
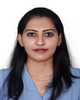
- Open Access
- Authors : Anju M Nair , Dr. James K C
- Paper ID : IJERTV11IS060231
- Volume & Issue : Volume 11, Issue 06 (June 2022)
- Published (First Online): 27-06-2022
- ISSN (Online) : 2278-0181
- Publisher Name : IJERT
- License:
This work is licensed under a Creative Commons Attribution 4.0 International License
Developing Angstrom – Prescott Equation with Seasonal Calibration to Estimate Solar Radiation in Three Cities of Kerala, India
1Anju M Nair 2Dr. James K C
*1 MTech student, Department of Statistics, Cochin University of Science and Technology, Kerala;
**2 Professor, Department of Statistics, Cochin University of Science and Technology, Kerala;
Abstract:- Solar energy is the most widely available renewable energy because of its long availability, and clean and green nature. Solar irradiation is a vital element for a wide range of applications. The major use of solar energy is in the design of photovoltaic systems. When designing a Photovoltaic system, it is important to know the amount of solar irradiation available at a given location at a given time. This paper attempts to calibrate the Angstrom-Prescott equation seasonally and annually for three cities in the southern state of India, Kerala. The seasonal calibration is made according to the prevailing seasons in the state. The measured data for the development of the model is solar radiation received on a horizontal surface and sunshine duration. The calculated and measured values of seasonal and annual calibration are compared and analyzed using statistical test procedures. The root mean square error, mean bias error, and mean percentage error are lower and the coefficient of determination, Willmotts index of agreement and Nash-Sutcliffe coefficient of efficiency were around unity, which is considered satisfactory. The proposed model's calculated values are in good agreement with the data.
Keywords:- Solar photovoltaic systems; total solar radiation; sunshine duration; statistical test methods; solar radiation models.
-
INTRODUCTION
In order to achieve the desired goals, nations require ascertaining renewable energy and water resources, for sustainable development. Hence, the alternate source of energy like wind, solar, hydel, etc. is an augmentation of energy potential and it will be very much helpful in reducing environmental impacts [1].
The radiation from the sun that can produce heat, cause chemical processes, or generate electricity is known as solar energy. Solar radiation that we receive as heat and light can be transformed into useful thermal energy or used to generate electricity via solar photovoltaic cells or solar thermal power. Solar energy has the ability to meet all future energy needs if properly harnessed. Geographic location, earth-sun movements, the tilt of the earths rotational axis, atmospheric attenuation due to suspended particles, etc are the factors that affect the solar energy incident on the earths surface. The provision of accurate solar radiation data is critical to the success of solar energy projects. The amount of electricity generated by PV systems depends on the intensity and wavelength of solar radiation available to the PV device. The intensity of solar energy/insolation quantifies the solar resource potential or availability of a region. The radiation which receives in the top layers of the atmosphere is well defined and hence it can be calculated easily. But the solar radiation reaching the ground depends mainly on location and atmospheric conditions [2]. Information on solar radiation at a location is obtained by measuring sunshine duration hourly and daily values of global, direct, and diffuse radiation. Sunshine duration is the most commonly measured parameter and, therefore, scientists have used various methods to correlate global radiation with sunshine duration [3]. Systematic measurements of diffuse solar energy and the global (total) irradiation incident on a horizontal surface are usually undertaken by a national agency, which is the national meteorological office in many countries. There is a relative abundance of sunshine duration data, and therefore it is a common practice to correlate solar radiation with sunshine duration measurements [5]. In the absence and scarcity of trustworthy solar radiation data, the need for an empirical model to predict and estimate global solar radiation seems inevitable. These models use climatological parameters of the location under study. Among all such parameters, sunshine hours are the most widely and commonly used. The models employing this common and important parameter are called sunshine-based models [4].
In recent years, energy demands for nations like India have amplified many folds due to speedy urbanization and industrial growth [6]. Several under-developed countries including India are experiencing issues of energy deficit due to an increase in energy requirements [9], industrial expansion, increased growth rate of population, increasing cost of energy imports, inappropriate organization of energy resources, and lack of better implementation of energy policies [10]. To cater to the rising energy demands India, has set up an independent ministry for alternative energy resources [7]. Solar energy and other clean energy are emerging and growing rapidly over the globe [8]. Kerala, a southern state of India is a power deficit state with a -5.8% deficit as of April 2022 [11]. Kerala depends on hydropower and reliance on this is dubious in the present scenario. Hence an alternate source is a must-have one and renewable energy is the best alternative.
This paper aims to build a monthly medium-term forecasting model for the estimation of solar radiation on a horizontal surface based on meteorological data of three cities in the southern state of India, Kerala namely Pothencode (Thiruvananthapuram), Edayar (Ernakulam), Kanjikode (Palakkad). These data include global horizontal irradiance and sunshine duration. The Angstrom- Prescott coefficients are estimated seasonally and annually to construct the model.
The models proposed for estimating global solar radiation on the horizontal surface are evaluated after the comparison of their
statistical parameters. The statistical parameters used in this study, include the mean bias error (MBE), the mean percentage error (MPE), the root mean square error (RMSE), the coefficient of determination (R2), Willmotts index of agreement (d) and Nash- Sutcliffe coefficient of efficiency (NSE). These measured values are compared to calculated ones and evaluated by the statistical methods mentioned above at the final stage.
-
AREA STUDIED
Kerala is a state in India's southwestern corner. It is a short strip of land that runs between the Western Ghats Mountain range on the Eastern side and the Arabian Sea on the western side. Kerala is located on the Malabar coast which is a part of the western coast of the country, which has a good potential for solar energy harvesting.
Thiruvananthapuram is the capital of the Indian state of Kerala. It is located on the west coast towards the southern tip of India. Pothencode, Thiruvananthapuram is located at latitude 8.16950N, and longitude 76.89870E. The city has a climate that lies on the border between a tropical savanna climate (Koppen Aw) and a tropical monsoon climate (Am). As a result, its only distinct seasons relate to rainfall rather than temperature. The mean maximum temperature is 34 °C (93 °F) and the mean minimum temperature is 21 °C (70 °F). Thiruvananthapuram has an equable climate because of its closeness to the sea.
Ernakulam is situated in the central part of the Indian state of Kerala. It is the largest agglomeration in Kerala and over the years has experienced an increasing trend in energy demand across different sectors (Chullikal Energy Survey,2020). Edayar, Ernakulam is located at latitude 9.87250N, and longitude 76.55170E. According to the Koppen Climate Classificatin, it features a Tropical Monsoon Climate, since the district is based on the western coastal plains it remains quite near to the sea. The maximum average temperature of the city in the summer season is 33 °C (91 °F) while the minimum temperature recorded is 22.5 °C (72.5 °F)
Palakkad is 11.5% of the state's area which makes it the largest district of Kerala. Kanjikode, Palakkad is located at latitude 10.79760N, and longitude 76.74300E. The district of Palakkad can make a transition to a 50 percent Renewable Energy (RE) scenario (including 100 percent electricity from RE sources). The district of Palakkad has a high potential for renewable energy resources, especially wind and solar. This makes the district a very suitable case for a RE-based (off-grid and on-grid) development model (WWF,2015). In Palakkad, the summers are rainier than the winters. This climate is categorized as Aw by Koppen and Geiger (Tropical wet and dry or savanna climate). The average annual temperature in Palakkad is 25.9 °C | 78.6 °F. Table 1 gives the location details of the area under study.
LOCATION
LATITUDE(0N)
LONGITUDE(0E)
ELEVATION (M)
Thiruvananthapuram (Pothencode)
8.1695
76.8987
76
Ernakulam (Edayar)
9.8725
76.5517
18
Palakkad (Kanjikode)
10.7976
76.7430
113
Table 1: Location details
-
MATERIALS AND APPROACHES
The paper outlines a method for developing and assessing the solar radiation model with seasonal and annual calibrations for the area under study. Based on the collected data, the models are developed to estimate the monthly average daily solar radiation on a horizontal surface. The variables used for the calibration are monthly average daily global solar radiation on a horizontal surface and monthly average daily sunshine duration.
It is reasonable to assume that solar radiation is proportionate to the length of sunshine. The proportionality formula can be determined from the measurements of the variables using scatter diagrams and, more often than not, statistical regression procedures. This link between solar radiation and sunshine length was first stated in solar energy models as linear. Such a pioneering relationship was presented by Angstrom in 1924.
-
Angstrom Model
Angstrom (1924) proposed a linear relationship between the ratio of average daily global radiation and the corresponding value. The model was developed under conditions of a clear sky day and the ratio of average daily sunshine duration to the maximum possible sunshine duration.
The fundamental Angstrom-type regression equation connects monthly average daily radiation to clear day radiation and the average proportion of potential sunshine hours in a given place.
where = monthly average daily global radiation (Wh/m2 /day), = monthly average clear sky daily global radiation for the location in a given day, = actual sunshine duration in a day, (hours), = monthly average maximum possible bright sunshine
duration in a day and also known as monthly mean length of the day in hours, a and b = empirical coefficients. These coefficients
are location-specific coefficients referred to as fractions of extraterrestrial radiation on overcast days and on average days,
respectively.
The cloudless index is defined as the ratio n/N. It provides information on the research area's atmospheric characteristics and conditions and the Clearness Index is defined as the ratio of solar radiation at the surface of the Earth (H) to extraterrestrial
radiation (0) i.e., H/H0
-
Angstrom-Prescott Model
The Angstrom equation needs clear sky radiation data. One of the most important problems of the Angstrom equation is defining the parameters n/N and Hc. Because estimating clear sky radiation accurately may be difficult. Prescott (1940) suggested the use of extraterrestrial radiation (H0) to replace it, and this modification led to the formation of the Angstrom-Prescott equation [16].
where 0 is the monthly average daily extraterrestrial radiation.
The regression coefficients can be used to estimate Global Horizontal Irradiance in the area under study. The calibrated
Angstrom-Prescott equation can be used with daily data, monthly, seasonal, and annual. The a and b coefficients should be adjusted locally and for different times of the year, in order to better represent the depending on the variation of solar radiation brightness [26]. The coefficient a quantifies physically the fraction of diffuse radiation to Ho, and b the fraction of direct radiation. The coefficients a and b of the Angstrom-Prescott equation have a dependency on the physical characteristics of the atmospheric layer and are influenced by local latitude and altitude, in addition, to depending on seasonal variability of the atmospheric variable (rainfall, wind, humidity, and aerosol concentrations). The aerosols influence the visible light most efficient [27]. The coefficients of the Angstrom-Prescott equation i.e., a and b (intercept and slope, respectively) were determined through linear regression analysis relating daily ratio values H/H0 (dependent variable) vs. the daily values of n/N ratio (independent variable) [21].
The monthly average daily extraterrestrial solar radiation on a horizontal surface, for latitudes from (-600,600) can be calculated by using the below equation [17] which also the calculate the monthly average daily extraterrestrial radiation (H0) [18] is,
where sc = solar constant with a value of 1367 W/m2, = day of the year from January 1 to December 31 taking January 1st as 1, = latitude of the location.
The value of solar constant used is defined as in Duffie and Beckman, and NASA The declination angle can be calculated as [20],
The sunset hour angle is given by,
= cos1( tan tan ) (5)
The maximum possible sunshine duration N (hours) for a horizontal surface is given by,
N = 2/15 * (6)
-
Input Parameters
The best source of data for determining average incident radiation is solar radiation data readings. Pyranometers are radiometers designed for measuring the irradiance on a plane surface, normally from solar radiation. The data used in the study is from Solcast and NASA Power. For this study, monthly average daily solar radiation data on a horizontal surface for a period of 11 years from 2010 to 2020 are used from the sites mentioned. Sunshine duration is calculated from the obtained data.
Figure 1 shows the sunshine hours of the three cities under study monthly and Table 2 shows the coefficients for the selected cities of the state of Kerala.
14.00
12.00
10.00
8.00
6.00
4.00
2.00
0.00
MONTH
Pothencode Edayar Kanjikode
SUNSHINE DURATION (H/DAY)
Figure 1: Sunshine duration for the three cities
The suggested model correlates (H H0) and (n/N). The equation is calibrated annually and for seasonal calibration, the data is grouped according to the prevailing seasons in Kerala, namely; Summer (March-April-May, MAM), Monsoon (June-July-August- September-October, JJASO), Winter (November-December-January-February, NDJF). And finally, coefficients were calculated for the seasonal and annual calibrations.
Parameter a represents the overall atmospheric transmission for total cloud conditions, and the sum a+b denotes the overall atmospheric transmission under clear sky conditions [25].
-
Means of Comparison
The following statistical tests were used to assess the effectiveness of the suggested solar radiation models: the mean bias error (MBE), the mean percentage error (MPE), the root mean square error (RMSE), the coefficient of determination (R2), Willmtts index of agreement (d) and Nash-Sutcliffe efficiency (NSE). These tests are generally used to validate solar radiation estimating
ic
models. The H-
represents the calculated values and H-im
represents the measured values.
The MBE (kWh/m²/day) is a measure of the calculated values average deviation from the measured data. The MBE gives information on a model's long-term performance. It is preferable to have a low value. The average amount of overestimation of the calculated values is shown by a positive value.
The MBE is defined as,
The MPE (%) is an accurate indicator that usually expresses accuracy as a percentage. It is preferable to have a low value of MPE and it is defined as,
The RMSE (kWh/m²/day) is a measure of the variability of calculated values around the measured data that offers information on the models short-term performance. It is desirable to have a low RMSE value, which is defined as,
The R2 is a metric that lets us know how definite a model's prediction is. It is preferable to have an R2 value that is near to the unity. It's defined as,
Willmott (1982) proposed an index of agreement (d) as a standardized measure of the degree of model prediction error which varies between 0 and 1. The index of agreement represents the ratio of the mean square error and the potential error. The agreement value of 1 indicates a perfect match, and 0 indicates no agreement at all.
The Nash-Sutcliffe coefficient of efficiency (NSE) is a normalized statistic that determines the relative magnitude of the residual variance compared to the measured data variance. Nash-Sutcliffe efficiency indicates how well the plot of observed versus simulated data fits the 1:1 line. NSE = 1, corresponds to a perfect match of the model to the observed data. NSE = 0, indicates that the model predictions are as accurate as of the mean of the observed data, Inf < NSE < 0, indicates that the observed mean is a better predictor than the model.
LOCATION
PERIOD
COEFFICIENTS
a
b
a+b
Thiruvananthapuram (Pothencode)
MAM
0.27
0.47
0.74
JJASO
0.14
0.52
0.66
NDJF
0.17
0.41
0.58
Annual
0.15
0.48
0.63
Ernakulam (Edayar)
MAM
0.20
0.49
0.69
JJASO
0.24
0.52
0.76
NDJF
0.31
0.40
0.71
Annual
0.21
0.47
0.68
Palakkad (Kanjikode)
MAM
0.23
0.50
0.73
JJASO
0.35
0.39
0.74
NDJF
0.25
0.43
0.68
Annual
0.19
0.63
0.82
Table 2: Coefficients of the area under study
-
-
MODEL DESIGN AND ASSESSMENT
The monthly average daily extraterrestrial solar radiation (H0) on a horizontal surface, the monthly average daily sunshine hours(N), and the ratios of (H/H0) and (n/N) are calculated using equations (3-6). The regression coefficients (a and b) depend on the measured values of (H) and (n).
To evaluate the performance of the proposed solar radiation models, the correlation between the calculated ratios of (H/H0) and (n/N) for each model, is shown in Figure 2 to Figure 5 below.
H/Ho
-
Pothencode (b) Edayar (c) Kanjikode
Fig 2: Correlation between the ratios of (H/Ho) and (n/N) annually
H/Ho
-
Pothencode (b) Edayar (c) Kanjikode
-
Fig 3: Correlation between the ratios of (H/Ho) and (n/N) during Summer
H/Ho
-
Pothencode (b) Edayar (c) Kanjikode
Fig 4: Correlation between the ratios of (H/Ho) and (n/N) during Monsoon
H/Ho
-
Pothencode (b) Edayar (c) Kanjikode
-
Fig 5: Correlation between the ratios of (H/Ho) and (n/N) during Winter
-
-
OBSERVATIONS
The values of the regression constants a and b are in close agreement with those given for 101 locations in the paper of MartÃnez- Lozano et al, ranging from 0.06 to 0.44 for a and from 0.19 to 0.87 for b. Coefficient b shows a value greater than coefficient a and hence can conclude that the fraction of direct normal irradiation is more than diffuse irradiation.
The correlation coefficient takes a value higher than 0.9 for all the places under study for both the seasonal and annual calibration. In this method, it is possible to conclude that these ratios for the models have a strong correlation. The correlation coefficient values alone are insufficient for evaluating the calibrated model. As a result, various statistical testing procedures are implemented. Table 3 shows the statistical test values generated through the application of statistical test methods for the considered models. All calculated values obtained from the solar radiation models offer a very high percentage in representing the solar radiation measured data.
LOCATION
PERIOD
2
R
RMSE
(kWh/m²/d ay)
MBE
(kWh/m²/ day)
MPE
(%)
d
(-)
NSE
(-)
Thiruvananthapuram (Pothencode)
MAM
0.879
0.033
0.002
5.0
0.97
0.879
JJASO
0.942
0.013
0.002
4.4
0.98
0.937
NDJF
0.823
0.014
-0.004
2.9
0.94
0.799
Annual
0.943
0.021
0.007
3.7
0.98
0.932
Ernakulam (Edayar)
MAM
0.870
0.034
-0.008
5.9
0.96
0.872
JJASO
0.932
0.016
-0.008
3.3
0.97
0.915
NDJF
0.823
0.014
-0.003
1.8
0.94
0.808
Annual
0.943
0.018
0.003
4.1
0.98
0.951
Palakkad (Kanjikode)
MAM
0.929
0.014
-0.006
2.2
0.98
0.934
JJASO
0.825
0.017
-0.001
1.9
0.92
0.717
NDJF
0.813
0.015
0.007
2.4
0.92
0.763
Annual
0.952
0.027
0.004
4.7
0.98
0.949
Table 3: Results of the statistical tests performed for the sites under study
From the statistical tabl, it is clear that there is a good agreement between the measured and calculated values of global radiation for the locations. The index of agreement shows a value close to unity which indicates a perfect match i e., the predicted model is in good agreement with the measured data. It is further established by very low values of MBE, RMSE, and MPE as shown in the Table. The percentage error is less than +/- 6% at the locations under study. This suggests that the Angstrom-Prescott correlations model is a good model to estimate solar radiation in Kerala. The lower values of RMSE, MBE, and MPE are preferred as they indicated differences between an estimated and measured value of solar radiation. The lowest value of RMSE is 0.013 kWh/m2/day for Thiruvananthapuram. Similarly, the lowest values of MBE and MPE are -0.008KWh/m2/day and 1.8 % for
Ernakulam. Lower values of MBE and MPE indicate the goodness of fit between clearness index and relative sunshine hour. Also, the value of MPE is less than 5.9% at any locations considered. This suggested that the AngstromPrescott correlation model is a good model to estimate the solar radiation in Kerala.
The coefficient of determination lies between 0.813 and 0.952 which shows a good agreement with measured data. The Nash- Sutcliffe efficiency (NSE) values that range from 0.717 to 0.951 are closer to 1 in all sites. This shows that the model predictions are as accurate as of the mean of the observed data.
It appears that the performances of both seasonal and annual calibrations were virtually identical. In sum, all the results of the statistical indicators and tests support the estimated values of the solar radiation are close to the measured ones.
The plot between estimated daily solar radiation by using seasonal calibration and measured daily solar radiation and between estimated daily solar radiation by using annual calibration and measured daily solar radiation are shown in Figures 6 to 9.
Fig 6: Measured (Hm) and Calculated (Hc) values Annually
Fig 7: Measured (Hm) and Calculated (Hc) values for Summer
Fig 8: Measured (Hm) and Calculated (Hc) values for Monsoon
Fig 9: Measured (Hm) and Calculated (Hc) values for Winter
-
CONCLUSION
For three cities in Kerala, India's southernmost state, the Angstrom-Prescott equation has been developed for each season (Summer, Monsoon, and Winter), as well as for the entire year (annual). The performance of the developed Angstrom-Prescott equation was evaluated. For both seasonal and annual calibrations, the statistical analysis confirmed that there is a good harmony between measured and estimated solar irradiation i.e., a good correlation, a high index of agreement, a high coefficient of determination, and a good Nash-Sutcliffe coefficient of efficiency with lower values of errors. The seasonal calibrations performance was comparable to that of the annual calibrations. Since their performances are comparable, using seasonal and annual calibrations to estimate daily global solar radiation is optional.
a, b [-]: regression coefficients cos [-]: cosine
exp [-]: exponential
NOMENCLATURE
H [kWh/(m2·day)]: monthly average daily total solar radiation on horizontal surfaces Him[kWh/(m2·day)]: measured values of H- for the i-th month
Hic[kWh/(m2·day)]: calculated values of H- for the i-th month
H0[kWh/(m2·day)]: monthly average daily extraterrestrial solar radiation on a horizontal surface Isc[kW/m2]: solar constant
d [-] : Index of Agreement
MBE [kWh/(m2·day)]: mean bias error MPE [%]: mean percentage error
d[-]: day number, the total number of observations n[h/day]: monthly average daily hours of bright sunshine N[h/day]: monthly average number of daylight hours
R [-]: correlation coefficient
R2[-]: coefficient of determination
RMSE [kWh/(m2·day)]: root mean square error sin [-]: sine
max: maximum; min: minimum
[º]: declination angle[%]: percentage error
[-]: constant [º]: latitude [º]: sunset hour angleREFERENCES
[1] Hirano, Y., Fujita, T., 2012. Evaluation of the impact of the urban heat island on residential and commercial energy consumption in Tokyo. Energy 37 (1), 371383 [2] Altin Maraj, Coskun Firat, 2014. Solar radiation models for the city of Tirana, Albania. International Journal of Renewable Energy Research Altin Maraj et al., Vol.4, No.2, 2014. [3] P. K. Veeran, Solar radiation analysis for Tamil Nadu. Unpublished M.Phil. dissertation. Madurai Kamaraj University, Madurai, India (1993) [4] Ahmad, F.and Ulfat, I (2004). Empirical model for the correlation of monthly average daily global solar radiation with hours of sunshine on a horizontal surface at Karachi, Pakistan. Turkish J. Physics 28: 301-307. [5] R. C. Srivastava and Harsha Pandey.,2013. Estimating Angstrom-Prescott Coefficients for India and Developing a Correlation between Sunshine Hours and Global Solar Radiation for India. [6] Moonen, Peter, et al., 2012. Urban physics: Effect of the micro-climate on comfort, health and energy demand.Front.Archit.Res.1(3),197228 (April 2, 2014). [7] Thirumurthy, Nisha, Harrington, Laura, Martin, D., Thomas, L., 2012. Opportunities and challenges for solar minigrid development in rural India. [8] Vinod, Raj Kumar, Singh, S.K., 2018. Solar photovoltaic modeling and simulation: As a renewable energy solution. Energy Rep. 4, 701712. [9] Redweik, P., Catita, C., Brito, M., 2013. Solar energy potential on roofs and facades in an urban landscape. Sol. Energy 97, 332341. [10] Inman, Rich H., Pedro, Hugo T.C., Coimbra, Carlos F.M., 2013. Solar forecasting methods for renewable energy integration. Prog. Energy Combust.Sci. 39 (6), 535576.
[11] Central Electricity Authority of India report 2005-2022. [12] World Wide Fund for Nature report 2015 [13] Chullikal Energy survey 2020. [14] A. Angstrom, Solar terrestrial radiation, Quarterly Journal of the Royal Meteorological Society, vol. 50, no. 210, pp. 121-126, 1924. [15] J. A. Prescott, Evaporation from water surface in relation to solar radiation, Transactions of The Royal Society of South Australia, vol. 40, pp. 114 118, 1940 [16] Liu, X.; Mei, X.; Li, Y.; Zhang, Y.; Wang, Q.; Jensen, J.R.; Porter, J.R. Calibration of the Angstrom-Prescott coefficients (a, b) under different time scales and their impacts in estimating global solar radiation in the Yellow River basin. Agricultural and Forest Meteorology, v. 149, p. 696-710, 2009. [17] R. Lazzarin, Sistemisolariattivi, Franco Muzzio & C., Padova, 1981. [18] J. A. Duffie and W. A. Beckman, Solar Engineering of Thermal Processing, John Wiley & Sons, Madison, Wis, USA, 2nd edition, 1991. [19] NASA- National Aeronautics and Space Administration. Solar Electromagnetic Radiation. NASA SP-8055, 1971. [20] P. I. Cooper, The absorption of solar radiation in solar stills, Solar Energy, vol. 12, no. 3, pp. 333-345, 1969. [21] Wilks, D.S. Statistical Methods in the Atmospheric Science. 2nd. Ed. San Diego: Academic Press, 2006. 627 p. [22] https://solcast.com [23] https://power.larc.nasa.gov/data-access-viewer [24] Willmott, C.J. Some Comments on the Evaluation of Model Performance. Bulletin of the American Meteorological Society, v. 63, p. 1309-1313, 1982. [25] Podesta, G. P., Nunez, L., Villanueva, C. A., Skansi, M. A. (2004): Estimating daily solar radiation in the Argentine Pampas. Agricultural and ForestMeteorology 123(1): 41-53.
[26] Allen, R.G.; Pereira, L.S.; Raes, D.; Smith, M. Crop evapotranspiration-guidelines for computing crop water requirements. Rome: United Nation FAO, Irrigation and drainage paper, paper n° 56, 1998. [27] Meesang, W.; Bualert, S.; Wonglakorn, P. Sea Salt Aerosols: Shortwave Radiative Forcing. International Journal of Environmental Science and Development, v. 4, n. 2, p. 104-106, 2013. [28] J. Martinez-Lozano, F. Tena, J. Onrubia, and J. De La Rubia, Th historical evolution of the angstrom formula and its modifications: review and bibliography, Agricultural and Forest Meteorology, vol. 33, no. 2-3, pp. 109128, 1984.