
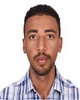
- Open Access
- Authors : Eyuoel Franco , Fitsum Tesfaye
- Paper ID : IJERTV9IS120272
- Volume & Issue : Volume 09, Issue 12 (December 2020)
- Published (First Online): 06-02-2021
- ISSN (Online) : 2278-0181
- Publisher Name : IJERT
- License:
This work is licensed under a Creative Commons Attribution 4.0 International License
Floodplain Inundation Modeling and Mapping for Gilgel Abay River:Nile Basin Ethiopia
Eyuoel Franco1,a*, Fitsum Tesfaye2,b
1Department of Hydraulic Engineering, College of Architecture and Civil Engineering, Addis Ababa Science and Technology University, Addis Ababa, Ethiopia
2Coordinator for Centre of Excellence in Construction Quality and Technology College of Architecture and Civil Engineering, Addis Ababa Science and Technology University, Addis Ababa, Ethiopia
Abstract:- Floods cause serious environmental and economic losses like loss of human life and damage to property. The objective of this research was to model the flow characteristics of Gilgel-Abay River and to develop flood map for the area. Daily discharge and rainfall data were used for HEC-HMS Calibration and Validation with both results showing good match between measured and simulated precipitation data, with acceptable range of coefficient of determination R2 (between 0.7 and 1, Calibration value being 0.72 and Validation 0.73) and Nash-Sutcliffe (NSE) (between 0.5 and 0.75, Calibration value being 0.51 and Validation 0.54). The result of hydrologic model demonstrated peak discharges of 223.9 m3/s, 367.5 m3/s, 438.4 m3/s, 440.5 m3/s and 501.6m3/s for
return periods of 2, 10, 25, 50 and 100 years respectively. According to the flood map generated, flooded area for the return periods 2, 10, 25, 50 and 100 years were 6.2 km2, 7.3 km2, 7.8 km2, 7.9km2 and 8.3 km2 respectively. Flood damage of the area was assessed using depth damage function method and the estimated damage to the agricultural land use with water depth greater than 1m was 32.18 km2. Therefore, non- structural measures like afforestation as well as structural flood protection measures like dikes and levees were recommended to be constructed around the agricultural area.
Key words: HEC-HMS, Flood map, Flood Damage and Gilgel Abay
INTRODUCTION
Flooding is a natural or a manmade phenomenon that is caused due to an overflowing of water over a land surface or river banks, when the volume of water is more than the
carrying capacity of the drainage system [1]. Floods are further classified into two. Flash floods which occur suddenly or unexpectedly are generated from high precipitation that creates excess runoff. And river flood which occurs naturally when the volume of water in water bodies like rivers is increased due to heavy rainfall [2]. Serious environmental and economic losses are caused by flood. Loss of human life, damage to property, destruction of crops, loss of health conditions owning to water borne diseases are major impacts of the phenomena [3]. Ethiopia is one of the east African countries which is severely affected by flooding due to its rainfall characteristics where more than 80% of its annual precipitation falls in four wet month. According to the 2020 year data of NMA, different regions of the country were affected by flood [4]. The objective of this study was to model the flow characteristics of Gilgel-Abay River and to develop flood map for the area by integrating HEC-HMS and HEC-RAS models with ARC-GIS tools ARC-HYDRO, HEC- GEOHMS AND HEC-GEORAS.
MATERIALS AND METHODS
Description of the Study Area: The Gilgel Abay basin is located in north western Ethiopia. Geographical coordinates of the area are 10056 to 11051N latitude and 36044 to 37023E longitudes. It is one of the main sub- basins of the Lake Tana basin. Runoff from the Gilgel Abay contributes about 60% of the flow to the Lake [5].
Fig. 1 Location map of the Gilgel Abay Catchmnet (Source:ASF)
Data Collection and Analysis: The hydrological data was collected from the FDRE ministries of water, irrigation and electricity; Metrological (rainfall and temperature) data was collected from National metrological agency of Ethiopia; the digital elevation model was downloaded from Alaska Satellite Facility (ASF) respectively.
Estimation of Missing Precipitation data: Common methods are station average, normal ratio, quadrant and regression methods. The station average method is the simplest method but may not be accurate when the total annual rainfall at any of the m region gauges differs from the annual rainfall at the point of interest by more than 10% [6]. In this case, the normal ratio
Table 1 List of selected gauging stations used for this study
No. |
Station Name |
Latitude |
Longitude |
Elevation |
Database |
1 |
Adet |
37.49 |
11.27 |
2179 |
1986-2019(33) |
2 |
Dangla |
36.85 |
11.43 |
2116 |
1987-2019(32) |
3 |
Meshenti |
37.28 |
11.47 |
1958 |
1987-2019(32) |
4 |
Merawi |
37.16 |
11.41 |
2000 |
1991-2019(28) |
method is preferred. Due to its simplicity, the normal ratio method was adopted for this study.
() = [( )] =1 |
(1) |
of the rain gauge, significant construction work or change of observational procedure. The method used to check the inconsistency of records is double mass curve analysis.
Determination of Areal Rainfall: Rainfall point that is recorded at a single point must be converted to an aerial rainfall. Thiessen polygon method was used to determine
Where,
is the estimate of missing data for gauged
the areal rainfall. The method is used when rainfall varies from place to place in intensity and duration.
station n, is measured rainfall values of surrounding
= =1 |
(2) |
= =1 |
(2) |
station i, . is normal annual rainfall of station n, is normal annual rainfall of surrounding stations i, is the observed value at station i, and r is a number of surrounding stations.
Adjustment of Rainfall records: There may be lack of consistency of records therefore adjustments may be needed to be done. This may be due to unreported shifting
Where, Ri is the rainfall at station i, Ai is the polygon area of station i, At is total catchment area, and n is the number of stations. The area functions Ai/At are known as the Thiessen coefficients.
Fitting a Flood Probability Distribution: Different probability distribution methods were considered to test the goodness of fit. Normal, Log-normal Log- Pearson type three, Gen. Extreme value and Gumbel distribution methods were estimated. Then sample datas were simulated and fit with statistical distributions by using easy fit software. Goodness of fit tests such as Kolmogorov- Smirnov, Anderson-Darling and Chi-square were conducted at significance level (=0.05) for choosing the best probability distribution [7]. The distribution with minimum statistics value was the value that is best fit with the data.
Terrain Pre-processing using ARC-GIS: Terrain processing is processing of DEM to delineate watersheds. Basic watershed properties such as area, slope, flow length and stream network density are extracted from delineating streams and watersheds.
ARC-HYDRO tool was used for such work. The following procedures: DEM reconditioning, fill snk, flow direction, flow accumulation, stream definition, stream segmentation, catchment grid delineation etc. were done. Then HEC- GEOHMS was used to undergo sub-basin delineation and prepare a number of hydrologic units that were provided as an input for HEC-HMS.
HEC-HMS Modeling: A basin model consisting of three sub basins was generated with Arc-GIS and was setup in HEC-HMS. The components of basin model are classified into four. These are loss Loss determination which is defined as the amount of rainfall infiltrated into the soil, was estimated using SCS curve number method. This was due to the methods capability of calculating precipitation excess as a function of cumulative precipitation, soil cover, land use and antecedent moisture.
( + ) = + |
(3) |
Where Q is accumulated direct runoff, mm, P accumulated rainfall (potential maximum runoff), mm, Ia initial frequency intensity values were found from the Ethiopian Roads Authority drainage manual [8].
abstraction including surface storage, interception, and infiltration prior to runoff, mm and S potential maximum retention, mm.
The relationship between Ia and S was developed from experimental catchment area data. It removes the necessity for estimating Ia for common usage. The empirical relationship used in the SCS runoff equation is:
1000 = 25.4 ( 10) |
(4) |
= 0.2 |
(5) |
By substituting the CN determined from HEC-GeoHMS in equation (4), the potential maximum retention was determined. Then the potential maximum retention was used to determine the initial abstraction using equation (5)
Transform method converts excess precipitation on a sub basin to direct runoff at the sub basin outlet. The SCS unit hydrograph model for direct runoff was chosen due to its simplicity, minimum data requirement, and good performance than the other methods.
Base flow which is an important parameter as it defines a minimum river depth over which additional runoff accumulates was estimated using the constant monthly flow method. This method was used for it is the simplest base flow model in the program and it represents the flow as a constant flow. The initial value before calibration was taken as the average of minimum flow.
The method that was used to route the channel for continuous hydrological modeling was the musk Ingham routing method.
Metrological Model: The metrological modeling succeeded after completing the basin model and so, the specified hyetograph method was chosen for this paper. For evapotranspiration, specified evapotranspiration was used.
Modeling by frequency storm method: With the input from HEC-GeoHMS and some edition from the main HEC-HMS, the model was simulated for rainfall intensity of 2, 10, 50, and 100 year return periods. The
Fig. 2 Rainfall regions and Gilgel Abay Catchment (MOWIE)
Calibration and Validation: HEC-HMS modeling can be performed different time series values. A total of sixteen years historical data from 2002-2017 was used. Two years was used for startup and then calibration was performed for a period of nine years (2004-2012) and validation was executed for a period of five years (2013-2017) on the catchment area using daily flow basis. By using the observed flow, calibration was done both manually and automatically at the outlet of the catchment.
than 50%, good if it is greater than 65% and very good if it is greater than 75% [9].
Model efficiency/performance: Model simulation has been evaluated using efficiency criteria such as coefficient of determination (R2) and [Nash Sutcliff (ENS), 1970]. The R2 coefficient and ENS simulation efficiency measure how well trends in the measured data are reproduced by the simulated results over a specified time period and for a specified time step.
The statistical index of modeling efficiency (ENS) values range from 1.0 (best) to negative infinity. The model simulation can be judged as satisfactory if NSE is greater
( )2 = 1 =1 ( )2 =1 |
(7) |
( )2 = 1 =1 ( )2 =1 |
(7) |
The range of values for R2 is 1.0 (best) to 0.0. Zero
indicates no linear relationship, 1 indicates a perfect positive linear relationship as one variable increases in its values and the other variable also increases in its values through an exact linear rule. Values between 0 and 0.3 indicate a weak linear relationship through a shaky linear rule. Values between 0.3 and 0.7 indicate moderate linear relationship through a fuzzy firm linear rule. Values between 0.7 and 1 indicate a strong linear relationship through a firm linear rule [10].
The Nash Sutcliffe (ENS) efficiency equation is given by:
And the standard R2 is given by:
[ 1( )( )]^2 2 = = ( )2 ( )2 =1 =1 |
(8) |
Where qoi is observed value at the i th time interval, qsi is simulated value at the i time interval qo is average value of the observed discharge and qs is average value of the simulated discharge.
Hydraulic Modeling: Hec-Ras computes water surface elevations at all locations using steady flow simulations or unsteady
flow simulation (routing hydrographs through the system). The data needed to carry out these computations include geometric data, steady flow data or unsteady flow data. The program allows users to determine surface elevations at any point of interest. For this paper the datas used to carry out the computations are geometric data and unsteady flow data. The input data was imported from ARC-GIS [11].
Pre-Ras processing: An interface method was used by HEC-GEORAS to provide a direct link to transfer information between ARC-GIS and HEC-RAS. The model uses geometric attribute data from an existing digital
terrain model (DEM) in TIN format and exports results to HEC-RAS model [11].
2D Spatial features Definition: After the digital terrain representation was generated, the geometric information that was required by Hec-Ras was extracted. The geometric data extracted included the stream center lines, left and right bank lines, flow paths and cross-sections along the streams.
The bank and flow path lines were drawn dependently. Cross-sectional lines drawn crossed stream centerlines exactly once, bank lines exactly twice (left and right) and flow paths exactly three times (left, right and center).
Cross-Section Geometry: Cross section that extends about 30 km was extracted from Google earth and the DEM data. The TIN was also made from the points that were extracted from Google earth and DEM. The channel geometry was best represented by the Google earth data than the DEM.
Fig. 3 Gilgel Abay River Cross-Section taken from Google Earth (2020)
Fig. 4 Gilgel Abay River Cross Section in Google Earth (2020)
Fig. 5 Digitized Gilgel Abay River Scheme with Ras Layers
A total number of 111 cross sections with varying width as well as distance of left and right bank and river center line were drawn along the river. Then the layers stream center line, flow path centerline, flow path lines (left and right), bank lines (left and right) and cross-sections were defined on the TIN layer, according to the data extracted from Google Earth. Finally, 3D spatial data, which identifies the stream network and the HEC-RAS model layout was generated. Then the generated geometric data was exported to HEC-RAS.
Two-Dimensional Modeling: After the geometric data was exported to HEC-RAS, it was opened in RAS-Mapper. The spatial co-ordinate projection of the data was defined and
the DE of the study area was imported to create the terrain model.
The terrain data did not include the actual terrain underneath the water surface in the channel region. This was improved by creating a terrain model of the channel region and combining it to the general surface terrain model. Terrain model of the channel was created using geometric datas: stream centerline, bank line, cross sections and cross sections interpolation surface [11].
Then the base terrain model was combined with the newly created channel only terrain model and a new combined terrain model was created.
(a)
(b)
Fig. 6 a) Original Terrain Model b) New Terrain model with channel data
2D Flow Area Mesh: The main concept of two dimensional modeling is the use of computational mesh. Water elevation at every computational grid cell generated is estimated for a given computational time step. The computational mesh generated depends on the slope of the water surface. If the slope of water surface is flat, larger grid cell sizes are appropriate and if it is steep, smaller grid, cells are used. A 2D flow area polygon, which defines the boundary for computation was drawn. After that, 100*100 size computational mesh was generated [11].
Boundary Conditions: An unsteady state simulation was conducted with the 2D mesh created and 1D geometry data. The most commonly used boundary condition flow
hydrograph, was assigned at the upstream end and the normal depth boundary condition was assigned at the downstream end. A flow hydrograph is the variation of discharge with respect to time. HEC-RAS allows flow hydrograph to be assigned at either upstream or downstream end of the 2D flow area. However, for most applications, the hydrograph reflects the upstream boundary [12].
Discharge data obtained from HEC-HMS was manually added in the flow hydrograph with datas starting time reference. The mannings equation is used by the program to compute the stage based on the flows obtained from the unsteady flow analysis.
-
2 year (b) 100 year
Fig. 7 Flow hydrograph
Flood Inundation Map: Finally, the model was executed by selecting the unsteady flow analysis from the run menu
RESULTS AND DISCUSSION
item on the main HEC-RAS window. A plan was created by selecting the geometry that contains the 2D geometric data and unsteady flow data file to examine the flood event. Depth, velocity and water surface elevation were the significant results obtained from the model.
Consistency and Homogeneity Results of Rainfall Data:
Consistency of Recorded datas was done by double mass curve analysis technique. Accumulated annual values at the station in question are plotted against those that are near and reliable group of stations. If an abrupt deviation in the slope of the curve were observed they should be corrected. However, all the selected stations in this study were consistent therefore there was no need of correction. The homogeneity of precipitation data was checked for the analysis of areal precipitation.
500
450
400
350
300
250
200
150
100
50
0
Consistency Graph
0 500 1000 1500
ADET DANGLA MESHENTI MERAWI
Linear (ADET) Linear (DANGLA) Linear (MESHENTI) Linear (MERAWI) Linear (MERAWI)
Fig. 8 Consistency of Selected Stations
400
350
300
250
200
150
ADET
DANGLA MESHENTI
MERAWI
400
350
300
250
200
150
ADET
DANGLA MESHENTI
MERAWI
100
50
0
100
50
0
0
2
4
6
8
10
12
14
0
2
4
6
8
10
12
14
Fig. 9 Homogeneity Test for Rainfall Stations
Best Fit Probability Distribution: Using easy fit software, the test statistics for Kolmogrov-Smirnov (D), Anderson Darling (A2) and Chi-square test (X2) were estimated for five probability distributions. Probability distribution having the first rank along with their test statistic is presented by the software. Five types of fitting distributions were compared and the statistic with minimum distribution value has been best fitted with the data.
Using Kolmogrov-Smirnov test (D) and Anderson Darling test (A2) test it was observed that the General Extreme value distribution provides good fit to the yearly discharge data at the outlet. Using Chi-squared test (X2), the result showed that normal distribution provides good fit at the outlet. Comparing the three goodness of fit tests, the Kolmogrov-Smirnov test (D) has been found to provide a good fit for selected discharge at the outlet.
Table 2 Goodness of Fit (GOF) Statistics Comparison
Terrain Processing
-
(b)
-
(c) (d)
(e) (f)
Fig. 10 Terrain preprocessing for Gilglel catchment using Arc hydro and HEC-GeoHMS
(a) Raw DEM (b) Fill Sink (c) Flow Direction (d) Catchment Grid Delineation (e) Batch point watershed delineation at the outlet of Gilgel Abay River (f) HMS Legend and Schematic
The output from terrain processing in Arc-Hydro is not only delineation and schematic for the catchment but also extraction of basin characteristics from physical properties of the catchment. Among the basin characteristics soil and land use are the major ones.
Curve number grid was created using Hec-GeoHMS. The application uses merged land use soil type features and CN look up table to create the grid. According to the output of the model, the following parameters were generated.
Table 3 Catchment Characteristics Parameters extracted from Arc-Hydro and Hec-GeoHMS
Components |
Parameters |
Value |
Unit |
Sub-Basin 1 |
CN |
77.18 |
Dimensionless |
Ia |
15 |
[mm] | |
A |
136.9 |
[KM2] | |
Basin Lag |
19.28 |
[min] | |
Sub-Basin 2 |
CN |
81.2 |
Dimensionless |
Ia |
11.75 |
[mm] | |
A |
285.5 |
[KM2] | |
Basin Lag |
14.7 |
[min] | |
Sub-Basin 3 |
CN |
75.6 |
Dimensionless |
Ia |
16.35 |
[mm] | |
A |
722.5 |
[KM2] | |
Basin Lag |
24.5 |
[min] |
HEC-HMS Calibration and Validation: A period of nine years (2004-2012) and five years (2013-2017) were used to calibrate and validate the model respectively. An ENS value of 0.513 and R2 value of 0.72 were determined for calibration and validation values had and ENS 0.54 and R2 0.73.
Both the Calibration and Validation results showed good match between measured and Simulated stream flow and rainfall-data with acceptable range, coefficient of determination (R2) which ranges from 1(best) to 0 and Nash-Sutcliffe (), which ranges from 1(best) to negative infinity.
Table 4 Optimized parameters of HEC-HMS for Gilgel catchment
Component |
Parameter |
Unit |
Initial |
Optimized |
Subbasin-1 |
SCS Curve Number – Curve Number |
77.18 |
58 |
|
Subbasin-1 |
SCS Curve Number – Initial Abstraction |
[mm] |
15 |
127 |
Subbasin-1 |
SCS Unit Hydrograph – Lag Time |
[min] |
19.28 |
19.28 |
Subbasin-2 |
CS Curve Number – Curve Number |
81.2 |
60.9 |
|
Subbasin-2 |
SCS Curve Number – Initial Abstraction |
[mm] |
11.75 |
131 |
Subbasin-2 |
SCS Unit Hydrograph – Lag Time |
[min] |
14.7 |
14.7 |
Subbasin-3 |
SCS Curve Number – Curve Number |
75.6 |
56.7 |
|
Subbasin-3 |
SCS Curve Number – Initial Abstraction |
[mm] |
16.35 |
15.3 |
Subbasin-3 |
SCS Unit Hydrograph – Lag Time |
[min] |
24.5 |
24.5 |
Reach-1 |
Muskingum K |
[hr] |
145 |
145.00 |
Reach-1 |
Muskingum X |
0.015 |
0.01500 |
The model simulation can be judged as satisfactory if NSE is greater than 50%, good if it is greater than 65% and very good if it is greater than 75% [9]. Therefore both values obtained being greater than 50%, show that the simulation is satisfactory. Additionally, the coefficient of determination result show that there is a good agreement between the observed and
simulated flow because both values are greater than 0.7 which indicates a strong linear relationship.
Overall, the results show that the efficiency evaluation criteria parameters resemble each other very well. Therefore, this values determined from calibration and validation of the HEC-HMS model are both acceptable.
Fig. 11 Calibration of HEC-HMS Observation and Simulation Daily Flow Hydrographs
Fig. 12 Validation of HEC-HMS Observation and Simulation Daily Flow Hydrographs
Output of HEC-HMS by Frequency Storm: Values were generated for different flow conditions (return periods) on HEC-HMS. Minimum peak flow occurred for 2 years return period and maximum peak flow
occurred for 100 year return period for 24 hours storm duration. The values obtained were 224 m3/s and 502 m3/s for the minimum and maximum frequency respectively.
Table 5 Peak discharge for different return periods
NO |
Return period |
Peak flow (m3/s) |
1 |
2 |
224 |
2 |
10 |
367 |
3 |
25 |
438 |
4 |
50 |
440 |
5 |
100 |
502 |
Flood Frequency Analysis Results: Finally, the results obtained from HEC-HMS values were then compared with frequency analysis results. The methods were selected according to their goodness of fit obtained from easy fit software.
The software output showed that Gen. Extreme value was best goodness of fit. Then Log-Pearson , Lognormal and Gumbel follow respectively.
The outcome of HEC-HMS values were then compared with output value of different distribution methods which were estimated using their respective formulas to determine magnitude of hydrological event.
Table 6 Comparison of flow values
Distribution Method |
2 |
10 |
25 |
50 |
100 |
Gen. Extreme Value |
354 |
417 |
440 |
455 |
468 |
Log Pearson |
350 |
419 |
449 |
471 |
492 |
Log Normal |
355 |
422 |
450 |
469 |
486 |
Gumbel |
346 |
418 |
454 |
481 |
507 |
HEC-HMS |
224 |
367 |
438 |
440 |
502 |
Flood Mapping on Gilgel-Abay Catchment: After the simulation was complete, the results were displayed in RAS-Mapper. The maximum depth, velocity and water surface elevation of the model were obtained and were exported to ARC-GIS for mapping.
The modeling results of each return period was exported from HEC-RAS to ARC-GIS to generate flood maps. HEC-GEORAS was the tool used for post processing. Bounding polygon and water surface TIN was created from the given profiles and underlying TIN. Water surface TIN was first generated from cross sections and was intersected with the digital terrain model to create the flood plain polygons for flow scenario.
The 2 year storm events flood depth ranges from 0-4.8m with flooded area of 6.2 km2. And the 100 year storm events flood shown has depth value that ranges from 0-6 with flood area of 8.3 km2.
Fig. 13 Two Year Flood Map and Depth for the Study Area
Fig. 14 Hundred Year Flood Map and Depth for the Study Area
Flood Damage: Damage caused by flood depends on several factors such as water depth, flood duration, velocity of flow, sediment concentration and pollution. This research only considered the damages due to flood water depth. The method used to estimate direct flood damage on the agricultural land in Gilgel Abay catchment was the method of depth damage functions. Using this method the estimated damage to the agricultural land use with depth greater than 1m is 32.18 km2.
Conclusion
Flood inundation mapping and modeling for Gilgel-Abay River presents a systematic approach in the preparation of flood mapping and simulation with the application of 2D unsteady flow models and ARC-GIS. The programs used in the research include HEC-RAS, HEC-HMS and ARC-GIS, with its extensions HEC-GEOHMS, HEC-GEORAS and ARCHYDRO for interfacing purpose. The paper is important in providing policy makers with relevant data concerning flood forecasting of the area and also informing them about the performance of different measures that can be adopted to mitigate the scenario and its effects. Catchment delineation and basin characterization were done using the appropriate extensions in ARC-GIS. Peak flood discharge for five return periods was estimated using HEC-HMS. The 2 and 100 year return peak flood discharge were found to be 223.9 and 501.6 m3/s respectively by using ERAs IDF curve, region A2 for analysis of flood on the plain. Flood affected areas were then delineated for 2 years and 100 years return peak flood discharge using HEC-RAS and ARC-GIS. Results showed that the total area affected by the flood was 8.3 km2 and the area affected by 2 year flood inundation of 4.8 m was 6.2km2. The total flood damage for the agricultural area with water depth greater than 1m was estimated to be 32.18 km2. Therefore non-structural and structural mitigation measures like afforestation, levees and dykes were recommended to be constructed around the area.
ACKNOWLEDGEMENTS
First of all, we thank and glorify God Almighty through His Son Jesus Christ for opening this door of education and for helping us from the start till the finish of this research. And we also thank the University of Addis Ababa Science and Technology for their cooperation, allowing us to use resources without payment.
REFERENCES
-
Ohimain, Elijah & Izah, Sylvester & Otobotekere, Dimie. (2014). Selective Impacts of the 2012 Water Floods on the Vegetation and Wildlife of Wilberforce Island, Nigeria. International Journal of Environmental Monitoring and Analysis. 2. 73. 10.11648/j.ijema.20140202.13.
-
Muh A, Marfai: GIS Modeling of River and Tidal Flood Hazards in a Waterfront City Case Study: Semarang City, Central Java, Indonesia. ENSCHEDE, the Netherlands: International Institute for Geo-Information Science and Earth Observation; 2003.
-
Lampros Vasiliades: Hydrological Reponse to Metrological Drought Using the Palmer Drought. Thessaly, Greece; 2009
-
Government of Ethiopia. Joint Government-Humanitarian Partners National Flood Contingency Plan 2020 Kiremt Season. 2020
-
Worqlul, Abeyou & Rientjes, Tom & Gieske, Ambro & Getachew, H.A.. (2009). Ungauged Catchment Contributions to Lake Tana's Water Balance. Hydrological Processes. 23. 3682 – 3693. 10.1002/hyp.7284.
-
WMO. Hydrology-from Measurement to Hydrological Information (Volume 1). Guide to Hydrological Practices. World Metrological Organization; 2008.
-
Alam, Md Ashraful & Farnham, Craig & Emura, Kazuo. (2018). Best-Fit Probability Models for Maximum Monthly Rainfall in Bangladesh Using Gaussian Mixture Distributions. Geosciences (Switzerland). 8. 10.3390/geosciences8040138.
-
Ethiopian Roads Authority. Drainage Design Manual. Addis Ababa, Ethiopia; 2013.
-
Moriasi, Daniel & Arnold, Jeff & Van Liew, Michael & Bingner, Ron & Harmel, R.D. & Veith, Tamie. (2007). Model Evaluation Guidelines for Systematic Quantification of Accuracy in Watershed Simulations. Transactions of the ASABE. 50. 10.13031/2013.23153.
-
Ratner, Bruce. (2009). The correlation coefficient: Its values range between +1/1, or do they?. Journal of Targeting, Measurement and Analysis for Marketing. 17. 10.1057/jt.2009.5.
-
Gary BW, HEC-RAS River Analysis System Hydraulic Reference Manual. Washington DC: US Army Corps of Engineers, Hydrologic Engineering Center, 2016, February, https://www.hec.usace.army.mil/software/hec- ras/documentation/HEC-RAS% 205.0%20Reference%20Manual.pdf
-
Alzahrani, Abdulaziz. (2017). APPLICATION OF TWO- DIMENSIONAL HYDRAULIC MODELING IN RIVERINE SYSTEMS USING HEC-RAS.