
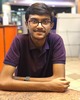
- Open Access
- Authors : Arinjoy Dutt , Sahil Bhogate , Sourasish Roy , Akhila Jagarlapudi, Prakash Nadgeri, D. P. Rathod
- Paper ID : IJERTV10IS070333
- Volume & Issue : Volume 10, Issue 07 (July 2021)
- Published (First Online): 07-08-2021
- ISSN (Online) : 2278-0181
- Publisher Name : IJERT
- License:
This work is licensed under a Creative Commons Attribution 4.0 International License
Error-Correcting Codes in 5G and Beyond
Sahil Bhogate
Department of Electrical Engineering VJTI, Mumbai
Arinjoy Dutt
Department of Electrical Engineering VJTI, Mumbai
Sourasish Roy
Department of Electrical Engineering VJTI, Mumbai
Prakash Nadgeri
Department of Electrical Engineering VJTI, Mumbai
Akhila Jagarlapudi
Department of Electrical Engineering VJTI, Mumbai
D. P. Rathod
Department of Electrical Engineering VJTI, Mumbai
Abstract – In recent years, communication networks have grown in size, and the potential for wireless communication has expanded. Error-correcting codes must be the foundation of these communication networks in order for them to function efficiently and accurately. Error-correcting codes are used to improve wireless communication performance. In this study, well look at different error-correcting codes and their applications in wireless communication. Every code has its respective advantages and drawbacks. 5G NR (New Radio) wireless mobile communication is the fifth-generation communication and successor to 4G communication network (LTE). There are three major application scenarios of 5G Standards: enhanced Mobile Broadband (eMBB), UltraReliable and Low Latency Communications (URLLC) and massive Machine Type Communications (mMTC).
The comparison of different error-correcting codes is made based on block lengths, coding rates and latency features. Different error-correcting codes mentioned in this paper are Low Density Parity Check codes, Polar Codes, Turbo codes, Convolution codes.
Talking about the future scope, we will be exploring the same in regards to 6G.
Keywords – 5G Communication, 6G Communication, Channel Coding, Error Correcting Codes
-
INTRODUCTION
With the demand for high data rates and low latency internet, wireless communication networks are implemented to improve performance. 5G is a heterogeneous framework that is based on the local-based IMT design in which the voice services and data services are consistent both indoors and outdoors [1]. 5G technology will provide improvements to net- work architectures across the board. In 5G systems, software- defined platforms will regulate networking functionality rather than hardware.
5G architecture enables a new kind of network to virtually connect everyone and everything together. 5G architecture gives a virtual access to the information with the help of cloud- based technologies and IT based automation. 5G wireless communication is preferred over 4G LTE in the present times. In comparison to LTE systems, several features such as scalable numerology, flexible spectrum, forward compatibility, and ultra-lean architecture are added to satisfy the aims of 5G NR. 5G wireless networks are developed in such a way that it provides high speed data rate up to gigabits
per second, greater dependability, ultra-low latency, amplified availability and greater user community for experience. There are specific modes of operation of the 5G communication network.
To achieve such growing demands in communication Ultra Reliable Communication (URC) has been implemented in 5G New Radio (NR). URC is a provision that provides guaranteed connectivity. URC is used to provide minimal connectivity, ad hoc networking including aspects of radio access. While the capability performance of the 5G era is exciting, knowing the eras capability isnt without its challenges [2]. Lithium battery technology is being looked at by researchers as a way to improve battery life and optimize 5G equipment for user expectations. Wave spectrum problems are particularly prevalent as a result of global 5G upgrades and modifications. MIMO (multiple-input, multiple-output) technology is proving to be an effective method for increasing 5G connectivity capacity and addressing signal path issues [3].
The error-correcting code is an algorithm for detecting and correcting errors in received message signals so that the original message can be recovered. The main goal of error- correcting codes is to recover the original message with the lowest possible Signal to Noise Ratio (SNR). The need for error-correcting codes began with the 2nd Generation (2G), which used BoseChaudhuriHocquenghem (BCH) codes, a type of cyclic error-correcting codes, in the early 1990s. 2G was then replaced by the 3rd Generation (3G) wireless system in the late 1990s. Rate-compatible raptor-like quasi-cyclic low-density parity-check (RL-QC-LDPC) codes, which are a typical class of multi-edge type LDPC codes with excellent performance and high flexibility, have been adopted in the technical specification of 5G new radio (5G-NR) [4].
Turbo Codes were used in 3G as they had higher coding gain. In 2009 the 4th Generation long-term evolution (4G LTE)
was introduced which is 10 times faster than the 3G. Turbo codes (improved variants) and LDPC codes are used in 4G LTE for error-correcting.
On April 3rd, 5th generation New Radio (5G NR) was introduced which is 100 times faster than 4G LTE. As short block size is preferred in 5G NR for Ultra Reliable Low Latency Communication (URLLC), choosing the most efficient error-correcting codes with sufficiently low Block Error Rate (BLER) is difficult. The 3GPP standardization group has recently debated whether it should be replaced by low density parity check (LDPC) or polar codes in 5G new radio, ultimately reaching the decision to adopt the LDPC code family for enhanced mobile broad band (eMBB) data and polar codes for eMBB control [5].
LDPC, Turbo, Polar, and Convolutional Codes should all be considered for 5G NR. Block codes and Convolutional codes are the two types of channel coding [6]. Convolutional codes work on data streams with more arbitrary bits, whereas block codes work on fixed-size data blocks.
The future steps for 6G are known to be heavily data- oriented with endless wireless connectivity. Keeping its main
-
OVERVIEW OF ERROR-CORRECTING CODES
A. LDPC
Low Density Parity Check codes were proposed by Robert
G. Gallager in 1963. But they were not implemented as the decoding algorithms were too complex. In 1997, LDPC codes were rediscovered by D MacKay, M Neal. On restudying the algorithm, they found that LDPC codes approached the Shannon Limit and could perform better than Turbo codes.
LDPC codes are linear block codes with a sparse parity check matrix and a minimum number of 1s, as the name implies. The parity check matrix is represented graphically by a Tanner graph. Tanner graph for LDPC code is a bipartite graph in which the check nodes represent the rows, while bit nodes represent the columns. Each check node specifies the single parity check code. The number of 1s in a row or column of a matrix is called weight. For Regular LDPC codes the weight is constant for rows and columns. For Irregular LDPC codes rows and columns have multiple weights. It is a powerful option for correcting forward errors. For a fixed rate, the minimum distance between these codes is linearly dependent on the block length. 5G LDPC codes are QC- LDPC (Quasi Cyclic-Low Density Parity Check codes) codes that belong to the protograph code family [10].
key points in mind, the hand-in-hand solution for a wireless B. Polar Codes
network solution comprises mainly two things – the effective
mass communication system and various network intelligence protocols [7]. Computational simplicity, scalability, increased convergence, minimal memory footprint, and high reliability are key features to successfully implement 6G [8].
It is expected that AI will strengthen 6G in allaspects ranging from network enhancement and management, physical layer signal processing, and data mining, to service- based context-aware communication.
With respect to the Machine-Type Communication in 5G and extended to 6G, the following 6 key outlining features are believed to be the most relevant and important [9] –
-
Effective mass communication (fast uplink, NOMA)
-
Multiple access edge computing as an enabler (Fast and localized data analysis, data/communication thinning)
-
Security for MTC (Advanced PHY security, Effective and cost friendly authentication)
-
Vertical aware solution (modular network design, new markets and revenue sources)
-
AI and Machine Learning are at the heart of this technol- ogy
Erdal Arkan first described polar codes in 2009. Polar codes have a unique structure which can attain the channel capacity of symmetric binary input memoryless channels using low- complexity encoding and decoding as the code length approaches infinity. The essential methodology in building these codes is channel polarization. A block code converts N discrete binary-input discrete memoryless channels into N synthesized channels with capacities of (near to) zero or one [11]. They have an upper hand over turbo codes in 5G(eMBB) enhanced Mobile Broadband control channels. Setting up 5G wireless communication links results in loss of the provided information in the transmitting end and corrupts it at the receiving end.
These codes work on algorithms and specific operations at the transmitter end on the original data stream and receiver end to correct the errors. Polar codes can provide 1-bit granularity for all coding rates and a wide variety of block sizes. The list decoders implementation complexity, on the other hand, increases as the list size is greater, especially with biggerblock sizes [11].
(Network Intelligence to deal with complexities andC. Turbo Codes
heterogeneous requirements)
-
Efficient with Energy (Energy harvesting, Energy- efficient transmissions)
This paper presents about the overview of possible Error Correcting Codes in Section II, the comparison of encoding and decoding methods in wireless communication using the mentioned error correcting codes in Section III, Conclusion about the preferred code based on their performance in Section IV and expected future in 6G communication in Section V.
Shannon discovered that as block length increased, the average performance of a randomly chosen ensemble of codes decreased exponentially, resulting in an exponentially decreas- ing error probability. Typically, the code designs contain a large amount of structure since it can be used to guarantee a good minimum distance as well as it is easier to decode highly structured code. Coding schemes were improved by Forney by attaching two or more building block codes. The resulting codes could correct errors as well as much longer codes,
and they had a structure that allowed for relatively simple to moderately complex decoding [12]. For the accompanying code sequence, a turbo code is known for its distinctive method of encoding and repetitive decoding technique. Berrou, Glavieux, and Thitimajshima proposed Turbo coding in 1993, which combines the concurrent concatenation of two convolutional encoders with iterative maximum a-posteriori probability (MAP) decoding. The operation range of Turbo Codes for larger block lengths are less than one by tenth decibel of Shannons Limit. They can use recursive convolution codes that are serial (concatenated) or parallel [13]. The output is recursive, which means it is dependent not only on the input sequence but also on the prior output. Turbo codes are used in deep space exploration and mobile communications (3G / 4G) [14].
D. Convolution
In 1955, Elias was the first to use convolutional coding. The encoder in linear block coding takes a k-bit message block and converts it to an n-bit codeword. As a result, codewords are created one at a time. When the system is busy, the allocation of the buffer is the most important consideration. When the message bits arrive serially rather than in blocks, however, the buffer may be unnecessary. Convolutional coding may be the best option in these situations. The encoder contains memory, which distinguishes them from block codes.
A convolutional encoder performs a continuous serial operation on an incoming message sequence. The symbols enter into the encoder one at a time. The M-stage shift register shifts the information symbols in sequential order. The convolutional encoder produces a coded output sequence of length n*(N+M) bits when given an N-bit message sequence, where n is the number of modulo-2-adders. r = N / n*(N+M) gives the code rate. The length K= M+1 is known as the constraint length of the code. A binary convolutional code with a rate of N / n*(N+M) bits per symbol is generated by a linear finite-state machine with an M-stage shift register, n modulo-2-adders connected to some of the shift registers, and a commutator that scans the output of the modulo-2-adders. The whole system is known as a convolutional encoder [14].
-
-
COMPARISON
We have seen the types of error-correcting codes above and can compare them using different modulation techniques by phase-shift keying and amplitude modulation. LDPC codes, Polar codes, Turbo codes and Convolutional codes have respective applications and advantages in wireless communication. This comparison can be successfully done by using the plots of BLER vs SNR and BER vs SNR.
LDPC codes have two types of possible decoding algorithms: a min-sum algorithm (MSA) and a sum-product algorithm (SPA). The commonly used algorithm is MSA. It is better than SPA as it is a much simpler algorithm. Yet it has some drawbacks, the number of iterations required by MSA is higher than that of SPA.
Polar codes are encoded by three types, systematic encoding, non-systematic encoding and generalized concatenated encoding techniques. These encoded outputs can be further decoded using Successive Cancellation (SC). But SC decoder is not optimized to the fullest. It cannot generate BER for short length block codes. This is rectified using a Successive Cancellation List (SCL) decoder.
Similarly, Convolution codes have 2 decoding algorithms that can be considered which are tailing bits and zero tail bits also called terminated decoding. The terminated CC technique produces better outcomes. This is due to the fact that the CC decoder is a 256-state decoder, whereas the TBCC decoder is only a 16-state decoder, ensuring that both investigated approaches are of comparable complexity [15]. LDPC with MSA terminated CC (zero tail bits), and polar codes with SCL are evaluated for comparison based on the preceding observations. Both the encoders and decoders proposed are shown to take advantage of the codes explicit decomposition into subcodes to decompose and simplify the associated computational processes [16].
The coding rate (also known as the information rate) is a fractional statistic that indicates how much of the redundant message is truly useful. The ratio of information bits generated by a coding process to the overall number of bits generated by the coding process is known as code rate. As a result, higher coding rates result in stronger codes.
Rc = K/n
, where k is the tally of useful bits and n is the total number of bits in the block code.
These encoding and decoding techniques of error- correcting codes require a bit of computational complexity. Other parity check bit techniques are also recommended like the Cyclic Redundancy Check which is used to improve the performance of wireless communication at the cost of high complexity.
-
CONCLUSION
In this paper, we have made a review about the error- correcting codes which can be implemented in wireless communication and compared it on the basis of various factors.
Collectively all the coding techniques are equally optmized on the basis of code lengths and their speed. But, when the block size is small, LDPC and polar codes perform better than turbo codes. When SCL decoding algorithm is used by Polar Codes, it is proved to be better than all other coding techniques by 0.25 to 2 dB, based on the coding speed and type of modulation used. The fact that LDPC does not require a CRC means that these codes work well for short messages. As a result, the effective coding rate of LDPC is slightly higher than that of the other coding methods. Turbo Codes on the other hand have poor performance since the codeword is too short to detect the turbo effect. For the medium-length frame, a different performance is seen. For all of the coding speeds and modulation techniques mentioned,
the turbo codes produce the best results.
When compared to TC, LDPC codes offer the second-best performance in all of the cases tested, with a 0.5 dB loss. On the other hand, BLER results and algorithmic complexity analysis can only be utilized to detect coding scheme changes to a limited extent. Many other variables, such as implementation complexity, latency, and flexibility, should be addressed when choosing a coding scheme for the URLLC usage scenario in 5G. For turbo, LDPC, and convolutional codes, we employed implementable decoding algorithms, and more research on latency constrained performance is needed before deciding on a coding scheme [15]. However, the use of polar codes for URLLC is currently dubious due to implementation challenges with list decoding and the sequential nature of SC decoding methods.
-
FUTURE SCOPE
The 6G vision is a data-driven society, enabled by near instant unlimited wireless connectivity [9]. With regards to 6G and its onset, the major focus is given on moving to- wards edge computing, incorporating healthier machine learning techniques and the most important of all being energy efficiency. Machine Learning and Artificial Intelligence have not been implemented till 4G and 5G whereas 6G can be made completely AI-driven. It has been said that an effective energy transmission is required to speed up the process judiciously. This would also include an effective and energy conserved error-correcting code [8].
The use of super-fast and high computational power processors at both the network and end devices will reduce network latency in 6G. The future networks mobile phones will be intelligent enough to sense the environment and take cautious and preventative steps. Talking about extending the LDPC codes from its 5G base, it gets difficult to support 6G due to its 100 x throughput requirements. So, given this, the encoder and decoder for this advanced error-correcting technique require robust latency and extremely efficient hardware.
A model-oriented deep learning approach for LDPC decoder and a linear programming method of LDPC decoding that was proposed by J. Feldman can be used as the most optimized solution in this case.
Now, moving onto Turbo Codes. For 6G, turbo codes will need to have exponentially high data rates at lower implementation complexity. A linear programming LDPC decoder which is DL based actually relies on a large data set and hence the computation becomes substantially complex. Incorporating domain knowledge will help reduce the requirement of large training data and will further help with minimizing the number of trainable parameters which will eventually lead to greatly improved performance.
Other ways to improve the efficiency of 6G is by using Optical Wireless Communication technologies (OWC) which is RF-based. The wavelengths ranging from 1330 nm to 1550 nm are used in optical wireless systems. These wavelengths are the most commonly used in optical fibre systems.
REFERENCES
-
S. Chen and J. Zhao, "The requirements, challenges, and technologies for 5G of terrestrial mobile telecommunication," in IEEE Communications Magazine, 2014.
-
P. Popovski, "Ultra-reliable communication in 5G wireless systems," in 1st International Conference on 5G for Ubiquitous Connectivity, IEEE, 2014, pp. 146-151.
-
W. H. Chin, Z. Fan and R. Haines, "Emerging technologies and research challenges for 5G wireless networks," IEEE Wireless Communications, pp. 106-112, 2014.
-
Y. Zhang, K. Peng, Z. Chen and J. Song, "Construction of Rate- Compatible Raptor-Like Quasi-Cyclic LDPC Code With Edge Classification for IDMA Based Random Access," IEEE Access, vol. 7, pp. 30818-30830, 2019.
-
S. Shao, P. Hailes, T.-Y. Wang, J.-Y. Wu, R. G. Maunder, B. M. Al- Hashimi and L. Hanzo, "Survey of Turbo, LDPC, and Polar Decoder ASIC Implementations," IEEE Communications Surveys Tutorials, vol. 21, no. 3, pp. 2309-2333, 2019.
-
H. Gamage, N. Rajatheva and M. Latva-aho, "Channel coding for enhanced mobile broadband communication in 5G systems," in 2017 European Conference on Networks and Communications (EuCNC), IEEE, 2017, pp. 1-6.
-
D. Kalbande, Z. khan, S. Haji and R. Haji, "6G-Next Gen Mobile Wire- less Communication Approach," in 2019 3rd International conference on Electronics, Communication and Aerospace Technology (ICECA), IEEE, 2019, pp. 1-6.
-
T. Melodia, A. Jagannath and J. Jagannath, "Redefining Wireless Communication for 6G: Signal Processing Meets Deep Learning," CoRR, 2020.
-
N. H. Mahmood, H. Alves, O. A. López, M. Shehab, D. P. M. Osorio and M. Latva-Aho, "Six Key Features of Machine Type Communication in 6G," in 2020 2nd 6G Wireless Summit (6G SUMMIT), IEEE, 2020, pp. 1-5.
-
M. V. Patil, S. Pawar and Z. Saquib, "Coding Techniques for 5G Networks: A Review," in 2020 3rd International Conference on Communication System, Computing and IT Applications (CSCITA), IEEE, 2020, pp. 208-213.
-
M. Shirvanimoghaddam, M. S. Mohammadi, R. Abbas, A. Minja, C. Yue, B. Matuz, G. Han, Z. Lin, W. Liu, Y. Li, S. Johnson and B. Vucetic, "Short Block-Length Codes for Ultra-Reliable Low Latency Communications," IEEE Communications Magazine, vol. 57, no. 2, pp. 130-137, 2019.
-
B. Sklar, "Turbo code concepts made easy, or how I learned to concatenate and reiterate," in MILCOM 97 MILCOM 97 Proceedings, IEEE, 1997, pp. 20-26.
-
T. Venugopal and S. Radhika, "A Survey on Channel Coding in Wireless Networks," in 2020 International Conference on Communication and Signal Processing (ICCSP), IEEE, 2020, pp. 784- 789.
-
Neha, "Implementation and Performance Analysis of Convolution Error Correcting Codes with Code Rate=1/2," in 2016 International Conference on Micro-Electronics and Telecommunication Engineering (ICMETE), IEEE, 2016, pp. 482-486.
-
M. Sybis, K. Wesolowski, K. Jayasinghe, V. Venkatasubramanian and
V. Vukadinovic, "Channel Coding for Ultra-Reliable Low-Latency Communication in 5G Systems," in 2016 IEEE 84th Vehicular Technology Conference (VTC-Fall), IEEE, 2016, pp. 1-5.
-
R. Tanner, "A recursive approach to low complexity codes," IEEE Transactions on Information Theory, vol. 27, pp. 533-547, 1981.