
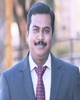
- Open Access
- Authors : Sharan Kumar Paratala Rajagopal
- Paper ID : IJERTV9IS070424
- Volume & Issue : Volume 09, Issue 07 (July 2020)
- Published (First Online): 23-07-2020
- ISSN (Online) : 2278-0181
- Publisher Name : IJERT
- License:
This work is licensed under a Creative Commons Attribution 4.0 International License
Data Visualization for Vehicle Selection Process
Sharan Kumar Paratala Rajagopal
IEEE Member
Senior Manager, Capgemini America Inc.
Dallas, TX, USA
Abstract – Data visualization makes big or small data easier to interpret for human brain to understand and it will help in determining patterns, trends and outliers in groups of data. Color selection in presenting the data visually plays a vital role. With the help of advanced data visualization tools such as tableau we are going to interpret the best car from the data set with average weighted score calculated which helps us to identify the best car that can be added to the fleet of cars.
Keywords – Data visualization, Data Analytics, Tableau, Data analysis.
-
INTRODUCTION
The concept of using pictures to understand data has been around for centuries, from maps and graphs in the 17th century to the invention of the pie chart in the early 1800s. Due to the evolution of technology and cloud based solutions there is significant amount of data gathered across and interpreting them and converting to human readable format is plays vital role in key decision making process. Computers with latest technologies and softwares helps to process large chunks of data at lightning fast speeds. Data visualization is rapidly evolving and its an art that is certain to change the corporate world over the next few years.
-
DATA VISUALIZATION FACTS AND IMPORTANCE
Data visualization is taking information or data in the required format and presenting into visual context by creating maps and graphs or its a story telling process which grabs the targeted audience attention and conveys the message clearly by presenting the data in patterns and colors which is easily decoded by the human brain.
It also presents the areas that need immediate attention or improvements by using color coding or patterns. It highlights which factors can influence or predict customer behavior. Helps to understand product placement and forecast or predict sales volumes. There are multiple areas where data can be used to increase the productivity.
-
DATA VISUALIZATION TYPES
The most frequently used data presenting types will be bar graph or pie charts. This is most integral part of visualizing data and most common baseline for many graphical data representation. There is much more types which can be used by pairing to present the right set of data in the right format. Some of the examples include and not limited to
-
Charts
-
Tables
-
Maps
-
Dashboards
-
Pie chart
-
Bar chart
-
Levelled bar chart
-
Stacked bar chart
We will use some of them to interpret the data which is use for this research of car selection process.
-
-
SELECTION OF CAR USING DATA VISUALIZATION AND WEIGHTED AVERAGE SCORE
METHOD
Business needs to choose a car in order to add to their fleet of cars based on certain criteria by calculating its weighted average score by defining criteria for selection process. And once the score is calculated it has to be presented with user readable format and create a dashboard to differentiate different cars based on the defined criteria and make a final decision on which car is best suited for the current need.
There are multiple criteria which will be used in this paper. The first criteria is defined by the company and second criteria is determined by the researcher.
Criteria 1 (Company Criteria): Safety features weighted at 10 Maintenance cost weighted at 5 Price point weighted at 7
Criteria 2 (Researcher Criteria): Insurance weighted at 10 Fuel Economy weighted at 5 Resale Value weighted at 7
Lets look at an example by choosing the category values for each car brand from the websites [1], [2].
Fig. 1 provides the assumed weightage in order to score each car model for defined criteria 1&2.
Fig.1 Assumed weightage for Criteria 1&2.
Fig.2 is calculated by using the defined criteria 1 and calculating the score for each car brand by category using range multiplied by weight to get the score and then sum up score of each car brand.
Fig.2 Calculated score based for criteria 1
Fig.3 gives the final calculated weighted score by summing up the scores for each category by car brand. The highlighted record shows the car model with highest weighted score for criteria 1.
Fig.3 Weighted score for Criteria 1
Fig.4 is calculated by using the defined criteria 2 and calculating the score for each car brand by category using range multiplied by weight to get the score and then sum up score of each car brand.
Fig.4 Calculated score based for criteria 2
Fig.5 gives the final calculated weighted score by summing up the scores for each category by car brand. The highlighted record shows the car model with highest weighted score for criteria 2.
Fig.5 Weighted score for Criteria 2
Now we have the calculated data ready and this can be represented in the data visualization types to see how this is easy to interpret by using different visual elements.
Fig.6 provides the Levelled bar chart for criteria 1 calculated score from Fig.2 using tableau.
Fig.6 Levelled bar chart for criteria 1
Fig.7 provides the Stacked bar chart for criteria 1 calculated score from Fig.2 using tableau.
Fig.7 Stacked bar chart for criteria 1
Fig.8 provides the Multiple pie chart for criteria 1 calculated score from Fig.2 using tableau.
Fig.8 Multiple pie chart
Fig.9 provides the Levelled bar chart for criteria 2 calculated score from Fig.4 using tableau.
Fig.9 Levelled bar chart for criteria 2
Fig.10 provides the Stacked bar chart for criteria 2 calculated score from Fig.4 using tableau.
Fig.10 Stacked bar chart for criteria 2
Fig.11 provides the Multiple pie chart for criteria 2 calculated score from Fig.4 using tableau.
Fig.11 Multiple pie chart for criteria 2
The data representation by individual charts gives the information regarding different scores for each category. Now lets see by combining data visualization for both the criterias using pie chart.
Fig.12 represents the data in Pie chart format for both criteria 1 and 2. Data from Fig.2 and Fig.4 set using Tableau.
Fig.12 Pie chart for criteria 1&2
Fig.13 represents the data in Stacked bar chart format for both criteria 1 and 2. Data from Fig.2 and Fig.4 set using Tableau.
Fig.13 Stacked bar chart for criteria 1&2
Fig.14 represents the data in Levelled bar chart format for both criteria 1 and 2. Data from Fig.2 and Fig.4 set using Tableau.
Fig.14 Levelled bar chart for criteria 1&2
Fig.15 represents the data in dashboard by using data from Fig.2 and Fig.4 set using Tableau. Dashboard provides holistic view of data and user can view in a better way to make decision wisely.
-
DECISION MAKING USING DATA VISUALIZATION
Based on the presented information, decision making is rapid and easier by seeing different segment values on dash board for each category and brand of car without going through each and every data point. This helps in saving time and making decision quickly and easily.
-
DECISION MAKING USING DATA VISUALIZATION
Data representation is achieved by using different visual elements and types of charts to represent the calculated data and helps in solving the use case for selection of car.
After considering calculated weightage score dat and visual data, 2017 Honda Toyota Rav4 provides enough information to convince the person to choose this car for their fleet.
-
RESULT AND DISCUSSION
Data representation is achieved by using different visual elements and types of charts to represent the calculated data and helps in solving the use case for selection of car.
After considering calculated weightage score data and visual data, 2017 Honda Toyota Rav4 provides enough information to convince the person to choose this car for their fleet.
Fig.15 Dashboard for criteria 1&2
REFERENCES
-
Sample Car Data obtained from Kelly Blue Book. Available online at https://www.kbb.com
-
Different insurance data for each car model obtained from Cars.com. Available online at https://www.cars.com