
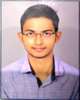
- Open Access
- Authors : Vipul Magare
- Paper ID : IJERTV10IS080239
- Volume & Issue : Volume 10, Issue 08 (August 2021)
- Published (First Online): 06-09-2021
- ISSN (Online) : 2278-0181
- Publisher Name : IJERT
- License:
This work is licensed under a Creative Commons Attribution 4.0 International License
Discuss Rough Fuzzy Clustering in Case of Leader and What Can Happen to Other Algorithms
Vipul Magare
CSE, JSPMs JSCOE, pune.
Abstract The clusters will in general have ambiguous or loose limits in certain fields, for example, web mining, since clustering has been broadly utilized. fuzzy clustering is delicate to commotions and possibilistic clustering is touchy to the introduction of group focuses and produces incidental clusters. In this presented paper discussion is about rough fuzzy clustering as leader and its impact on other algorithms.
Keywords Fuzzy set, rough set, leader clustering, fuzzy leader, possibilistic clustering, leader-based classifier, fuzzy K-means algorithm, clusters, fuzzy clustering.
-
INTRODUCTION
Needless to say each cluster is summed-up using one or more attorney patterns. For instance, centroid and pioneer are prominently utilized agents of a cluster. Leader clustering and Hard K-means algorithms can be suitably revamped to realize their fuzzy doppelgangers; among all of them the Fuzzy K-means algorithm is more prevalent. It is assured that both the hard and fuzzy K-means algorithms will obtain the locally preeminent resolution of respective individual goal parameters. A similar form of softness can be widespread to realize fuzzy versions of other hard clustering algorithms.
The indiscernibility connection, which is established based on comparability classes of objects, is used to create a rough set. Additionally, Cluster examination is a strategy that makes a piece of a given informational index into a bunch of groups so that two articles from a similar group are however comparative as could really be expected and the items from various clusters seem to be just about as unmistakable as could really be expected. In such manner, a cross breed solo learning calculation, assigned as unpleasant fuzzy c-implies, is presented in this part. It is an even blend of fuzzy set and harsh set qualities. While the idea of lower and upper approximations of unpleasant sets manages incertitude, significance, and deficiency in class definition, the participation capacity of fuzzy sets empowers employable treatment of covering parcels.
-
LITERATURE SURVEY
-
An adaptive rough fuzzy single pass algorithm for clustering large data sets. (S. Asharaf and M. N. Murty, 2003)
As indicated by Murty (2003), this paper introduces an effective variation of the pioneer calculation, called versatile unpleasant fuzzy pioneer calculation, which bludgeons the overall chief calculation. It utilizes a compound
of rough set hypothesis and fuzzy set hypothesis to catch the native vulnerability engaged with group examination. Moreover, Leader clustering calculation makes just a solitary pass through the informational index and tracks down a bunch of pioneer as the clusters appointees. It utilizes a client distinct edge and one of the examples as the beginning chief. The Adaptive rough fuzzy leader (ARFL) clustering plan that proposed in this paper isolates the informational collection into a bunch of covering clusters.
(Source:https://courses.cs.ut.ee/2003/dm-seminar- fall/uploads/Main/cluster-4.pdf)
-
Rough-fuzzy weighted k-nearest leader classifier for large data sets
(V. S. Babu and P. Viswanath, 2009)
As indicated by Viswanath (2009), to diminish the computational commitment of a classifier a leader set which is inferred utilizing the pioneers grouping strategy can be utilized instead of an immense preparing set. As of late, a presto and productive leader-based classifier called weighted k-closest leader-based classifier is demonstrated to be quicker and an effective classifier. Yet, there exist some dubiety while ascertaining the relative significance (weight) of the models. This report suggests a speculation over the prior suggested k- nearest leader-based classifier where a novel delicate registering strategy is utilized to determine the vulnerability. To examine the proposed strategy, interfused standards of rough set hypothesis and fuzzy set hypothesis are utilized. The
proposed technique called rough fuzzy weighted k-nearest leader classifier (RF-wk-NLC) utilizes a two-level chain of importance of models alongside their comparative centrality. RF-wk-NLC is shown by utilizing certain standard informational indexes to have further developed execution and is acclimatized with the previous related strategies.
proposed technique called rough fuzzy weighted k-nearest leader classifier (RF-wk-NLC) utilizes a two-level chain of importance of models alongside their comparative centrality. RF-wk-NLC is shown by utilizing certain standard informational indexes to have further developed execution and is acclimatized with the previous related strategies.
(source:https://content.iospress.com/articles/journal-of- intelligent-and-fuzzy-systems/ifs179078)
C. ROUGH-FUZZY CLUSTERING FOR GROUPING FUNCTIONALLY SIMILAR GENES FROM MICROARRAY DATA
(P. MAJI AND S. PAUL, 2013)
C. ROUGH-FUZZY CLUSTERING FOR GROUPING FUNCTIONALLY SIMILAR GENES FROM MICROARRAY DATA
(P. MAJI AND S. PAUL, 2013)
According to Maji (2013), quality articulation data clustering is one of the significant undertakings of practical genomics as it gives an incredible asset to considering useful connections of qualities in a biological process. There is the basic contest in gene clustering problem that is distinguishing co-expressed groups of genes. As a result, a high-quality clustering algorithm known as robust rough-fuzzy c-means is proffered, which wisely combines the advantages of fuzzy and rough sets. While the idea of lower and upper approximations of rough sets manages vulnerability, ambiguity, and deficiency in bunch definition, the combination of probabilistic and possibilistic enrolments of fuzzy sets empowers productive treatment of covering parts in loud climate. The idea of possibilistic lower bound and probabilistic limit of a cluster, habilitates proficient choice of quality cluster which is raised in vigorous robust rough-fuzzy c-means. A productive strategy is aimed to choose starting models of various quality groups, which empowers the offered c-means algorithms to join to an ideal or close to ideal arrangements and helps to discover co- expressed gene clusters. On 14 yeast microarray data sources, the algorithm's efficacy is validated both quantitatively and qualitatively, together with a reference to other algorithms.
-
-
FEW APPROACHES
Since clustering has been widely utilized, the clusters will in general have muzzy or loose limits in certain fields, for example, web mining. Fuzzy clustering is discerning to commotions and possibilistic clustering is insightful to the instatement of cluster focuses and effectuates incidental groups. In addition, considering mix of fuzzy clustering and possibilistic clustering, a novel possibilistic fuzzy leader (PFL) clustering calculation is provided in this work article to get around these abandonments. The framework of the leader algorithm is used by evaluating the benefits of the leader method in terms of time effectiveness and cluster initialization.
Ideal rough fuzzy clustering for customer profile mysticism based website page proposition assessment: Personalized data suggestion taking into account social naming is a hot issue in the academic local area and this site page information gathered from the Internet of Things (IoT). To achieve customized web pages, the current examination proposes a suggestion system with two approaches on client access conduct utilizing Rough-Fuzzy Clustering (RFC) procedure.
(source: https://content.iospress.com/articles/journal-of-intelligent- and-fuzzy-systems/ifs179078)
A gathering of randomly chose clients was secluded and based on the acquired information, their clustering was performed by cluster investigation In comparison to existing clustering techniques, the findings were evaluated with performance metrics and a number of top preferred pages were given to users based on the existing conceptual scheme.
-
CONCLUSION
From above research and papers it come to conclusion that leader algorithm catalyzes cluster abstraction in a exclusive data set scan. This is a measurable algorithm and the necessity of recollection of the algorithm is limited to the space required for two simple set accomplishments. At present, we are avouching a rise in the research and application of challenges in the context of Big Data. This is basically because of the extraordinary preferences which come from the information extraction from a high volume of data. Among the various methodologies utilized in Data Mining, those models dependent on fuzzy frameworks stand apart for some applications. Among their favourable circumstances, we should pressure the utilization of a portrayal near the normal language.
-
REFERENCES
-
S. Asharafa, M. N. Murty(2003, December 26). An adaptive rough fuzzy single pass algorithm for clustering. Retrieved from elsevier: https://courses.cs.ut.ee/misc/datamining/2003/dm-seminar- fall/uploads/Main/cluster-4.pdf
-
Babu, V., & P.Viswanathb. (2009, September). Rough-fuzzy weighted k-nearest leader classifier for large data sets. Retrieved from ScienceDirect:
https://www.sciencedirect.com/science/article/abs/pii/S00313203 08005037
-
Maji, P., & Paul, S. (2013, March 1). Rough-Fuzzy Clustering for Grouping Functionally Similar Genes from Microarray Data. Retrieved from ACM Digital Library: https://dl.acm.org/doi/10.1109/TCBB.2012.103
-
Nandan, S., & Pravin, R. (2019, July 09). Optimal rough fuzzy clustering for user profile ontology based web page recommendation analysis. Retrieved from IOS Press: https://content.iospress.com/articles/journal-of-intelligent-and- fuzzy-systems/ifs179078
-
Paul, P. M. (2017). Fundamentals of Rough-Fuzzy Clustering and Its Application in Bioinformatics. Retrieved from World Scientific: https://www.worldscientific.com/doi/abs/10.1142/978981314455 2_0015