
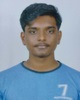
- Open Access
- Authors : Dandala Bharath Reddy , Doma Akhil Sai , Guggilla Akash Nagaraju
- Paper ID : IJERTV10IS110003
- Volume & Issue : Volume 10, Issue 11 (November 2021)
- Published (First Online): 08-11-2021
- ISSN (Online) : 2278-0181
- Publisher Name : IJERT
- License:
This work is licensed under a Creative Commons Attribution 4.0 International License
Identification of Hate Content on Social Media
Dandala Bharath Reddy
Dept of Information Technology ACE Engineering college Ghatkesar, India
Spotting and Treating
Guggilla Akash Nagaraju Dept of Information Technology ACE Engineering College Ghatkesar,India
Doma Akhil Sai
Dept of Information Technology ACE Engineering College Ghatkesar, India
Abstract As online substance keeps on developing, so does the spread of disdain discourse. We distinguish and look at difficulties looked by online programmed approaches for disdain discourse recognition in text. Among these hardships are nuances in language, varying definitions on what establishes disdain discourse, and impediments of information accessibility for preparing and testing of these frameworks. Besides, numerous new methodologies experience the ill effects of an interpretability issuethat is, it tends to be hard to comprehend the reason why the frameworks settle on the choices that they do. We propose a multi-view SVM approach that accomplishes close to cutting edge execution, while being more straightforward and delivering more effectively interpretable choices than neural strategies. We additionally talk about both specialized and down to earth difficulties that stay for this errand.
Keywords Hate speech Detection ; Machine Learning
;Natural Language Processing ;Logistic Regression ;TF-IDF; Web Scrapping/Datasets
I. INTRODUCTION
Disdain violations are sadly the same old thing in the public arena. Notwithstanding, web-based media and different method for online correspondence have started assuming a bigger part in disdain wrongdoings. For example, suspects in a few late disdain related dread assaults had a broad online media history of disdain related posts, proposing that web- based media adds to their radicalization.
Now and again, web-based media can assume a much more straightforward part; video film from the suspect of the 2019 dread assault in Christchurch, New Zealand, was communicated in real time on Facebook. Tremendous internet based correspondence gatherings, including web- based media, empower clients to communicate their thoughts openly, now and again, secretly.
While the capacity to uninhibitedly articulate one's thoughts is a basic freedom that ought to be appreciated, instigating and spreading disdain towards another gathering is a maltreatment of this freedom. For example, The American Bar Association declares that in the United States, disdain discourse is lawful and ensured by the First Amendment,
albeit not on the off chance that it straightforwardly calls for viciousness .
In that capacity, numerous web-based discussions, for example, Facebook, YouTube, and Twitter consider disdain discourse hurtful, and have approaches to eliminate disdain discourse content . Because of the cultural concern and how inescapable disdain discourse is becoming on the Internet , there is solid inspiration to concentrate on programmed recognition of disdain discourse. Via computerizing its location, the spread of scornful substance can be diminished.
Contending definitions give difficulties to assessment of disdain discourse recognition frameworks; existing datasets contrast in their meaning of disdain discourse, prompting datastets that are from various sources, yet additionally catch distinctive data. This can make it hard to straightforwardly get to which parts of disdain discourse to distinguish. We talk about the different datasets accessible to prepare and gauge the presentation of disdain discourse identification frameworks in the following segment. Subtlety and nuances in language give further difficulties in programmed disdain discourse distinguishing proof, again relying upon the definition.
Disregarding contrasts, some new procedures found promising results for recognizing scorn talk in printed content
. The proposed courses of action use AI strategies to portray text as scorn talk. One limitation of these approaches is that the decisions they make can be foggy and difficult for individuals to unravel why the decision was made. This is a rational worry since structures that therefore alter a singular's talk presumably need a manual appeal process. To determine this issue, we propose another contempt talk portrayal approach that thinks about a prevalent cognizance of the decisions and show that it can even beat existing techniques on some datasets. A part of the current philosophies use outside sources, for instance, a hatred talk jargon, in their systems. This can be convincing, but it requires staying aware of these sources and keeping awake with the most recent which is an issue in itself. Here, our technique doesn't rely upon outside resources and achieves reasonable accuracy. We cover these subjects in the going with region.
As a general rule, notwithstanding, there are useful difficulties that stay among all frameworks. For example, outfitted with the information that the stages they use are attempting to quietness them, those looking to spread scornful substance effectively attempt to find ways of going around measures set up. We cover this point in more detail in the last segment.
In outline, we talk about the difficulties and approaches in programmed recognition of disdain discourse, including contending definitions, dataset accessibility and development, and existing methodologies. We additionally propose another methodology that now and again beats the cutting edge and examine remaining weaknesses. At last, we finish up the accompanying:
-
Automatic disdain discourse identification is actually troublesome;
-
Some methodologies accomplish sensible execution;
-
Specific difficulties stay among all arrangements;
-
Without cultural setting, frameworks can't sum up adequately.
-
Defining disdain discourse
The meaning of disdain discourse is neither generally acknowledged nor are individual aspects of the definition completely settled upon. Ross, et al. accept that a reasonable meaning of disdain discourse can help the investigation of distinguishing disdain discourse by making clarifying disdain discourse a simpler errand, and subsequently, making the explanations more solid . Nonetheless, the line between disdain discourse and suitable free articulation is foggy, making some attentive to give disdain discourse an exact definition. For example, the American Bar Association doesn't give an authority definition, however rather affirms that discourse that adds to a criminal demonstration can be rebuffed as a component of a disdain wrongdoing . Likewise, we pick not to propose a particular definition, yet rather inspect existing definitions to acquire experiences into what normally establishes disdain discourse and what specialized difficulties the definitions may bring. We sum up driving meanings of disdain discourse from differing sources, just as certain parts of the definitions that make the identification of disdain discourse troublesome.
-
Encyclopedia of the American Constitution: "Disdain discourse is discourse that assaults an individual or gathering based on properties like race, religion, ethnic beginning, public beginning, sex, incapacity, sexual direction, or sex personality."
-
Facebook: "We characterize disdain discourse as an immediate assault on individuals dependent on what we call ensured qualitiesrace, nationality, public beginning, strict connection, sexual direction, station, sex, sex, sex personality, and genuine infection or handicap. We additionally give a few securites to migration status. We characterize assault as savage or dehumanizing discourse, articulations of mediocrity, or calls for rejection or isolation."
-
Twitter: "Contemptuous lead: You may not advance savagery against or straightforwardly assault or undermine others based on race, identity, public beginning, sexual direction, sex, sex personality, strict association, age, handicap, or genuine infection."
-
Davidson et al.: "Language that is utilized to communicates scorn towards a designated bunch or is expected to be disparaging, to embarrass, or to affront the individuals from the gathering."
-
de Gilbert et al.: "Disdain discourse is an intentional assault coordinated towards a particular gathering of individuals persuaded by parts of the gathering's personality."
-
Fortuna et al. "Disdain discourse is language that assaults or reduces, that induces brutality or disdain against gatherings, in light of explicit attributes like actual appearance, religion, drop, public or ethnic beginning, sexual direction, sex personality or other, and it can happen with various etymological styles, even in unpretentious structures or when humor is utilized.". This definition depends on their examination of different definitions.
It is remarkable that in a portion of the definitions over, a vital condition is that it is coordinated to a gathering. This contrasts from the Encyclopedia of the American Constitution definition, where an assault on an individual can be viewed as disdain discourse. A typical topic among the definitions is that the assault depends on some part of the gathering or people groups character. While in de Gilbert's definition the actual personality is left dubious, a portion of different definitions give explicit character attributes. Specifically, ensured attributes are parts of the Davidson et al. what's more, Facebook definitions. Fortuna et al's. definition explicitly calls out varieties in language style and nuances. This can be testing, and goes past what regular text- based grouping approaches can catch.
Fortuna et al's. definition depends on an investigation of the accompanying qualities from different definitions:
-
Hate discourse is to prompt savagery or disdain
-
Hate discourse is to assault or reduce
-
Hate discourse has explicit targets
-
Whether humor can be viewed as disdain discourse
A specific issue not covered by numerous definitions identify with authentic proclamations. For instance, "Jews are pig" is obviously disdain discourse by most definitions (it is an assertion of mediocrity), yet "Numerous Jews are legal advisors" isn't. In the last case, to decide if every assertion is disdain discourse, we would have to check if the assertion is authentic utilizing outside sources. This kind of disdain discourse is troublesome on the grounds that it identifies with certifiable truth checkanother troublesome undertaking. All the more along these lines, to assess legitimacy, we would at first need to characterize exact word translations, to be specific, is "many" a flat out number or by relative level of the populace, further entangling the check.
Another issue that emerges in the meaning of disdain discourse is the potential applauding of a gathering that is contemptuous. For instance, lauding the KKK is disdain discourse, but applauding another gathering can obviously be non disdain discourse. For this situation realize what gatherings are disdain gatherings and what precisely is being lauded about the gathering as some applauding is without a doubt, and shockingly, valid. For instance, the Nazis were exceptionally productive as far as their "Last Solution". In this manner, acclaim handling alone is, now and again, troublesome.
-
-
Datasets
Gathering and explaining information for the preparation of programmed classifiers to recognize disdain discourse is testing. In particular, distinguishing and concurring whether explicit text is disdain discourse is troublesome, according to recently referenced, there is no all inclusive meaning of disdain discourse. Ross, et al. concentrated on the unwavering quality of disdain discourse comments and recommend that annotators are problematic. Arrangement between annotators, estimated utilizing Krippendorff's , was extremely low (up to 0.29). Be that as it may, they analyzed explanations dependent on the Twitter definition, versus comments dependent on their own viewpoints and tracked down a solid relationship.
Moreover, web-based media stages are a hotbed for disdain discourse, yet many have exceptionally severe information utilization and dispersion strategies. This outcomes in a moderately modest number of datasets accessible to people in general to consider, with generally coming from Twitter (which has a more merciful information use strategy). While the Twitter assets are important, their overall relevance is restricted because of the novel sort of Twitter posts; the person impediment brings about curt, short-structure text. Conversely, posts from different stages are ordinarily longer and can be essential for a bigger conversation on a particular point. This gives extra setting that can influence the importance of the text.
Another challenge is that there simply are not many publicly- available, curated datasets that identify hateful, aggressive, and insulting text.
-
HatebaseTwitter . One Twitter dataset is a bunch of 24,802 tweets given by Davidson, et al. Their
methodology for making the dataset was as per the following. First they took a disdain discourse vocabulary from Hatebase and looked for tweets containing these terms, bringing about a bunch of tweets from around 33,000 clients. Next they took a course of events from this load of clients bringing about a bunch of approximately 85 million Tweets. From the arrangement of around 85 million tweets, they took an irregular example, of 25k tweets, that contained terms from the vocabulary. Through publicly supporting, they commented on each tweet as disdain discourse, hostile (however not disdain discourse), or neither disdain discourse nor hostile. If the understanding between annotators was too low, the tweet was avoided from the set. A usually utilized subset of this dataset is additionally accessible, containing 14,510 tweets.
-
WaseemA. Waseem and Hovy likewise give a dataset from Twitter, comprising of 16,914 tweets named as bigoted, chauvinist, or not one or the other. They originally made a corpus of around 136,000 tweets that contain slurs and terms identified with strict, sexual, sex, and ethnic minorities. From this corpus, the actual creators explained (named) 16,914 tweets and had a sex concentrates on significant survey the comments.
-
WaseemB . In a subsequent paper, Waseem makes another dataset by examining another arrangement of tweets from the 136,000 tweet corpus. In this assortment, Waseem enlisted women's activists and hostile to prejudice activists alongside publicly supporting for the comment of the tweets. The marks in that are bigoted, chauvinist, neither or both.
-
Stormfront . de Gilbert, et al. give a dataset from posts from a racial oppressor gathering, Stormfront. They clarify the posts at sentence level bringing about 10,568 sentences marked with Hate, NoHate, Relation, or Skip. Disdain and NoHate names show presence or deficiency in that department, individually, of disdain discourse in each sentence. The mark "Connection" demonstrates that the sentence is disdain discourse when it is joined with the sentences around it. At long last, the name "skip" is for sentences that are non-English or not containing data identified with disdain or non- disdain discourse. They additionally catch the measure of setting (i.e., past sentences) that an annotator used to arrange the message.
-
TRA. The 2018 Workshop on Trolling, Aggression, and Cyberbullying (TRAC) facilitated a common undertaking zeroed in on distinguishing forceful text in both English and Hindi. Forceful text is frequently a part of disdain discourse. The dataset from this assignment is accessible to people in general and contains 15,869 Facebook remarks marked as clearly forceful, clandestinely forceful, or non-forceful. There is likewise a little Twitter dataset, comprising of 1,253 tweets, which has similar marks.
-
HatEval. This dataset is from SemEval 2019 (Task
5) for contest on multilingual identification of disdain focusing to ladies and foreigners in tweets. It comprises of a few arrangements of marks. The first shows whether the tweet communicates disdain towards ladies or foreigners, the second, regardless of whether the tweet is forceful, and the third, whether the tweet is aimed at an individual or a whole gathering. Note that focusing on an individual isn't really viewed as disdain discourse by all definitions.
-
Kaggle Kaggle.com facilitated a common errand on recognizing offending remarks. The dataset comprises of 8,832 online media remarks named as annoying or not annoying. While not really disdain discourse, offending text might demonstrate disdain discourse.
-
GermanTwitter. As a feature of their investigation of annotator unwavering quality, Ross, et al. made a Twitter dataset in German for the European evacuee emergency. It comprises of 541 tweets in German, named as communicating disdain or not.
Note that these datasets fluctuate extensively in their size, scope, qualities of the information clarified, and attributes of disdain discourse considered. The most well-known wellspring of text is Twitter, which comprises of short- structure online posts. While the Twitter datasets do catch a wide assortment of disdain discourse viewpoints in a few unique dialects like assaulting various gatherings, the development cycle including the separating and examining strategies present uncontrolled components for investigating the corpora. Besides, corpora developed from web-based media and sites other than Twitter are uncommon, making examination of disdain discourse hard to cover the whole scene.
There is likewise the issue of awkwardness in the quantity of disdain and not disdain texts inside datasets. On a stage like Twitter, disdain discourse happens at an extremely low rate contrasted with non-disdaindiscourse. Despite the fact that datasets mirror this irregularity to a degree, they don't plan the real rate because of preparing needs. For instance, in the WaseemA dataset], 20% of the tweets were marked chauvinist, 11.7% bigot, and 68.3% not one or the other. For this situation, there is as yet an irregularity in the quantity of chauvinist, bigot, or neither tweets, yet it may not be just about as imbalanced true to form on Twitter.
-
-
-
Automatic approaches for disdain discourse discovery Most online media stages have set up client decides that preclude disdain discourse; upholding these principles, notwithstanding, requires overflowing difficult work to survey each report. A few stages, like Facebook, as of late expanded the quantity of content mediators. Programmed instruments and approaches could speed up the exploring system or dispense the human asset to the posts that require close human assessment. In this segment, we outline programmed approaches for disdain discourse location from text.
-
Keyword-based methodologies
A fundamental methodology for recognizing disdain discourse is utilizing a watchword based methodology. By utilizing a metaphysics or word reference, text that contain possibly derisive watchwords are recognized. For example, Hatebase keeps an information base of injurious terms for some gatherings across 95 dialects. Such all around kept up with assets are important, as phrasing changes over the long haul. Notwithstanding, as we saw in our investigation of the meanings of disdain discourse, basically utilizing a scornful slur isn't really enough to establish disdain discourse..
Catchphrase based methodologies are quick and clear to comprehend. Be that as it may, they have serious restrictions. Distinguishing just racial slurs would bring about an exceptionally exact framework however with low review where accuracy is the level of important from the set recognized and review is the percent of significant from inside the worldwide populace. As such, a framework that depends essentially on watchwords would not recognize contemptuous substance that doesn't utilize these terms. Interestingly, including terms that could yet are not generally contemptuous (e.g., "junk", "pig", and so forth) would make an excessive number of bogus cautions, expanding review to the detriment of accuracy.
Besides, watchword based methodologies can't recognize disdain discourse that doesn't have any derisive catchphrases (e.g., metaphorical or nuanced language). Shoptalk, for example, "assemble that divider" in a real sense implies building an actual boundary (divider). In any case, with the political setting, some decipher this is a judgment of some moves in the United States.
-
Source metadata
Extra data from web-based media can help further comprehend the attributes of the presents and possibly lead on a superior distinguishing proof methodology. Data like socioeconomics of the posting client, area, timestamp, or even friendly commitment on the stage would all be able to give further comprehension of the post in various granularity.
Notwithstanding, this data isn't frequently promptly accessible to outside analysts as distributing information with delicate client data raises protection issues. Outer scientists may just have part or even none of the client data. Subsequently, they conceivably tackle some unacceptable riddle or take in dependent on off-base information from the information. For example, a framework prepared on these information may normally inclination towards hailing content by specific clients or gatherings as disdain discourse dependent on coincidental dataset attributes.
Utilizing client data conceivably raises some moral issues. Models or frameworks may be one-sided against specific clients and habitually banner their posts as derisive regardless of whether some of them are not. Additionally, depending a lot on segment data could miss posts from clients who don't commonly post scornful substance. Hailing posts as disdain
dependent on client insights could make a chilling impact on the stage and in as far as possible right to speak freely.
-
Machine learning classifiers
AI models take tests of named text to deliver a classifier that can identify the disdain discourse dependent on names commented on by content commentators. Different models were proposed and demonstrated fruitful before. We portray a determination of publicly released frameworks introduced in the new examination.
a. Content preprocessing and feature selection.
To recognize or arrange client created content, text highlights demonstrating disdain should be separated. Clear components are individual words or expressions (n-grams, i.e., arrangement of n continuous words). To work on the coordinating of components, words can be stemmed to get just the root eliminating morphological contrasts. Metaphore handling, e.g., Neuman, et. al. in like manner can separate elements.
The pack of-words supposition that is usually utilized in text arrangement. Under this suspicion, a post is addressed just as a bunch of words or n-grams with practically no requesting. This suspicion surely discards a significant part of dialects yet by and by demonstrated incredible in various errands. In this setting, there are different ways of relegating loads to the terms that might be more significant, like TF-IDF.
Other than distributional provisions, word embeddings, i.e., relegating a vector to a word for example, word2vec, are normal while applying profound learning techniques in regular language handling and text mining. Some profound learning designs, for example, repetitive and transformer neural organizations, challenge the pack of-words supposition by demonstrating the requesting of the words by handling over an arrangement of word embeddings.
-
Hate speech detection approaches and baselines.
Guileless Bayes, Support Vector Machine and Logistic Regression. These models are regularly utilized in text arrangement. Innocent Bayes models name probabilities straightforwardly with the supposition that the components don't communicate with each other. Backing Vector Machines (SVM) and Logistic Regression are straight classifiers that anticipate classes dependent on a mix of scores for each element. Open-source executions of the these models exist, for example in the notable Python AI bundle sci-unit learn.
Davidson, et al. Davidson, et al. proposed a cutting edge highlight based characterization model that consolidates distributional TF-IDF highlights, grammatical form labels, and other semantic elements utilizing support vector machines. The joining of these semantic elements recognizes disdain discourse by recognizing various uses of the terms,
yet experiences a few nuances, like when regularly hostile terms are utilized from an uplifting outlook (e.g., eccentric in "He's a damn decent entertainer. As a gay man, it's great to see a transparently eccentric entertainer given the lead job for a significant film.", from HatebaseTwitter dataset.
Neural Ensemble. Zimmerman, et al. propose a group approach, which joins the choices of ten convolutional neural organizations with various weight instatements. Their organization structure is like the one proposed by, with convolutions of length 3 pooled over the whole archive length. The aftereffects of each model are consolidated by averaging the scores, similar to.
FastText. FastText is an effective characterization model proposed by analysts in Facebook. The model produces embeddings of character n-grams and gives expectations of the model dependent on the embeddings. Over the long haul, this model has turned into a solid pattern for some text order undertakings.
-
BERT
BERT is a new transformer-based pre-prepared contextualized installing model extendable to a characterization model with an extra yield layer. It accomplishes cutting edge execution in text characterization, question addressing, and language deduction without considerable assignment explicit adjustments. At the point when we explore different avenues regarding BERT, we add a direct layer on the order token, and test all recommended tuning hyperparameters
C-GRU. C-GRU, a Convolution-GRU Based Deep Neural Network proposed by Zhang, et al., consolidates convolutional neural organizations (CNN) and gated repetitive organizations (GRU) to identify disdain discourse on Twitter. They direct a few assessments on openly accessible Twitter datasets exhibiting their capacity to catch word grouping and request in short text. Note, in the HatebaseTwitter dataset, they treat both Hate and Offensive as Hate bringing about twofold mark rather than its unique multi-class name. In our assessment, we utilize the first multi-class marks where distinctive model assessment results are normal..
-
Our proposed classifier: Multi-view SVM
We propose a multi-view SVM model for the order of disdain discourse. It applies a various view stacked Support Vector Machine (mSVM). Each sort of component (e.g., a word TF- IDF unigram) is fitted with an individual Linear SVM classifier (backwards regularization steady C = 0.1), making a view-classifier for those elements. We further consolidate the view classifiers with another Linear SVM (C = 0.1 to deliver a meta-classifier. The components utilized in the meta- classifier are the anticipated likelihood of each name by each view-classifier. That is, if we have 5 sorts of elements (e.g., character unigram to 5-gram) and 2 classes of marks, 10 elements would fill in as contribution to the meta-classifier.
.
Consolidating AI classifiers is definitely not another idea. Past endeavors have shown that consolidating SVM with various classifiers gives upgrades to different information
mining undertakings and text grouping. Consolidating numerous SVMs (mSVMs) has additionally been demonstrated to be a compelling methodology in picture handling undertakings for lessening the huge dimensionality issue.
.
In any case, applying numerous SVMs to distinguish disdain discourse extends the space of utilization for such arrangement past that recently investigated. Multi-view learning is known for catching various perspectives on the information. With regards to loathe discourse location, joining various perspectives catches varying parts of disdain discourse inside the order interaction. Rather than joining all provisions into a solitary component vector, each view- classifier figures out how to order the sentence dependent on just one sort of element. This permits the view-classifiers to get various parts of the example separately.
Coordinating all element types in a single model, by regularization, hazards the veiling of somewhat frail however key signs. For instance, "yellow" and "individuals" independently would show up a larger number of times than "yellow individuals" consolidated; posts having these terms exclusively are probably not going to be disdain. In any case, "yellow individuals" is reasonable disdain discourse (particularly when other disdain discourse perspectives are available), yet the sign may be uncommon in the assortment, and hence, is logical covered by the regularization if all components are consolidated together. For this situation, mSVM can get this element in one of the view-classifiers, where there are less boundaries.
Besides, this model offers the chance to decipher the model to recognize which view-classifier contributes most through the meta-classifier gives human instinct to the arrangement. The view-classifier contributing most to an official conclusion distinguishes key jargon (highlights) bringing about a disdain discourse mark. This differences with well- performing neural models, which are frequently murky and hard to comprehend. Indeed, even best in class strategies that utilize self-consideration experience the ill effects of impressive commotion that immensely decreases interpretability.
-
Experimental setup
Utilizing various disdain discourse datasets, we assessed the exactness of existing just as our disdain discourse discovery draws near.
-
Data preprocessing and features.
For straightforwardness and consensus, preprocessing and highlight recognizable proof is deliberately negligible. For pre-handling, we apply case-collapsing, tokenization, and accentuation expulsion (while keeping emoticon). For highlights, we basically remove word TF-IDF from unigram to 5-gram and character N-gram counts from unigram to 5- gram.
-
Datasets
We assess the methodology on the Stormfront, TRAC, HatEval, and HatebaseTwitter datasets recently depicted. These datasets give an assortment of disdain discourse definitions and viewpoints (counting different sorts of hostility), and various kinds of online substance (counting on the web gatherings, Facebook, and Twitter content). For Stormfront, we utilize the fair train/test split proposed in, with an arbitrary determination of 10% of the preparation set held out as approval information. For the TRAC dataset, we utilize the English Facebook preparing, approval, and test parts gave by. For HatEval, we utilize a split of the preparation set for approval and utilize the authority approval dataset for testing on the grounds that the authority test set isn't public. At long last, for the HatebaseTwitter dataset, we utilize the standard train-approval test split.
-
Evaluation
We assess the presentation of each approach utilizing exactness and full scale arrived at the midpoint of F1 score. There are not an agreement in writing concerning which assessment measurements to utilize. Notwithstanding, we accept that zeroing in on both exactness and large scale F1 offers great experiences into the overall qualities and shortcomings of each approachExperimental results
We report the most elevated score of the methodologies depicted above on each dataset in Table. Complete assessment results are accessible in supporting archive Table (counting exactness breakdown by mark).
In the Stormfront and TRAC datasets, our proposed approach gives cutting edge or cutthroat outcomes for disdain discourse location. On Stormfront, the mSVM model accomplishes 80% exactness in recognizing disdain discourse, which is a 7% improvement from the best distributed earlier work (which accomplished 73% precision). BERT performs 2% better than our methodology, however the interpretability of the choices the BERT model made are hard to clarify.
On the TRAC dataset, our mSVM approach accomplishes 53.68% full scale F1 score. Note that through streamlining on the approval set, we found that utilizing TF-IDF loads for character N-grams works better on Facebook dataset, so we report results utilizing those TF-IDF rather than crude counts. This outflanks any remaining methodologies we tried different things with, including the solid BERT framework. We additionally contrasted our methodology with different frameworks that took an interest in the common undertaking, and saw that we outflank them too as far as the metric they announced (weighted F-score) by 1.34% or higher. This is especially great in light of the fact that our methodology beat frameworks which depend on outer datasets and information expansion systems..
Our methodology outflanked the highest level outfit technique by 3.96% as far as exactness and 2.41% as far as F1. This shows that mSVM gains from various perspectives
and jam more signals when contrasted with a basic outfit strategy that utilizes all elements for every first-level classifier. BERT accomplished 3% lower as far as precision and 1% lower as far as F1 than our proposed technique and still gave negligible interpretability, exhibiting that renouncing interpretability doesn't really give higher exactness. For HatEval and HatebaseTwitter, the neural outfit approach outflanks our technique proposing that neural methodologies are more qualified for Twitter information than mSVM-based arrangement. Past works announced different measurements, for example a help weighted F1 in Davidson, et. al., making examination between models troublesome. We report large scale F1 to alleviate the impact of irregularity between classes, which is an impact that has been prepared in during the development of the datasets. For a reasonable and complete correlation between the frameworks, we execute the frameworks from the past works and compute full scale F1 on the datasets announced in this review. The past best exhibition on the Stormfront dataset utilized a repetitive neural organization to accomplish an exactness of 0.73; our methodology effectively beats this technique. On the TRAC dataset, others revealed a weighted F1 execution of 0.6425 utilizing a repetitive neural organization, without announcing precision or full scale found the middle value of F1 . On HatebaseTweitter, others detailed a large scale F1 score of 0.94, however this is accomplished by joining the disdain and hostile classifications, extraordinarily improving on the errand.
In Table, we see that for most datasets and approaches, the exactness is one-sided towards the larger part class in the preparation information. This proposes the requirement for datasets that are more delegate of genuine information appropriations for future assessment.
Considering the above blended as far as strength assessment results, given potential moral concerns identified with disdain discourse location, we decide in favor alert and settle on interpretability over questionable enhancements for the assessment measurements.
-
-
Interpretation of mSVM.
We examined the top components of the mSVM classifier on the Stormfront dataset. The meta-classifier loads character 4- grams and word unigrams as the most noteworthy supporters of the general score. 4-grams, for example, "jew", "chimp", "mud", "egro" are among the most grounded signs of being disdain. (Note that whitespace adds to character 4-grams.) This class seems to catch the part of a gathering's personality. Word unigrams, for example, "intrusion" and "savagery" contribute exceptionally to despise arrangement, and seem to catch the assault viewpoint. The top word unigrams, 2-grams and 3-grams from the view-classifier aftereffects of each dataset are in Table. We tracked down that the precision of all view-classifiers is something like two percent lower than the meta-classifier. The full correlation between view-classifier and meta-classifier results are given in valuable data Table. We additionally saw as that, albeit three other view- classifiers outflank the word unigram model, the meta- classifier actually loads its score higher than those models, further recommending that it catches an alternate disdain discourse viewpoint.
-
Interpretation of BERT.
Since the BERT model utilizes a self-consideration component, one can envision the terms that the model depends most upon for grouping purposes. We present consideration perceptions from BertViz for the prepared BERT model on the mis-characterized gathering post "I don't think anybody is hinting that we are equivalent to non whites, or that we would disregard white countries." (this post doesn't fulfill the creators' conditions for disdain discourse, however the BERT model grouped it as scornful). We present an itemized consideration loads for every one of the 12 consideration tops of the order token on layer 11 in. In spite of seeming, by all accounts, to be the most useful layer, we see that Layer 11 doesn't give an unambiguous response to why the model named the post as contemptuous; the consideration is disseminated among most words in the sentence, and a large number of the loads with the most consideration don't seem, by all accounts, to be instructive (e.g., we). When researching different layers and different posts, we comparably don't see solid patterns that would empower interpretability. This exhibits the impediment of utilizing profound neural modelseven those with cases of interpretabilitywhen attempting to decipher the choices made. These perceptions are in accordance with earlier work that has viewed consideration signals as uproarious and not really characteristic of term significance. While our methodology can be joined with neural models, it would come to the detriment of expanded model intricacy and decreased interpretability.
-
Error analysis.
To acquire a superior comprehension of our mSVM classifier's slip-ups, we subjectively examine its bogus positive (FP) and bogus negative (FN) tests on the Stormfront dataset. We arranged the misclassified posts dependent on their shared etymological provisions, semantic components, and length. 41% of the posts misclassified as not disdain required encompassing setting to comprehend that the post is disdain discourse. 7% of the FN were implied disdain, making it hard to order, for example, "To be sure, I haven't seen or heard machines assaulting or denying individuals in the roads of Stockholm yet, non-european migrants notwithstanding ". Besides, considering that the between annotator arrangement isn't wonderful in the dataset (earlier work shows that high between annotator understanding for disdain discourse is hard to accomplish, we broke down some marginal cases with the meaning of disdain discourse utilized for explanation. At the point whn physically re-surveying the misclassified posts, we tracked down that the gold mark of the 17% of the FN and 10% of the FP posts don't coordinate with our translation of the post substance. Another serious issue is with posts that are forceful however don't meet the important conditions to be viewed as disdain discourse. These comprise 16% of the FP. At last, short posts (6 or less terms, addressing under 3% of disdain discourse sentences found in the dataset) expanded FP too, happening 7% of the time. The excess misclassified posts were random cases including posts that are snide or figurative
-
Shortcomings and future work
A mission confronted thru computerized hate speech detection systems is the converting of attitudes in the path of topics over the years and historic context. Do not forget the subsequent excerpt of a facebook put up:
the cruel indian savages, whose known rule of struggle, is an undistinguished destruction of every age, sexes and situations
Instinct suggests that this is hate speech; it refers to local americans as cruel indian savages, and dehumanizes them by means of suggesting that they may be inferior. Certainly, the textual content satisfies conditions used in maximum definitions of hate speech. However, this article is surely a quote from the assertion of independence. Given the ancient context of the text, the person who published it is able to now not have intended the dislike speech result, however alternatively intended to quote the historical document for different purposes. This indicates that consumer rationale and context play an important role in hate speech identity.
As every other example, remember the word the nazi enterprise turned into exceptional. this would be considered hate speech because it shows help for a hate institution. However, the nazis organisation turned into super isnt supporting their beliefs however as an alternative commenting on how nicely the organization became organized. In a few contexts, this could no longer be considered hate speech, e. G., if the writer was evaluating organizational effectiveness over the years. The difference in these phrases is subtle, however may be enough to make the difference between hate speech or no longer.
Any other remaining mission is that computerized hate speech detection is a closed-loop machine; individuals are conscious that it's miles taking place, and actively attempt to steer clear of detection. For example, on-line structures eliminated hateful posts from the suspect inside the current new zealand terrorist assault (albeit manually), and implemented guidelines to routinely cast off the content while re-published by others. Customers who favored to spread the hateful messages speedy found approaches to bypass those measures via, as an example, posting the content as images containing the text, in place of the text itself. Although optical individual recognition can be hired to solve the specific hassle, this further demonstrates the difficulty of hate speech detection going forward. It will be a regular conflict between the ones seeking to spread hateful content material and those trying to block it
-
Conclusion
As disdain discourse keeps on being a cultural issue, the requirement for programmed disdain discourse discovery frameworks turns out to be more obvious. We introduced the current methodologies for this undertaking just as another framework that accomplishes sensible exactness. We likewise proposed another methodology that can beat existing frameworks at this undertaking, with the additional advantage of further developed interpretability. Given every one of the difficulties that stay, there is a requirement for more exploration on this issue, including both specialized and pragmatic matters.
-
ACKNOWLEDGMENTS
We thank proff.Jaya Bharathi for reviewing early versions of this paper and for helpful feedback on this work. We also thank the anonymous reviewers for their insightful comments
-
REFERENCES
-
Robertson C, Mele C, Tavernise S. 11 Killed in Synagogue Massacre; Suspect Charged With 29 Counts. 2018;.
-
The New York Times. New Zealand Shooting Live Updates: 49 Are Dead After 2 Mosques Are Hit. 2019;.
-
Hate SpeechABA Legal Fact CheckAmerican Bar Association;. Available
from: https://abalegalfactcheck.com/articles/hate-speech.html.
-
Community Standards;. Available from: https://www.facebook.com/communitystandards/objectiona ble_content.
-
Hate speech policyYouTube Help;. Available from: https://support.google.com/youtube/answer/2801939.
-
Hateful conduct policy;. Available from: https://help.twitter.com/en/rules-and-policies/hateful- conduct-policy.
-
Mondal M, Silva LA, Benevenuto F. A Measurement Study of Hate Speech in Social Media. In: ACM HyperText; 2017.
-
Fortuna P, Nunes S. A Survey on Automatic Detection of Hate Speech in Text. ACM Comput Surv. 2018;51(4):85:185:30.
-
Davidson T, Warmsley D, Macy MW, Weber I. Automated Hate Speech Detection and the Problem of Offensive Language. ICWSM. 2017;.
-
Zimmerman S, Kruschwitz U, Fox C. Improving Hate Speech Detection with Deep Learning Ensembles. In: LREC; 2018.
-
Ross B, Rist M, Carbonell G, Cabrera B, Kurowsky N, Wojatzki
M. Measuring the Reliability of Hate Speech Annotations: The Case of the European Refugee Crisis. In: The 3rd Workshop on Natural Language Processing for Computer-Mediated Communication @ Conference on Natural Language Processing; 2016.
-
Wermiel SJ. The Ongoing Challenge to Define Free Speech. Human Rights Magazine. 2018;43(4):14.
-
View Article
-
Google Scholar
-
-
Nockleby JT. Hate Speech. Encyclopedia of the American Constitution. 2000;3:127779.
-
View Article
-
Google Scholar
-
-
de Gibert O, Perez N, Garcia-Pablos A, Cuadros M. Hate Speech Dataset from a White Supremacy Forum. In: 2nd Workshop on Abusive Language Online @ EMNLP; 2018.
-
Popat K, Mukherjee S, Yates A, Weikum G. DeClarE: Debunking Fake News and False Claims using Evidence-Aware Deep Learning. In: EMNLP; 2018.
-
Hatebase;. Available from: https://hatebase.org/.
-
Waseem Z, Hovy D. Hateful Symbols or Hateful People? Predictive Features for Hate Speech Detection on Twitter. In: SRW@HLT-NAACL; 2016.
-
Waseem Z. Are you a racist or am i seeing things? annotator influence on hate speech detection on twitter. In: Proceedings of the first workshop on NLP and computational social science; 2016. p. 138142.
-
Kumar R, Ojha AK, Malmasi S, Zampieri M. Benchmarking Aggression Identification in Social Media. In: Proceedings of the First Workshop on Trolling, Aggression and Cyberbullying (TRAC-2018). ACL; 2018. p. 111.
-
CodaLabCompetition;. Available from: https://competitions.codalab.org/competitions/19935.
-
Detecting Insults in Social Commentary;. Available from: https://kaggle.com/c/detecting-insults-in-social- commentary.
-
Neuman Y, Assaf D, Cohen Y, Last M, Argamon S, Howard N, et al. Metaphor Identification in Large Texts Corpora. PLoS ONE. 2013;8(4).
-
Salton G, Yang CS, Wong A. A Vector-Space Model for Automatic Indexing. Communications of the ACM. 1975;18(11):613620.
-
Grossman DA, Frieder O. Information Retrieval: Algorithms and Heuristics. Berlin, Heidelberg: Springer-Verlag; 2004.
-
Mikolov T, Sutskever I, Chen K, Corrado GS, Dean J. Distributed Representations of Words and Phrases and their Compositionality. In: Burges CJC, Bottou L, Welling M, Ghahramani Z, Weinberger KQ, editors. Advances in Neural Information Processing Systems 26. Curran Associates, Inc.; 2013. p. 31113119.
-
Devlin J, Chang MW, Lee K, Toutanova K. BERT: Pre-training of Deep Bidirectional Transformers for Language Understanding. arXi:181004805 [cs]. 2018;.
-
Yang Z, Chen W, Wang F, Xu B. Unsupervised Neural Machine Translation with Weight Sharing. In: Proceedings of the 56th Annual Meeting of the Association for Computational Linguistics (Volume 1: Long Papers). Association for Computational Linguistics; 2018. p. 4655. Available from: http://aclweb.org/anthology/P18-1005.
-
Kuncoro A, Dyer C, Hale J, Yogatama D, Clark S, Blunsom P. LSTMs Can Learn Syntax-Sensitive Dependencies Well, But Modeling Structure Makes Them Better. In: Proceedings of the 56th Annual Meeting of the Association for Computational Linguistics (Volume 1: Long Papers). Melbourne, Australia: Association for Computational Linguistics; 2018. p. 14261436. Available from: http://aclweb.org/anthology/P18-1132.
-
Pedregosa F, Varoquaux G, Gramfort A, Michel V, Thirion B, Grisel O, et al. Scikit-learn: Machine Learning in Python. JMLR. 2011;12:28252830.
-
Kim Y. Convolutional Neural Networks for Sentence Classification. In: EMNLP; 2014.
-
Hagen M, Potthast M, Büchner M, Stein B. Webis: An Ensemble for Twitter Sentiment Detection. In: SemEval@NAACL-HLT; 2015.
-
Joulin A, Grave E, Bojanowski P, Mikolov T. Bag of Tricks for Efficient Text Classification. In: Proceedings of the 15th Conference of the European Chapter of the Association for Computational Linguistics: Volume 2, Short Papers. ACL; 2017. p. 427431.
-
33.Zhang Z, Robinson D, Tepper J. Detecting hate speech on twitter using a convolution-gru based deep neural network. In: European Semantic Web Conference. Springer; 2018. p. 745760.
-
Zhao J, Xie X, Xu X, Sun S. Multi-view learning overview: Recent progress and new challenges. Information Fusion. 2017;.
-
Opitz D, Maclin R. Popular ensemble methods: An empirical study. Journal of artificial intelligence research. 1999;11:169 198.
-
Chand N, Mishra P, Krishna CR, Pilli ES, Govil MC. A comparative analysis of SVM and its stacking with other classification algorithm for intrusion detection. In: 2016 International Conference on Advances in Computing, Communication, & Automation (ICACCA)(Spring). IEEE; 2016. p. 16.
-
Dong YS, Han KS. Boosting SVM classifiers by ensemble. In: Special interest tracks and posters of the 14th international conference on World Wide Web. ACM; 2005. p. 10721073.
-
Abdullah A, Veltkamp RC, Wiering MA. Spatial pyramids and two-layer stacking SVM classifiers for image categorization: A comparative study. In: 2009 International Joint Conference on Neural Networks. IEEE; 2009. p. 512.
-
Jain S, Wallace BC. Attention is not Explanation. ArXiv. 2019;abs/1902.10186.
-
Serrano S, Smith NA. Is Attention Interpretable? In: ACL; 2019.
-
Arroyo-Fernández I, Forest D, Torres JM, Carrasco-Ruiz M, Legeleux T, Joannette K. Cyberbullying Detection Task: The EBSI-LIA-UNAM system (ELU) at COLING18 TRAC-1. In: The First Workshop on Trolling, Aggression and Cyberbullying
@ COLING; 2018.
-
Aroyehun ST, Gelbukh A. Aggression Detection in Social Media: Using Deep Neural Networks, Data Augmentation, and Pseudo Labeling. In: Proceedings of the First Workshop on Trolling, Aggression and Cyberbullying (TRAC-2018). Santa Fe, New Mexico, USA: Association for Computational Linguistics; 2018.
p. 9097. Available from: https://www.aclweb.org/anthology/W18-4411.
-
Vig J. Visualizing Attention in Transformer-Based Language Representation Models. arXiv preprint arXiv:190402679. 2019;.
-
Waseem Z. Are You a Racist or Am I Seeing Things? Annotator Influence on Hate Speech Detection on Twitter. In: NLP+CSS @ EMNLP; 2016.