
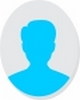
- Open Access
- Authors : Dr. Shiva Murthy G , Rajanna , Dr. G Varaprasad ,
- Paper ID : IJERTV9IS090160
- Volume & Issue : Volume 09, Issue 09 (September 2020)
- Published (First Online): 08-10-2020
- ISSN (Online) : 2278-0181
- Publisher Name : IJERT
- License:
This work is licensed under a Creative Commons Attribution 4.0 International License
Effective Sink Placement Mechanism for Multisink Wireless Sensor Networks
Mr. Rajanna #1, Dr. Shiva Murthy G*2, Dr. G Varaprasad #3
#Research Scholar, Department of CSE, VTU PG Center, Muddenahalli
Abstract: Wireless Sensor Network (WSN) contains hundreds or thousands of these sensor nodes. These sensors have the ability to communicate with each other or directly to the sink node. A large number of sensors in the phenomena increase the accuracy of sensing and coverage of phenomena. Routing is one of the critical issues in WSNs. Researchers proposed routing protocols for single sink WSNs. Identifying the location of the sink placement in the multiple sink wireless sensor networks is a challenge. In this work effective sink node placement mechanism is proposed by verifying the node density, the number of hops needed to route the data from node to sink node is minimized.
Keywords:- Multilink, WSNs, Energy Efficient, Sink Placement, Node Density
-
INTRODUCTION
Recent advancements in Micro Electronics and Mechanical Systems (MEMS) made cost-effective radio-enabled transducers introduced. These are also called Sensors and also known as a sensor node. The sensors are capable of sensing, computing, and communication. It has also had optional mobilizer, location finding systems, and energy harvesters. These autonomous sensors self configures a wireless sensor Network (WSN). These WSNs have few hundreds to few thousands of sensor nodes. The number of sensor nodes in the network is varied depending on the requirement of specific WSNs applications. Larger the number of sensor nodes in the network covers the larger geographical regions with acceptable accuracy. In most of the applications of WSNs, sensors are randomly deployed in the phenomena or region of interest. As the WSNs are infrastructure-less networks, Sensor nodes coordinate among themselves to produce high-quality data or information about the physical environment [1][2][3]. These sensed data communicate to the common sink node or base station. These sink nodes may be a static node or having mobility that has the capability of reaching all the nodes in the network and connecting the WSNs to an established infrastructure-based network or to the remote data storage through the Internet.
The WSNs have shown its importance in a wide variety of applications. For example, military applications like target identification and intrusions detection, environmental applications like agricultural farm monitoring, Precision Agriculture, habitat monitoring in the forest, forest fire detection, health applications like remote diagnostic systems, medication reminders as well as medical devices reminder systems[4][5]. It also includes health care systems like predictive diagnostic systems, biomedical feedback
control systems, and telemedicine systems. Another area of application is like monitoring home appliances, monitoring elder people at home. It has its importance in the construction industry for monitoring material strength monitoring, managing construction inventory, monitoring construction quality, managing office building, etc. In WSNs, sensor nodes are interconnected or self-organized to form a network. Sensor nodes send the data to the sink node through multiple hops. It has the following advantages: In single sink sensor networks, the nodes which are near to the sink node may exhaust its energy sooner than the other nodes in the network. The nodes with less remaining energy and the heavily loaded node may participate in the routing. These situations are avoided in the multi sink wireless sensor networks. Most of the applications in WSNs have thousands of sensor nodes in phenomena. Sensor nodes in WSNs with thousands of nodes need to send the sensed data to the single sink node.
Single sink node in such a large number of sensor nodes is causing a high end to end delay, usage of high control messages in the network, and results in increased energy consumption and reduced network lifetime. Multiple sink nodes in the WSNs are an alternate to these problems of single sink WSNs as in Fig 1.
Fig 2: WSNs with 2 Sink Nodes
Todays application of WSNs usually contains a larger number of nodes in the networks. All the sensor nodes in the network communicate the sensed data to the sink node. Unfortunately, existing routing protocols for many-to-one communication are inherently ill-suited to enhance efficiency with scenarios where a large number of sensors in the network [10][12]. This papers proposes an effective sink node placement mechanism by verifying the node density, the number of hops needed to route the data from node to sink node is minimized.
The rest of this paper is organized as follows. In Section 2, discusses literature on multisink WSNs. Background on
the theoretical model is presented in Section 3. In Section 4, Sink placement mechanism is discussed. Results and discussion is presented in section 5 and We conclude with final remarks in Section 6.
-
LITERATURE REVIEW
Many researchers proposed the energy-efficient routing protocol for multi sink wireless sensor networks[8]-[17]. The major issue with multi sink wireless sensor network is how to place the sink nodes in the phenomena and balancing the energy cost uniformly across the sensor networks. In this section, sink node location mechanisms are studied. Many researchers presented their mechanisms to place the sink node where a larger number of nodes in the network. Few researchers identified the location of the sink node based on the node density.
Renke et.al [14] Proposed QoS routing protocol for multisink wireless sensor networks. QoS routing method based on field theory for the QoS guarantee in WMSNs. The method abstract the WMSNs as a gravitational field inspired by the sink node, each node in the network has a corresponding potential, which represents the distance to the sink node. The intermediate nodes select the next hop node according to QoS evaluation function. Under the guidance of the potential, all data will eventually flow to the sink node. The proposed Multisink QoS routing protocol can spend lower cost to establish the QoS path compared with SMR, and our algorithm also can lead to more rational energy distribution among nodes and higher energy efficiency during data transmission. The proposed protocol not discussed the multisink placement and how the energy is minimized.
Energy Optimized Routing Algorithm for multi-sink wireless sensor network is proposed by Haifeng Jiang et al[18]. The virtual potential fields force of the sensors is considered for data routing. To achieve load balancing and low energy nodes in the routing, potential values of the nodes were used to balance the energy consumption among the sensor nodes. Nodes with less residual energy are avoided to part of route between sources and sink node by adjusting intermediate nodes potential value in residual energy potential field. Authors set the node as low-energy node when its residual energy is less than 10% of the initial energy. In the routing process, the low-energy node with fewer hops is avoided by adjusting its potential value and the node with the same hop will be selected as relay node. The avoid strategy for low-energy nodes and load balancing strategy for multiple sinks are adopted to achieve effective balanced energy consumption.
Hui et al [16] proposed listening and Ant Colony Optimization based routing protocol for multisink WSNs. The ACOMSR identifies and establishes the routes and its maintenance by means listening. Ant colony optimization is used to update pheromone and select the route between the sensos and sink node. The ACOMSR reduces the energy resources in route discovery and its maintenance. It improves the route reliability using the power control and listening mechanism. The QoS optimization of multisink
WSNs is achieved. The proposed protocol not shown how the energy efficiency is achieved in the routing and balanced energy distribution is not shown.
Jayashree et al [17] proposed WSNs architecture. The WSNs is divided into number of clusters. Multiple sinks are used to reduce the energy utilization of the node and increases the network manageability. Every sensor node in a cluster is allotted to its sensed data to the designated sink node in order to ensure efficient usage of the sensor nodes and sensed information. The proposed Energy Efficient QoS Routing (EEQR) protocol addresses the end-to-end delay requirement and throughput of real-time data and non real- time data respectively to the designated sink node. The proposed EEQR is not justified how the energy efficiency is achieved and how the multiple sinks are placed and selection of sinks among the multiple sinks are not shown.
Do DuyTan et al [18] proposed a distributed traffic- balancing routing algorithm is proposed for multi-sink wireless sensor networks. It distributes traffic from sources to sinks. Distribution of traffic based on the nodes gradient field. It is used to find the neighbor node to route the data to the sink node.
The traffic through the neighboring nodes and distance cost from a source to the respective sink node is in the node gradient index. The nodes forward the data using a gradient search for routing and providing optimal paths and possible congestion on the path from source to corresponding sink nodes. The work balances the traffic between the source and destination by identifying data congested nodes and distributing the data through idle and underloaded nodes.
Zheng Ma et al [20] proposed a spatial query processing algorithm for multi sink WSNs. The proposed algorithm reduces the data messages to be communicated in the network by effective query processing. It reduces the energy consumption and minimum response time of query processing. A data collector node in each region collects the data and aggregates the data to sends the data to the next region. For achieving minimal energy consumption and minimal response time, our query processing model ensures that the nodes capable of execution of a query are involved in the query execution.
In [19] authors presented the work on to detect the density of nodes in a region that is communicating through a wireless medium. The node density function d(r), measured in nodes per m2 . To define it, let us take a set A of incremental size
|A|, that contains the point r = (x, y), and covering N(A) nodes. The node density is defined as the limit d(r) = lim
|A|0 N(A)/|A|. Formally, this limit is equal to 0 in all locations r, except in those where nodes are placed, where it is . It assumes that there is a range of sizes |A| such that A is (i) small enough to contain a uniform part of the network, but also (ii) sufficiently large so that the number of nodes within the set is very large and the ratio N(A)/|A| is stabilized to a finite and positive value, which we take to be the limit.
Bulusu et al [19] presented the effective Localized algorithms to detect the density of the network itself. The
network density () in terms of number of nodes per nominal coverage area. Thus, if N nodes are scattered in a region of area A, and the nominal range of each node is
2
() =
R is the transmission range of the sensor, the number of
sensor nodes in the network varies in each application. For an example, in a wireless sensor network with a uniform distribution of nodes, when () is 6 nodes, the probability
is the node degree indicates the number of neighbouring nodes.
Definition 2 :Closeness Centrality
The average length of the shortest path between the node and all other nodes in the network is closeness centrality of a node.
1
(, )
() =
1
that a node is connected reaches 1 regardless of actual node placement. For problems where such a critical saturation
Where, 1
(,)
= 0, if, there is no path from i to j and N is
density exists, the solution space density S can be related to network density. Let be the critical density required to accomplish a certain task. Is ()> , only a subset of nodes in any local neighborhood of size () needs to participate in the task. The size of the solution space S, is the number of distinct subsets of nodes that could be active in any neighborhood.
= ((())) In other words, for a given and are
()2 and grows rapidly with ().
-
BACKGROUND
Based on above observation, we propose density detection technique by measuring the degree of the node connectivity. The degree of connectivity of a node measures the number of nodes connected. The connectedness of the node defines the successful connectivity of the nodes in the network. It also takes into account of relative distance between nodes. WSNs are an undirected and unweighted network which denoted in the graph notation G = (V, E), where G represents the WSNs, V stands for the set of all nodes and E for the set of all edges. WSN is represented as an adjacent matrix A, which is to characterize the connectivity among nodes with 0 or 1.
Let there are P sink nodes in the network. Where each sink node collects the data from set of sensors Ti respectively. Ti covers all the sensors in the network. Where,{ : 1
=1
=1
} suchthat = =
the number of nodes in the network.
-
SINK NODE PLACEMENT MECHANISM
In this section, the sink node placement mechanism, Identification of Sink Node Location and Node Assignment Mechanism mechanisms are discussed,
Algorithm 1: Sink Node Placement and Network Initialization
Input Adjacency matrix A of network G = (V, E). Output: Sink Node Locations P = {S1, S2 ,,Sk} 1: Label all nodes in V unclassified U;
2: Calculate the Node degree and Node Closeness
3: Identify the location of the sink node in the Network
-
Fix the Sink node to the location of
5: Identify all the nodes in the network are connected to any one of the sink node
6: Repeat 5 until all nodes have been assigned; 6: return P
Algorithm 2: Identification of Sink Node Location
Input: ; {1, , N}
Output: Sink Node Locations P = {S1, S2 ,,Sk}
-
For all 1 to N If (() )
Then ()
-
Sort
-
3. For k =1 to ||
For j = k+1 to ||
If( {(), ()} > )
()
, is the disjoint set of nodes. Given a P number of sinks where each sink collects data from , where P is optimally small and covers all the sensors in the network is NP-hard optimization problem[20]. The objective of the sink placement in large scale WSNs is to uniformly distribute the data traffic among multiple sink nodes of instead of single sink node. And achieve higher energy efficiency and increase the network lifetime.
Definition 1: Node Degree
Let A be the adjacency matrix of network. The degree of node i in the network is defined as:
= j (di,j Tr)
Where, is the node degree, (x) =1 if 0 and (x)
=0 otherwise; di,j denotes the distance between node i and j in A, and Tr is the transmission range of node i and it may be configured using the objective of the routing protocol.
End for
End for
4. Return argmax
Algorithm 3: Node Assignment Mechanism
1: S
2: while not all sensors in the Network are covered do
3: T set that covers the most sensors that are not yet covered by S and less thn the threshold Hc
4: S S
5: end while
6: returns S
Proof:
Let Suj denote the number of nodes in the WSN that are still not covered in jth iteration, and let m denote the optimal number of sinks in the WSNs m = OPT.
In every iteration j, m optimal sink nodes are used to include all the nodes in the WSNs, and in particular to cover Suj. Therefore, there must exist at least one sink in the optimal solution that includes at least Suj/m nodes.
It is selected a sink in every iteration that which includes maximum number of nodes to a sink node and at least Suj/m
nodes.
Suj Suj/m nodes are available for next iteration j+, That is,
n
Where the last equality is due to the fact that = n since there are exactly n Sensor Nodes in the WSNs before the first iteration.
There are n number of sensor nodes before the first iteration, i.e, = n.
Also, it is clear that when in the stage i for which 1. It shows that it need to select at most one more group or cluster.
A simple bound shows that whenever i m · ln n then we has that 1:
if the size of the sensor nodes is reducing by a factor of 1/e approximate the number of iterations;
we have that after i = m · ln n steps the remaining number of nodes is smaller or equal to 1. Thus, after at most m · ln n
+ 1 = OPT ln n + 1 iterations the algorithm will terminate with a nodes are grouped to or assigned to any of the sink whose size is at most m · ln n + 1 = OPT ln n + 1 (ln n · OPT) = (log n · OPT).
-
-
RESULTS AND DISCUSSION
The performance parameters taken into consideration are Node degree, and closeness index in the sensor nodes. The simulation of the work is carried out using NS2. The area of simulation terrain is 200 * 200 Sq.m, MAC Type 802.15.4, Omni Directional Antenna, Transmission Range is 15 mtrs, and Number of nodes 100 and 200 nodes. The Fig 2, Fig 3, and Fig 4 shows the sink node placements when Number of Node is 100 and threshold hop count is varied between 3 to 5 respectively. The Fig 5, Fig 6, and Fig 7 shows the sink node placements when Number of Node is 200 and threshold hop count is varied between 3 to 5 respectively.
Fig 2. Sink Node placement when Threshold hop count is 3 when Number of Node is 100
Fig 3. Sink Node placement when Threshold hop count is 4 when Number of Node is 100
Fig 4.Sink Node placement when Threshold hop count is 5 when Number of Node is 100
Fig 5. Sink Node placement when Threshold hop count is 3 when Number of Node is 200
Fig 6. Sink Node placement when Threshold hop count is 4 when Number of Node is 200
Fig 7. Sink Node placement when Threshold hop count is 5 when Number of Node is 200
The experimentation clearly indicates that, when the number of nodes is 100, and its threshold hop count varied between 3 to 5, the number of sink locations identified is 4,4 and 3 respectively. Also, when the number of nodes is 200, and its threshold hop count varied between 3 to 5, the number of sink locations identified is 5,5 and 4 respectively. The result shows that uniform coverage of sensors nodes in the
network. It is seen that all the sensor nodes are binded to atleast any one of the sink node in the network. Clarity on which sensor nodes is connected to which sink node is need to be studied. Also uniform sensor nodes distribution based on their resource utilization also need to be addressed.
-
CONCLUSION
This research work being carried out in the direction of achieving the sink placement and sink node selection mechanisms in the WSNs. The study identified that placing minimum number of sink nodes in the WSNs is a NP Hard Problem. Near approximate mechanisms is proposed. The proposed sink placement mechanism identifies the sink position by calculating the node degree and closeness. It clearly the indicates that the sink placement where high density nodes are located. It is also taken consideration of placing the sink nodes having a minimum number of hops away from the each sink node. The novelty in sink placement is to by measuring the node closeness or node neighbouring index. The node degree and closeness is supports to identify the location of the sink node to be placed. The placement of sink node mechanism which enables a sink node communicates with number of nodes. The future work will be to investigate further optimization of sink nodes and further better communication among all the nodes in the WSNs. Also uniform sensor nodes distribution based on their resource utilization also need to be addressed.
REFERENCES
-
Muhamad AzmanMiskam ,Azwan bin Nasirudin and InzarulfaishamAbd. Rahim Preliminary Design on the Development of Wireless Sensor Network for Paddy Rice Cropping Monitoring Application in Malaysia, European Journal of Scientific Research, Vol.37 No.4 ,2009, pp.649-657.
-
R. Min, et al., "Low Power Wireless Sensor Networks", in the Proceedings of International Conference on VLSI Design, Bangalore, India pp.205-210, January 2001.
-
J.M. Rabaey, et al., "PicoRadio supports ad hoc ultra-low power wireless networking," IEEE Computer, Vol. 33, pp. 42-48, July 2000.
-
V. Raghunathan, C. Schurgers, Park.S, and M.B. Srivastava, Energy-aware wireless microsensor networks, IEEE Signal Processing Magazine, Volume: 19 Issue: 2 , March 2002 Page(s): 40
50.
-
Jennifer Yick, Biswanath Mukherjee, DipakGhosal, WirelessSensor Network Survey, Computer Networks 52, pages 2292-2330, 2008.
-
I. F. Akyildiz, W. Su, Y. Sankarasubramaniam, and E. Cayirci. Wireless Sensor Networks: A Survey. Computer Networks, 38(4):393422, March 2002.
-
http://en.wikipedia.org/wiki/List_of_wireless_sensor_nodes
-
Al-Karaki, J. N., and Kamal, A. E. Routing Techniques in Wireless Sensor networks: A Survey, Volume 11, Issue 6, pp.6-28, Dec 2004.
-
M. Min, PBR: priority based routing in multi-sink sensor networks, in Proceedings of the Conference on Wireless Sensor Networks, pp. 2528, Las Vegas, Nev, USA, 2007.
-
R. Kawano and T. Miyazaki, Distributed data aggregation in multi- sink sensor networks using a graph coloring algorithm, in Proceedings of the 22nd International Conference on Advanced Information Networking and Applications Workshops/Symposia (AINA 08), pp. 934940, March 2008.
-
H. Kim, Y. Seok, N. Choi, Y. Choi, and T. Kwon, Optimal multi- sink positioning and energy-efficient routing in wireless sensor networks, in Proceedings of the International Conference on Information Networking (ICOIN 05), pp. 264274, Jeju Island, Korea, February 2005.
-
M. Kalantari and S. Mark, Design optimization of multisink sensor networks by analogy to electrostatic theory, in Proceedings of the IEEE Wireless Communications and Networking Conference (WCNC 06), pp. 431438, Las Vegas, Nev, USA, April 2006.
-
A. Lukosius, Opportunistic Routing in Multi-Sink Mobile Ad Hoc Wireless Sensor Networks, University of Bremen, Bremen, Germany, 2007.
-
Renke Sun, Enjie Ding, Haifeng Jiang, RuntongGeng, Wei Chen, Peng Liu A QoS Routing Algorithm for Multi-Sink Wireless Multimedia Sensor Networks Advances in information Sciences and Service Sciences(AISS) Volume4, Number6, April 2012 pp. 203-210.
-
Haifeng Jiangand Renke Sun Energy Optimized Routing Algorithm in Multi-sink Wireless Sensor Networks Appl. Math. Inf. Sci. 8, No. 1, 2014 pp 349-354.
-
Hui Zhou, Dongliang Qing, Xiaomei Zhang, Honglin Yuan, and Chen Xu A Multiple-Dimensional Tree Routing Protocol forMultisink Wireless Sensor Networks Based on Ant Colony Optimization Hindawi Publishing Corporation, International Journal of Distributed Sensor etworks Volume 2012, pp 1-10.
-
JayashreeAgarkhed, G. S. Biradar, V. D. Mytri, Energy Efficient QoS Routing in Multi-Sink Wireless Multimedia Sensor Networks IJCSNS International Journal of Computer Science and Netwrk Security, VOL.12 No.5, May 2012 pp 25-31.
-
Do-Duy, Tan & Dinh, Nguyen & Kim, Dong-Seong. GRATA: Gradient-based traffic-aware routing for wireless sensor networks. Wireless Sensor Systems, IET. 3. 104-111. 10.1049/iet- wss.2012.0083, 2013.
-
Bulusu, Nirupama&Heidemann, John, Density Adaptive Algorithms for Beacon Placement in Wireless Sensor Networks, 2001.
-
A. Bhattacharya, A. Rao, K. P. Naveen, P. P. Nishanth, S. V. R. Anand and A. Kumar, "QoS constrained optimal sink and relay placement in planned wireless sensor networks," 2014 International Conference on Signal Processing and Communications (SPCOM), Bangalore, 2014, pp. 1-
Ma Z., Zheng J., Jia W., Wang G., An Efficient Spatial Query
Processing
Algorithm
in
Multi-sink
Directional
Sensor
Network. In: Wang G., Zomaya A., Martinez G., Li K. (eds)
Algorithms and Architectures for Parallel Processing. ICA3PP
2015. Lecture Notes in Computer Science, vol 9532. Springer,
Cham. https://doi.org/10.1007/978-3-319-27161-3_2
Ma Z., Zheng J., Jia W., Wang G., An Efficient Spatial Query
Processing
Algorithm
in
Multi-sink
Directional
Sensor
Network. In: Wang G., Zomaya A., Martinez G., Li K. (eds)
Algorithms and Architectures for Parallel Processing. ICA3PP
2015. Lecture Notes in Computer Science, vol 9532. Springer,
Cham. https://doi.org/10.1007/978-3-319-27161-3_2