
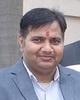
- Open Access
- Authors : Punit Kumar Chaubey
- Paper ID : IJERTV11IS070084
- Volume & Issue : Volume 11, Issue 07 (July 2022)
- Published (First Online): 20-07-2022
- ISSN (Online) : 2278-0181
- Publisher Name : IJERT
- License:
This work is licensed under a Creative Commons Attribution 4.0 International License
How IoT and Big Data Are Driving Smart Traffic Management and Smart Cities
Mr. Punit Kumar Chaubey
Research Scholar, Department of Computer Science Sunrise University, Alwar, Rajasthan
India
Abstract Smart Traffic System (STS) is a one of the important aspect for future smart city. STS is more expensive and highly configurable to provide better quality of service for public traffic management. This paper proposes a low cost future STS to provide better service by deploying traffic update instantly. Low cost vehicle detecting sensors are fixed in the middle of road for every 500 meters. Internet of Things (IoT) is being used to attain public traffic data quickly and send it for data processing. The Real time streaming data is sent for Big Data analytics. There are several analytical scriptures to analyze the traffic density and provide solution through predictive analytics.
Keywords: Internet of Things; Big Data; Smart Cities; Smart Traffic Management System.
-
INTRODUCTION
Internet of Things (IoT) is a recent communication paradigm that envisions a near future, in which the objects of everyday life will be equipped with microcontrollers, transceivers for digital communication, and suitable protocol stacks that will make them able to communicate with one another and with the users, becoming an integral part of the internet [1]. A smart city is an urban improvement vision to incorporate various Internet of Things (IoT) and Information and Communication Technology (ICT) arrangements in a safe style to deal with a city's advantages and benefits incorporate, yet are not constrained to, neighborhood offices data frameworks, schools, libraries, transportation frameworks, clinics, power plants, water supply systems, waste administration, law requirement, and other group administrations [2].
The objective of building a smart city is to enhance personal satisfaction by utilizing innovation to enhance the proficiency of administrations and address occupants' issues. ICT permits city authorities to communicate straight forwardly with the group and the city base and to screen what is going on in the city, how the city is developing, and how to empower a superior personal satisfaction. Using sensors coordinated with ongoing checking frameworks, information is gathered from nationals and gadgets then handled and broke down. The data and information assembled are keys to handling inefficiency.
-
How Big Data and IoT are Being Used in Traffic Management
Traffic management is one of the biggest infrastructure hurdles faced by developing countries today. Developed countries and smart cities are already using IoT and big data to their advantage to minimize issues related to traffic.
The culture of the car has been cultivated speedily among people in all types of nations. In most cities, it is common for people to prefer riding their own vehicles no matter how good or bad the public transportation is or considering how much time and money is it going to take for them to reach their destination.
Thus, the increased use of cars has caused an immense amount of traffic congestion. Several countries are overcoming this traffic bottleneck by fetching information from CCTV feeds and transmitting vehicle-related data to city traffic management centres to help create improvements. Better- organized traffic systems mean a better flow of vehicles on the road, and it means no idling cars, buses, and trucks in traffic jams. All this eventually translates to lower run times, proper utilization of natural resources (gas), and less pollution. The emittance of gases is the largest during stop-start driving, and this happens in spots where traffic is regulated by lights. Hence, if you go for smart traffic, this helps in pollution reduction throughout the entire city.
However, smart traffic management also involves other factors, like smart parking sensors, smart streetlights, smart highways, and smart accident assistance amongst other things.
-
Traffic Lights
Traffic lights that use real-time data feeds are being used to smooth traffic load. Sensors mounted at strategic places can use IoT technology to gather data about high traffic junctions and areas diverting vehicles from these places. Big data can analyze this information further and figure out alternative routes, as well as better traffic signalling to ease congestion. Meanwhile, road-side lights can also work according to weather sensors mounted on them. Dimming of light happens not only as a part of the day-night process but also when weather conditions turn murky. Roadside light sensors can pick up these signals and turn them on and off accordingly.
-
Smart Parking
Parking has become an Achilles heel in the urban planning scenario. Lack of parking spaces, as well as parallel parking, has heightened traffic snarls at important junctions in cities. IoT-based sensors in parking lots can give out real- time information about empty spots to cars coming from a long distance and looking for a parking space. Such sensors have already been installed in European cities like Paris, France, and Kansas in the US. They have all seen remarkable results with a double-digit percentage reduction in parking issues observed in the span of a year.
-
Smart Assistance
-
Road accidents have been one of the top causes of deaths across the world. However, what adds to this gloomy number is the untimely help and assistance to victims in these accidents. CCTVs and sensors on roads can help in locating accident spots and communicating these to the nearest emergency rooms. Once this communication is established in time, all else can be better handled.
-
-
LITERARY REVIEW
According to the United Nations Population Fund, in 2025, 64% of the worlds population lived in urban areas, approximately 5.2 billion people [3]. By 2035, roughly 66%, or 6 billion people will live in urban areas. This not only represents a massive challenge in how we build and manage cities but significant opportunity to improve the lives of billions of people. Rising to that challenge, engineers worldwide are turning to new technology such as the Cyber Physical Systems, 5G and data analytics searching for new approaches and solutions that will improve city transportation, water and waste management, energy usage, and a host of other infrastructure issues that underpin the operation of cities and the lifestyle of urban citizens [4].
According to the research on Smart Cities, the Smart City market is estimated at hundreds of billion dollars by 2025, with an annual spending reaching nearly 23 billion [5]. On the technical side, the most relevant issue consists in the no interoperability of the heterogeneous technologies currently used in city and urban developments. In this respect, the IoT vision can become the building block to realize a unified urban scale ICT platform, thus unleashing the potential of the STS vision [67].
STS vision activity congestion on street systems is only slower speeds, expanded excursion time and expanded lining of the vehicles. At the point when the number of vehicles surpasses the limit of the street, activity congestion happens like metropolitan urban communities. Movement congestion is brought about when the interest surpasses the accessible street limit.
In [8] this paper proposed a strategy for deciding traffic blockage on streets utilizing picture preparing procedures and a model for controlling traffic signals in light of data got from pictures of streets taken by camcorder. Variable traffic cycle and wighted time for every street in view of traffic thickness and control traffic lights in a consecutive way it is very time complex as well as expansive.
Consequently, it is high time to adequately deal with the traffic jam [9] issue. There are different strategies accessible for traffic administration, for example, video information analysis, infrared sensors, inductive circle recognition, remote sensor system, etc. Henceforth another innovation called Radio Frequency Identification (RFID) is presented which can be combined with the current flagging framework that can go about as a key to brilliant traffic administration [10] continuously.
In [11] introduced model called dynamic traffic monitoring system. It using their various parameter factors towards collecting Gathering of high quality travels time and speed. It means that various affecting factors are should counting. In [12] invented that GPS based vehicle tracking
system. It provides to decreasing the short distance traveling. As well as same content in [13] focused on various factors are to be considering timely data acquiescing by using VSNs vector distance routing algorithm. It provides the high reliable communication [14].
-
PROBLEM STATEMENT
All pros become more quantifiable with cons. While IoT and big data present a path-breaking opportunity in smart traffic management and solutions, they also have some limitations. Firstly, current cities already suffer from infrastructure issues like road planning, zoning, and other construction-related issues that could potentially pose problems when implementing IoT technology.
Secondly, all these fancy, hi-tech solutions need high- speed data transfer techniques and, thus, can work only in cities with great internet connectivity. If for any reason, this connectivity is hampered, the entire smart city could fall apart.
Thirdly, the number of devices accessing the central network means more opportunities for hackers to conduct their malicious attacks. An added layer of security, apart from the usual one and another on top of that, will be needed to make an impenetrable hack-proof smart traffic solution. Data privacy will also have to be maintained, looping in lawmakers and engineers.
A Novel Technical support for traffic control system for better emerging traffic management system for smart cities [15]. Deploying booming technologies like Internet of Things and Big data. User friendly App based traffic updates, status of road based vehicle strength etc. interaction provided by using these technologies.
The portability model utilized for regenerations depends on the comfortable driving model (CDM) in [16]. It is a traffic cell robot where space is discredited in units of
0.5 m and time in interims of 1 s. The position and the speed of a vehicle named as n are given by and, individually. The model incorporates expectant impacts by considering the status of the first vehicle's brake light by suspecting its speed and by plan a straightforward activity calculation [17] to execute in the movement framework. The activity focuses are dealt with as autonomous areas. The movement densities of various streets at a specific time as info in light of out information, we have created two yields. Movement Cycle is the aggregate time required for one complete revolution of the sign lights at any activity point.
This strategy is connected for more cycle span when there is more movement so that more vehicles can breathe easy. At the point when there is less movement, the activity cycle is abbreviated with the goal that vehicles don't need to sit tight for a drawn out stretch of time in sign moves. The second parameter is weighted time portion shown in Fig.1
Fig.1 STS Architecture
IoT and Big Data on Smart City
A smart city is classified as a place where citizens live well-organized urban life with the help of information and communication technology (ICT) while maintaining sustainability and causing the least harm to the environment. In greater detail, this means living in a city that plans smart infrastructure and urban services to keep them as efficient as possible. Citizens can easily interact with local bodies, thus, playing a larger role in the citys management.
A smart city is a place where all the citys systems like water management, waste management, healthcare, policing and governance, smart buildings, education, energy, etc. are managed in an optimal fashion that benefits citizens, government, and the environment. Reduction in cost and resource consumption is integral to the layout of a smart city. And, all this is impossible without this offshoot of ICT the IoT technology that can offer governing bodies with real- time solutions for all urban challenges.
What Is IoT?
The Internet of Things or IoT is basically a network of interconnected devices, like sensors and smart devices, that pass on information to each other and a supreme console via the internet. It is that way that we interact with our belongings. All these devices generate data that is so humongous in the amount that it will need hi-tech cloud applications to store, process, and mine. This process is conducted by big data analytics. Any smart city project will use big data to capture, store, process, and analyze large amounts of data generated by several sources and to transform the data into useful knowledge that enables better decision-making processes.
Evaluation Model
In practical approach it consists the three different modules are adding.
Experimental Setup
For experimental setup three different modules are there for overall application designing. Based on below Fig 1 shows deploying technologies on root phase.
Internet of things module
Approach is to completely IoT based vehicle information gathering system. Intel IoT kit with all latest features and vehicle detection sensors. Connected the sensors based on our criteria deploy on road ½ km or 1 km and more it depends best is to deploy very near distance for getting better results.
At least 5 sensors are connected in each other and it communicates to the single IoT kit. All kits are connected to the network access sharing information among the Internet. It continues monitoring for vehicles and updates are sending to the big data storage and analytics.
Big Data Analytics Module
It receives the sensors information with sensor Id. Compute all the information performing analytics operations. Various factors are considering for calculating individual sensor strength and add each other sensor entry and leaving vehicle details road capacity. Etc. factors are considered compute analyzed report are to be produced it make ready through access by using internet either mobile APP or internet browser. Here apply various forms are approaches [18] are connected with latest real-time streaming data processing mechanisms are used shown in Fig.2.
Fig.2 Big Data Analytics Module flow
User Interaction Modules
In this module consists of the latest analytics and decision tools are providing for travelers. Capacity of road number of vehicles are there status everything shown accessing internet. Multiple ways user wants to access the information example mobile APP, internet browser throw enabling GPS on Device, etc. In user point of view very faster interaction and fast data processing are to be done by using background as big data stream analytics.
STS Algorithm
Input:
Red – maximum time of congestion.
Green – maximum time for congestion free network.
Count – minimum frequency of vehicles passing per second stored statically in controllers.
Algorithm:
Signal turns green
While (Timer< green and Timer is not 0) do If (
Count> count)
Keep the signal green.
— Count by 1. Else
if (
Count<= count) Goto 2. End Signal red.
Turn the adjacent signal green. Go to 1. Output:
Effective congestion management
-
DATA AN ANALYSIS METHODOLOGY
We describe the video data and calculation method for traffic volume and vehicle speed in this section. The temporal scope is approximately 9 min of the approximately 1 h and approximately 2 min 30 s of approximately 4 h. The spatial scope is the road near the Hoedong-jeolgaeji and Yet-sicheong in Busan.
CCTV Video Data
The CCTV video data are provided from BUSAN METRO PORITAN CITY, at thirty frames per second. For analysis of the pattern change, we extract pixel data from the video. The extracted data consists of x- and y-coordinates, Hue, Saturation, and Brightness (Value) (HSB or HSV), as shown in TABLE I.
TABLE I. Example of extracted HSV data
X
Y
Hue
Saturation
Value
0
0
13
19
92
0
1
13
20
91
Line Selection
We select lines on a lane for vehicle detection. After we select vertical and horizontal lines like red lines in Figure 1, we extract the HSV data from contact points of the lines. Here, we use only the data value as shown in Figure 2 (c), because there is no pattern change in hue and saturation when a vehicle passes the lines in Figure 2 (a) and (b).
Fig. 1. Example of line and a point of contact selection in image
Vehicle Detection
If a vehicle enters a particular line, it is detected, and the data is checked for the pattern until a vehicle escapes last line. The check process is as follow.
Similarity of average of Value when a vehicle passed. The number of frames changed when a vehicle passes.
First, a contact point is compared by using two conditions. Then, if one of them is satisfied, we judge the same vehicle. For example, if a vehicle enters the Line1 as shown in Figure 3, then compare A of Line1 with A of Line2. After that, if both conditions are satisfied, then we compare the next contact point in B-B, C-C, and D-D order. If all If all contact points satisfy the two conditions, then we compare all contact points of Line2 with Line3. Finally, we check whether it is the same vehicle by comparing all contact points of Line3 with Line4.
Example of change of Hue
Example of change of Saturation
Example of change of Value Fig. 2. Example of change of HSV
Fig. 3. A method of the vehicle dection
Further, if lines and points of the contact extend as shown in Figure 4, it is possible to detect vehicles that turn right and left on the intersection. This requires a method that can process large amount data.
distance between Linei and Linej. Further, the parameter S represents the number of frames that changed when a vehicle passed. Finally, the vehicle speed is calculated as per equation (1).
-
RESULT ANALYSIS
(1)
Fig. 4. Example of lines and points of contact on the
intersection
Algorithm for the Compare between Points of Contact
The input data are the value data list of the first and second points in the process. The first procedure compares the similarity of the average value when a vehicle passed between the points of contact (line 5 of Algorithm 1). The second procedure compares the number of frames changed when a vehicle passes between the points of contact (line 6 of Algorithm 1). The third procedure compares the number of up and down inflection points as a value of when a vehicle does not pass between the points of contact (line 7 of Algorithm 1). Finally, after checking whether one of the three element satisfy the condition, the result of comparison between the points of contact is returned (line 8-15 Algorithm 1). The method for calculating traffic volume is as follows. If step is C completed, then increase the traffic volume.
Algorithm 1. Compare between points of contact
Input: FST_PL, SEC_PL // FST_PL is the Value data list of first point and SEC_PL is the Value data list of second point
Output: CR// the result of compare points of contact based on the Value change
compare_result=FALSE; similar_result=FALSE; frameCount_reuslt=FALSE; updownCount_result=FALSE;
similar_result = compareSimilarValue(FST_PL, SEC_PL);
frameCount_result = compareFrameCount(FST_PL, SEC_PL);
updown_result = compareUpDownCount(FST_PL, SEC_PL); if similar_result is TRUE then
compare_result = TRUE;
else if frameCount is TRUE then compare_result = TRUE;
else if upDownCount is TRUE then compare_result = TRUE;
end if
CR = compare_result; return CR
E. Vehicle Speed Measurement
The vehicle speed, which is denoted by equation (1), is as follows. The parameter S represents the distance from
entering first Linei to last Linei. The distance was referred to standard of lanes[6], and the distance rate per pixel was calculated for lane in image. After that, it calculated the
We calculated traffic volume and vehicle speed based on a method of analysis. The target is the urban highway and the road on the city. The result of the traffic volume verified by comparing the actual calculated result with the result calculated after observing through the naked eye of the CCTV video and vehicle speed were verified using vehicle speed information of NAVER Map Web page in Korea.
-
Analysis of the Result on the urban highway
Figure 5-(a) shows the results for 9 min, which detected 43 vehicles of the total 48 vehicles. The five missed vehicles cannot be detected because Values of the front and back in a container truck are different.
Figure 6-(a) shows the result of vehicles speed when the time scope is 9 min. Although it could not check the speed information in the past, the vehicles average speed showed approximately 65 km/h ~ 75 km/h on average in the case of urban highways which corresponded with the experimental data. The result of the vehicular speed we calculated approximately 69.15 km/h showed similarities in comparison with the real vehicles average speed.
-
Analysis of the Result on the Road of the City
The result of the Figure 5-(b) shows it detected all ten vehicles except the vehicles entering different lanes.
Figure 6-(b) shows the result of the vehicular speed for the road of the city. The vehicles average speed of this road showed 35 km/h ~ 45 km/h which corresponded with the experimental data, and the result about 44.14 km/h showed similarities in comparison with the real vehicles average speed.
-
The result on the urban highway
-
The result on the road of the city Fig. 5. The result on comparison of real traffic volume
-
and calculated traffic volume
-
The result on the urban highway
-
The result on the road of the city Fig. 6. The result of vehicles speed
-
-
-
CONCLUSION
The proposed system discussed about a low cost STS to provide better service by deploying traffic indicators to update the traffic details instantly. Low cost vehicle detecting sensors are shown in the middle of road for every
500 meters. IoT are being used to acquire traffic data quickly and send it for processing. The streaming data is sent for Big Data analytics. There are several analytical scriptures to analyze the traffic density and provide solution through predictive analytics. Moreover, our approach is provided a better result while comparing to the existing systems. In future advanced sensors used for detecting nature of capacity of vehicle using big data analytics to create more flexible to travelers.
Traffic is a crucial aspect that determines a citys liability factor and efficiency status. Population surge will stop mattering if data and sensors are used capably to manage traffic. As smart cities evolve and increase in number in the coming years, IoT and big data will play a key role in the development and integration of serices and infrastructure. With passing time, other issues besides traffic, like waste management, energy conservation, etc., will greatly benefit from the growth of IoT and big data.
The preferred spelling of the word acknowledgment in America is without an e after the g. Avoid the stilted
expression one of us (R. B. G.) thanks …. Instead, try R.
B. G. thanks…. Put sponsor acknowledgments in the unnumbered footnote on the first page.
-
REFERENCES
doi:10.1016/j. compeleceng.2017.09.016
[16] Y. Tian and L. Pan, Predicting Short-Term Traffic Flow by Long Short-Term Memory Recurrent Neural Network, 2015 IEEE International Conference on Smart City/SocialCom/ SustainCom (SmartCity), Dec. 2015. [17] Arulmurugan, R., Sabarmathi, K. R., & Anandakumar, H. (2017). Classification of sentence level sentiment analysis using cloud machine learning techniques. Cluster Computing. doi:10.1007/s10586-017- 1200-1 [18] Imawan, F. Putri, and J. Kwon, TiQ: A Timeline Query Processing System over Road Traffic Data, 2015 IEEE International Conference on Smart City/SocialCom/SustainCom (SmartCity), Dec. 2015.