020).
-
Tang, S. H., Kong, Y. M., Sapuan, S. M., Samin, R., and Sulaiman, S (2006), "Design and thermal analysis of plastic injection mould," Journal of Materials Processing Technology, vol. 171, no. 2, pp. 259-267.
-
Ansys Corporation Ansys Help 2020 R2, 2020. Accessed on: 18 December 2020.
-
Autodesk Moldflow Insight (2019), "Cross-WLF viscosity model." [Online]. Available: https://help.autodesk.com/view/MFIA/2019/ENU/?guid=GUID-7BC3A8F0-8B41-4FCB-BDF1-F1159E4DD175 (accessed 13 July 2021).
-
Cross, M. M (1979), "Relation between viscoelasticity and shear-thinning behaviour in liquids," Rheologica Acta, vol. 18, pp. 609614.