
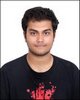
- Open Access
- Authors : Sanjay Rao Kadali , John Sapsford
- Paper ID : IJERTV9IS100281
- Volume & Issue : Volume 09, Issue 10 (October 2020)
- Published (First Online): 04-11-2020
- ISSN (Online) : 2278-0181
- Publisher Name : IJERT
- License:
This work is licensed under a Creative Commons Attribution 4.0 International License
Development of Smart Bin System Technology To Reduce Improper Disposal
Where Garbage Gives You Wi-Fi.
Sanjay Rao Kadali
MSc Advanced Computer Science with Advanced Research
University of Hertfordshire Hatfield, AL10 9DE London, United Kingdom
Prof. John Sapsford Project Supervisor University of Hertfordshire Hatfield, AL10 9DE London, United Kingdom
AbstractAlthough the synthetic or plastic matters that are toxic to the environment, they are littered around by human beings, lacking the sense to bin it into an appropriate waste container. This carelessness causes a drastic change in environmental health, causing numerous types of pollutions and on top of all, affects living beings. To figure out a way for reducing improper disposals, this paper proposes an idea to spread awareness on binning such non-degradable components into a smart bin system, which is interactive with the public around it. The paper talks about building a high-tech smart bin system that keeps track of the amount of waste getting collected and it has some special features of providing internet access to the users who approach to dispose some plastic wastes into the bin. The bin is also fully-fledged in allowing users to collect points at every disposal of waste.
KeywordsWaste disposal; smart bin systems; dynamic QR code; web application; wifi.
I. INTRODUCTION
A. The Problem
With various advancements in the field of technology and manufacturing industries, mankind has always found ways to enhance the methods, to improve productivity and thus, increase the standards of it. The demand for such has been never-ending. There is one such approach that mankind has made, perfect use of wastes for recycling.
This methodology enables the industries and organizations to reuse the matter and components, again and again by recycling it and giving it shape for reusability. Although, such recyclable matter, when exposed to the clean and green environment, becomes harmful to the living being s and nature as well. The possibility of such happening is when human beings neglect to maintain the cleanliness of the surroundings by not binning the wastes into garbage bins.
Also, it becomes difficult for the waste collectors to fetch back all the waste matter that the organizations have produced and need them back for further production. As a resultant, the amount of recyclable matter that was produced by the industries does not reach back for the process of recycling. The cause occurs due to improper binning of the plastic wastes and littering around in the surroundings.
B. Global Scenario
Littering affects the environment badly. The litter bins, toxic wastes or chemicals that are exposed to the surroundings can meet the rivers, forests, lakes and oceans, causing soil, air and water pollution. A most recent data shows that 7 billion tons of the long-lasting plastics enter the ocean bodies annually [1]. The littering also reduces the air quality due to the presence of toxic matter in plastics. These plastics are very often mistaken for food by animals on land and marine species. Around 100,000 sea mammals die due to the intake of littered plastics every year [1][2].
C. Research Question
My main work is to answer the research questions by developing advancements in some current technologies and working standards of waste management systems.
How technology can be more effectively used for the reduction of improper disposal of plastics and waste matter in the urban and domestic regions?
How plastic wastes and recyclable materials can be managed and collected back by the manufacturers and garbage collecting agencies for ensuring that no wastage is being drained or thrown off in the environment, causing a bad impact on the living beings?
D. Research Aim
I aim to provide a solution to gradually reduce litter into the water bodies and prevent the contact of toxic matter and chemicals with air and soil. The idea is to provide something to the public which values them, as they dispose of some wastes and plastics into the bin. The one such valuable and never-ending want is the internet. So, my motive is to develop a smart bin, that provides internet to the users, as they dispose of waste matter and plastics into it. The project is specific to the proper collection of waste items and gives out the 2 internets in return. This objective might create a positive response by the people of various groups and classes, by binning plastic wastes properly, and reducing litter.
The project also has an additional feature of the point collection system for the users to collect points as they bin and
use of later to benefit their lives. The overall outcome can be measured later by comparing the current waste management records, with the datasets generated by the smart bins. Also, it will be helpful to keep track of the number of wastes collected back for recycling. This idea can be achievable by surveying a region that shows chances of litter and then, implementing the smart bin idea over there and making the public aware of these intelligent bins around. The main goal is to collect more and more waste matter, rather than being littered on the roads and surroundings.
II. LITERATURE REVIEW
A. Survey on Littering
Surveys were conducted on the context of littering and the evidence states that everyone, almost everyone has littered at some point, with the majority of people doing it. Surveys involving attitude and behavior of the people suggested that around 50% of people litter or have littered at some point. Observational studies were attempted by the researchers to record and watch peoples littering and disposal behavior. The littering rates were calculated by observing under 10,000 disposal actions of the people. The surveys and observations postulated some factors that affected littering.
B. Current Technologies
Nowadays, there is no wonder that we use technology for nearly everything, from a keypress to dumping out wastes or garbage. Recent studies and papers have acknowledged various methods and standards of waste management systems. Some of these technologies are reviewed in this report. One such system was introduced by the OnePlus Systems [3] where an ultrasonic dust bin sensor tells how full the trash container is at every time. It allows the users to keep track of the bins. As having complete track of the waste can help in reducing the cost that arises with overfilling a dumpster. Another such technology was introduced by Ecube Labs [4], that focused on streamline trash pickups.
It maintained an integrated fleet management tracker, more like a cloud platform that combines waste container monitoring, route optimization, data analytics and fill-level forecasting. It helps in reducing operational costs by eliminating unnecessary pick-ups, providing dynamic collection routes and schedules for complete optimization of the collection operation. The concept of [5] is of real interest. It has been installed in over 2800 kiosks in malls, large retailers and grocery stores across the US. It provides immediate payment for old electronics devices. This smart waste collection system helped in managing and collecting 14 million smartphones and tablets from landfills.
A user can either recycle the device or refurbish for resale. Among a few more technologies that help in waste management systems, [6] solves the problem of improper waste sorting. Also, it tracks and sends data about the collected waste to an integrated cloud. It identifies the waste materials shape, color and type (plastic, paper or glass).
C. Researh on Improper Disposal of Waste
From generations, people needed to find ways of disposing of their waste. It becomes an important factor for proper disposal to avoid possible health danger, risk or threat. Due to its capability of polluting an area, it has become a major
sociological concern at present. Such improper waste is not just about human health, but also about nature which is our ultimatum goal of sustaining it for the future. The Municipality Solid Waste Management system (MSWM) [7] in Cambodia lacks system regulations, where the improper household items are burned, buried or dumped openly causing an impact on the environment and the surroundings. The improper waste reached from 361,000 tons of solid waste in 2008, to 635,000 tons in 2015.
In Chennai, a city in India generates about 3200 tons of improper waste every day. These wastes include various types of matter among which, the metals in the water imposes serious health risks to humans. The concentration rises in the topmost layer of soil to a depth of 5.5 meters [8]. Such improper disposals lead to various types of hazards involving health risks to living beings and pollution. A very recent report published in 2019, stated that the Mediterranean Sea contained around 94.6% of the microplastic matter of all the plastics, representing an abundance of 55% of the weight of all the plastics [9]. The remaining 5.3% of plastics represented mesoplastic matter in the Sea and 0.1% of the plastic that gets collected by the waste management systems.
D. The gap in Current Technologies
Although various techniques and management systems were introduced to reduce improper disposal and spend a lot more on waste transportation, there are still some stages that need to be worked on. With the explained technologies that have made people aware of the systems that make their lives easier than before, it lacks the motivation to follow proper disposal of waste by binning them appropriately. The present systems available are specific to waste collection by enhancing the techniques required for collecting them and consistently transferring them to the dumping destinations. But no system or devices have attempted to spread the importance of proper disposal of waste and thus avoiding harmful impact on the environment.
It is necessary to have a proper understanding of the basic measure to bin the plastic wastes and harmful matter appropriately so that the current technology can actively handle the further doings on the waste collection and waste tracking. There should be one potential motivational factor for the public who would follow proper binning of waste, that would always remind them to properly dispose of the waste that they are either carrying or looking to the bin, rather than littering around. This is where my idea fills the gap by providing the public with something that is a never-ending demand, internet. So, by introducing internet services through the bins, it makes it way smarter than before and spreading awareness of disposing the waste at the right place as well. This will not only focus on expanding the spread of the internet but also it will become an effective measure, reducing the chances of littering done by the public and other factors that cause concern.
III. DATA VISUALISATION AND ANALYSIS
The data visualization and analysis chapter present a detailed study of the attributes and data entities collected from the smart bin systems. These data entities hold properties of the proper functioning of the smart bin system and provide
information for practicing statistical analysis on various test cases and statuses where the smart bin actively operates. The datasets in this chapter are programmed and structured using data science modules and libraries written in python, that enable data visualization operations and study the informative resultants through it.
A. Dataset
The dataset (Fig. 1) used in this section is a dummy dataset which is structured as the data generated by the smart bin system. For performing data analysis on the functioning of the desired system, the dataset had to be well informed with some essential attributes that hold meaningful variables which could be used to visualize the data. The attributes that contributed to the dataset were the location of the smart bin, the status of it whether it is empty or full, the time at which a user attempted to dispose of some waste, time is taken to generate a QR code, time is taken to generate point collection code, time is taken to shift to next console for system-user interaction, the input choice given by a user to either get instant Wi-Fi or collect points.
The data attributes and analysis on it, mainly focus on resulting in the efficiency of the smart bin systems. It also helps to understand and predict the importance of having such smart bins in the societies under urban and sub-urban regions. The dataset also explains the need for having such smart bin systems for reducing the improper disposal of wastes and plastics. Other benefits of working on this dataset are to predict the trend among the user choices inputted during waste disposal. The smart bin offer features of collecting points or getting instant internet access. By the dataset generated, it will make us understand the want of the users through the choices inputted. The data studied would explain the importance of having such features for the users and how well it is benefitting in waste collection and the spread of the internet.
B. Data Attributes
Location: The location attribute specifies the region of the smart bin system functioning. This information helps in learning the type of crowd around the system and how often these smart bin systems are being used every day. This attribute is studied by pairing it with other timing attributed to the dataset to analyze the proper usage of the smart bins.
Bin Status: The bin status attribute specifies the status of the smart bin, whether it is empty, operating or filled. This information will help the waste collection services to locate those smart bins and collect the garbage out of it. The data information will also explain the number of times these bins got filled up completely. Further, such information will be studied for expanding the smart bin system setup at those regions.
Day: The day attribute in the dataset model will help in training the data for studying the usage of the smart bins weekly. The attribute will also explain the potential use of the smart bins depending on the region and type of day. The information of relational data information among the type of day, time and region will help in predicting the potential use of such smart
bin systems and how efficient enough are these systems for performing daily operations.
Date: The date attribute in the dataset model acts more like a tagging element where it will be helpful to study the usage of the smart bin every month. This attribute is essential for the analysis as this will 20 generate the frame for learning the total amount of waste getting collected every month. The data will be statistically compared with the real-world data sets from previous waste collection management systems. By this, a conclusion could be set depending on the impact created by the smart bin systems.
Time: The time attribute allows the dataset to be clustered into different sets of models for studying the use of smart bins at certain time frames. The information will be helpful to set a population graph among different timings, compared with other attributes like location, user choice and bin status. This element plays a vital role in producing various graph plots and charts for studying the operations and efficiency of the system.
User Choice: This attribute specifies the choice of input given by the users. A user choice attribute explains the importance of either feature that the smart bin provides. Features like generating QR code for instant Wi-Fi and generating a secure unique code for validating a point collection request acts as the fundamentals of the smart bin systems. The user choice attribute will help in understanding the impact presence of such features and how these features could be enhanced for making the system more efficient and user friendly and which feature is preferred more.
Unit: The unit attribute acts as a counter in the dataset model for recording the total number of system process cycle and training the data for many population gathering plots to analyze the data model. The attribute helps in clustering the entire dataset depending upon the categories like a type of location, day and time. Block of datasets will be produced through paring the user choice attribute among all the other categorical attributes for data visualization and analysis.
QR Code Generate Time: As the system is involved in loading and reading database files from a cloud storage server, it takes some time in performing data loading and framing it into a data model. The QR code time generation attribute provides the amount of time taken to generate a code within that system process cycle. A lot of factors affect the efficiency of the system cycle as it is continuously linked with the cloud server for loading and updating the data model.
Code Generate Time: The code generation time is the time taken by the smart bin system to generate a unique code whenever a user opts for collecting points. The time attribute, in this case, is used to study the time differences in every system process cycle. The attribute varies at every cycle as the system is connected to the cloud server and at every attempt of the generation of the code, the system calculates the time needed for generating and sending the code to the server. The attribute explains the time efficiency for loading the code to the server.
Shift Time: A shift time entity is a time taken for generating the QR code and returning the resultant on to the next console window to display the entire information along with the code on the user interface. This time attribute varies with changes in the network and complexity of the code generation. The shift times are plotted against several other categorical factors of the dataset model to study the time efficiency of the smart bin systems.
Fig. 1. Smart Bin System dataset sample
C. Data Collection
The data collection operation involves the collection of information in every system process cycle. A system cycle explained above in the report involves various steps and functionalities upon which the system triggers to invoke a function for collecting data elements at real-time. These data entities are independent of other attributes generated during a process cycle. The data attributes such a location of the smart bin, status of the bin, day, date and time are concentrated with the nature of smart bin operations. The attributes like user choice and unit are generated according to the users preference of choice for either collecting points or accessing instant internet through the smart bins.
The remaining attributes such as time taken to generate a QR code, time taken to generate the unique code for point collection and time taken between the system console shifts in a process, are focused on the efficiency of the system and finding out the need of some advancements in the functioning of the system. These data attributes are set in such a way that the smart bin system operations are monitored in a well- structured frame of parameters that are paired up accordingly, over a graphical plot for visualizing the resultants.
D. Data Visualisation
Data visualization techniques involve the graphical representation of information and data. The tools used in visualizing data provides various visual elements like graphs, maps, plots, etc., to study the data patterns and trends for making predictions on the working system cycle and advance the progress. The tools used for this project are purely developed through Python modules that are used in studying Data Science [10].
The modules such as Pandas, Numpy, Matplotlib, Seaborn, etc., are with equipped with learning the data and structuring it for performing data visualisation. The graph plots and other charts showcased in the upcoming sub-sections visualises the datasets that are generated by the smart bin system, looping in hundreds of system process cycles.
Fig. 2. Regression plots for QR code generation time and point code generation
The regression plot (Fig. 2) for the QR code generation time and the point collection code generation time provides visualized data to find the dependencies of the data points paired from the desired dataset attributes. The data points in this plot are clustered depending on the status of the smart bins. The regression line in each plot constructs the point distribution of all the data pairs based on the statuses of smart bins. The plot helps in the understanding correlation of independent and dependent values from the dataset.
Fig. 3. Smart bin usage area wise
The strip plot (Fig. 3) for the status of the bins to the corresponding areas visualises the data points that represent the usage of the smart bin system area wise. The plot tries to describe the usage of the smart bins in various areas where the smart bins are installed and provide information about the status of the bins. The data plotted on the graph helps in studying the population of data points in a specific area depending on the current status of the smart bins.
Fig. 4. Waste disposal time frame
The waste disposal time frame (Fig. 4) provides the data distribution of the waste disposal count functioned at various time intervals. The distribution of the data points is grouped
every column attribute and calculates the average,masxtandard 0.300000 0.300000
deviation and the distribution of the value across the entire
75% 0.251724 0.251724
depending on the type of day and the plot visualizes the activity Code Generate Time count 7359.000000 7641.000000 |
||||
of the smart bin system usage at various time frame specified |
mean std |
0.059421 0.023734 |
0.059695 0.023865 |
|
in the graphical strip plot. The time frames such as morning, |
min |
0.020000 |
0.020000 |
|
afternoon, evening and late-night are segmented as different |
25% 50% |
0.039310 0.058621 |
0.039310 0.058621 |
|
timing intervals on which the system cycle is plotted for every |
75% |
0.080690 |
0.080690 |
|
specific type of the day. The plot explains the population of the |
max |
0.100000 |
0.100000 |
|
smart bin system usage. |
Shift Time |
count |
7359.000000 |
7641.000000 |
mean |
0.199818 |
0.199657 |
||
E. Data Analysis |
std |
0.059439 |
0.059591 |
|
The smart bin system data model (Fig. 5) describes all the |
min 25% |
0.100000 0.148276 |
0.100000 0.148276 |
|
numerical data attributes. The table analyses the total count for |
50% |
0.196552 |
0.203448 |
depending on the type of day and the plot visualizes the activity Code Generate Time count 7359.000000 7641.000000 |
||||
of the smart bin system usage at various time frame specified |
mean std |
0.059421 0.023734 |
0.059695 0.023865 |
|
min |
0.020000 |
0.020000 |
||
afternoon, evening and late-night are segmented as different |
25% 50% |
0.039310 0.058621 |
0.039310 0.058621 |
|
timing intervals on which the system cycle is plotted for every |
75% |
0.080690 |
0.080690 |
|
specific type of the day. The plot explains the population of the |
max |
0.100000 |
0.100000 |
|
smart bin system usage. |
Shift Time |
count |
7359.000000 |
7641.000000 |
mean |
0.199818 |
0.199657 |
||
E. Data Analysis |
std |
0.059439 |
0.059591 |
|
The smart bin system data model (Fig. 5) describes all the |
min 25% |
0.100000 0.148276 |
0.100000 0.148276 |
|
numerical data attributes. The table analyses the total count for |
50% |
0.196552 |
0.203448 |
Fig. 6. User Choice Analysis
dataset. From the table, it is studied that the average or mean count of the waste disposal units is 27 approximately.
The count falls under the distribution of 50% of the entire unit count. The average mean for QR code generation time is
2.49 or 2.50. This value falls under the distribution of 50% of the total count of QR code generation times. The resultant data is similar for the remaining column attributes and all the mean value falls under the 50% of the entire dataset samples.
Unit QR Generate Time Code Generate Time Shift Time
count 15000.000000 15000.000000 15000.000000 15000.0000
00
mean 27.371667 2.496368 0.059560 0.1997
36
std 13.274892 1.482904 0.023801 0.0595
15
min 5.000000 0.000000 0.020000 0.1000
00
25% 16.000000 1.206897 0.039310 0.1482
76
50% 27.000000 2.413793 0.058621 0.2000
00
75% 39.000000 3.793103 0.080690 0.2517
24
max 50.000000 5.000000 0.100000 0.3000
00
Fig. 5. Smart bin system dataset description
The user choice analysis (Fig. 6) provides statistical information regarding the preferences of the smart bin features opted by the users. The user choice holds two data entities in the choice attribute column, that are Wi-Fi and Points. The data set is analyzed to find the frequencies of both the data entities by describing the entire dataset and performing data
The table also compares the column attributes against the generation time attributes and shift time. For both the generation time attributes, the mean value falls under the distribution of 50% of the entire dataset and it deviates within the range of 0.02 to 1.49.
The bin status analysis (Fig. 7) describes the mean, standard deviation and data distribution of the statuses of the bins against all the time attributes.
Bin Status Empty Filled Operating Unit count 5003.000000 5015.000000 4982.000000
mean 27.572656 27.503290 27.037334
std 13.220127 13.328216 13.272256
min 5.000000 5.000000 5.000000
25% 16.000000 16.000000 15.000000
50% 28.000000 27.000000 27.000000
75% 39.000000 39.000000 39.000000
max 50.000000 50.000000 50.000000
QR Generate Time count 5003.000000 5015.000000 4982.000000
mean 2.507323 2.473029 2.508859
std 1.492167 1.477824 1.478691
min 0.000000 0.000000 0.000000
25% 1.206897 1.206897 1.206897
50% 2.586207 2.413793 2.586207
75% 3.793103 3.793103 3.793103
max 5.000000 5.000000 5.000000
Code Generate Time count 5003.000000 5015.000000 4982.000000
mean 0.059521 0.059436 0.059725
std 0.023858 0.023557 0.023990
min 0.020000 0.020000 0.020000
25% 0.039310 0.039310 0.039310
50% 0.058621 0.058621 0.061379
75% 0.080690 0.080690 0.080690
max 0.100000 0.100000 0.100000
Shift Time count 5003.000000 5015.000000 4982.000000
mean 0.199365 0.200377 0.199463
std 0.058781 0.060278 0.059481
min 0.100000 0.100000 0.100000
25% 0.148276 0.148276 0.148276
50% 0.203448 0.203448 0.196552
75% 0.251724 0.251724 0.251724
distribution over the specified entity values. The table belowmax 0.300000 0.300000 0.300000
calculates the mean value for each type of user choices and the distribution among the occurrence of it under the set of value count. From the analyzed data, the frequency of the Wi-Fi is highest with a mean value of 27.53 and a standard deviation of 13.32.
User Choice Unit |
count |
Points 7359.000000 |
WiFi 7641.000000 |
mean |
27.213616 |
27.523884 |
|
std |
13.220444 |
13.326213 |
|
min |
5.000000 |
5.000000 |
|
25% |
16.000000 |
16.000000 |
|
50% |
27.000000 |
28.000000 |
|
75% |
39.000000 |
39.000000 |
|
max |
50.000000 |
50.000000 |
|
QR Generate Time |
count |
7359.000000 |
7641.000000 |
mean |
2.496896 |
2.495859 |
|
std |
1.490847 |
1.475310 |
|
min |
0.000000 |
0.000000 |
|
25% |
1.206897 |
1.206897 |
|
50% |
2.413793 |
2.413793 |
|
75% |
3.793103 |
3.793103 |
|
max |
5.000000 |
5.000000 |
Fig. 7. Bin Status Analysis
IV. RESULTS AND CONCLUSION
A. System Operation Results
The data analysis done on the smart bin systems process time describes that every system process cycle function independently. The timing attributes like QR code generation time, point collection code generation time and shift time show dependencies on the system cycle operations. The QR code generation time from the dataset description table conveys that, the average time taken to generate a QR code is around
2.49 seconds. The time consumed for the code generation varies with the intensity of the network through which the smart bin system cycles on transmitting data to the cloud storage servers.
This specifies that the code generated could be made more efficient by enhancing the efficiency of the network and system process.
The point collection code generation time is an independent attribute, as it does not depend on the network strength of the system or the other parameters that affect the system cycle. The code generation occurs within the system by randomly generating a numeric code. The mean time taken by the system to generate code is 0.05 seconds.
The distribution of the generation time falls from a range between 0.02 seconds and 1.0 seconds. The system generates the code very efficiently with less than half a second and the variation is negligible. The shift time mainly focuses on the overall time taken to shift to the next console interface after loading the point collection code to the cloud storage server. The timing attribute is dependent on the network strength of the system and the meantime calculated for processing the code to the server is 0.19 seconds. The rangeof the shift time falls within 0.1 to 0.3 seconds. The variation is very minimal and does not affect the system process cycle as it is negligible.
B. Smart Bin System Results
The smart bin system operations are analyzed with various data elements that vary from being a region or type of choice or the time of disposal. The categorical attributes set for performing statistical and data visualizing methods are independent of each other. The attributes like the location of the smart bin, status of the bins and the time of the disposal, are all recorded during the system process cycle and these data triggers to various possibilities that are discussed in this section. As per the motive of keeping track of the waste disposals, the smart bin system was able to collect information of all the waste disposals attempted by the users.
The data analysis showed that almost every area that had smart bins installed, was able to collect enough waste with an average of 150 units of waste disposals every area. By visualizing the data onto graphical plots, the pattern in the waste disposal was studied by pairing all the categorical attributes to understand the population of the different time patterns at which the waste items were disposed corresponding to the type of bin status and location.
The dummy dataset for the smart bin system consists of total 15,000 data entries with all the attributes that support the functioning of the model. From the analysis performed on creating a random sample, it could be noted that a total of 4,10,575 units of wastes got disposed into the smart bin systems.
Unit QR Generate Time Code Generate Time Shift Time Location
Area1 |
42062 |
3777.586207 |
90.412414 |
310. |
703448 |
||||
Area10 |
41904 |
3848.275862 |
90.735862 |
310. |
375862 |
||||
Area2 |
43300 |
3942.413793 |
93.578621 |
308. |
396552 |
||||
Area3 |
40811 |
3748.620690 |
89.044138 |
300. |
537931 |
||||
Area4 |
40754 |
3791.724138 |
88.439310 |
294. |
148276 |
||||
Area5 |
42422 |
3828.448276 |
91.322759 |
302. |
220690 |
||||
Area6 |
38782 |
3541.724138 |
85.286897 |
283. |
613793 |
||||
Area7 |
40778 |
3709.482759 |
89.503448 |
297. |
144828 |
||||
Area8 |
41198 |
3655.344828 |
89.211034 |
299. |
2 |
Fig. 8. Waste Disposal
The table (Fig. 8) depicts an assumable amount of waste getting collected from every location on an annual scale where it totals up to 4,10,575 units every year. Assuming that, if each unit of waste disposal weighs around 1 Kilogram on a n average, then according to the data analysis, the smart bin s ystem would be able to collect around 410 tons of wastes incl uding plastics and other harmful substances that pollute the na ture. Also, by finding the least variation in the usage of the sm art bin systems, by viewing the time frame of the system cycl e, it would become to conclude success in making the system running in across every corner of some region. Although the o utcome does not answer the research question, the results fro m this project explain the possibility of following such a trend in reducing improper littering.
C. Conclusion
Even though the mankind has always focused on introducing newer techniques and getting the job done with putting lesser efforts to even bother about sustaining the resources, this idea would bring a difference in focusing more on advancing the similar techniques but, for conserving the extensive use of resources. For now, the idea is centrally focusing on spreading awareness on reducing improper littering of plastics and other harmful matters in the environment. The idea would focus on creating a positive impact on the public to make good use of such smart bins available to them at every corner they visit very often. With some certain features that the bin offers, it would help people benefit their own lives by either using the internet or collecting points as a bonus.
After making progress in designing such a smart bin, few things get added to the conclusion which includes the motive of creating it. The project idea aimed at reducing the litter due to improper disposal and how to make use of the technologies available to avoid such littering occurring to the least. Although, the functioning of the system at this stage wont be able to make any strong point on finding the potential use of the system. It would take years to determine the possible reduction in the flow of harmful wastes and plastics into the environment through littering. However, the data collected from these smart bin systems demonstrate valid points on having the waste getting collected responsively.
Also, the attempts on disposing of waste by the general users have provided information on the constant use of the smart bins, no matter which region or location it is installed at. Such responses and approaches made by the users result in active functioning of the smart bins and this leads to an impact on creating ideas, for building environment and user-friendly systems that not only acknowledges the crowd to follow sustainable development but to become more responsible towards nature and the surroundings. Concluding the project with a line of phrase,
No wire can complete a circuit unless it conducts electricity and no molecule on Earth remains fresh until the bane is returned to its good state. Sanjay Rao Kadali.
ACKNOWLEDGMENT
I am very thankful to my supervisor Mr. John Sapsford for his guidance and support throughout the project. The journey could never be so wonderful and exciting without the continuous support and hearty feedback of his.
The credit for my succeeding nature goes to my parents who gave endless support and encouraged me every time they could, by providing every possible resource and aid that helped me in figuring out the work I aimed to accomplish.
REFERENCES
[1] Waste Management, #homeishere Publications. Reasons, Consequences and Possible Solutions of Littering – http://environment.cenn.org/waste- management/publications/reasons-consequences- possiblesolutionslittering/ [2] Rinkesh (2018). Rinkesh, Conserve Energy Future |Page 3, Chan:60050384 |RSSing.com". [online] rinkesp.rssing.com. Available at: http://rinkesp.rssing.com/chan- 60050384/all_p3.html [Accessed 29 Aug. 2020]. [3] OnePlus Systems. (n.d.). OnePlus Systems | Waste Monitoring Sensor Solutions & the Internet of Tract. [online] Available at: https://oneplussystems.com/ [Accessed 29 Aug. 2020]. [4] Ecube Labs. (n.d.). Ultrasonic fill-level sensor | Clean FLEX. [online] Available at: https://www.ecubelabs.com/ultrasonic-fill- level-sensor/ [Accessed 29 Aug. 2020]. [5] uk.ecoatm.com. (n.d.). ecoATM UK – Sell Your Old Mobile Phones for Quick Payment – ecoATM UK. [online] Available at: https://uk.ecoatm.com/ [Accessed 29 Aug. 2020]. [6] bine.world. (n.d.). Bin-e Smart Waste Bin. [online] Available at: https://bine.world/ [Accessed 29 Aug. 2020]. [7] Chiemchaisri C., Juanga J.P., Visvanathan C. Municipal solid waste management in Thailand and disposal emission inentory. Environ. Monit. Assess. 2007;135:1320. doi: 10.1007/s10661- 007-9707-1. [8] Parameswari K., Padmini T.K., Mudgal B.V. Assessment of soil contamination around municipal solid waste dumpsite. Indian J. Sci. Technol. 2015;8:36. doi: 10.17485/ijst/2015/v8i36/87437. [9] de Haan W.P., Sanchez-Vidal A., Canals M., Party N.S.S. Floating microplastics and aggregate formation in the Western Mediterranean Sea. Mar. Pollut. Bull. 2019;140:523535. doi: 10.1016/j.marpolbul.2019.01.053. [10] Paffenroth, Randy & Kong, Xiangnan. (2015). Python in Data Science Research and Education. 164-170. 10.25080/Majora- 7b98e3ed-019.